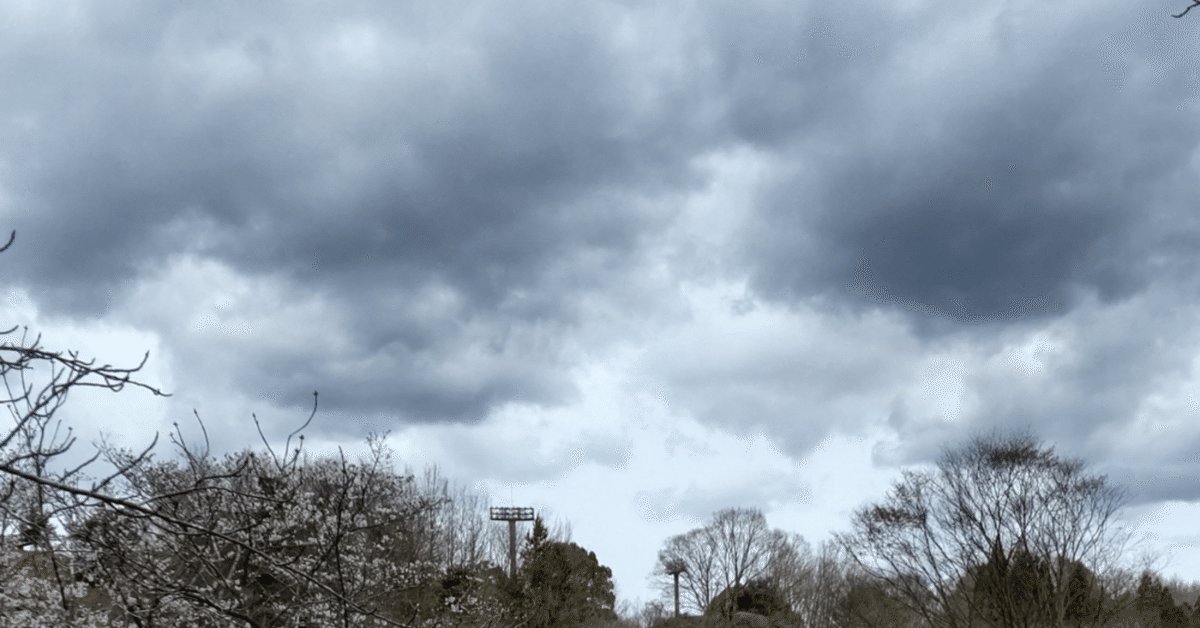
生成AI近況分析:変わりつつあるITハードウェアベンダのビジネス戦略
さて生成AIに関係するようになってから、一年が経過した。表向きはGoogleやMicrosoftのようにGAFAMと呼ばれる5社とOracleがNVIDIA GPUを買いまくって話題になっているけど、その裏でHPEやDellは随分と様変わりしてきた。
一年前は「我々も大規模システムを次々と受注できるかもしれない」という夢が膨らんだけれども、結局のところはマッキンゼーなどのコンサルティング企業やIDC/Gartnerといったコンピュータシステム系な調査会社の予想が正しかった。
最近は予想通り、「推論(生成)案件を数多く受注して将来的な全社売上に期待したい」という流れが強まっている。今回は決算報告に目を通す機会があったので、生成AI関連部分を抽出させて頂いた。
どー見ても、やっぱり「高リスクなことには手を出さず、それでも楽してボロ儲け」というのは無理そうだ。当たり前と言えば、当たり前の話ですな。
今は独り勝ちするNVIDIA
世間的にはNVIDIAが先月発表した四半期決算が注目されている。「これだけビジネス用にGPUが売れれば、さぞやそれを利用したシステム関連のハードウェア、ソフトウェア、サービスの売上拡大が期待されるだろうという構図だ。
427%… そりゃ誰もが期待したくなるだろう。しかし実際のところ、他のIT企業の業績は爆上がっていない。せいぜいNVIDIA GPUを搭載したシステムを販売するSupermicroが前年同期比2倍の全社売上を記録している程度だ。
もちろんGPUだけでは役に立たないから、コンピュータシステムに組み込んでから販売される。だからGPU売上とハードウェアシステム、ソフトウェア、サービスの売上は遅れることになる。しかしそれにしても、これは少しばかり遅れたという程度では無さそうに見える。
そのカラクリは、NVIDIAが販売しているのがGAFAMとOracle中心であることに拠る。これらの大企業は将来のために投資しているので、その成果は数年後に反映されることになる。
おまけに… 例えばMetaのLlama 2を開発したシステムでは、NVIDIAだけではなくてPure Storageの大規模受注が話題となった。しかしLlama 3では、Supermicroに取って代わられてしまった。
いやハードウェア部分はSupermicroだけれども、ファイルシステム部分はMeta自製である。これだけ案件規模が大きければ、汎用品ではなくて案件向けに自製した方が成果を期待できるようになって来る。
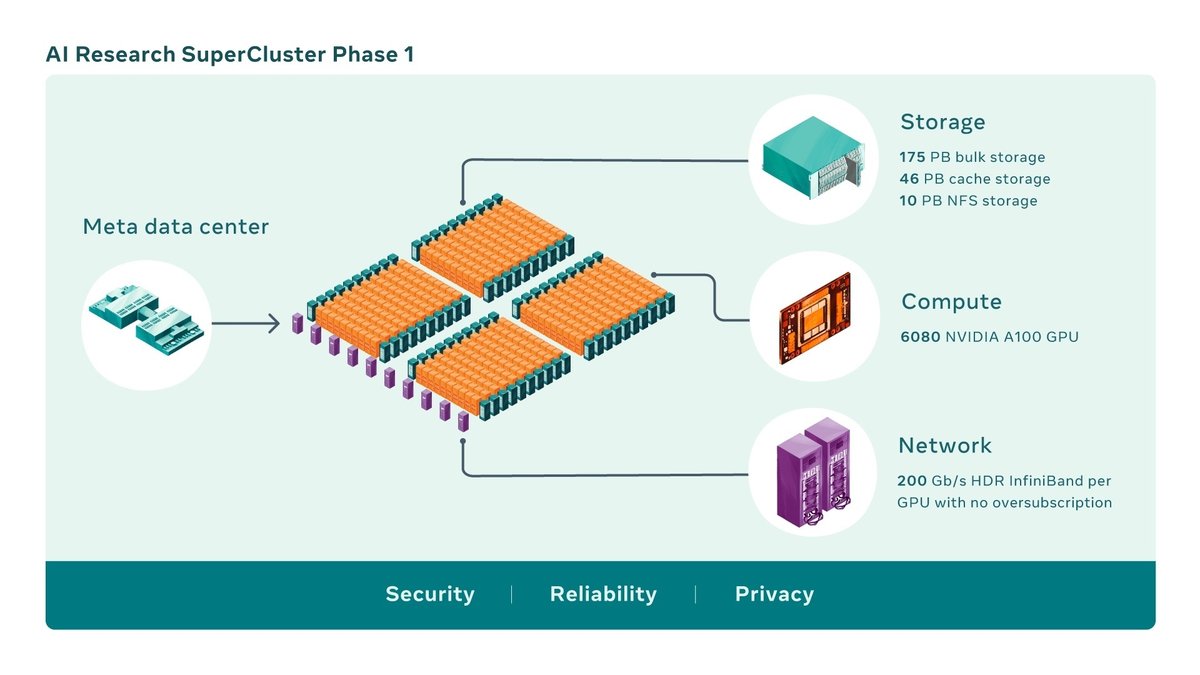
