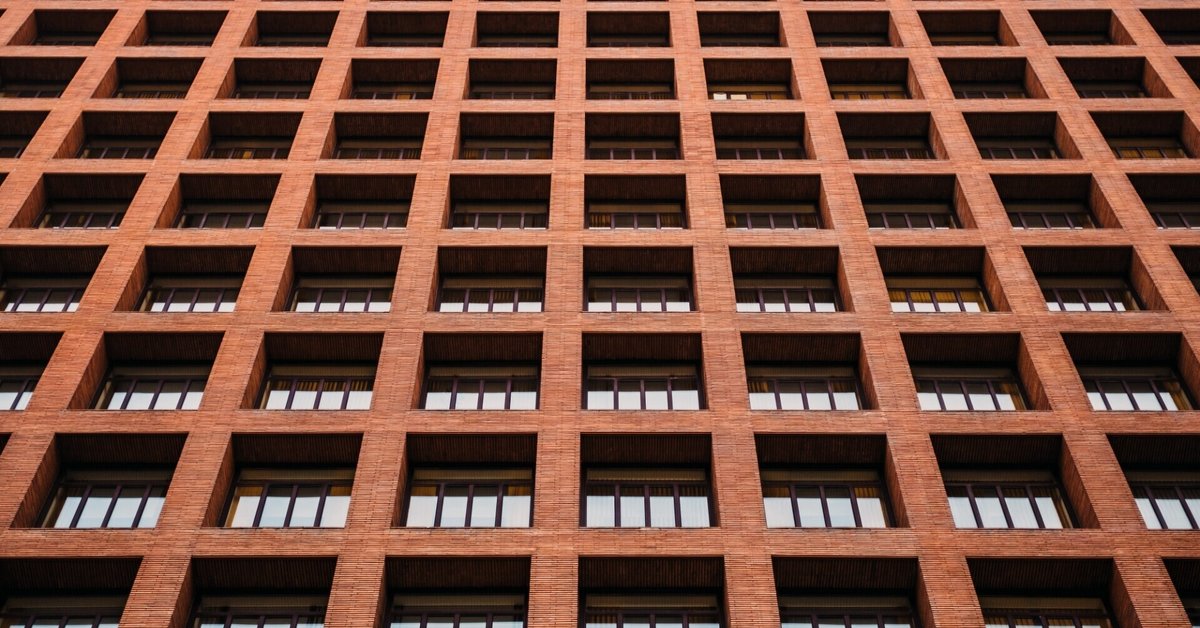
Automatic Sudoku (Number Place) Solver with Digit Recognition and Integer Linear Programming
Photo by Maria Teneva on Unsplash
※本稿はテックブログからの転載です。
Sudoku is a logic-based number placement puzzle that consists of 81 cells which are divided into 9 columns, rows and blocks. The goal of this game is to fill out each cells with numbers 1–9 so that there are no repeating numbers in each row, column and blocks.
In this post, I aim to introduce a digit recognition and integer linear programming based automatic sudoku solver that uses the following: Keras (based on the MNIST database [1]) and OpenCV for digit recognition and PuLP for integer linear programming. Also, I plan on detailing out the integer linear programming part and only lightly touch upon the digit recognition and extraction part (image processing)
[1] The MNIST database is a large database of handwritten digits that is commonly used for training various image processing systems. The database is also widely used for training and testing in the field of machine learning.
The rest of this post is organized as follows:
1.Digit Extraction with OpenCV & Digit Recognition with Keras
2.Formulas and Python3 code with PuLP used for a Sudoku Puzzle Solver
3.Digit placement on the image of a Sudoku puzzle
4.Testing of the developed solver with a sample Sudoku puzzle
Fig1. Concept Image of Automatic Sudoku Solver
1. Digit Extraction with OpenCV & Digit Recognition with Keras
In this section, I explain the overview of image processing for digit recognition. Below, I have laid out a simple description of the sample code.
