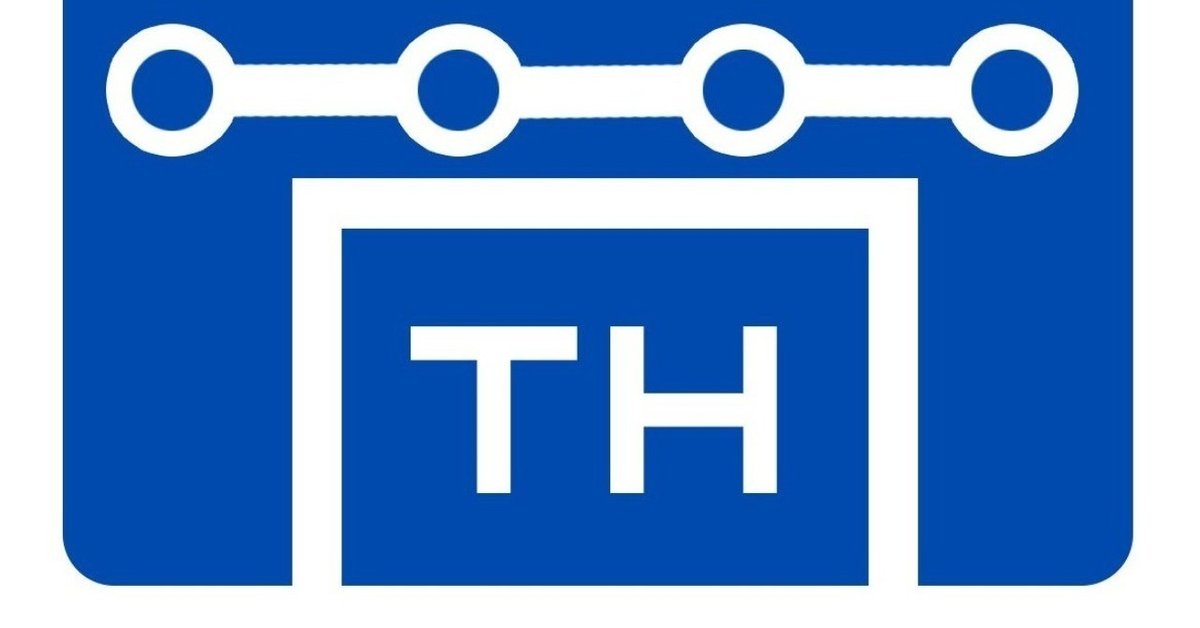
フットボール統計学 人工知能を導入する前にデータ分析について考察する
The Orlando Magic, Dan Altman, and why we should walk before we run
Mar 28, 2019
A few days ago, a headline caught my eye in a daily sports science newsletter I receive: “Orlando Magic Are Pioneers In Introducing Artificial Intelligence To NBA Scouting.” The article details the Magic’s partnership with STATS in applying AI to broadcast feed-based tracking data in using OpenPose, which, according to STATS, “‘unlocks new layers of body-pose information, providing a deeper quality of player tracking data like body position, shot form, torque and other aspects of the game.’”
数日前、受け取った毎日のスポーツ科学ニュースレターの中で見出しが目を引いた。「オーランド・マジックはNBAスカウトに人工知能を導入する先駆者である」この記事では、OpenPoseを使用してフィードベースのトラッキングデータを放送するためのAIを使ったマジックのSTATS社との提携について詳しく説明する。「身体姿勢情報の新しい層を解放し、身体の位置やシュートフォーム、トルク(回転軸のまわりの力のモーメント)、その他の試合の側面など、より高品質の選手トラッキングデータを提供する。」
Me being myself, I was immediately skeptical; when most teams struggle to even put into place a set of basic best practices when it comes to recruitment, are we really at the stage where we need to leap forward to applying AI to scour tracking data?
すぐに懐疑的だった。ほとんどのチームが、採用に関して基本的な最良の実行をまとめることさえできない場合、本当にトラッキングデータを洗練するためにAIを適用することに飛躍する必要がある段階にいるのか。
However my beat isn’t basketball, and the NBA tend to be leaders in this area. But when I read paragraphs like this from the article, more than a few questions come to mind.
しかし自分の鼓動はバスケットボールではなく、NBAはこの分野のリーダーになりうる。しかしこの記事からこのような段落を読むと、いくつかの質問が頭に浮かぶ。
Although technology and its place in player evaluation can be a polarizing issue, OpenPose could be a game changer. If the results are proved accurate, a longstanding subjective criterion such as shooting mechanics might finally be flipped objective. That’s intriguing for a front office.
