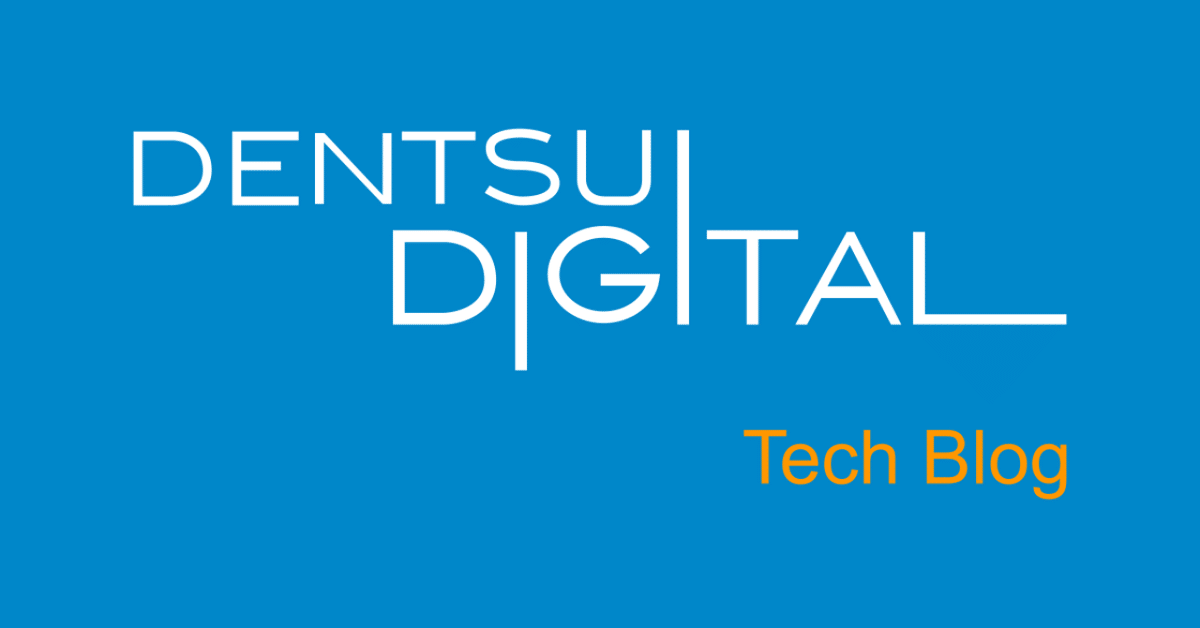
SageMakerで学習したXGBoostモデルのFeature Importanceを取得するAirflowオペレーター
電通デジタルでデータサイエンティストとして働いている長島です。
本記事ではSageMakerで学習したXGBoostモデルのFeature Importance取得をAirflowで自動化する方法を紹介します。
SageMakerにはXGBoostをはじめとする組み込みモデルが多数用意されており、容易に学習・推論を行うことができます[1]。 これらに対応したAirflowオペレーターもあるため、機械学習フローをまとめて管理するのに非常に便利です[2]。 一方、モデル依存の情報を抜き出すような機能は備えていないため、XGBoostの強みの一つである、Feature Importanceの取得はSageMakerの機能として備わっていません。
今回は、SageMakerXGBoostImportanceOperatorという名前で、タイトルのような機能を持ったオペレーターを作成していきます。 これをトレーニングやチューニングオペレーターの後ろに繋げることで、学習が済んだモデルでどのfeatureが重要度が高いのか、自動的に出力することができます。
DAGイメージ
トレーニングとデプロイを行うDAGに、importance出力用のオペレータを追加すると以下のようになります。
train_xgb_op >> {deploy_xgb_op, plot_importance_op}
全体の流れ
基本的な流れは、ローカルやノートブックで実行するときと変わりません。
- 1. モデルファイル(model.tar.gz)をS3からダウンロード&解凍
2. pickleでロード
3. モデルにfeature名を紐づける
4. importanceをファイル出力
5. 出力結果をS3にアップロード - という流れになります。
3.モデルにfeature名を紐づける
これは、モデルファイルに学習時のfeature名が保存されていないために別途用意が必要です。 出力後に照らし合わせても良いのですが、便利な xgboost.plot_importance を使いたいので、先に紐づけることにします。
事前準備
以下のライブラリをAirflow環境に事前にインストールしておきます。
- ・pickle
・xgboost==0.90
→最新版は1.0.2ですが、SageMakerのxgboostは0.90が最新です。(公開時点)
・japanize_matplotlib
→これは必須ではないですが、feature_namesに日本語が入るときに簡単に文字化けが解消できるのでおすすめです。
コンストラクタ
オペレーターに渡す情報は以下4つです。
- ・Airflowに登録したS3コネクションID
・S3バケット名
・モデルファイルのS3キー
トレーニングの際、output_pathで指定した場所に、 model.tar.gz ファイルが出力されます。バケット名よりも後ろの部分になります。
・feature名
S3へのアクセスは、 airflow.hooks.S3_hook で提供されています[3]。
def __init__(self,
s3_conn_id: str,
s3_bucket: str,
model_key: str,
feature_names: list,
**kwargs) -> None:
"""
Args:
s3_conn_id: Airflow connection ID for S3
s3_bucket: S3 bucket name
model_key: key for trained XGBoost model
feature_names: list of feature names
Returns:
S3 key where importance plot will be uploaded
"""
self.s3_hook = S3Hook(aws_conn_id=s3_conn_id)
self.s3_bucket = s3_bucket
self.model_key = model_key
self.feature_names = feature_names
super(SageMakerXGBoostImportanceOperator, self).__init__(**kwargs)
モデルファイルのダウンロード・解凍
model.tar.gz ファイルには、唯一 xgboost-model というファイルが含まれています。 これをS3からダウンロードし、tarfileで解凍します。
## S3にファイルがあるか確認
if not self.s3_hook.check_for_key(f's3://{self.s3_bucket}/{self.model_key}'):
raise AirflowException(f'{self.model_key} is not created')
## 作業用に一時ディレクトリを作成
tempdir = tempfile.TemporaryDirectory()
## ダウンロード&解凍
local_path = path.join(tempdir.name, 'model.tar.gz')
