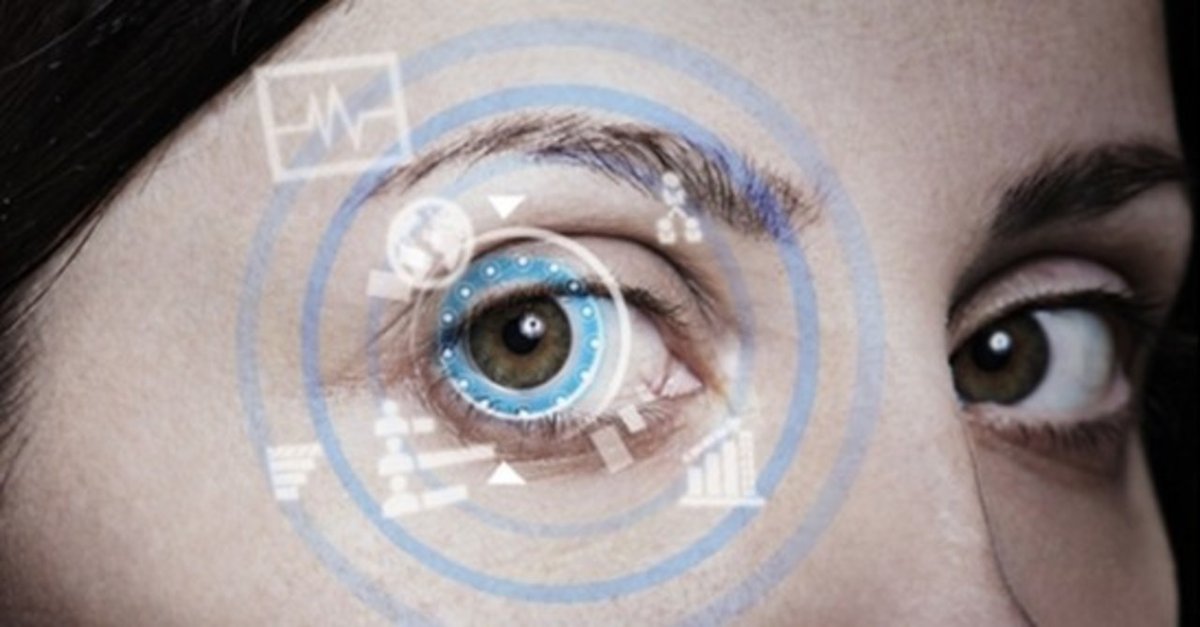
CVPR2020 - 顔関連の論文リスト
顔認識プロダクトの企画をしています。その一環で論文調査も兼ねてCVPR2020にAcceptされた顔関連論文(62件)をリストアップしました。
企業として、この領域でプロダクトをリリースするためには、認識精度の向上はもちろんですが、レピュテーションリスクにも最大限の配慮をする必要があります。これらに対処するために最新の論文やその動向をチェックすることが重要になります。
1. 顔認識(12)
1-1. Learning Meta Face Recognition in Unseen Domains
Authors: CBSR & NLPR, Institute of Automation, Chinese Academy of Sciences, School of Artificial Intelligence, University of Chinese Academy of Sciences, Mininglamp Academy of Sciences, Mininglamp Technology
School of Engineering, Westlake University
Units:
Face recognition systems are usually faced with unseen domains in real-world applications and show unsatisfactory performance due to their poor generalization. For example, a well-trained model on webface data cannot deal with the ID vs. Spot task in surveillance scenario. In this paper, we aim to learn a generalized model that can directly handle new unseen domains without any model updating. To this end, we propose a novel face recognition method via meta-learning named Meta Face Recognition (MFR). MFR synthesizes the source/target domain shift with a meta-optimization objective, which requires the model to learn effective representations not only on synthesized source domains but also on synthesized target domains. Specifically, we build domain-shift batches through a domain-level sampling strategy and get back-propagated gradients/meta-gradients on synthesized source/target domains by optimizing multi-domain distributions. The gradients and meta-gradients are further combined to update the model to improve generalization. Besides, we propose two benchmarks for generalized face recognition evaluation. Experiments on our benchmarks validate the generalization of our method compared to several baselines and other state-of-the-arts. The proposed benchmarks will be available at this https URL.
1-2. Vec2Face: Unveil Human Faces from their Blackbox Features in Face Recognition
Authors: Concordia University, University of Arkansas, VinAI Research, North Carolina A&T State University
Units:
Unveiling face images of a subject given his/her high-level representations extracted from a blackbox Face Recognition engine is extremely challenging. It is because the limitations of accessible information from that engine including its structure and uninterpretable extracted features. This paper presents a novel generative structure with Bijective Metric Learning, namely Bijective Generative Adversarial Networks in a Distillation framework (DiBiGAN), for synthesizing faces of an identity given that person's features. In order to effectively address this problem, this work firstly introduces a bijective metric so that the distance measurement and metric learning process can be directly adopted in image domain for an image reconstruction task. Secondly, a distillation process is introduced to maximize the information exploited from the blackbox face recognition engine. Then a Feature-Conditional Generator Structure with Exponential Weighting Strategy is presented for a more robust generator that can synthesize realistic faces with ID preservation. Results on several benchmarking datasets including CelebA, LFW, AgeDB, CFP-FP against matching engines have demonstrated the effectiveness of DiBiGAN on both image realism and ID preservation properties.
1-3. Data Uncertainty Learning in Face Recognition
Authors: Jie Chang, Zhonghao Lan, Changmao Cheng, Yichen Wei
Units: Megvii Inc., University of Science and Technology of China
Modeling data uncertainty is important for noisy images, but seldom explored for face recognition. The pioneer work, PFE, considers uncertainty by modeling each face image embedding as a Gaussian distribution. It is quite effective. However, it uses fixed feature (mean of the Gaussian) from an existing model. It only estimates the variance and relies on an ad-hoc and costly metric. Thus, it is not easy to use. It is unclear how uncertainty affects feature learning.This work applies data uncertainty learning to face recognition, such that the feature (mean) and uncertainty (variance) are learnt simultaneously, for the first time. Two learning methods are proposed. They are easy to use and outperform existing deterministic methods as well as PFE on challenging unconstrained scenarios. We also provide insightful analysis on how incorporating uncertainty estimation helps reducing the adverse effects of noisy samples and affects the feature learning.
1-4. GroupFace: Learning Latent Groups and Constructing Group-Based Representations for Face Recognition
Authors: Yonghyun Kim, Wonpyo Park, Myung-Cheol Roh, Jongju Shin
Units: Kakao Enterprise, Kakao Corp.
