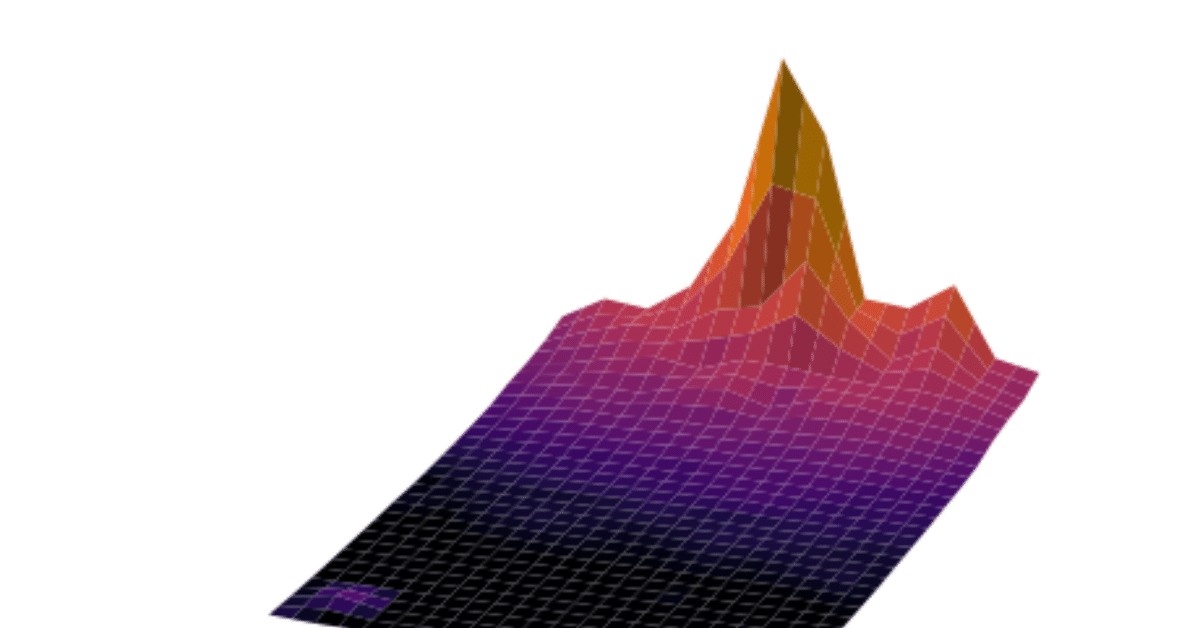
フットボール統計学 脅威期待値の導入(前編) スカラー場との類似
Introducing Expected Threat (xT)
Modelling team behaviour in possession to gain a deeper understanding of buildup play.
Karun Singh (@karun1710)
Before we begin...
As you may have inferred from my previous post on interactive, weighted passing networks, I'm a big fan of going beyond static visualizations. Quantitative analysis is great, but I believe that we can amplify the gains of such analysis by being more adventurous with presentation. This post in particular contains a lot of interactivity – much of it experimental – in an effort to probe new areas of the design space and hopefully spark productive discussions.
対話式で重み付けされたパスネットワークに関する前回の投稿から推測したように、静的な視覚化を超えたものになるのが大好きである。定量分析は素晴らしいが、プレゼンテーションにもっと冒険的になることで、そのような分析の利点を増幅できると思う。特にこの記事では、デザインスペースの新しい分野を探り、生産的な議論を巻き起こすために、実験的な対話性を多く含む。
Credit where credit's due
To motivate the rest of this post, consider Arsenal’s opening goal in a recent 3-1 win against Burnley:
この記事の残りの部分をやる気にさせるために、バーンリー戦の最近の3-1の勝利におけるアーセナルの先制点を考えよう。
After some intricate passing on the right, Mesut Özil slices the Burnley defence open with a ball through to Sead Kolašinac, whose timely cutback finds Pierre-Emerick Aubameyang for the finish. Thanks to @lastrowview, here's a neat top-down visualization of the same sequence of play:
右サイドでの入り込んだパスの後、メスト・エジルがセアド・コラシナツへとボールを広げ、ピエール=エメリク・オーバメヤンがフィニッシュを決めた。@lastrowviewのおかげで、これは同じポゼッション連鎖の上からの視覚化である。
Of course, on paper the assist for this goal is given to Kolašinac. But as an analyst you might (rightly) ask where Özil's credit is. Where's the metric that can capture both, Özil and Kolašinac's contributions in a proportional manner?