技術と選手評価におけるその位置は、偏極的な問題になりうるが、OpenPoseは、試合変更者になる可能性がある。
結果が正確と証明されれば、シュート力学のような長年の主観的基準は最終的に客観的に弾かれるかもしれない。
フロントオフィスにとって魅力的である。
But as with many other industries threatened with automation, that could be bad news for the small community of scouts and others in basketball operations. As front offices have continued to grow around the league, owners will undoubtedly be tasked with choosing whether to keep large staffs or go the way of artificial intelligence.
しかし、自動化に脅かされる他の多くの業界と同様に、これは小規模なスカウトコミュニティやバスケットボール事業における他のコミュニティにとっては悪いニュースとなりうる。
フロントオフィスはリーグの周りで成長し続けているので、オーナーは間違いなく大規模なスタッフを維持するか人工知能の道を進むかを選択するように任される。
So the questions I’m asking myself are: do we know whether OpenPose has already identified markers related to improved shooting mechanics (or defensive mechanics for that matter)? Do we know how predictive these measures are, or repeatable, or consistent in certain players, which would make it valuable in recruitment? Or are these markers translatable into coaching techniques? Can you make a good player great through actionable insights based on the technology?
よって質問は、OpenPoseがすでにシュート力学(またはそれに対する守備力学)の改善に関連する目印をすでに特定しているか知っているか。これらの指標が特定の選手でどの程度予測可能であるか、または反復可能であるか、または一貫しているかを知っているか。または、これらの目印はコーチング技術に翻訳可能か。テクノロジーに基づいた実用的な洞察を通して、優れた選手を素晴らしくできるか。
And if we, the public, don’t know this yet, does the Magic?
そしてもし我々公衆がまだ知らないなら、マジックは知っているのか。
If they don’t, I suppose the Magic could justify the investment with the understanding, “we’ll see, hopefully before the next autoSTATS client does.” But if I worked for the team, I would be far less interested in the potential of this technology, but whether those who have developed it can demonstrate the value of any insights it has already produced, whether to sports science or to recruitment, and how we can realistically integrate that into our team process.
そうでなければ、「うまくいけば次のautoSTATS顧客がやる前」と理解して、マジックは投資を正当化できると思う。しかしチームのために働く場合、この技術の可能性にあまり興味を持っておらず、しかしそれを開発した人々がすでに生み出したあらゆる洞察の価値を実証できるか、スポーツ科学か採用か、そしてどのように現実的にチームプロセスに統合できるか。
And then today, I read this tweet, a quote from very smart person Luke Bornn:
Agree 100% with Luke Bornn - NBA-analytic expert: “There are a lot of clubs who are spending in this area, whether its purchasing data or hiring analytical talent, that don’t have the culture or the process in place to make decisions from this data”...
— Mads Davidsen (@MadsRDavidsen) March 27, 2019
そして今日このとても賢いLuke Bornn氏のツイートを読んだ。
NBAの分析専門家であるLuke Bornn氏に100%賛成する。
「購買データであろうと分析的な才能を雇っていようとこの分野で支出しているクラブはたくさんあるが、このデータに基づいて意思決定をするための文化やプロセスが整っていない。」
This is in line with what I’m getting at here. And before you start wondering whether this is still, in fact, a soccer analytics newsletter, Bornn’s thinking on this matter also echoes the words of Dan Altman, formerly a consultant at Swansea City and creator of smartscout, who spoke this past week with Paul Riley for the latter’s blog on his experiences working with the Swans:
これはここで得ていることと一致する。そして実際、これがまだサッカー分析のニュースレターであるか疑問に思い始める前に、この問題についてのBornn氏の考えは、以前スウォンジー・シティのコンサルタントでsmartscoutの創業者であり、先週Paul Riley氏とスウォンジーでのについてブログで語った、Dan Altman氏の言葉にも反響する。
―Do you think the turnover in managers at the club contributed to ideas not being taken up or are we talking about getting decisions implemented at executive level?
―クラブの指揮官の離職が、アイデアが取り上げられなかったことに貢献したと思うか、それとも実行レベルで決定を実行することについて話し合っているのか。
I worked for several years as a strategic consultant before getting into football, and I have some ideas about how organisations ought to run and how decision making processes ought to look. I feel very strongly that even if you didn’t have an analyst at all, if you had a good decision making process, you’d probably make better decisions than a club with a great analyst and no process. That was one of the things I thought could improve at Swansea.
フットボールに入る前に戦略コンサルタントとして数年間働いており、組織がどう走るべきか、そして意思決定プロセスがどのように見えるべきかについていくつか考えを持っている。
アナリストがまったくいなくても、良い意思決定プロセスがあれば、優れたアナリストがいるのにプロセスがないクラブよりも優れた決定を下すことができることを非常に強く感じる。
それはスウォンジーで改善できると思ったものの1つだった。
―What kind of structure should be in place?