try:
self.conn.download_file(
Bucket=self.s3_bucket,
Key=s3_key,
Filename=local_path
)
with tarfile.open(local_path, 'r') as tar:
mem_name = tar.getnames()[0]
tar.extract(mem_name, tempdir.name)
except Exception as e:
raise e
モデルのロード
xgboost-model はpickleで保存されたモデルファイルなので、 pickle.load を使うことで、メモリ上にモデルを復元することができます。 前述したとおり、このモデルファイルはfeature名を含まないので、別途用意したfeature名を紐づけています。
## モデルのロード
model_path = path.join(tempdir.name, mem_name)
model = pkl.load(open(model_path, 'rb'))
model.feature_names = self.feature_names
特徴量出力
通常の学習済みXGBoostモデルの扱いと同じです。 今回は、全featureのimportance一覧と、importanceの高いfeatureをplotしたものを出力します。
## importanceのファイル出力
score_dict = model.get_score(importance_type='weight')
csv_name = path.join(tempdir.name, 'importance.csv')
with open(csv_name, 'wt') as f:
f.write('\n'.join([name + ',' + str(imp) for name, imp in enumerate(score_dict)]))
## plot
fig = plt.figure(figsize=(10, 20))
fig.subplots_adjust(left=0.2)
ax = fig.add_subplot(1, 1, 1)
xgboost.plot_importance(model,
ax=ax,
importance_type='weight',
show_values=False,
max_num_features=20)
plt_name = path.join(tempdir.name, 'importance.png')
plt.savefig(plt_name, format='png')
plotは以下のような形で出力されます。(feature名はマスクしてあります)
結果のアップロード
出力した2つのファイルを保存します。 ダウンロード同様、S3_hookを使って、モデルファイルがあった場所と同じ階層に置くことにします。
## 結果のアップロード
try:
csv_key = self.model_key.replace('model.tar.gz', 'importance.csv')
self.conn.upload_file(
Bucket=self.s3_bucket,
Key=csv_key,
Filename=csv_name
)
plt_key = self.model_key.replace('model.tar.gz', 'importance.png')
self.conn.upload_file(
Bucket=self.s3_bucket,
Key=plt_key,
Filename=plt_name
)
except Exception as e:
raise e
全コード
from airflow.models import BaseOperator
from airflow.hooks.S3_hook import S3Hook
import xgboost
import pickle as pkl
import matplotlib.pyplot as plt
import tarfile
import tempfile
from os import path
import japanize_matplotlib ## importするだけでOK
class SageMakerXGBoostImportanceOperator(BaseOperator):
def __init__(self,
s3_conn_id: str,
s3_bucket: str,
model_key: str,
feature_names: list,
**kwargs) -> None:
"""
Args:
s3_conn_id: Airflow connection ID for S3
s3_bucket: S3 bucket name
model_key: key for trained XGBoost model
feature_names: list of feature names
Returns:
S3 key where importance plot will be uploaded
"""
self.model_key = model_key