・Image processing: Gaussian blur, Thresholding, Resizing for prediction with MNIST based digit recognition model
・Digit Extraction: Based on Contours, crop and warp image
・Digit Recognition: MNIST based digit recognition model (As I used an existing trained model, I won’t provide a sample code for digit recognition modeling.)
import cv2
import pulp
import numpy as np
import tensorflow as tf
import matplotlib.pyplot as plt
from PIL import Image
from itertools import product
from tensorflow.keras.models import load_model
from keras.preprocessing import image
MNIST_MODEL_PATH = 'model/mnist_model.h5' # trained model path
SUDOKU_PUZZLE_IMAGE_PATH = 'image/sample_sudoku_puzzle.png' # sample sudoku puzzle image path
Part1. Setup
automatic_sudoku_solver_part_1.py hosted with ❤ by GitHub
def _image_processing_for_extracting_digits(_SUDOKU_PUZZLE_IMAGE_PATH):
# read image as gray scale
_image = cv2.imread(_SUDOKU_PUZZLE_IMAGE_PATH)
_gray_scale_image = cv2.cvtColor(_image, cv2.COLOR_BGR2GRAY)
# applying gaussian blurring to reduce background noise
_gaussian_blurred_gray_scale_image = cv2.GaussianBlur(_gray_scale_image,(1,1),cv2.BORDER_DEFAULT)
# applying inverse binary threshold
_ret, _inverse_binary_threshold_image = cv2.threshold(_gray_scale_image, 180, 255,cv2.THRESH_BINARY_INV)
# applying probabilistic hough transform on binary image
_minLineLength = 100
_maxLineGap = 60
_lines = cv2.HoughLinesP(_inverse_binary_threshold_image, 1, np.pi/180, 100, minLineLength=_minLineLength, maxLineGap=_maxLineGap)
for _line in _lines:
x1,y1,x2,y2 = _line[0]
cv2.line(_image, (x1,y1), (x2,y2), (0,255,0), 2, cv2.LINE_AA)
cv2.imwrite('image/sample_sudoku_puzzle_hough.jpg',_image)
# finding contours
_image_hough = cv2.imread('image/sample_sudoku_puzzle_hough.jpg',0)
_contours, _hierarchy = cv2.findContours(_image_hough, cv2.RETR_TREE, cv2.CHAIN_APPROX_NONE)
_cnt = _contours[0]
_max_area = cv2.contourArea(_cnt)
for _contour in _contours:
if cv2.contourArea(_contour) > _max_area:
_cnt = _contour
_max_area = cv2.contourArea(_contour)
# replaceing the contour by approximate encompassing polygon
_epsilon = 0.01*cv2.arcLength(_cnt, True)
_poly_approx = cv2.approxPolyDP(_cnt, _epsilon, True)
_processed_image = _points_transforming(_inverse_binary_threshold_image, _poly_approx)
return _processed_image
def _points_cordinating(_points):
_cordinated_points = np.zeros((4, 2), dtype = "float32")
_cordinated_points[0] = _points[0]
_cordinated_points[2] = _points[2]
_cordinated_points[3] = _points[3]
_cordinated_points[1] = _points[1]
return _cordinated_points
def _points_transforming(_image, _points):
# obtain cordinated points
_cordinated_points = _points_cordinating(_points)
# tl: top left
# tr: top right
# br: bottom right
# bl: bottom left
(tl, tr, br, bl) = _cordinated_points
# calculate max height
_width_1 = np.sqrt(((br[0]-bl[0])**2) + ((br[1]-bl[1])**2))
_width_2 = np.sqrt(((tr[0]-tl[0])**2) + ((tr[1]-tl[1])**2))
_max_width = max(int(_width_1), int(_width_2))
# calculate max height
_height_1 = np.sqrt(((tr[0]-br[0])**2) + ((tr[1]-br[1])**2))
_height_2 = np.sqrt(((tl[0]-bl[0])**2) + ((tl[1]-bl[1])**2))
_max_height = max(int(_height_1), int(_height_2))
# the set of destination points
_destination_points = np.array([[0, 0], [0, _max_height - 1], [_max_width - 1, _max_height - 1], [_max_width - 1, 0]], dtype = 'float32')