さてそれではPure Storageはどうしているかというと、最近はLLM… じゃなくてLVMスタートアップ(ベンチャー)企業の株を購入して協業体制を構築している。GAFAMは独自路線を突き進むようだし、大手企業でも大規模GPUクラスタシステムを導入するところは多くない。そりゃ生成AIというものはビジネスを助けてくれるアシスタントして役立ってくれるけれども、無ければ他社競合で生き残れないという訳ではない。
数十年前にOfficeを構成するドキュメント作成ツールやスプレッドシートが一気に普及したけれども、現段階の生成AIにそこまでの影響力はない。だから次に紹介する四半期決算の説明会のように、調査会社などと一致したトーンの説明内容に変わって来ている。
各社の決算コメント
と、いう訳で、ハードウェア系企業の四半期決算が終わったので、生成AIに関する部分を抽出してみた。明らかに一年前とはトーンが変わっている。
Pure Storage: AI学習環境一辺倒から推論 (生成) 環境へ対象拡大
Pure Storageにとって生成AIビジネスは、現時点で三分野が存在
(1) Of greatest interest to the media and financial analysts has been the high-performance data storage market for large public or private GPU farms.
(2) A second opportunity is providing specialized storage for enterprise inference engine or RAG environments.
(3) The third opportunity, which we believe to be the largest in the long term, is upgrading all enterprise storage to perform as a storage cloud, simplifying data access and management, and eliminating data silos, enabling easier data access for Al.
顧客のAI投資は始まったばかりとの認識: "Also, while storage spending for Al is still in its early stages, we believe that we are well positioned as demand for data storage accelerates."