self.feature_names = feature_names
self.s3_hook = S3Hook(aws_conn_id=s3_conn_id)
self.s3_bucket = s3_bucket
self.conn = self.s3_hook.get_conn()
super(SageMakerXGBoostImportanceOperator, self).__init__(**kwargs)
def execute(self, context) -> str:
## S3にファイルがあるか確認
if not self.s3_hook.check_for_key(f's3://{self.s3_bucket}/{self.model_key}'):
raise AirflowException(f'{self.model_key} is not created')
## 作業用に一時ディレクトリを作成
tempdir = tempfile.TemporaryDirectory()
## ダウンロード&解凍
local_path = path.join(tempdir.name, 'model.tar.gz')
try:
self.conn.download_file(
Bucket=self.s3_bucket,
Key=self.model_key,
Filename=local_path
)
with tarfile.open(local_path, 'r') as tar:
mem_name = tar.getnames()[0]
tar.extract(mem_name, tempdir.name)
except Exception as e:
raise e
## モデルのロード
model_path = path.join(tempdir.name, mem_name)
model = pkl.load(open(model_path, 'rb'))
model.feature_names = self.feature_names
## importanceのファイル出力
score_dict = model.get_score(importance_type='weight')
csv_name = path.join(tempdir.name, 'importance.csv')
with open(csv_name, 'wt') as f:
f.write('\n'.join([name + ',' + str(imp) for name, imp in enumerate(score_dict)]))
## plot
fig = plt.figure(figsize=(10, 20))
fig.subplots_adjust(left=0.2)
ax = fig.add_subplot(1, 1, 1)
xgboost.plot_importance(model,
ax=ax,
importance_type='weight',
show_values=False,
max_num_features=20)
plt_name = path.join(tempdir.name, 'importance.png')
plt.savefig(plt_name, format='png')
## 結果のアップロード
try:
csv_key = self.model_key.replace('model.tar.gz', 'importance.csv')
self.conn.upload_file(
Bucket=self.s3_bucket,
Key=csv_key,
Filename=csv_name
)
plt_key = self.model_key.replace('model.tar.gz', 'importance.png')
self.conn.upload_file(
Bucket=self.s3_bucket,
Key=plt_key,
Filename=plt_name
)
except Exception as e:
raise e
return plt_key
まとめ
本記事では、SageMakerで学習したXGBoostモデルのFeature Importanceを取得・描画するAirflowオペレーターを作成してみました。 今回はXGBoostを取り上げましたが、他の組み込みモデルでもpickleやMXNet checkpoint形式でS3に保存されます。 学習済みのモデルから情報を抜き出したい場合は同じような手順で解決できますので、独自の分析を行いたい場合には試してみるとよいかもしれませんね。
参考
[1]https://docs.aws.amazon.com/ja_jp/sagemaker/latest/dg/algos.html
[2]https://aws.amazon.com/jp/blogs/news/build-end-to-end-machine-learning-workflows-with-amazon-sagemaker-and-apache-airflow/
[3]https://airflow.readthedocs.io/en/stable/_modules/airflow/hooks/S3_hook.html