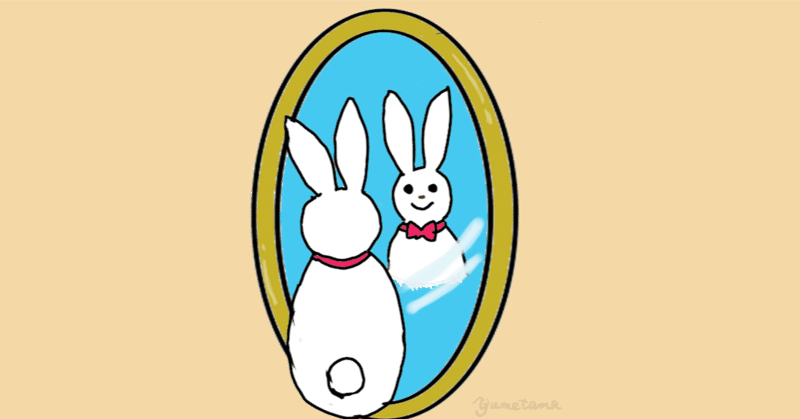
LLM(AI)は自己認識ができるか? 意識をもっているのか? 予備知識編
「ミラー自己認識テスト」「インテリジェンスの進化」「機械学習と進化アルゴリズム」について辞書的な生成をしました。
いつもどおり、以下の解説は、下記のGPT4で生成しています。
なお翻訳は、GPT4はなぜか最近バグるので、Claudeにしてもらいました。
ミラー自己認識テスト
序論
ミラー自己認識テスト (MSR テスト) は、動物と人間の乳幼児における自己意識を評価するために心理学で開発された行動学的手法です。これは、個体がミラー内の自分の姿を認識できるかどうかに基づいています。このような能力を持つことは、自己意識や高度な認知プロセスの指標とみなされており、心理学、動物行動学、認知科学の分野で関心が寄せられています。
定義と説明
ミラー自己認識テストでは、動物や人間の乳幼児に刺激のない染料やシールを、鏡を使わないと見えない場所に付けます。その後、被験体にミラーを提示し、その反応を観察します。被験体がミラー内の自分の体の標識に触れた場合、それは自分の姿を認識しているということを示唆します。
歴史
MSR テストは、1970年に心理学者のゴードン・ガラップ Jr.によって初めて考案されました。ガラップはチンパンジーに対してこのテストを行い、彼らが自分の姿を認識できることを発見しました。その後、このテストは他の大型類人猿、イルカ、ゾウ、さらには一部の鳥類にも適用されてきました。また、人間の乳幼児における自己意識の発達を研究するためにも使われています。
概念と原理
MSR テストの核となる原理は、ミラー内の自分の姿を認識するには、自己概念を形成し、自己と他者を区別する能力などの一定レベルの認知的精緻さが必要だということです。このテストは言語を必要とせずに、これらの認知能力の有無を調べることができるため、さまざまな種に適用可能です。
意義と応用
MSR テストの意義は、異なる種の動物の認知能力、特に自己意識の理解に対する洞察を提供することにあります。これらの知見は、動物の権利、保護、知性の進化など、さまざまな分野に応用されます。人間の乳幼児に対しては、自己意識の発達とその他の認知的milestone との関係を理解する上で重要な役割を果たします。
関連トピックと説明
心の理論: 自己と他者の心的状態(信念、欲求、意図)を区別する能力。
認知動物学: 動物の認知プロセスを研究する動物行動学の分野。MSR テストはこの分野で自己意識を評価する手段の1つです。
発達心理学: 人間の心理的発達過程を研究する分野。MSR テストは、乳幼児における自己意識の発達過程を調べるのに用いられます。
動物の認知: 動物の精神能力を探求する分野。MSR テストは、特に自己意識に関する動物の認知能力の理解を深める上で重要な役割を果たしてきました。
主要な研究者
ゴードン・ガラップ Jr.: MSR テストを開発し、チンパンジーでの初期実験を行った心理学者。
ダイアナ・ライス: イルカの鏡自己認識に関する研究で知られる心理学者。
フランス・ドゥ・ワール: 類人猿の認知能力、とりわけ鏡自己認識能力について extensively 研究した霊長類学者。
現状と今後の展望
MSR テストは、自己意識の研究に有用な手法として継続的に活用されています。ただし、テスト結果の解釈や、自己意識の指標としてのミラー自己認識の妥当性については、議論が続いています。今後の研究では、自己意識を評価する新たな手法の開発や、ミラー自己認識の神経学的基盤の解明など、さらなる探究が期待されます。
結論
ミラー自己認識テストは、種を超えた自己意識の研究における画期的な手法として位置づけられています。その簡便さと非言語的アプローチにより、動物や乳幼児の認知世界に迫ることができ、意識の本質や知性の進化に関する貴重な洞察を提供してきました。
次世代のための関連トピックは以下のようなものが考えられます:
動物の意識
乳幼児期の認知発達
比較認知
知性の進化
心理学における自己概念
オリジナル英語バージョン
Mirror Self-Recognition Test
Introduction
The mirror self-recognition test (MSR test) is a behavioral technique developed in psychology to assess self-awareness in animals and human infants. It is based on whether an individual can recognize itself in a mirror. The ability to do so is considered an indicator of advanced cognitive processes such as self-awareness and is a topic of interest in the fields of psychology, ethology, and cognitive science.
Definition and Description
The mirror self-recognition test involves marking an animal or a human infant with a non-irritating dye or sticker in a place that they cannot see without a mirror. The subject is then exposed to a mirror and observed. If the subject touches the mark on their body while looking in the mirror, it suggests that they recognize the reflection as an image of themselves rather than another individual.
History
The MSR test was first designed by psychologist Gordon Gallup Jr. in 1970. Gallup conducted the test on chimpanzees and found that they were able to recognize themselves in the mirror. Since then, the test has been applied to various other species, including other great apes, dolphins, elephants, and even some birds. It has also been used to study the development of self-awareness in human infants.
