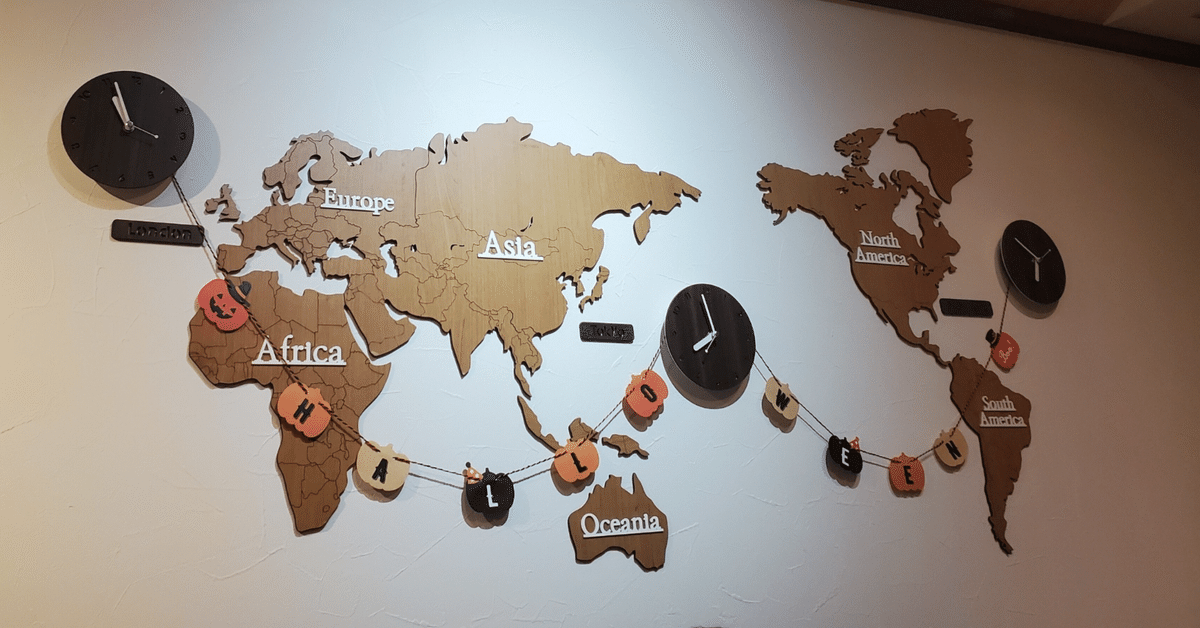
【最適なモデル選択と注意点🌟】『Japanese Foreign Exchange Interventions, 1971-2018:Estimating a Reaction Function Using the Best Proxy』:先行研究解説 No.7💛2023/10/14
Introduction:卒業論文は早めに仕上げたい💛
私もいよいよ卒業論文の執筆に
取りかかる時期がやって参りました👍
何事もアウトプット前提のインプットが
大事であると、noteで毎日発信してきました
これは、どのような内容で
あっても当てはまります👍
論文を一概に読んでも
記憶に残っていなかったり
大切な観点を忘れてしまっていたりしたら
卒業論文の進捗は滞ってしまうと思います
だからこそ、この「note」をフル活用して
卒業論文を1%でも
完成に向けて進めていきたいと思います
私の卒論執筆への軌跡を
どうぞご愛読ください📖
今回の参考文献🔥
今回、読み進めていく論文は
こちらのURLになります👍
『Japanese Foreign Exchange Interventions, 1971-2018: Estimating a Reaction Function Using the Best Proxy』
Takatoshi Ito(a), Tomoyoshi Yabu(b)
(a) School of International and Public Affairs, Columbia University, and GRIPS, Tokyo
(b) Department of Business and Commerce, Keio University
読み終えた先行研究📚
『日本の為替介入の分析』 伊藤隆敏・著
経済研究 Vol.54 No.2 Apr. 2003
『Effects of the Bank of Japan’s intervention on yen/dollar exchange rate volatility』21 November 2004
Toshiaki Watanabe (a), Kimie Harada(b)
『The Effects of Japanese Foreign Exchange Intervention: GARCH Estimation and Change Point Detection』
Eric Hillebrand Gunther Schnabl Discussion
Paper No.6 October 2003
Japanese Foreign Exchange Interventions, 1971-2018: Estimating a Reaction Function Using the Best Proxy
December 12, 2019
Takatoshi Ito(a), Tomoyoshi Yabu(b)
(a) School of International and Public Affairs, Columbia University, and GRIPS, Tokyo
(b) Department of Business and Commerce, Keio University
3. In search of the best proxy:前回のお復習い📝
3.2. Ito and Yabu (2017)
This section first introduces candidate proxies. Then, we will give an overview of the yet to be translated Japanese paper by Ito and Yabu (2017) and review their testing procedure and estimation results.