In the field of face recognition, a model learns to distinguish millions of face images with fewer dimensional embedding features, and such vast information may not be properly encoded in the conventional model with a single branch. We propose a novel face-recognition-specialized architecture called GroupFace that utilizes multiple group-aware representations, simultaneously, to improve the quality of the embedding feature. The proposed method provides self-distributed labels that balance the number of samples belonging to each group without additional human annotations, and learns the group-aware representations that can narrow down the search space of the target identity. We prove the effectiveness of the proposed method by showing extensive ablation studies and visualizations. All the components of the proposed method can be trained in an end-to-end manner with a marginal increase of computational complexity. Finally, the proposed method achieves the state-of-the-art results with significant improvements in 1:1 face verification and 1:N face identification tasks on the following public datasets: LFW, YTF, CALFW, CPLFW, CFP, AgeDB-30, MegaFace, IJB-B and IJB-C.
1-5. CurricularFace: Adaptive Curriculum Learning Loss for Deep Face Recognition
Authors: Yuge Huang, Yuhan Wang, Ying Tai, Xiaoming Liu, Pengcheng Shen, Shaoxin Li, Jilin Li, Feiyue Huang
Units: Youtu Lab, Tencent, Zhejiang University, Michigan State University
As an emerging topic in face recognition, designing margin-based loss functions can increase the feature margin between different classes for enhanced discriminability. More recently, the idea of mining-based strategies is adopted to emphasize the misclassified samples, achieving promising results. However, during the entire training process, the prior methods either do not explicitly emphasize the sample based on its importance that renders the hard samples not fully exploited; or explicitly emphasize the effects of semi-hard/hard samples even at the early training stage that may lead to convergence issue. In this work, we propose a novel Adaptive Curriculum Learning loss (CurricularFace) that embeds the idea of curriculum learning into the loss function to achieve a novel training strategy for deep face recognition, which mainly addresses easy samples in the early training stage and hard ones in the later stage. Specifically, our CurricularFace adaptively adjusts the relative importance of easy and hard samples during different training stages. In each stage, different samples are assigned with different importance according to their corresponding difficultness. Extensive experimental results on popular benchmarks demonstrate the superiority of our CurricularFace over the state-of-the-art competitors.
1-6. Mitigating Bias in Face Recognition Using Skewness-Aware Reinforcement Learning
Authors: Mei Wang, Weihong Deng
Units: Beijing University of Posts and Telecommunications
Racial equality is an important theme of international human rights law, but it has been largely obscured when the overall face recognition accuracy is pursued blindly. More facts indicate racial bias indeed degrades the fairness of recognition system and the error rates on non-Caucasians are usually much higher than Caucasians. To encourage fairness, we introduce the idea of adaptive margin to learn balanced performance for different races based on large margin losses. A reinforcement learning based race balance network (RL-RBN) is proposed. We formulate the process of finding the optimal margins for non-Caucasians as a Markov decision process and employ deep Q-learning to learn policies for an agent to select appropriate margin by approximating the Q-value function. Guided by the agent, the skewness of feature scatter between races can be reduced. Besides, we provide two ethnicity aware training datasets, called BUPT-Globalface and BUPT-Balancedface dataset, which can be utilized to study racial bias from both data and algorithm aspects. Extensive experiments on RFW database show that RL-RBN successfully mitigates racial bias and learns more balanced performance for different races.
1-7. Hierarchical Pyramid Diverse Attention Networks for Face Recognition
Authors: Qiangchang Wang, Tianyi Wu, He Zheng, Guodong Guo
Units: West Virginia University, Morgantown /USA, Institute of Deep Learning, Baidu Research, Beijing /China, National Engineering Laboratory for Deep Learning Technology and Application, Beijing /China
Deep learning has achieved a great success in face recognition (FR), however, few existing models take hierarchical multi-scale local features into consideration. In this work, we propose a hierarchical pyramid diverse attention (HPDA) network. First, it is observed that local patches would play important roles in FR when the global face appearance changes dramatically. Some recent works apply attention modules to locate local patches automatically without relying on face landmarks. Unfortunately, without considering diversity, some learned attentions tend to have redundant responses around some similar local patches, while neglecting other potential discriminative facial parts. Meanwhile, local patches may appear at different scales due to pose variations or large expression changes. To alleviate these challenges, we propose a pyramid diverse attention (PDA) to learn multi-scale diverse local representations automatically and adaptively. More specifically, a pyramid attention is developed to capture multi-scale features. Meanwhile, a diverse learning is developed to encourage models to focus on different local patches and generate diverse local features. Second, almost all existing models focus on extracting features from the last convolutional layer, lacking of local details or small-scale face parts in lower layers. Instead of simple concatenation or addition, we propose to use a hierarchical bilinear pooling (HBP) to fuse information from multiple layers effectively. Thus, the HPDA is developed by integrating the PDA into the HBP. Experimental results on several datasets show the effectiveness of the HPDA, compared to the state-of-the-art methods.
1-8. Towards Universal Representation Learning for Deep Face Recognition
Authors: Yichun Shi, Xiang Yu, Kihyuk Sohn, Manmohan Chandraker, Anil K. Jain
Units: Michigan State University, NEC Labs America, University of California, San Diego
Recognizing wild faces is extremely hard as they appear with all kinds of variations. Traditional methods either train with specifically annotated variation data from target domains, or by introducing unlabeled target variation data to adapt from the training data. Instead, we propose a universal representation learning framework that can deal with larger variation unseen in the given training data without leveraging target domain knowledge. We firstly synthesize training data alongside some semantically meaningful variations, such as low resolution, occlusion and head pose. However, directly feeding the augmented data for training will not converge well as the newly introduced samples are mostly hard examples. We propose to split the feature embedding into multiple sub-embeddings, and associate different confidence values for each sub-embedding to smooth the training procedure. The sub-embeddings are further decorrelated by regularizing variation classification loss and variation adversarial loss on different partitions of them. Experiments show that our method achieves top performance on general face recognition datasets such as LFW and MegaFace, while significantly better on extreme benchmarks such as TinyFace and IJB-S.
1-9. Rotation Consistent Margin Loss for Efficient Low-Bit Face Recognition
Authors: Yudong Wu, Yichao Wu, Ruihao Gong, Yuanhao Lv, Ken Chen, Ding Liang, Xiaolin Hu, Xianglong Liu, Junjie Yan
Units: SenseTime Group Limited, BeiHang University, Tsinghua University
In this paper, we consider the low-bit quantization problem of face recognition (FR) under the open-set protocol. Different from well explored low-bit quantization on closed-set image classification task, the open-set task is more sensitive to quantization errors (QEs). We redefine the QEs in angular space and disentangle it into class error and individual error. These two parts correspond to inter-class separability and intra-class compactness, respectively. Instead of eliminating the entire QEs, we propose the rotation consistent margin (RCM) loss to minimize the individual error, which is more essential to feature discriminative power. Extensive experiments on popular benchmark datasets such as MegaFace Challenge, Youtube Faces (YTF), Labeled Face in the Wild (LFW) and IJB-C show the superiority of proposed loss in low-bit FR quantization tasks.