3つ目の分野は、現時点では “将来的” な話: “I do think Al has caused customers to take a second look as to how they are going to be spending their money this year. But overall, I would say it's a modest recovery from last year, but I haven't seen a big inflection point." "Data stored on widely diverse platforms, with different operating and management systems, which are siloed and individually managed are unable to feed real-time data to Al inference engines."
AI学習環境関連の受注見込みは昨年想定 (売上の10%以上)を下回る見通し: “That being said, we think that, probably in the past 12 months, about $1 billion was spent on storage specifically tied to Al training environments."
重要なのはAI学習環境向けデータ保管庫の視点ではなく、推論 (生成)も含めたあらゆるAI環境で必要となるデータをスムーズに提供できること: "Upgrade the arrays that you have in place and make them available and accessible to your Al application inference environment. It's a strategy that costs less, it's a strategy that enables more access to real-time data, and it's a strategy that at the same time simplifies their environment."
金融、エネルギー、ライフサイエンス等が当面の有望分野: “Their accelerated environment for both large language models and inferencing delivers top-tier Al infrastructure and training solutions for their financial services, energy and life sciences customers."
HPE:Azure Open Alサービスとの連携などで対応サービスを強化
推論 (生成)を中心としたAIシステムの需要と技術ノウハウ: “Our leadership in Al at scale and across the entire Al life cycle, from training to fine-tuning to inferencing, continues to attract new customers." "HPE benefits from a strong ecosystem of Al partners, including NVIDIA. We introduced co-engineered enterprise solutions with NVIDIA last year to streamline the model development process, as well as to enable enterprises to fine-tune large language models with their private data to accelerate inferencing."
Azure OpenAIサービスとの連携: "For example, in partnership with Microsoft, we are extending the Azure Al platform to HPE Infrastructure, which provides Microsoft with additional capacity to serve even more customers, including OpenAl."
事例: "For example, [QBox] (ph), a facial and image recognition company in Korea, is developing new generative Al models using HPE Al systems to enhance identity verifications at locations such as the Incheon International Airport in South Korea." "JT Group, based in Japan, operates a pharmaceutical business and is planning to use our HPE AI systems to support Al model training and simulations to accelerate drug discovery."
HPEもNetAppと同じく始まったばかりとの見識: "We remain in the very early days of Al, yet it is already driving strong interest, pipeline, orders, and revenue across our portfolio from service to storage to services to financing."
汎用サーバであるGen 11でAI推論ニーズによる購入が進行: "We are encouraged that our Gen11 pipeline is starting to include Al inferencing activity and enterprise applications, and we see more evidence of adoption in the enterprise in Q2."
生成AIの心臓部はファイルストレージが主役: "Storage ARR growth of over 50% year-over-year offers early confidence into the migration. The second transition is from block storage to file storage driven by Al. While early, this is also on the right trajectory. Our new file offerings plus the sales force investment Antonio mentioned tripled our pipeline of file storage deals sequentially in Q2."
Dell同様、HPEもAIシステムには利益マージン課題あり: "While the rising Al systems mix is a gross margin headwind, we are balancing this with higher-margin services revenue, improving scale and cost discipline."
Dell: 利益率の低さゆえか、 将来展望 (期待) の説明が中心
学習対応の8GPU搭載サーバ以外でも需要が高いとのこと(汎用サーバに搭載可能なGPUレベルのLLM推論 (生成)も需要ありとのこと): “As we said in our prepared remarks, demand for our XE9680, that's our 16G and first-to-market purpose-built Al server with eight NVIDIA H100 or A100 GPUs has been very good, but we're also seeing demand across our portfolio. It's not simply the specialized eight-way GPU servers that can run Al, not everything needs billions of parameters.
"全サーバレベルで需要があるとは言え、需要そのものは全社売上に寄与しないレベル。 「将来が期待できる」という段階: “So, net look, we look at it and say. Al is going to drive demand for our business. It's going to drive demand for our business on-premise and at the edge, which is incremental growth and profitability for us. I just caution as we said in our prepared remarks, that we are early in the demand cycle and it's going to take time for that to translate to the P&L. Excitement for Al applications is ahead of GPU supply right now and Al-optimized servers are still a very small part of our overall server mix. So, ultimately to see a full recovery in our server business, it's going to require mainstream service to accelerate as well."
COOとしては、「顧客はまだデータ活用方法を模索中」との見解: “And then where all the buzz is, is on generative Al and large language models. And it's an incredible opportunity. Demand, as Chuck referred to, is been great. I think that continues because quite frankly what customers are trying to do is to figure out how to use their data with their business context to get better business outcomes and greater insight to their customers and to their business."