Moreover, this section establishes that it is important to distinguish transaction-based intervention from settlement-based intervention in the testing procedure.
This has not been discussed at all in the existing literature.
このセクションでは、まず候補となる代理変数(proxies)を紹介します
次に、Ito and Yabu (2017)による未翻訳の日本語論文の概要を示し、そのテスト手順と推定結果をレビューします
さらに、このセクションでは、テストの過程のなかで、取引ベースの介入と決済ベースの介入を区別することが重要であることを確立します
このことは既存の文献ではまったく議論されていません
3.1. Candidates for Proxies
We review the testing procedure and estimation results of Ito and Yabu (2017).
In order to test the accuracy of the proxies, monthly data of the four proxies from April 1991 to March 1999 are tested against actual interventions in the same period.
The disclosed data regarding interventions were observed with daily frequency but have been aggregated to monthly values for the purposes of testing.
この先行研究は、Ito and Yabu(2017)のテスト手順と推定結果をレビューします
代理変数(Proxy)の精度をテストするために、1991年4月から1999年3月までの4つの代理変数の月次データを、同期間の実際の介入に対してテストします
介入に関する開示されたデータは、毎日の頻度で観察されましたが、テストの目的で月次の値に集計されています📝
The sample period ends March 1999, because in April 1999, “MOF FEEF” becomes impossible to adjust due to changes in categorization.
The tests are conducted with the following regression:
1999年4月に区分変更により「財務省手数料」が調整できなくなるため、サンプル期間は1999年3月までとなっている点に留意します
そして、このテストは、次の回帰を使用して実行されます👏
$$
Int_t=\beta_0+\beta_1Proxy_t +u_t\cdot\cdot\cdot(1)
$$
where Intt denotes the transaction-based intervention amount (Intt > 0 implies selling yen, buying dollars) and Proxyt denotes a particular proxy.
Both variables are measured in units of 100 million yen.
The null hypothesis is β0= 0 and β1= 1 .
If the null is not rejected, it implies that a proxy is an unbiased estimator of actual interventions.
Among the proxies that are shown to be unbiased estimators, the best estimator is the one in which R^2 is closest to 1.
ここで、(Int_t)は取引ベースの介入額 (Intt > 0 は円売り、ドル買いを意味します)、(Proxy_t)は特定の代理変数を示します
どちらの変数も億円単位で測定されます
帰無仮説は β0= 0 およびβ1= 1です
帰無仮説が棄却されない場合、代理変数が実際の介入の不偏推定量(unbiased estimator)であることを意味します
不偏推定量であることが示されている代理変数の中で、最良の推定量は、次の推定量です
どの代理変数によって回帰されるモデルの決定係数(R^2)が1に最も近いか、を確かめることでそのモデルの当てはまりの良さが見出せます
Proxies to be tested are (1) Change in Reserve, 3-month T-bills; (2) Change in Reserve, 3-year T-bonds; (3) Change in Reserve, 10-year Tbonds; (4) BOJ EF; (5) MOF FEEF; and (6) Adjusted MOF FEEF. Table 2 shows the estimated results of Eq. (1) with the null hypothesis of H0:β0 = 0 and H0: β1= 1.