1-9. Domain Balancing: Face Recognition on Long-Tailed Domains
Authors: Dong Cao, Xiangyu Zhu, Xingyu Huang, Jianzhu Guo, Zhen Lei
Units: CBSR & NLPR, Institute of Automation, Chinese Academy of Sciences, Beijing /China, School of Artificial Intelligence, University of Chinese Academy of Sciences, Beijing /China, Tianjin University
Long-tailed problem has been an important topic in face recognition task. However, existing methods only concentrate on the long-tailed distribution of classes. Differently, we devote to the long-tailed domain distribution problem, which refers to the fact that a small number of domains frequently appear while other domains far less existing. The key challenge of the problem is that domain labels are too complicated (related to race, age, pose, illumination, etc.) and inaccessible in real applications. In this paper, we propose a novel Domain Balancing (DB) mechanism to handle this problem. Specifically, we first propose a Domain Frequency Indicator (DFI) to judge whether a sample is from head domains or tail domains. Secondly, we formulate a light-weighted Residual Balancing Mapping (RBM) block to balance the domain distribution by adjusting the network according to DFI. Finally, we propose a Domain Balancing Margin (DBM) in the loss function to further optimize the feature space of the tail domains to improve generalization. Extensive analysis and experiments on several face recognition benchmarks demonstrate that the proposed method effectively enhances the generalization capacities and achieves superior performance.
1-10. RDCFace: Radial Distortion Correction for Face Recognition
Authors: He Zhao, Xianghua Ying, Yongjie Shi, Xin Tong, Jingsi Wen, Hongbin Zha
Units: Key Laboratory of Machine Perception (MOE), School of EECS, Peking University
The effects of radial lens distortion often appear in wide-angle cameras of surveillance and safeguard systems, which may severely degrade performances of previous face recognition algorithms. Traditional methods for radial lens distortion correction usually employ line features in scenarios that are not suitable for face images. In this paper, we propose a distortion-invariant face recognition system called RDCFace, which directly and only utilize the distorted images of faces, to alleviate the effects of radial lens distortion. RDCFace is an end-to-end trainable cascade network, which can learn rectification and alignment parameters to achieve a better face recognition performance without requiring supervision of facial landmarks and distortion parameters. We design sequential spatial transformer layers to optimize the correction, alignment, and recognition modules jointly. The feasibility of our method comes from implicitly using the statistics of the layout of face features learned from the large-scale face data. Extensive experiments indicate that our method is distortion robust and gains significant improvements on LFW, YTF, CFP, and RadialFace, a real distorted face benchmark compared with state-of-the-art methods.
1-11. Global-Local GCN: Large-Scale Label Noise Cleansing for Face Recognition
Authors: Yaobin Zhang, Weihong Deng, Mei Wang, Jiani Hu, Xian Li, Dongyue Zhao, Dongchao Wen
Units: Beijing University of Posts and Telecommunications, Canon Information Technology (Beijing) Co., Ltd
In the field of face recognition, large-scale web-collected datasets are essential for learning discriminative representations, but they suffer from noisy identity labels, such as outliers and label flips. It is beneficial to automatically cleanse their label noise for improving recognition accuracy. Unfortunately, existing cleansing methods cannot accurately identify noise in the wild. To solve this problem, we propose an effective automatic label noise cleansing framework for face recognition datasets, FaceGraph. Using two cascaded graph convolutional networks, FaceGraph performs global-to-local discrimination to select useful data in a noisy environment. Extensive experiments show that cleansing widely used datasets, such as CASIA-WebFace, VGGFace2, MegaFace2, and MS-Celeb-1M, using the proposed method can improve the recognition performance of state-of-the-art representation learning methods like Arcface. Further, we cleanse massive self-collected celebrity data, namely MillionCelebs, to provide 18.8M images of 636K identities. Training with the new data, Arcface surpasses state-of-the-art performance by a notable margin to reach 95.62% TPR at 1e-5 FPR on the IJB-C benchmark.
1-12. FM2u-Net: Face Morphological Multi-Branch Network for Makeup-Invariant Face Verification
Authors: Wenxuan Wang, Yanwei Fu, Xuelin Qian, Yu-Gang Jiang, Qi Tian, Xiangyang Xue
Units: Fudan University, China 2Noah’s Ark Lab, Huawei Technologies
It is challenging in learning a makeup-invariant face verification model, due to (1) insufficient makeup/non-makeup face training pairs, (2) the lack of diverse makeup faces, and (3) the significant appearance changes caused by cosmetics. To address these challenges, we propose a unified Face Morphological Multi-branch Network (FMMu-Net) for makeup-invariant face verification, which can simultaneously synthesize many diverse makeup faces through face morphology network (FM-Net) and effectively learn cosmetics-robust face representations using attention-based multi-branch learning network (AttM-Net). For challenges (1) and (2), FM-Net (two stacked auto-encoders) can synthesize realistic makeup face images by transferring specific regions of cosmetics via cycle consistent loss. For challenge (3), AttM-Net, consisting of one global and three local (task-driven on two eyes and mouth) branches, can effectively capture the complementary holistic and detailed information. Unlike DeepID2 which uses simple concatenation fusion, we introduce a heuristic method AttM-FM, attached to AttM-Net, to adaptively weight the features of different branches guided by the holistic information. We conduct extensive experiments on makeup face verification benchmarks (M-501, M-203, and FAM) and general face recognition datasets (LFW and IJB-A). Our framework FMMu-Net achieves state-of-the-art performances.
2. 顔検出(3)
2-1. RetinaFace: Single-Shot Multi-Level Face Localisation in the Wild
Authors: Jiankang Deng, Jia Guo, Evangelos Ververas, Irene Kotsia, Stefanos Zafeiriou
Units: Imperial College, InsightFace, FaceSoft, Middlesex University London
Though tremendous strides have been made in uncontrolled face detection, accurate and efficient 2D face alignment and 3D face reconstruction in-the-wild remain an open challenge. In this paper, we present a novel single-shot, multi-level face localisation method, named RetinaFace, which unifies face box prediction, 2D facial landmark localisation and 3D vertices regression under one common target: point regression on the image plane. To fill the data gap, we manually annotated five facial landmarks on the WIDER FACE dataset and employed a semi-automatic annotation pipeline to generate 3D vertices for face images from the WIDER FACE, AFLW and FDDB datasets. Based on extra annotations, we propose a mutually beneficial regression target for 3D face reconstruction, that is predicting 3D vertices projected on the image plane constrained by a common 3D topology. The proposed 3D face reconstruction branch can be easily incorporated, without any optimisation difficulty, in parallel with the existing box and 2D landmark regression branches during joint training. Extensive experimental results show that RetinaFace can simultaneously achieve stable face detection, accurate 2D face alignment and robust 3D face reconstruction while being efficient through single-shot inference.