Concepts and Principles
The core principle behind the MSR test is that recognizing oneself in a mirror requires a certain level of cognitive sophistication, including the ability to form a mental representation of oneself (self-concept) and to distinguish between oneself and others. The test examines the presence of these cognitive abilities without relying on language, making it suitable for use across different species.
Significance and Applications
The significance of the MSR test lies in its ability to provide insights into the cognitive abilities of different species, including the understanding of self. The implications of these findings extend to areas such as animal rights, conservation, and the evolution of intelligence. In human infants, it helps in understanding the development of self-awareness and its relation to other cognitive milestones.
Related Topics with explanations
Theory of Mind: This is the ability to attribute mental states to oneself and to others, understanding that others have beliefs, desires, and intentions that are different from one's own.
Cognitive Ethology: A branch of ethology that focuses on the study of cognitive processes in animals. The MSR test is one of the tools used in this field to assess self-awareness.
Developmental Psychology: This field studies the psychological development of humans throughout their lives. The MSR test is used to study when and how self-awareness develops in infants.
Animal Cognition: This field explores the mental capacities of animals. The MSR test has been crucial in advancing the understanding of animal cognition, particularly in relation to self-awareness.
Key Figures or Contributors
Gordon Gallup Jr.: The psychologist who developed the MSR test and conducted the initial experiments on chimpanzees.
Diana Reiss: A psychologist known for her work on mirror self-recognition in dolphins.
Frans de Waal: A primatologist who has extensively studied the cognitive abilities of primates, including their ability to recognize themselves in mirrors.
Current Status and Future Directions
The MSR test continues to be a useful tool in the study of self-awareness. There is ongoing debate regarding the interpretation of the test results and whether passing the test is the only indicator of self-awareness. Future research might focus on developing additional methods to assess self-awareness and exploring the neurological basis of mirror self-recognition.
Conclusion
The mirror self-recognition test remains a landmark method for investigating self-awareness across species. Its simplicity and nonverbal approach allow scientists to peer into the cognitive worlds of animals and young children, providing valuable insights into the nature of consciousness and the evolution of intelligence.