代理変数としてテストされる通貨は、次のとおりです
(1) 準備金の変動、3 か月物国庫短期証券
(2) 準備金、3年物Tボンドの変動
(3) 準備金、10 年国債の変動
(4) 日銀EF
(5) 財務省の手数料
(6) 調整されたMOF FEEF
表2は、式(1)の推定結果を示しています
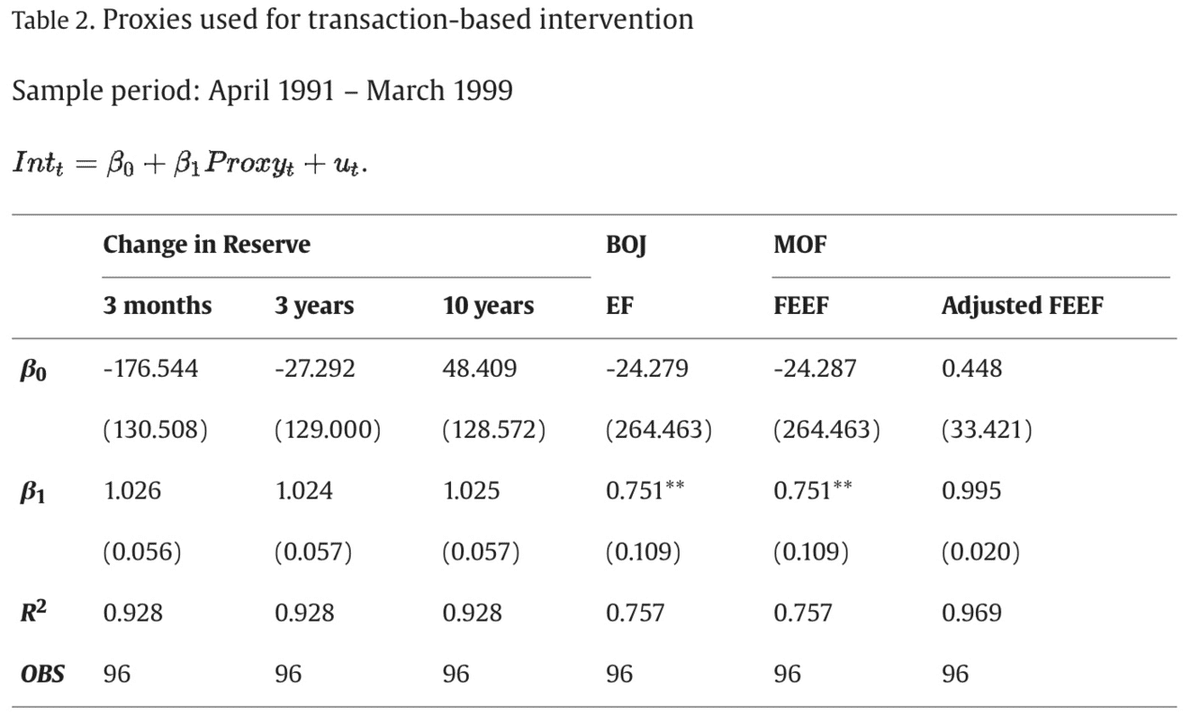
そして、回帰モデル(1)は「H0:β0 = 0 および H0:β1= 1」の帰無仮説という仮説検定を実施します
The null hypothesis of H0: β1= 1 is rejected when we adopt “BOJ EF” or “MOF FEEF.”
Thus, they are not unbiased estimators.
Their coefficients of determination (R^2 ) are low as well.
This is surprising, given that alternatives to “Change in Reserve” were popular among researchers before actual intervention data were made available to the public.
The null hypothesis of an unbiased estimator is not rejected when either “Change in Reserve” with an interest income adjustment or “Adjusted MOF FEEF” is used. The estimated coefficient of β0 is closest to 0 and β1 is closest to 1 when “Adjusted MOF FEEF” is adopted.
Moreover when "Adjusted MOF FEEF” is adopted, R^2 is 0.969 and higher than those with “Change in Reserve” (with any of the three possible interest rate assumptions): 0.928.
Therefore, “Adjusted MOF FEEF” is concluded to be the best proxy for transaction-based interventions.