2-2. BFBox: Searching Face-Appropriate Backbone and Feature Pyramid Network for Face Detector
Authors: Yang Liu, Xu Tang
Units: North China Electric Power University, Baidu, Inc.
Popular backbones designed on image classification have demonstrated their considerable compatibility on the task of general object detection. However, the same phenomenon does not appear on the face detection. This is largely due to the average scale of ground-truth in the WiderFace dataset is far smaller than that of generic objects in theCOCO one. To resolve this, the success of Neural Archi-tecture Search (NAS) inspires us to search face-appropriate backbone and featrue pyramid network (FPN) architecture.Firstly, we design the search space for backbone and FPN by comparing performance of feature maps with different backbones and excellent FPN architectures on the face detection. Second, we propose a FPN-attention module to joint search the architecture of backbone and FPN. Finally,we conduct comprehensive experiments on popular bench-marks, including Wider Face, FDDB, AFW and PASCALFace, display the superiority of our proposed method.
2-3. HAMBox: Delving Into Mining High-Quality Anchors on Face Detection
Authors: Yang Liu, Xu Tang, Xiang Wu, Junyu Han, Jingtuo Liu, Errui Ding
Units: Department of Computer Vision Technology (VIS), Baidu Inc., National Laboratory of Pattern Recognition, Institute of Automation, Chinese Academy of Sciences.
Current face detectors utilize anchors to frame a multi-task learning problem which combines classification and bounding box regression. Effective anchor design and anchor matching strategy enable face detectors to localize faces under large pose and scale variations. However, we observe that more than 80% correctly predicted bounding boxes are regressed from the unmatched anchors (the IoUs between anchors and target faces are lower than a threshold) in the inference phase. It indicates that these unmatched anchors perform excellent regression ability, but the existing methods neglect to learn from them. In this paper, we propose an Online High-quality Anchor Mining Strategy (HAMBox), which explicitly helps outer faces compensate with high-quality anchors. Our proposed HAMBox method could be a general strategy for anchor-based single-stage face detection. Experiments on various datasets, including WIDER FACE, FDDB, AFW and PASCAL Face, demonstrate the superiority of the proposed method. Furthermore, our team win the championship on the Face Detection test track of WIDER Face and Pedestrian Challenge 2019. We will release the codes with PaddlePaddle.
3. なりすまし検出(4)
3-1. Searching Central Difference Convolutional Networks for Face Anti-Spoofing
Authors: Zitong Yu, Chenxu Zhao, Zezheng Wang, Yunxiao Qin, Zhuo Su, Xiaobai Li, Feng Zhou, Guoying Zhao
Units: CMVS, University of Oulu, Mininglamp Academy of Sciences, Mininglamp Technology, Aibee, Northwestern Polytechnical University
Face anti-spoofing (FAS) plays a vital role in face recognition systems. Most state-of-the-art FAS methods 1) rely on stacked convolutions and expert-designed network, which is weak in describing detailed fine-grained information and easily being ineffective when the environment varies (e.g., different illumination), and 2) prefer to use long sequence as input to extract dynamic features, making them difficult to deploy into scenarios which need quick response. Here we propose a novel frame level FAS method based on Central Difference Convolution (CDC), which is able to capture intrinsic detailed patterns via aggregating both intensity and gradient information. A network built with CDC, called the Central Difference Convolutional Network (CDCN), is able to provide more robust modeling capacity than its counterpart built with vanilla convolution. Furthermore, over a specifically designed CDC search space, Neural Architecture Search (NAS) is utilized to discover a more powerful network structure (CDCN++), which can be assembled with Multiscale Attention Fusion Module (MAFM) for further boosting performance. Comprehensive experiments are performed on six benchmark datasets to show that 1) the proposed method not only achieves superior performance on intra-dataset testing (especially 0.2% ACER in Protocol-1 of OULU-NPU dataset), 2) it also generalizes well on cross-dataset testing (particularly 6.5% HTER from CASIA-MFSD to Replay-Attack datasets). The codes are available at https://github.com/ZitongYu/CDCN.
Authors: Guoqing Wang, Hu Han, Shiguang Shan, Xilin Chen
Units: Key Laboratory of Intelligent Information Processing of Chinese Academy of Sciences (CAS), Institute of Computing Technology, CAS, Beijing /China, University of Chinese Academy of Sciences, Beijing /China, Peng Cheng Laboratory, Shenzhen /China
Face presentation attack detection (PAD) has been an urgent problem to be solved in the face recognition systems. Conventional approaches usually assume the testing and training are within the same domain; as a result, they may not generalize well into unseen scenarios because the representations learned for PAD may overfit to the subjects in the training set. In light of this, we propose an efficient disentangled representation learning for cross-domain face PAD. Our approach consists of disentangled representation learning (DR-Net) and multi-domain learning (MD-Net). DR-Net learns a pair of encoders via generative models that can disentangle PAD informative features from subject discriminative features. The disentangled features from different domains are fed to MD-Net which learns domain-independent features for the final cross-domain face PAD task. Extensive experiments on several public datasets validate the effectiveness of the proposed approach for cross-domain PAD.
3-3. Deep Spatial Gradient and Temporal Depth Learning for Face Anti-Spoofing
Authors: Zezheng Wang, Zitong Yu, Chenxu Zhao, Xiangyu Zhu, Yunxiao Qin, Qiusheng Zhou, Feng Zhou, Zhen Lei
Units: AIBEE, CMVS, University of Oulu, Academy of Sciences, Mininglamp Technology, CBSR&NLPR, CASIA, Northwestern Polytechnical University, JD DigitsCBSR&NLPR, CASIA, Northwestern Polytechnical University, JD Digits
Face anti-spoofing is critical to the security of face recognition systems. Depth supervised learning has been proven as one of the most effective methods for face anti-spoofing. Despite the great success, most previous works still formulate the problem as a single-frame multi-task one by simply augmenting the loss with depth, while neglecting the detailed fine-grained information and the interplay between facial depths and moving patterns. In contrast, we design a new approach to detect presentation attacks from multiple frames based on two insights: 1) detailed discriminative clues (e.g., spatial gradient magnitude) between living and spoofing face may be discarded through stacked vanilla convolutions, and 2) the dynamics of 3D moving faces provide important clues in detecting the spoofing faces. The proposed method is able to capture discriminative details via Residual Spatial Gradient Block (RSGB) and encode spatio-temporal information from Spatio-Temporal Propagation Module (STPM) efficiently. Moreover, a novel Contrastive Depth Loss is presented for more accurate depth supervision. To assess the efficacy of our method, we also collect a Double-modal Anti-spoofing Dataset (DMAD) which provides actual depth for each sample. The experiments demonstrate that the proposed approach achieves state-of-the-art results on five benchmark datasets including OULU-NPU, SiW, CASIA-MFSD, Replay-Attack, and the new DMAD. Codes will be available at this https URL.