Related topics for the next generation might include:
Animal Consciousness
Cognitive Development in Infancy
Comparative Cognition
Evolution of Intelligence
Self-Concept in Psychology
インテリジェンスの進化
序論
インテリジェンスの進化とは、知的行動が進化の時間スケールにおいてどのように発展してきたかを指すプロセスです。このトピックは生物学、人類学から心理学、人工知能に至るまで幅広い分野を包含し、異なる生物、特に人間を含む中で、認知能力がどのように出現し、多様化してきたかを理解しようとします。
定義と説明
インテリジェンスは、学習、推論、複雑なアイディアの理解、そして環境への適応能力として広く定義されます。進化の文脈では、インテリジェンスは特定の生態問題を解決するために自然選択によって形成された認知特性のセットと見なされます。インテリジェンスの進化は、これらの特性の歴史的発展、それらの複雑さを推進する要因、および種を横断するそれらの異なる表現を研究します。
歴史
インテリジェンスの概念は何世紀にもわたって議論されてきましたが、その進化の体系的な研究はチャールズ・ダーウィンの自然選択と人類の系譜に関する業績に始まります。それ以来、インテリジェンスの出現を説明するために数多くの理論が提唱されており、その中には複雑な社会的相互作用が高度な認知能力の発展を促進したとする社会的インテリジェンス仮説も含まれます。
概念と原則
インテリジェンスの進化を研究する上で中心となる考え方は、インテリジェンスは単一の特性ではなく、異なる種において様々な程度で進化した認知プロセスの集合であるというものです。主要な概念には、脳と体の比率(脳化指数)、道具の使用、問題解決能力、言語、文化などがあります。これらの概念は動物界における認知能力を比較・対照するため、そして進化史における知的行動の系統をたどるために使用されます。
重要性と応用
インテリジェンスの進化を理解することは、多数の分野において深い意味を持ちます。生物学や人類学では、人類の起源と私たちの認知能力を形成した要因に光を当てます。心理学では、人間と動物の行動についての理論に情報を与えます。人工知能では、インテリジェンスの進化から得られた洞察がより洗練された機械やアルゴリズムの設計に影響を与えることがあります。
関連トピックとその説明
インテリジェンスの神経科学: この分野は、脳の構造と```markdown
機能を含む、知的行動の根底にある神経メカニズムを研究します。人工知能(AI): AI研究は、コンピュータ内で知的行動を再現またはシミュレートすることを目指し、しばしばインテリジェンスの進化から導かれる原則に基づいて行われます。
社会的インテリジェンス仮説: この仮説は、社会生活の複雑さが高度な認知能力の進化を推進したと提唱します。
人類学と人類の進化: 人類の起源を研究するこの分野は、インテリジェンスがホモ・サピエンスおよび私たちの祖先の発展をどのように形作ってきたかに焦点を当てます。
動物の行動と認知: 非人間動物の認知能力を検討することは、インテリジェンスの多様性と進化を理解するのに役立つ比較データを提供します。
主要人物または貢献者
チャールズ・ダーウィン: 彼の自然選択による進化理論は、インテリジェンスの進化を研究するための基盤を築きました。
リチャード・バーンとアンドリュー・ホワイトン: 社会的インテリジェンス仮説に関する彼らの業績で知られています。
ジェーン・グドール: 彼女のチンパンジーに関する観察は、非人間霊長類の知性理解に大きく貢献しています。
現状と今後の方向性
この分野は絶えず進化しており、最近の遺伝学と神経イメージングの進歩により、知的行動が私たちのDNAにどのようにエンコードされ、脳内で実現されるかについての新たな洞察がもたらされています。将来の研究は、インテリジェンスの遺伝的基盤、文化が認知能力の進化に及ぼす影響、および進化プロセスを模倣することができるAIシステムの開発に焦点を当てるかもしれません。
結論
インテリジェンスの進化は、私たちの過去と未来の間のギャップを埋める広大で複雑な主題です。さまざまな種や時代を通じてインテリジェンスがどのように発展してきたかを研究することにより、私たちは自身の心についての洞察を得るだけでなく、いつの日にか私たち自身の能力に匹敵するか、それを超えるかもしれない知的システムを創り出す道を切り開きます。
次世代に関連するトピックとしては以下のようなものがあります:
ニューロエボリューション
認知考古学
進化心理学
動物の道具使用と文化
機械学習と進化的アルゴリズム
オリジナル英語バージョン
Evolution of Intelligence
Introduction
The evolution of intelligence refers to the process by which intelligent behavior has developed over evolutionary time scales. This topic encompasses a wide range of disciplines, from biology and anthropology to psychology and artificial intelligence, seeking to understand how cognitive abilities have emerged and diversified among different organisms, including humans.