# compute the perspective transform matrix and then apply it
_matrix = cv2.getPerspectiveTransform(_cordinated_points, _destination_points)
_warped_image = cv2.warpPerspective(_image, _matrix, (_max_width, _max_height))
# return the warped image
return _warped_image
def _calculating_cell_positions(_image):
# resize input image for digit recognition (NN model trained with MNIST)
_image = cv2.resize(_image,(252,252))
# computing position of each cell and storing in an array of arrays
_cell_positions = []
_cell_width = _image.shape[1]//9
_cell_height = _image.shape[0]//9
# set start points
x1,x2,y1,y2 = 0,0,0,0
# append each cell position
for i in range(9):
y2 = y1 + _cell_height
x1 = 0
for j in range(9):
x2 = x1 + _cell_width
_current_cell = [x1, x2, y1, y2]
_cell_positions.append(_current_cell)
x1 = x2
y1 = y2
return _cell_positions
def _digit_prediction_with_NN_model(_cell, _image):
# resize image find contours
_image = cv2.resize(_image, (252,252))
_image = _image[_cell[2]+2:_cell[3]-3, _cell[0]+2:_cell[1]-3]
_contours, _hierarchy = cv2.findContours(_image.copy(), cv2.RETR_TREE, cv2.CHAIN_APPROX_SIMPLE)
# calculate positions of each image
_positions = []
if len(_contours) != 0:
for _contour in _contours:
x, y, w, h = cv2.boundingRect(_contour)
if (w < 15 and x > 2) and (h < 25 and y > 2):
_positions.append((x, y, x+w, y+h))
break
# prediction
if _positions != []:
_croped_image = cv2.resize(_image, (28,28))
_croped_image = _croped_image.reshape(1,28,28,1)
_croped_image = tf.cast(_croped_image, tf.float32)
_result = _predict_digit(_croped_image)
else:
_result = 0
return _result
def _extract_digits(_image):
# set list
_digits = []
_cells = _calculating_cell_positions(_image)
for _cell in range(len(_cells)):
_prediction_result = _digit_prediction_with_NN_model(_cells[_cell], _image)
_digits.append(_prediction_result)
_results = [_digits[i:i+9] for i in range(0, len(_digits), 9)]
return _results
def _predict_digit(_image):
_mnist_model = load_model(MNIST_MODEL_PATH)
_prediction_result = _mnist_model.predict_classes(_image)
return _prediction_result[0]
Part2. Function for Digit Extraction and Recognition
automatic_sudoku_solver_part_2.py hosted with ❤ by GitHub
2. Formulas and Python3 code with PuLP used for a Sudoku Puzzle Solver
Unlike typical integer linear programming problems, there is no objective function in sudoku puzzles. In this case, we just find a feasible answer which satisfies some given constraints.
The constraints to solving a Sudoku puzzle is that each row, column, and blocks is filled with an integer between 1–9 and that no duplicates exists within each row, column and block. Fig2 shows constraints of sudoku puzzles and we use parameter x with i for columns, j for rows and k for values. In other words, x _(i, j, k) assumes the value of 1, if element (i, j) of the sudoku matrix contains k, and 0 otherwise.
Fig2. Formula of Sudoku Puzzle
(1) the constraint on columns
(2) the constraint on rows
(3) the constraint on 3 x 3 block
(4) assures that all the cells of the Sudoku puzzle is filled
(5) means that where the corresponding sudoku matrix are assumed to equal one.
Based on the above formulas , I wrote a sudoku solving function with PuLP.