3-4. Single-Side Domain Generalization for Face Anti-Spoofing
Authors: Yunpei Jia, Jie Zhang, Shiguang Shan, Xilin Chen
Units: Key Lab of Intelligent Information Processing of Chinese Academy of Sciences (CAS), Institute of Computing Technology, CAS, Beijing /China, University of Chinese Academy of Sciences, Beijing /China, CAS Center for Excellence in Brain Science and Intelligence Technology, Shanghai /China
Existing domain generalization methods for face anti-spoofing endeavor to extract common differentiation features to improve the generalization. However, due to large distribution discrepancies among fake faces of different domains, it is difficult to seek a compact and generalized feature space for the fake faces. In this work, we propose an end-to-end single-side domain generalization framework (SSDG) to improve the generalization ability of face anti-spoofing. The main idea is to learn a generalized feature space, where the feature distribution of the real faces is compact while that of the fake ones is dispersed among domains but compact within each domain. Specifically, a feature generator is trained to make only the real faces from different domains undistinguishable, but not for the fake ones, thus forming a single-side adversarial learning. Moreover, an asymmetric triplet loss is designed to constrain the fake faces of different domains separated while the real ones aggregated. The above two points are integrated into a unified framework in an end-to-end training manner, resulting in a more generalized class boundary, especially good for samples from novel domains. Feature and weight normalization is incorporated to further improve the generalization ability. Extensive experiments show that our proposed approach is effective and outperforms the state-of-the-art methods on four public databases.
4. 顔偽造検出(4)
4-1. Face X-Ray for More General Face Forgery Detection
Authors: Lingzhi Li, Jianmin Bao, Ting Zhang, Hao Yang, Dong Chen, Fang Wen, Baining Guo
Units: Peking University, Microsoft Research Asia
In this paper we propose a novel image representation called face X-ray for detecting forgery in face images. The face X-ray of an input face image is a greyscale image that reveals whether the input image can be decomposed into the blending of two images from different sources. It does so by showing the blending boundary for a forged image and the absence of blending for a real image. We observe that most existing face manipulation methods share a common step: blending the altered face into an existing background image. For this reason, face X-ray provides an effective way for detecting forgery generated by most existing face manipulation algorithms. Face X-ray is general in the sense that it only assumes the existence of a blending step and does not rely on any knowledge of the artifacts associated with a specific face manipulation technique. Indeed, the algorithm for computing face X-ray can be trained without fake images generated by any of the state-of-the-art face manipulation methods. Extensive experiments show that face X-ray remains effective when applied to forgery generated by unseen face manipulation techniques, while most existing face forgery detection or deepfake detection algorithms experience a significant performance drop.
4-2. On the Detection of Digital Face Manipulation
Authors: Hao Dang, Feng Liu, Joel Stehouwer, Xiaoming Liu, Anil Jain
Units: Department of Computer Science and Engineering Michigan State University
Detecting manipulated facial images and videos is an increasingly important topic in digital media forensics. As advanced face synthesis and manipulation methods are made available, new types of fake face representations are being created which have raised significant concerns for their use in social media. Hence, it is crucial to detect manipulated face images and localize manipulated regions. Instead of simply using multi-task learning to simultaneously detect manipulated images and predict the manipulated mask (regions), we propose to utilize an attention mechanism to process and improve the feature maps for the classification task. The learned attention maps highlight the informative regions to further improve the binary classification (genuine face v. fake face), and also visualize the manipulated regions. To enable our study of manipulated face detection and localization, we collect a large-scale database that contains numerous types of facial forgeries. With this dataset, we perform a thorough analysis of data-driven fake face detection. We show that the use of an attention mechanism improves facial forgery detection and manipulated region localization.
4-3. Global Texture Enhancement for Fake Face Detection in the Wild
Authors: Zhengzhe Liu, Xiaojuan Qi, Philip H.S. Torr
Units: University of Oxford, The University of Hong Kong
Generative Adversarial Networks (GANs) can generate realistic fake face images that can easily fool human beings. On the contrary, a common Convolutional Neural Network(CNN) discriminator can achieve more than99.9%accuracyin discerning fake/real images. In this paper, we conduct an empirical study on fake/real faces, and have two important observations: firstly, the texture of fake faces is substantially different from real ones; secondly, global texture statistics are more robust to image editing and transferable to fake faces from different GANs and datasets. Motivated by the above observations, we propose a new architecture coined as Gram-Net, which leverages global image texture representations for robust fake image detection. Experimental results on several datasets demonstrate that our Gram-Netoutperforms existing approaches. Especially, our Gram-Netis more robust to image editings, e.g. down-sampling, JPEGcompression, blur, and noise. More importantly, our Gram-Net generalizes significantly better in detecting fake faces from GAN models not seen in the training phase and can perform decently in detecting fake natural images.
4-4. DeeperForensics-1.0: A Large-Scale Dataset for Real-World Face Forgery Detection
Authors: Liming Jiang, Ren Li, Wayne Wu, Chen Qian, Chen Change Loy
Units: Nanyang Technological University, SenseTime Research
We present our on-going effort of constructing a large- scale benchmark for face forgery detection. The first version of this benchmark, DeeperForensics-1.0, represents the largest face forgery detection dataset by far, with 60, 000 videos constituted by a total of 17.6 million frames, 10 times larger than existing datasets of the same kind. Extensive real-world perturbations are applied to obtain a more challenging benchmark of larger scale and higher diversity. All source videos in DeeperForensics-1.0 are carefully collected, and fake videos are generated by a newly proposed end-to-end face swapping framework. The quality of generated videos outperforms those in existing datasets, validated by user studies. The benchmark features a hidden test set, which contains manipulated videos achieving high deceptive scores in human evaluations. We further contribute a comprehensive study that evaluates five representative detection baselines and make a thorough analysis of different settings.
5. 表情認識(2)
5-1. Label Distribution Learning on Auxiliary Label Space Graphs for Facial Expression Recognition
Authors: Shikai Chen, Jianfeng Wang, Yuedong Chen, Zhongchao Shi, Xin Geng, Yong Rui
Units: Southeast University, University of Oxford, Nanyang Technological University , AI Lab, Lenovo Research
Many existing studies reveal that annotation inconsistency widely exists among a variety of facial expression recognition (FER) datasets. The reason might be the subjectivity of human annotators and the ambiguous nature of the expression labels. One promising strategy tackling such a problem is a recently proposed learning paradigm called Label Distribution Learning (LDL), which allows multiple labels with different intensity to be linked to one expression. However, it is often impractical to directly apply label distribution learning because numerous existing datasets only contain one-hot labels rather than label distributions. To solve the problem, we propose a novel approach named Label Distribution Learning on Auxiliary Label Space Graphs(LDL-ALSG) that leverages the topological information of the labels from related but more distinct tasks, such as action unit recognition and facial landmark detection. The underlying assumption is that facial images should have similar expression distributions to their neighbours in the label space of action unit recognition and facial landmark detection. Our proposed method is evaluated on a variety of datasets and outperforms those state-of-the-art methods consistently with a huge margin.