H0:β1=1 の帰無仮説は「日銀 EF」または「財務省 FEEF」を採用すると棄却されます
したがって、それらは不偏推定量ではありません
モデルの当てはまりの良さを示す決定係数 (R^2) も同様に低いです
実際の介入データが一般に公開される前に「準備金の変動」を代替変数に用いる案が、研究者の間で人気があったことを考えると、これは驚くべきことです
不偏推定量の帰無仮説は「準備金の変動」に金利収入調整を加えた場合でも棄却されません💦
または「調整されたMOF FEEF」が使用されています
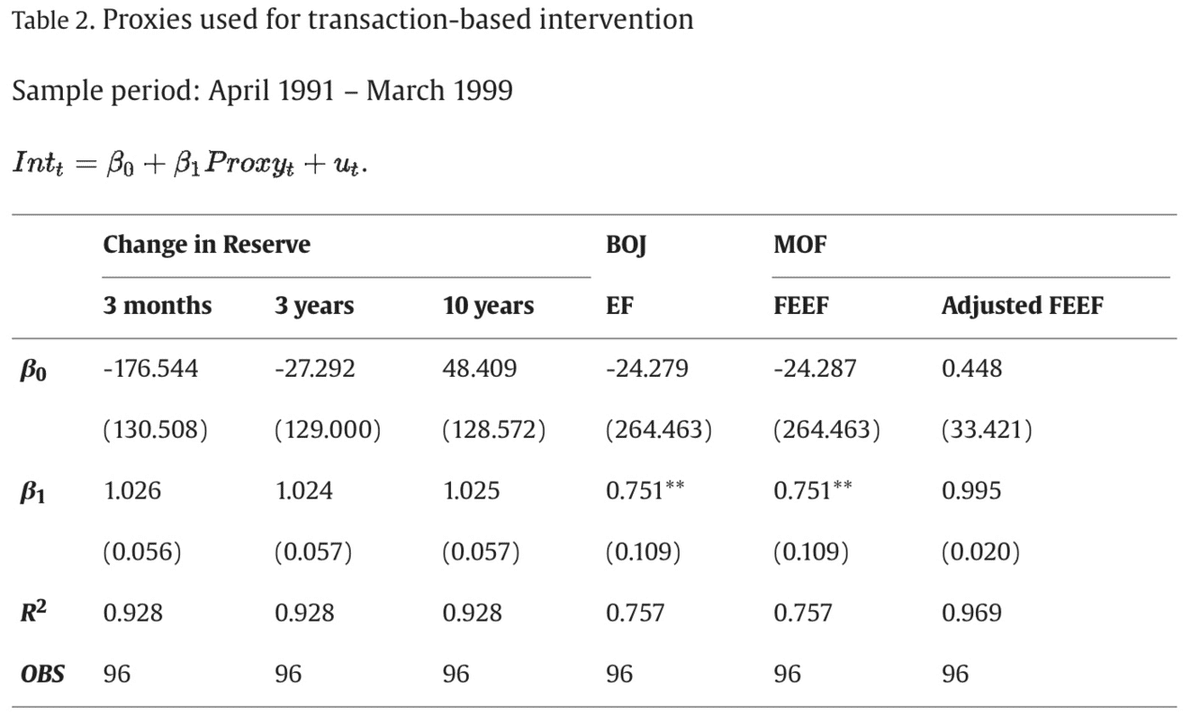
「調整済みMOF FEEF」を採用した場合、推定係数β0は、0に最も近く、β1は1に最も近くなります👏
さらに調整財務省手数料」を採用した場合、R^2 は 0.969 となり「準備金の変動」(3つの可能な金利における仮定のいずれかを使用した上で)の場合の0.928よりも高くなります
したがって「調整財務省手数料」は、取引ベースの介入(transaction-based interventions)に最適な代理変数であるということです
In order to determine the accuracy of the best proxy, Fig. 1 shows the time series of transaction-based intervention amounts (Intt) and the estimated errors of the proxy (Adjusted MOF FEEF), which are defined as:
最適な代理変数の正確さを決定するために、図1に取引ベースの介入量(Intt)とプロキシの推定誤差(調整済み MOF FEEF)の時系列を示します
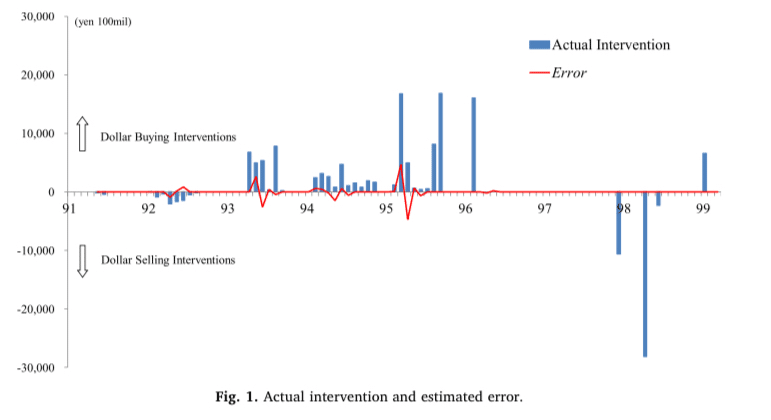
これらは、次のように定義されます
$$
Error_t=Int_t -Proxy_t
$$
The error is small overall; however, there is a negative serial correlation in the error (Errort), meaning that when the error is positive in one month, there is a negative error of a similar amount the following month.