def _sudoku_solver_with_pulp(_board):
problem = pulp.LpProblem()
line = list(range(9))
x = [[[pulp.LpVariable(f'x{i}{j}{k}', cat='Binary') for k in line] for j in line] for i in line]
# constraints
for i, j in product(line, line):
if _board[i][j] > 0:
problem += x[i][j][_board[i][j]-1] == 1
for j, k in product(line, line):
problem += pulp.lpSum(x[i][j][k] for i in line) == 1
for k, i in product(line, line):
problem += pulp.lpSum(x[i][j][k] for j in line) == 1
for i, j in product(line, line):
problem += pulp.lpSum(x[i][j][k] for k in line) == 1
_3x3_square = [[ii, ii+1, ii+2] for ii in [0, 3, 6]]
for a, b in product(_3x3_square, _3x3_square):
for k in line:
problem += pulp.lpSum(x[i][j][k] for i in a for j in b) == 1
# calculate
problem.solve()
# make result board
_result = _board.copy()
for i, j, k in product(line, line, line):
if x[i][j][k].value() == 1:
_result[i][j] = k + 1
return _result
Part3. Function for Solving Sudoku Puzzles
automatic_sudoku_solver_part_3.py hosted with ❤ by GitHub
4. Digit placement on the image of a Sudoku puzzle
As in section 1, description for details of writing calculated results is omitted.
def _finding_empty_cell(_cell, _image):
# prepare
_positions = []
_image = cv2.resize(_image,(252,252))
_image = _image[_cell[2]+2:_cell[3]-3,_cell[0]+2:_cell[1]-3]
_contours, _hierarchy = cv2.findContours(_image.copy(), cv2.RETR_TREE, cv2.CHAIN_APPROX_SIMPLE)
# calculate posisions
if len(_contours) != 0:
for _contour in _contours:
x, y, w, h = cv2.boundingRect(_contour)
if (w < 15 and x > 2) and (h < 25 and y > 2):
_positions.append((x, y, x+w, y+h))
break
if _positions == []:
return _positions
else:
return 0
def _place_digit_on_empty_cell(_image):
# read image for placing
_image_for_placing = cv2.imread(SUDOKU_PUZZLE_IMAGE_PATH)
_image_for_placing = cv2.cvtColor(_image_for_placing, cv2.COLOR_BGR2GRAY)
_image_for_placing = cv2.resize(_image_for_placing,(252,252))
# resize image
_image = cv2.resize(_image,(252,252))
_cells = _calculating_cell_positions(_image)
n = 9
_reshaped_cell = [_cells[i:i+n] for i in range(0, len(_cells), n)]
_sudoku_digits = _extract_digits(_image)
_answer_digits = _sudoku_solver_with_pulp(_sudoku_digits)
for i in range(len(_reshaped_cell)):
for j in range(len(_reshaped_cell[i])):
_posisions = _finding_empty_cell(_reshaped_cell[i][j], _image)
_digit_text = _answer_digits[i][j]
if _posisions == []:
cv2.putText(_image_for_placing, str(_digit_text), ((_reshaped_cell[i][j][0]+8), (_reshaped_cell[i][j][2]+19)), cv2.FONT_HERSHEY_SIMPLEX, 0.7, (0, 0, 0), 2, cv2.LINE_AA)
else:
continue
cv2.imwrite('image/sample_sudoku_puzzle_answer.jpg', _image_for_placing)
plt.imshow(_image_for_placing, cmap='gray')
return _image_for_placing
Part4. Function for Placing Digits on Image
automatic_sudoku_solver_part_4.py hosted with ❤ by GitHub
5. Testing of the developed solver with a sample Sudoku puzzle
In this section, I will test the solver, which was built using functions outlined in Section1~4, on a sample sudoku puzzle. Fig3 shows the sample Sudoku puzzle that I used for testing. Fig4, Fig5, Fig6 shows the code on Jupyter Notebook that I used for recognizing digits from the image of a sudoku puzzle, solving the sudoku puzzle and writing in the calculated results on the original image of the puzzle respectively.
Fig3. A Sample Sudoku Puzzle
Fig4. Test of Digit Recognition
Fig5. Test of Sudoku Solver with extracted digits from a sample sudoku puzzle
Fig6. Test of Digit Placement
Fig7. Output Results
Closing Remarks
In this post, I introduced an automatic Sudoku solver using digit recognition and integer linear programming, However, this is one of many methods for building a sudoku solver and I only chose it from the purpose of mathematical optimization. Hence, I recommend you also implementing other methods for building a sudoku solver!
この記事が気に入ったらサポートをしてみませんか?