5-2. Suppressing Uncertainties for Large-Scale Facial Expression Recognition
Authors: Kai Wang, Xiaojiang Peng, Jianfei Yang, Shijian Lu, Yu Qiao
Units: ShenZhen Key Lab of Computer Vision and Pattern Recognition, SIAT-SenseTime Joint Lab, Shenzhen Institutes of Advanced Technology, Chinese Academy of Science, University of Chinese Academy of Sciences, China, Nanyang Technological University Singapore
Annotating a qualitative large-scale facial expression dataset is extremely difficult due to the uncertainties caused by ambiguous facial expressions, low-quality facial images, and the subjectiveness of annotators. These uncertainties lead to a key challenge of large-scale Facial Expression Recognition (FER) in deep learning era. To address this problem, this paper proposes a simple yet efficient Self-Cure Network (SCN) which suppresses the uncertainties efficiently and prevents deep networks from over-fitting uncertain facial images. Specifically, SCN suppresses the uncertainty from two different aspects: 1) a self-attention mechanism over mini-batch to weight each training sample with a ranking regularization, and 2) a careful relabeling mechanism to modify the labels of these samples in the lowest-ranked group. Experiments on synthetic FER datasets and our collected WebEmotion dataset validate the effectiveness of our method. Results on public benchmarks demonstrate that our SCN outperforms current state-of-the-art methods with \textbf{88.14}\% on RAF-DB, \textbf{60.23}\% on AffectNet, and \textbf{89.35}\% on FERPlus. The code will be available at \href{this https URL}{this https URL}.
6. 顔の高解像度化(2)
6-1. Learning to Have an Ear for Face Super-Resolution
Authors: Givi Meishvili, Simon Jenni, Paolo Favaro
Units: University of Bern, Switzerland
We propose a novel method to use both audio and a low-resolution image to perform extreme face super-resolution (a 16x increase of the input size). When the resolution of the input image is very low (e.g., 8x8 pixels), the loss of information is so dire that important details of the original identity have been lost and audio can aid the recovery of a plausible high-resolution image. In fact, audio carries information about facial attributes, such as gender and age. To combine the aural and visual modalities, we propose a method to first build the latent representations of a face from the lone audio track and then from the lone low-resolution image. We then train a network to fuse these two representations. We show experimentally that audio can assist in recovering attributes such as the gender, the age and the identity, and thus improve the correctness of the high-resolution image reconstruction process. Our procedure does not make use of human annotation and thus can be easily trained with existing video datasets. Moreover, we show that our model builds a factorized representation of images and audio as it allows one to mix low-resolution images and audio from different videos and to generate realistic faces with semantically meaningful combinations.
Authors: Cheng Ma, Zhenyu Jiang, Yongming Rao, Jiwen Lu, Jie Zhouo
Units: Department of Automation, Tsinghua University /China, State Key Lab of Intelligent Technologies and Systems /China, Beijing National Research Center for Information Science and Technology /China, Tsinghua Shenzhen International Graduate School, Tsinghua University /China
Recent works based on deep learning and facial priors have succeeded in super-resolving severely degraded facial images. However, the prior knowledge is not fully exploited in existing methods, since facial priors such as landmark and component maps are always estimated by low-resolution or coarsely super-resolved images, which may be inaccurate and thus affect the recovery performance. In this paper, we propose a deep face super-resolution (FSR) method with iterative collaboration between two recurrent networks which focus on facial image recovery and landmark estimation respectively. In each recurrent step, the recovery branch utilizes the prior knowledge of landmarks to yield higher-quality images which facilitate more accurate landmark estimation in turn. Therefore, the iterative information interaction between two processes boosts the performance of each other progressively. Moreover, a new attentive fusion module is designed to strengthen the guidance of landmark maps, where facial components are generated individually and aggregated attentively for better restoration. Quantitative and qualitative experimental results show the proposed method significantly outperforms state-of-the-art FSR methods in recovering high-quality face images.
7. 3D顔再構成(5)
Authors: Jiangke Lin, Yi Yuan, Tianjia Shao, Kun Zhou
Units: NetEase Fuxi AI Lab, Hangzhou /China, State Key Lab of CAD&CG, Zhejiang University, Hangzhou /China
3D Morphable Model (3DMM) based methods have achieved great success in recovering 3D face shapes from single-view images. However, the facial textures recovered by such methods lack the fidelity as exhibited in the input images. Recent work demonstrates high-quality facial texture recovering with generative networks trained from a large-scale database of high-resolution UV maps of face textures, which is hard to prepare and not publicly available. In this paper, we introduce a method to reconstruct 3D facial shapes with high-fidelity textures from single-view images in-the-wild, without the need to capture a large-scale face texture database. The main idea is to refine the initial texture generated by a 3DMM based method with facial details from the input image. To this end, we propose to use graph convolutional networks to reconstruct the detailed colors for the mesh vertices instead of reconstructing the UV map. Experiments show that our method can generate high-quality results and outperforms state-of-the-art methods in both qualitative and quantitative comparisons.
7-2. ReDA:Reinforced Differentiable Attribute for 3D Face Reconstruction
Authors: Wenbin Zhu HsiangTao Wu Zeyu Chen Noranart Vesdapunt Baoyuan Wang
Units: Microsoft Cloud and AI
The key challenge for 3D face shape reconstruction is to build the correct dense face correspondence between the deformable mesh and the single input image. Given the ill-posed nature, previous works heavily rely on prior knowledge (such as 3DMM [2]) to reduce depth ambiguity. Although impressive result has been made recently [42, 14, 8], there is still a large room to improve the correspondence so that projected face shape better aligns with the silhouette of each face region (i.e, eye, mouth, nose, cheek, etc.) on the image. To further reduce the ambiguities, we present a novel framework called “Reinforced Differentiable Attributes” (“ReDA”) which is more general and effective than previous Differentiable Rendering (“DR”). Specifically, we first extend from color to more broad attributes, including the depth and the face parsing mask. Secondly, unlike the previous Z-buffer rendering, we make the rendering to be more differentiable through a set of convolution operations with multi-scale kernel sizes. In the meanwhile, to make “ReDA” to be more successful for 3D face recon-struction, we further introduce a new free-form deformation layer that sits on top of 3DMM to enjoy both the prior knowledge and out-of-space modeling. Both techniques can be easily integrated into existing 3D face reconstruction pipeline. Extensive experiments on both RGB and RGB-D datasets show that our approach outperforms prior arts.