もちろん、紙の上でこの得点のアシストはコラシナツに与えられる。しかし、アナリストとして、エジルのクレジットがどこにあるのか(正しい)尋ねるかもしれない。 エジルとコラシナツの貢献を比例的に捉えることができる指標はどこにあるのか。
How should we divide up the credit between Özil and Kolašinac for creating this opportunity? Drag the slider to enter what you think!
この機会を創出するために、どのようにエジルとコラシナツの間でクレジットを分けるべきか。スライダーをドラッグして思いのままに動かそう。
The purpose of this exercise isn't to converge on a universally accepted answer, but rather to show that breaking down buildup play and assigning credit to individual actors is a hard problem.
この演習の目的は、普遍的に受け入れられている答えに集中することではなく、むしろビルドアッププレーを分割して個々のアクションを行った選手にクレジットを割り当てることが難しい問題であることを示すことである。
Existing approaches
There are several existing quantitative frameworks you might want to use to approach this problem:
この問題に取り組むために使用したいと思うかもしれないいくつかの既存の量的なフレームワークがある。
- You can look at assists, but then contributions such as Özil's will go unnoticed in the numbers.
- You can look at xGChain, where the xG of the final shot (= 0.13 in this case) will be equally divided amongst every player involved in the play. Kolašinac, Özil, and even Aubameyang, Maitland-Niles, and Lacazette would all be credited with the same amount of xGChain here, which is not reflective of true contribution. A related quantity, xGBuildup, will divide up the xG equally amongst everyone who was involved before the assist (i.e. Özil, Maitland-Niles, and Lacazette), but this too suffers from the same problem.
- You can look at the difference in xG induced by each action in the buildup. This is better, but a threatening pass is not always one that goes to a good shooting position. For example, Özil's pass split the defence open, yet it wasn't received in a particularly good shooting position by Kolašinac. Rather, what makes Özil's pass special is that it puts Kolašinac in a position from where he can in turn easily create a good chance.
- アシストを見ることができるが、その場合このエジルのような貢献は数に気づかれない。
- xGChainを見ることができ、そこでは最後のシュートのxG(この場合 0.13)がプレーに関わったすべての選手の間で等しく分けられる。コラシナツ、エジル、さらにはオーバメヤン、エインズリー・メイトランド=ナイルズ、そしてアレクサンドル・ラカゼットも、ここでは同じ量のxGChainとみなされ、これは真の貢献を反映したものではない。関連量xGBuildupは、アシストの前に関与していた全員(すなわちエジル、メイトランド=ナイルズ、およびラカゼット)の間でxGを均等に分割するが、これも同じ問題を抱えている。
- ビルドアップの各アクションによって引き起こされるxGの違いを見ることができる。これはより良いが、脅威的なパスは常に良いシュート位置に行くものではない。例えば、エジルのパスが守備陣をオープンに分割したが、それでもコラシナツによる特に良いシュート位置には届かなかった。 むしろ、エジルのパスを特別なものにしているのは、コラシナツが簡単に決定機を生み出すことができる位置に置くということである。
Can we do better?
Building off the deficiencies of existing approaches, we would like a framework that can:
1. Reward individual player actions (passes, dribbles) in buildup play.
2. Operate on event-level data, due to availability constraints.
3. Reward actions independent of the end outcome of the possession (i.e. Özil's reward shouldn't depend on Aubameyang shooting or scoring).
4. Reward moving the ball not just into high-xG shooting positions, but also into 'threatening' positions that can in turn lead to high-xG shooting positions with high likelihood.
既存のアプローチの欠点を排除して、次のことができるフレームワークを望む。
1. ビルドアッププレーで個々の選手のアクション(パス、ドリブル)に報酬を与える。
2. 可用性の制約により、イベントレベルのデータを操作する。
3. ポゼッションの最終結果とは無関係のアクション報酬(すなわちエジルの報酬はオーバメヤンのシュートや得点に依存するべきではない)。
4. ボールをxGの高いシュート位置だけでなく、「脅威的」な位置で結果としてxGの高いシュート位置に移動させると報酬を与える。
There is of course no single solution that is 'correct' here. As always, there's a trade-off between modelling complexity and accuracy. The purpose of this post, though, is to introduce one possible modelling approach, and walk through how it can be implemented and used to analyze buildup play.
もちろん、ここで「正しい」唯一の解決策はない。いつものように、モデリングの複雑さと正確さの間にはトレードオフがある。ただし、この記事の目的は、1つの可能なモデリング手法を紹介し、それを実装してビルドアッププレーの分析に使用する方法を説明することである。
Let's go through those requirements again, this time proposing and refining a solution as we go along:
これらの要件をもう一度見て、今回は解決策を提案し、改良する。
1. Reward individual player actions: our model should assign a score to each player action (pass or dribble) based on how much it contributed to the buildup play.