―どのような構造が整っているべきか。
Any club should have regular meetings at middle management level for tactics and recruitment and regular, less frequent meetings about those things at a higher level. I think that decisions in that context can be consensus-based, where you are able to collect input from all the relevant parties and keep everybody informed about what’s going on.
どのクラブも戦術と求人のために中間管理職レベルでの定例会議と、より高いレベルでこれらについて頻度は低いが定期的に会議を開くべきである。
このような状況での決定は総意に基づくものになると思い、そこでは関係者全員から意見を集め、何が起こっているのかについて全員に知らせ続けることができる。
Italics mine. As Paul himself acknowledges, this all sounds like common sense! Yet the fact many clubs still put the cart before the horse in hiring analysts before understanding how to implement their work into the overall team process speaks to something I touched on last week, and a common trope in the sports analytics business.
引用の太字は筆者による。Paul氏自身が認めているように、これはすべて常識に思える。それでも、チームのプロセス全体に自分たちの仕事をどのように組み入れるかを理解する前に、多くのクラブがいまだにアナリストを雇うという事実は、先週触れたこと、そしてスポーツ分析ビジネスの共通の強みを語る。
This is the problem of clubs not being able to understand the value-add of data and analytics before they invest in them, i.e. how are non-data scientists in a position to separate analytics snake oil from the good stuff?
これはクラブが投資する前にデータと分析の付加価値を理解できないという問題で、すなわち非データ科学者は分析用のヘビ油を良いものから分離する立場にあるのか。
I think this is a real issue, but I also believe that a club with intelligent decision-making processes in place will ask the right questions of analysts as a matter of course.
これは本当の問題だと思うが、知的な意思決定プロセスが整っているクラブは、当然アナリストの正しい質問をするだろう。
So, for example, a team with a decent player recruitment process will probably want some concrete information from potential analytics hires, such as (and this is off the top of my head):
よって例えば、きちんとした選手の採用プロセスを持つチームでは、次のような潜在的な分析採用からの具体的な情報が必要になるだろう(そしてこれは頭上にない)。
- How precisely will your model improve our overall success rate in recruitment?
- Does your model provide KPIs across all positions and for defensive and attacking ability?
- How does your model perform among players with a transfer fee or wages within these limits?
- How would your work assist us in deciding between two similar players in a particular position, and on what basis?
- How successful is your model in separating team effects from individual impact?
- How agile is your model, and how complex/expensive are the data which drive it?
- Do you have access to enough event or tracking data for players in our realistic target markets?
- モデルは採用における全体的な成功率をどれほど正確に向上させるか。
- モデルはすべてのポジションにわたって守備力と攻撃力のKPI(Key Performance Indicators:重要業績評価指標)を提供するか。
- モデルは移籍金または制限内の賃金で選手間でどのように機能するか。
- 作業は特定のポジションの2人の似たような選手を決める際にどのように、そしてどのような根拠で助けるか。
- チームの影響を個人の影響から分離することで、モデルはどの程度成功するか。
- モデルはどれほど敏捷か、そして運用するデータはどれくらい複雑で高価か。
- 現実的なターゲット市場の選手のための十分なイベントデータやトラッキングデータへのアクセスを持っているか。
For a team that is serious about maximizing ROI in player recruitment, these will be very basic questions indeed. And because analysts will spend a significant amount of time on building databases and cleaning data anyway, it shouldn’t be up to them to figure out how their work should fit into the team process—this is the job of the technical director or director of football or chief executive or whatever.
選手獲得でROI(Return on Investment:投資利益率)を最大化するのに真剣に取り組んでいるチームにとって、これらはまさに非常に基本的な質問になる。とにかくアナリストはデータベースの構築とデータの整理にかなりの時間を費やすので、作業がチームプロセスにどのように適合するべきかを判断するのは、彼らの責任ではない。これはテクニカルディレクター、フットボールディレクター、最高経営責任者などの仕事である。
All this again underlines why I think our collective obsession with analytics ignores the problem that even now, in 2019, some clubs insist on running before they walk. And if that’s the case, no amount of sophisticated technology is going to help improve the situation. In other words, let’s talk far less about data science and more on what a good process looks like, which can (and in some cases should) be analytics-agnostic.
これらすべてが、分析への集合的なこだわりが2019年現在でも、彼らが歩く前に走るという問題を無視すると思う理由を再び強調する。それが事実だとしたら、状況を改善するのに役立つ洗練された技術はない。言い換えれば、データサイエンスについてはあまり話をせず、分析にとらわれない良いプロセスがどのように見えるかについてもっと話をする。
Spot on by @RWhittall about football clubs & data analysis.
— 11tegen11 (@11tegen11) March 28, 2019
Clubs tend to invest in data, before they even have an infrastructure in place to deal with the information, let alone the expertise to judge the quality of the products they're buying.https://t.co/cOmA03dk7c
ここから先は
¥ 100
#フットボール統計学