7-3. AvatarMe: Realistically Renderable 3D Facial Reconstruction "In-the-Wild"
Authors: Alexandros Lattas, Stylianos Moschoglou, Baris Gecer, Stylianos Ploumpis, Vasileios Triantafyllou, Abhijeet Ghosh, Stefanos Zafeiriou
Units: Imperial College London /UK, FaceSoft.io
Over the last years, with the advent of Generative Adversarial Networks (GANs), many face analysis tasks have accomplished astounding performance, with applications including, but not limited to, face generation and 3D face reconstruction from a single "in-the-wild" image. Nevertheless, to the best of our knowledge, there is no method which can produce high-resolution photorealistic 3D faces from "in-the-wild" images and this can be attributed to the: (a) scarcity of available data for training, and (b) lack of robust methodologies that can successfully be applied on very high-resolution data. In this paper, we introduce AvatarMe, the first method that is able to reconstruct photorealistic 3D faces from a single "in-the-wild" image with an increasing level of detail. To achieve this, we capture a large dataset of facial shape and reflectance and build on a state-of-the-art 3D texture and shape reconstruction method and successively refine its results, while generating the per-pixel diffuse and specular components that are required for realistic rendering. As we demonstrate in a series of qualitative and quantitative experiments, AvatarMe outperforms the existing arts by a significant margin and reconstructs authentic, 4K by 6K-resolution 3D faces from a single low-resolution image that, for the first time, bridges the uncanny valley.
7-4. Uncertainty-Aware Mesh Decoder for High Fidelity 3D Face Reconstruction
Authors: Gun-Hee Lee, Seong-Whan Lee
Units: Department of Computer and Radio Communications Engineering, Korea University, Department of Artificial Intelligence, Korea University
3D Morphable Model (3DMM) is a statistical model of facial shape and texture using a set of linear basis functions. Most of the recent 3D face reconstruction methods aim to embed the 3D morphable basis functions into Deep Convolutional Neural Network (DCNN). However, balancing the requirements of strong regularization for global shape and weak regularization for high level details is still ill-posed. To address this problem, we properly control generality and specificity in terms of regularization by harnessing the power of uncertainty. Additionally, we focus on the concept of nonlinearity and find out that Graph Convolutional Neural Network (Graph CNN) and Generative Adversarial Network (GAN) are effective in reconstructing high quality 3D shapes and textures respectively. In this paper, we propose to employ (i) an uncertainty-aware encoder that presents face features as distributions and (ii) a fully nonlinear decoder model combining Graph CNN with GAN. We demonstrate how our method builds excellent high quality results and outperforms previous state-of-the-art methods on 3D face reconstruction tasks for both constrained and in-the-wild images.
7-5. Deep Facial Non-Rigid Multi-View Stereo
Authors: Ziqian Bai, Zhaopeng Cui, Jamal Ahmed Rahim, Xiaoming Liu, Ping Tan
Units: Simon Fraser University, ETH Zurich, Michigan State University
We present a method for 3D face reconstruction from multi-view images with different expressions. We formulate this problem from the perspective of non-rigid multi-view stereo (NRMVS). Unlike previous learning-based methods, which often regress the face shape directly, our method optimizes the 3D face shape by explicitly enforcing multi-view appearance consistency, which is known to be effective in recovering shape details according to conventional multi-view stereo methods. Furthermore, by estimating face shape through optimization based on multi-view consistency, our method can potentially have better generalization to unseen data. However, this optimization is challenging since each input image has a different expression. We facilitate it with a CNN network that learns to regularize the non-rigid 3D face according to the input image and preliminary optimization results. Extensive experiments show that our method achieves the state-of-the-art performance on various datasets and generalizes well to in-the-wild data.
8. 顔画像修正(2)
8-1. Enhanced Blind Face Restoration With Multi-Exemplar Images and Adaptive Spatial Feature Fusion
Authors: Xiaoming Li, Wenyu Li, Dongwei Ren, Hongzhi Zhang, Meng Wang, Wangmeng Zuo
Units: School of Computer Science and Technology, Harbin Institute of Technology, College of Intelligence and Computing, Tianjin University, Hefei University of Technology
In many real-world face restoration applications, e.g., smartphone photo albums and old films, multiple high-quality (HQ) images of the same person usually are available for a given degraded low-quality (LQ) observation. However, most existing guided face restoration methods are based on single HQ exemplar image, and are limited in properly exploiting guidance for improving the generalization ability to unknown degradation process. To address these issues, this paper suggests to enhance blind face restoration performance by utilizing multi-exemplar images and adaptive fusion of features from guidance and degraded images. First, given a degraded observation, we select the optimal guidance based on the weighted affine distance on landmark sets, where the landmark weights are learned to make the guidance image optimized to HQ image reconstruction. Second, moving least-square and adaptive instance normalization are leveraged for spatial alignment and illumination translation of guidance image in the feature space. Finally, for better feature fusion, multiple adaptive spatial feature fusion (ASFF) layers are introduced to incorporate guidance features in an adaptive and progressive manner, resulting in our ASFFNet. Experiments show that our ASFFNet performs favorably in terms of quantitative and qualitative evaluation, and is effective in generating photo-realistic results on real-world LQ images. The source code and models are available at https://github.com/csxmli2016/ASFFNet.
8-2. Lightweight Photometric Stereo for Facial Details Recovery
Authors: Xueying Wang, Yudong Guo, Bailin Deng, Juyong Zhang
Units: University of Science and Technology of China, Cardiff University
Recently, 3D face reconstruction from a single image has achieved great success with the help of deep learning and shape prior knowledge, but they often fail to produce accurate geometry details. On the other hand, photometric stereo methods can recover reliable geometry details, but require dense inputs and need to solve a complex optimization problem. In this paper, we present a lightweight strategy that only requires sparse inputs or even a single image to recover high-fidelity face shapes with images captured under near-field lights. To this end, we construct a dataset containing 84 different subjects with 29 expressions under 3 different lights. Data augmentation is applied to enrich the data in terms of diversity in identity, lighting, expression, etc. With this constructed dataset, we propose a novel neural network specially designed for photometric stereo based 3D face reconstruction. Extensive experiments and comparisons demonstrate that our method can generate high-quality reconstruction results with one to three facial images captured under near-field lights. Our full framework is available at this https URL.