Definition and Description
Intelligence can be broadly defined as the capacity for learning, reasoning, understanding complex ideas, and adapting to environments. In the context of evolution, intelligence is seen as a set of cognitive traits that have been shaped by natural selection to solve specific ecological problems. The evolution of intelligence studies the historical development of these traits, the factors driving their complexity, and their different manifestations across species.
History
The concept of intelligence has been discussed for centuries, but the systematic study of its evolution began with Charles Darwin's work on natural selection and the descent of man. Since then, numerous theories have been put forward to explain the emergence of intelligence, including the social intelligence hypothesis, which suggests that complex social interactions drove the development of advanced cognitive abilities.
Concepts and Principles
Central to the study of the evolution of intelligence is the idea that intelligence is not a single trait but a collection of cognitive processes that have evolved to varying degrees in different species. Key concepts include brain-to-body ratio (encephalization quotient), tool use, problem-solving abilities, language, and culture. These concepts are used to compare and contrast cognitive abilities across the animal kingdom and to trace the lineage of intelligent behavior in evolutionary history.
Significance and Applications
Understanding the evolution of intelligence has profound implications for multiple fields. In biology and anthropology, it sheds light on human origins and the factors that have shaped our cognitive capabilities. In psychology, it informs theories about human and animal behavior. In artificial intelligence, insights from the evolution of intelligence can inspire the design of more sophisticated machines and algorithms.
Related Topics with explanations
Neuroscience of Intelligence: This field studies the neural mechanisms underlying intelligent behavior, including brain structure and function.
Artificial Intelligence (AI): AI research is often informed by principles derived from the evolution of intelligence, seeking to replicate or simulate intelligent behavior in computers.
Social Intelligence Hypothesis: This hypothesis suggests that the complexities of social life have driven the evolution of advanced cognitive abilities.
Anthropology and Human Evolution: The study of human origins often focuses on how intelligence has shaped the development of Homo sapiens and our ancestors.
Animal Behavior and Cognition: Examining the cognitive abilities of non-human animals provides comparative data that helps in understanding the diversity and evolution of intelligence.
Key Figures or Contributors
Charles Darwin: His theory of evolution by natural selection set the foundation for studying the evolution of intelligence.
Richard Byrne and Andrew Whiten: Known for their work on the social intelligence hypothesis.
Jane Goodall: Her observations of chimpanzees have contributed significantly to the understanding of intelligence in non-human primates.
Current Status and Future Directions
The field is continually evolving, with recent advancements in genetics and neuroimaging providing new insights into how intelligent behaviors are encoded in our DNA and realized in our brains. Future research may focus on the genetic basis of intelligence, the influence of culture on the evolution of cognitive abilities, and the development of AI systems that can mimic evolutionary processes.
Conclusion
The evolution of intelligence is a vast and intricate subject that bridges the gap between our past and future. By studying how intelligence has developed across different species and epochs, we not only gain insight into our own minds but also pave the way for creating intelligent systems that could one day match or surpass our own capabilities.