This may suggest some accounting issue: When actual intervention takes place near the end of the month, settlement and booking may spill over into the next month. This may explain the negative correlation.
この分析における全体的に誤差は小さいです
ただし、誤差 (Error_t)には負の系列相関があります
これは、ある月の誤差が正の場合、翌月には同様の量の負の誤差が存在することを意味します
本日の解説は、ここまでとします
このような歴史や先行研究をしっかり理解した上で、卒業論文執筆に取り組んでいきたいです
今回、私が卒業論文執筆において取り上げる
24年ぶりの「円安是正」介入は本当にレアな経済政策
ということを再認識できたような気がします💖
私の研究テーマについて🔖
私は「為替介入の実証分析」をテーマに
卒業論文を執筆しようと考えています📝
日本経済を考えたときに、為替レートによって
貿易取引や経常収支が変化したり
株や証券、債権といった金融資産の収益率が
変化したりと日本経済と為替レートとは
切っても切れない縁があるのです💝
(円💴だけに・・・)
経済ショックによって
為替レートが変化すると
その影響は私たちの生活に大きく影響します
だからこそ、為替レートの安定性を
担保するような為替介入はマクロ経済政策に
おいても非常に重要な意義を持っていると
推測しています
決して学部生が楽して執筆できる
簡単なテーマを選択しているわけでは無いと信じています
ただ、この卒業論文をやり切ることが
私の学生生活の集大成となることは事実なので
最後までコツコツと取り組んで参ります🔥
本日の解説は、以上とします📝
今後も経済学理論集ならびに
社会課題に対する経済学的視点による説明など
有意義な内容を発信できるように
努めてまいりますので
今後とも宜しくお願いします🥺
マガジンのご紹介🔔
こちらのマガジンにて
卒業論文執筆への軌跡
エッセンシャル経済学理論集、ならびに
【国際経済学🌏】の基礎理論をまとめています
今後、さらにコンテンツを拡充できるように努めて参りますので何卒よろしくお願い申し上げます📚
最後までご愛読いただき誠に有難うございました!
あくまで、私の見解や思ったことを
まとめさせていただいてますが
その点に関しまして、ご了承ください🙏
この投稿をみてくださった方が
ほんの小さな事でも学びがあった!
考え方の引き出しが増えた!
読書から学べることが多い!
などなど、プラスの収穫があったのであれば
大変嬉しく思いますし、投稿作成の冥利に尽きます!!
お気軽にコメント、いいね「スキ」💖
そして、お差し支えなければ
フォロー&シェアをお願いしたいです👍
今後とも何卒よろしくお願いいたします!
この記事が参加している募集
この記事が気に入ったらサポートをしてみませんか?