2. Event-level data: we do not have access to any player tracking data; we only have a list of sequential events along with basic attributes for each event, such as the player in possession, time elapsed in the match, start location, end location, etc.
3. Independence from end outcome: each action should be assigned a score in isolation, disregarding what happened before and after it in the possession. As far as relevant input signals go, this effectively leaves us with just the start and end locations of the action. How can we assign a score based on just those? We can build off the 'difference in xG' approach and assign a value to every location on the pitch. Then, if a certain action resulted in the ball moving from A to B, the score for the action can simply be the value at B minus the value at A.
4. Recognize 'threatening' positions: while assigning a value to every location on the pitch, we must look beyond xG. The value generated by xG assumes that we will shoot in the next action. Yet there are many locations from where scoring directly is hard, but it is easy to move the ball into other higher-xG areas. While assigning values to locations, we need to recognize these high-threat locations. In other words, xG allows us only 1 action (i.e. shoot) from the current position, while to value threat we must consider the possibility of stringing together multiple actions.
1. 個々の選手のアクションに報酬を与える。モデルはどれだけビルドアッププレーに貢献したかに基づいて、各選手のアクション(パスまたはドリブル)にスコアを割り当てるべき。
2. イベントレベルのデータ。いかなる選手のトラッキングデータにもアクセスできない。ポゼッション連鎖の選手、試合の経過時間、開始地点、終了地点など、各イベントの基本的な属性とともに、イベントのリストだけがある。
3. 最終結果からの独立性。各アクションには、それがポゼッション内の前後の動作を無視して、個別にスコアを割り当てる必要がある。関連するインプットシグナルに関する限り、これはアクションの開始位置と終了位置だけを残す。それらに基づいてスコアを割り当てるにはどうすればよいか。「xGの差」アプローチを構築し、ピッチ上のすべての場所に値を割り当てることができる。そして、あるアクションの結果、ボールがAからBに移動した場合、その行動のスコアは単純にBの値からAの値を引いたものになる。
4. 「脅威的」な位置を認識する。ピッチ上のすべての位置に値を割り当てながら、xGを超えて見なければならない。xGによって生成された値は、次のアクションでシュートすることを前提とする。それでも、直接得点するのは難しいが、ボールを他のより高いxGの領域に移動するのは簡単な場所がたくさんある。場所に値を割り当てるとき、これらの脅威の高い場所を認識する必要がある。言い換えれば、xGは現在の位置から唯一のアクション(すなわちシュート)を許すが、脅威を測るには複数のアクションをつなぎ合わせる可能性を考えなければならない。
Having made these modelling assumptions, our problem is now more digestible: given a repository of event-level data, can we assign a threat value to every location on the pitch?
これらのモデル化の仮定をしたので、問題はもっと消化しやすくなる。イベントレベルのデータの保管を考えて、ピッチ上のあらゆる場所に脅威の値を割り当てることができるか。
Note: the idea of assigning a value to every location on the pitch, or creating a 'value surface', isn't new. In fact, it goes beyond much further than football analytics. For instance, there's a cool physics analogy with electric potential fields to think about (or more generally, with any kind of scalar field), where attributing a score to a player action is analogous to potential difference! Relatedly, it means that assigning values to actions in this manner leads to properties exhibited by conservative forces. For example, the exact path taken by a player while dribbling is irrelevant (path independence), while moving the ball in a loop results in a reward of 0. In reality, the exact dribbling path can of course be important, while moving in a loop might actually draw defenders out of position, so this is an inaccuracy we tolerate for simplicity as well as a lack of tracking data.
注:ピッチ上のすべての場所に値を割り当てる、または「価値面」を作成するという考えは新しいものではない。実際、それはフットボール分析よりもはるかに進んでいる。例えば、考えるべき電位場(あるいはより一般的にあらゆる種類のスカラー場)との物理学の類似性があり、選手の行動にスコアを割り当てることは、ポテンシャルの差に似ている。これに関連して、このようにしてアクションに値を割り当てることは、保存力によって示される特性をもたらすことを意味する。例えば、ドリブル中に選手がたどった正確な道筋は無関係(道筋に独立)だが、ボールをループ内で移動すると、報酬は0になる。実際には、正確なドリブルの道筋はもちろん重要で、ループ内を移動すると実際に守備陣の位置がずれてしまう可能性があるため、これはトラッキングデータの不足と単純さのために許容される不正確さである。
When in possession...
ここから先は
¥ 100
#フットボール統計学