AIスパコン首位のHPEには若干遅れたものの、COO等の幹部が当初期待よりも案件規模が小さいことに気づきつつある様子: “And while there's a lot of discussion around these large generalized Al models, we think the more specific opportunity is around domain-specific and process-specific generative Al, where customers can use their own data. The datasets tend to be smaller. Those datasets then can be trained more quickly and they can use their business context to help them inform and run their businesses better. We think that's the opportunity in front of us."
案件規模は小さくても導入容易になればDC外のエッジで幅広く利用されると予想(期待): "And that's quite honestly why we announced Project Helix last week with NVIDIA: an opportunity to take enterprise Al at scale, make it easy to deploy, easy to design, easy to put and
install, and use pre-trained models, tuned models and be able to drive inference out in the data center and at the edge. So, we're pretty excited about the opportunities in front of us."AI向けストレージ(データ)需要はAIサーバに遅れて生じる見し: "Storage, it's a slightly different story."
NetApp: NetAppも推論 (生成) 環境向けビジネスを強化
学習、 推論 (生成)、 モデル修正まで幅広くカバーする方針 (FlexPodは推論用AIへ): “Customers choose NetApp to support them at every phase of the Al lifecycle." "In conjunction with Cisco, we updated FlexPod Al reference architectures to support the NVIDIA AI enterprise software platform, giving customers an end-to-end blueprint to efficiently design, deploy, and operate Al infrastructure."
学習環境、データレイク、推論(生成)用RAGまで幅広く受注: "We've had strong wins across multiple elements of the Al opportunity, from data lakes to model training to rag on our customers' installed data, and we see that across multiple verticals." "I think on the Al side, listen, we are the installed incumbent for the world's largest unstructured data set, and we are helping customers unify those data sets, building data lakes."
事例(大手ガス企業の学習環境から各部門のRAG付き生成AI環境を受注): “And then we talked about the success we had at one of the world's largest oil and gas companies where we not only built their supercomputer for a trillion-parameter large language model, but we are also the backbone for all of their RAG at their Al center of excellence."
50を超えるAI案件を受注。 ただし売上金額に影響するような規模になるのは2025年以降の見通し(まだストレージ活用は初期段階との評価): "Listen, broadly speaking, we had about more than 50 Al wins in Q4 across all elements of the Al landscape I talked about, both in data foundations like data lakes as well as model training and inferencing across all of the geographies. I would tell you that in the Al market, the ramp on Al servers will be much ahead of storage because what clients are doing is they're building new computing stacks but using their existing data. And so we expect that over time, there will be a lot more data created and unified to continue to feed the model. But at this stage, we are in proof of concepts. We think that there's a strong opportunity over time for us and all of the Al growth is factored into our guidance for next year."
ファイル型SDSを通じたパブクラとの協業: "And then finally, Al. This is the opportunity that will become much more meaningful over time. We are well-positioned with the huge install base of unstructured data, which is the fuel for GenAl. And we are focused on helping customers do in-place RAG and inferencing of that data. Second, our unified data storage systems with integrated data services provide an ideal foundation for customers looking to build a data lake or model training landscapes. And then our hybrid cloud architecture gives us a unique place in the environment, allowing customers to use public cloud Al platforms such as Vertex from Google or Bedrock from AWS with their on-premises data and be able to scale inferencing to where every piece of customer's data and IT landscape exists."
しめくくり: 生成AIは実用化が進行中
さて一年が経過して、ハードウェア関係者による生成AIへの認識は大きく変化した。コンサルティング企業や調査会社の予想データも発表され、それは大手企業が大規模GPU学習クラスタの構築に突進することはなく、まずは現場での推論(生成)環境の導入が進むというものであった。(一番の成長が期待できるのはサービス部分)
そんな訳で、企業内では織田病院の事例のように、既存システムに少しばかり追加投資が追加される形で、生成AIによる支援機能が実装されていくことになりそうだ。
こういった流れは、おそらく今後は加速していくことになるかと思う。なぜならSI系ベンダはハードウェア企業よりも早く気付いているだろうし、今は生成AIにデータを蓄積して顧客提案に活用するという方法が存在する。
100%完璧な提案でなくても、つまり顧客に取って手間や費用をかけるだけの価値のある提案ができれば良いのだ。さて誰が生成AIを活用して、推論(生成)環境への導入案件を受注増加させて行くか。
他人事のように言ってはいけないのかもしれないけれども、なかなか興味深い状況である。
それでは今回は、この辺で。ではまた。
------
記事作成:小野谷静(オノセー)
この記事が気に入ったらサポートをしてみませんか?