Related topics for the next generation might include:
Neuroevolution
Cognitive Archaeology
Evolutionary Psychology
Animal Tool Use and Culture
Machine Learning and Evolutionary Algorithms
こちらは上記の内容を日本語に翻訳したものです。
機械学習と進化アルゴリズム
序論
人工知能の分野において、機械学習は、データから学習し、予測や意思決定を行うことができるコンピューターシステムを実現する革新的な手法として登場しています。機械学習のアプローチの中でも、進化アルゴリズムは特に注目に値する手法で、自然選択および生物進化のプロセスに着想を得たものです。
定義と説明
機械学習 (ML) は、人工知能の一部分で、統計的手法を用いてコンピューターシステムがデータから学習し、予測や意思決定を行うことができるようにするものです。ML アルゴリズムは、「学習用データ」に基づいてモデルを構築し、明示的なプログラミングなしで課題を実行できるようになります。
進化アルゴリズム (EA) は、自然選択と遺伝学の原理に基づいた最適化アルゴリズムの一種です。EA では、定義された適応度関数に従って、変異、交叉 (組換え)、選択などの演算子を適用しながら、世代を重ねて、より良い解のcandidate集団を進化させていきます。
歴史
進化アルゴリズムの概念は1960年代に初めて提唱され、ダーウィンの進化理論に着想を得たものです。1970年代のジョン・ホランドによる遺伝的アルゴリズムに関する研究は、この分野をコンピューター科学の領域に導入した基礎的な取り組みとして知られています。その後、遺伝的アルゴリズム、遺伝的プログラミング、進化戦略、進化的プログラミングなど、さまざまな形態の進化アルゴリズムが発展してきました。
概念と原理
進化アルゴリズムは、解のcandidate集団に対して操作を行います。EA の主要な過程は以下の通りです:
選択: 適応度の高い個体を選んで交配させる。
交叉: 2つの親個体の一部を組み合わせて子個体を生成する。
変異: 解の一部をランダムに変化させて多様性を生み出す。
適応度関数: 解の良さを評価する尺度。
これらの過程を繰り返し行うことで、集団は徐々に最適または準最適な解に収束していきます。
意義と応用
進化アルゴリズムは、従来の手法では解決が困難な複雑な最適化問題やサーチ問題に適しています。以下のような分野で活用されています:
工学設計と最適化
人工生命とロボティクス
金融モデリング
ゲーム開発と戦略最適化
バイオインフォマティクスと創薬
機械学習と進化アルゴリズムを組み合わせることで、データから学習しつつ新しい状況にも適応できるシステムの開発が可能になっています。
関連トピックと説明
遺伝的アルゴリズム (GA): 生物進化の手法に着想を得た進化アルゴリズムの一種で、最適化やサーチ問題の解決に用いられる。
ニューラルネットワーク: 人間の脳に着想を得た計算モデルで、画像認識や音声認識などの機械学習タスクで広く使われている。
群知能: 蟻コロニーや鳥の群れなどの自己組織化するシステムの集団行動をモデル化したアプローチ。
強化学習: 環境との相互作用によって報酬を最大化するよう学習するML手法。
最適化問題: 制約条件の中で最良の解を見つける問題で、EAなどのアルゴリズムを利用して解決される。
主要な研究者
ジョン・ホーランド: 遺伝的アルゴリズムの提唱者として知られ、コンピューター科学分野への進化概念の導入に貢献した。
インゴ・レヒェンバーグ、ハンス=パウル・シュヴェーフェル: 進化戦略と呼ばれる進化アルゴリズムの重要な亜種を開発した。
ケネス・ド・ヨング: 遺伝的アルゴリズムの理解と改善に関する重要な貢献者。
現状と今後の展望
機械学習は急速に発展を遂げており、進化アルゴリズムがその中で重要な役割を果たしています。今後は、深層学習などの他の機械学習手法と組み合わせた方式がさらに注目されると考えられます。ニューラルネットワークの自動進化設計や、機械学習モデルの自動設計 (AutoML) などの研究も活発に行われており、次世代のAI革新につながると期待されています。
結論
機械学習と進化アルゴリズムは、EAの適応性と最適化能力が MLの予測・分析力を補完し合う相乗効果のある組み合わせです。この学際的なアプローチは自然界のプロセスの複雑さを反映しつつ、さまざまな分野における困難な問題に対しても堅牢な解決策を提供しています。
次世代に関連するトピックには以下のようなものが考えられます:
AutoML (自動機械学習)
深層学習と遺伝的プログラミング
スウォームロボティクス
進化アルゴリズムによる多目的最適化
人工生命における進化アルゴリズムの役割
オリジナル英語生成文
Machine Learning and Evolutionary Algorithms
Introduction
In the realm of artificial intelligence, machine learning has emerged as a revolutionary technique that enables computers to learn from and make predictions or decisions based on data. Among the various approaches within machine learning, evolutionary algorithms stand out as a fascinating and powerful subset that draws inspiration from the process of natural selection and biological evolution.
Definition and Description
Machine Learning (ML) is a subset of artificial intelligence that involves the use of statistical techniques to enable computer systems to learn from and make predictions or decisions based on data. ML algorithms build a model based on sample data, known as "training data," to make predictions or decisions without being explicitly programmed to perform the task.
Evolutionary Algorithms (EAs) are a class of optimization algorithms based on the principles of natural selection and genetics. They iteratively evolve a population of candidate solutions towards better solutions by applying operators such as mutation, crossover (recombination), and selection according to a defined fitness function.
History
The concept of evolutionary algorithms was first introduced in the 1960s, inspired by Darwin’s theory of evolution. John Holland's work on genetic algorithms in the 1970s is often cited as the foundational effort that brought these ideas into the field of computer science. Over the years, various forms of evolutionary algorithms have been developed, including genetic algorithms, genetic programming, evolution strategies, and evolutionary programming.
Concepts and Principles
Evolutionary algorithms operate on a population of potential solutions. The key processes in an EA include:
Selection: Choosing the fittest individuals to reproduce.
Crossover: Combining parts of two parents to create offspring.
Mutation: Randomly altering parts of a solution to create variation.
Fitness Function: A way to measure how good a solution is compared to others.
These processes are repeated over many generations, with the population gradually converging to an optimal or near-optimal solution.
Significance and Applications
Evolutionary algorithms are particularly suited for solving complex optimization and search problems where traditional methods may fail. They are used in a variety of fields such as:
Engineering design and optimization
Artificial life and robotics
Financial modeling
Game development and strategy optimization
Bioinformatics and drug design
The combination of machine learning with evolutionary algorithms has led to the development of systems that can both learn from data and adapt to new, unforeseen scenarios.
Related Topics with explanations
Genetic Algorithms (GAs): A subset of evolutionary algorithms that use techniques inspired by biological evolution to find solutions to optimization and search problems.
Neural Networks: Computational models that are inspired by the human brain and are widely used in machine learning for tasks such as image and speech recognition.
Swarm Intelligence: Another bio-inspired algorithmic approach that models the collective behavior of decentralized, self-organized systems such as ant colonies or bird flocking.
Reinforcement Learning: A type of machine learning where an agent learns to make decisions by taking actions in an environment to maximize some notion of cumulative reward.
Optimization Problems: Problems that require finding the best solution from all feasible solutions, often utilizing algorithms like EAs to solve them.
Key Figures or Contributors
John Holland: Known as the father of genetic algorithms, his work laid the groundwork for the adoption of evolutionary concepts in computer science.
Ingo Rechenberg and Hans-Paul Schwefel: They developed evolution strategies, an important variant of evolutionary algorithms.
Kenneth De Jong: A significant contributor to the field of evolutionary computation, known for his work on understanding and improving genetic algorithms.
Current Status and Future Directions
Machine learning continues to grow rapidly, with evolutionary algorithms playing a significant role in solving complex problems. The future may see more hybrid approaches that combine EAs with other machine learning techniques, like deep learning, to push the boundaries of what is possible. Research into evolving neural network architectures or the automated design of machine learning models (AutoML) is ongoing and holds promise for the next wave of AI innovations.
Conclusion
Machine learning and evolutionary algorithms represent a synergistic combination where the adaptability and optimization power of EAs complement the predictive and analytical strengths of ML. This interdisciplinary approach not only mirrors the complexities of natural processes but also offers robust solutions to some of the most challenging problems across various domains.
Related topics for next generation could include:
AutoML (Automated Machine Learning)
Deep Learning and Genetic Programming
Swarm Robotics
Multi-objective Optimization with Evolutionary Algorithms
The Role of Evolutionary Algorithms in Artificial Life
この記事を最後までご覧いただき、ありがとうございます!もしも私の活動を応援していただけるなら、大変嬉しく思います。