9. 顔画質評価(1)
9-1. SER-FIQ: Unsupervised Estimation of Face Image Quality Based on Stochastic Embedding Robustness
Authors: Philipp Terhorst, Jan Niklas Kolf, Naser Damer, Florian Kirchbuchner, Arjan Kuijper
Units: Fraunhofer Institute for Computer Graphics Research IGD, Technical University of Darmstadt
Face image quality is an important factor to enable high performance face recognition systems. Face quality assessment aims at estimating the suitability of a face image for recognition. Previous work proposed supervised solutions that require artificially or human labelled quality values. However, both labelling mechanisms are error-prone as they do not rely on a clear definition of quality and may not know the best characteristics for the utilized face recognition system. Avoiding the use of inaccurate quality labels, we proposed a novel concept to measure face quality based on an arbitrary face recognition model. By determining the embedding variations generated from random subnetworks of a face model, the robustness of a sample representation and thus, its quality is estimated. The experiments are conducted in a cross-database evaluation setting on three publicly available databases. We compare our proposed solution on two face embeddings against six state-of-the-art approaches from academia and industry. The results show that our unsupervised solution outperforms all other approaches in the majority of the investigated scenarios. In contrast to previous works, the proposed solution shows a stable performance over all scenarios. Utilizing the deployed face recognition model for our face quality assessment methodology avoids the training phase completely and further outperforms all baseline approaches by a large margin. Our solution can be easily integrated into current face recognition systems and can be modified to other tasks beyond face recognition.
10. 顔のクラスタリング(2)
10-1. Density-Aware Feature Embedding for Face Clustering
Authors: Senhui Guo, Jing Xu, Dapeng Chen, Chao Zhang, Xiaogang Wang, Rui Zhao
Units: SenseTime Group Limited, Samsung Research China - Beijing(SRC-B), CUHK
Clustering has many applications in research and industry. However, traditional clustering methods, such as K-means, DBSCAN and HAC, impose oversimplifying assumptions and thus are not well-suited to face clustering. To adapt to the distribution of realistic problems, a natural approach is to use Graph Convolutional Networks (GCNs) to enhance features for clustering. However, GCNs can only utilize local information, which ignores the overall characterisitcs of the clusters. In this paper, we propose a Density-Aware Feature Embedding Network (DA-Net) for the task of face clustering, which utilizes both local and non-local information, to learn a robust feature embedding. Specifically, DA-Net uses GCNs to aggregate features locally, and then incorporates non-local information using a density chain, which is a chain of faces from low density to high density. This density chain exploits the non-uniform distribution of face images in the dataset. Then, an LSTM takes the density chain as input to generate the final feature embedding. Once this embedding is generated, traditional clustering methods, such as density-based clustering, can be used to obtain the final clustering results. Extensive experiments verify the effectiveness of the proposed feature embedding method, which can achieve state-of-the-art performance on public benchmarks.
10-2. Learning to Cluster Faces via Confidence and Connectivity Estimation
Authors: Lei Yang, Dapeng Chen, Xiaohang Zhan, Rui Zhao, Chen Change Loy, Dahua Lin
Units: The Chinese University of Hong Kong, SenseTime Group Limited, Nanyang Technological University
Face clustering is an essential tool for exploiting the unlabeled face data, and has a wide range of applications including face annotation and retrieval. Recent works show that supervised clustering can result in noticeable performance gain. However, they usually involve heuristic steps and require numerous overlapped subgraphs, severely restricting their accuracy and efficiency. In this paper, we propose a fully learnable clustering framework without requiring a large number of overlapped subgraphs. Instead, we transform the clustering problem into two sub-problems. Specifically, two graph convolutional networks, named GCN-V and GCN-E, are designed to estimate the confidence of vertices and the connectivity of edges, respectively. With the vertex confidence and edge connectivity, we can naturally organize more relevant vertices on the affinity graph and group them into clusters. Experiments on two large-scale benchmarks show that our method significantly improves clustering accuracy and thus performance of the recognition models trained on top, yet it is an order of magnitude more efficient than existing supervised methods.
11. 顔の位置合わせ(3)
11-1. LUVLi Face Alignment: Estimating Landmarks' Location, Uncertainty, and Visibility Likelihood
Authors: Abhinav Kumar, Tim K. Marks, Wenxuan Mou, Ye Wang, Michael Jones, Anoop Cherian, Toshiaki Koike-Akino, Xiaoming Liu, Chen Feng
Units: University of Utah, Mitsubishi Electric Research Labs (MERL), University of Manchester, Michigan State University, 5New York University
Modern face alignment methods have become quite accurate at predicting the locations of facial landmarks, but they do not typically estimate the uncertainty of their predicted locations nor predict whether landmarks are visible. In this paper, we present a novel framework for jointly predicting landmark locations, associated uncertainties of these predicted locations, and landmark visibilities. We model these as mixed random variables and estimate them using a deep network trained with our proposed Location, Uncertainty, and Visibility Likelihood (LUVLi) loss. In addition, we release an entirely new labeling of a large face alignment dataset with over 19,000 face images in a full range of head poses. Each face is manually labeled with the ground-truth locations of 68 landmarks, with the additional information of whether each landmark is unoccluded, self-occluded (due to extreme head poses), or externally occluded. Not only does our joint estimation yield accurate estimates of the uncertainty of predicted landmark locations, but it also yields state-of-the-art estimates for the landmark locations themselves on multiple standard face alignment datasets. Our method's estimates of the uncertainty of predicted landmark locations could be used to automatically identify input images on which face alignment fails, which can be critical for downstream tasks.
11-2. 3FabRec: Fast Few-Shot Face Alignment by Reconstruction
Authors: Bjorn Browatzki, Christian Wallraven
Units: Dept. of Artificial Intelligence, Korea University
Current supervised methods for facial landmark detection require a large amount of training data and may suffer from overfitting to specific datasets due to the massive number of parameters. We introduce a semi-supervised method in which the crucial idea is to first generate implicit face knowledge from the large amounts of unlabeled images of faces available today. In a first, completely unsupervised stage, we train an adversarial autoencoder to reconstruct faces via a low-dimensional face embedding. In a second, supervised stage, we interleave the decoder with transfer layers to retask the generation of color images to the prediction of landmark heatmaps. Our framework (3FabRec) achieves state-of-the-art performance on several common benchmarks and, most importantly, is able to maintain impressive accuracy on extremely small training sets down to as few as 10 images. As the interleaved layers only add a low amount of parameters to the decoder, inference runs at several hundred FPS on a GPU.
11-3. Attention-Driven Cropping for Very High Resolution Facial Landmark Detection
Authors: Prashanth Chandran, Derek Bradley, Markus Gross, Thabo Beeler
Units: Department of Computer Science, ETH Zurich, DisneyResearch|Studios, Zurich
Facial landmark detection is a fundamental task for many consumer and high-end applications and is almost entirely solved by machine learning methods today. Existing datasets used to train such algorithms are primarily made up of only low resolution images, and current algorithms are limited to inputs of comparable quality and resolution as the training dataset. On the other hand, high resolution imagery is becoming increasingly more common as consumer cameras improve in quality every year. Therefore, there is need for algorithms that can leverage the rich information available in high resolution imagery. Naively attempting to reuse existing network architectures on high resolution imagery is prohibitive due to memory bottlenecks on GPUs. The only current solution is to downsample the images, sacrificing resolution and quality. Building on top of recent progress in attention-based networks, we present a novel, fully convolutional regional architecture that is specially designed for predicting landmarks on very high resolution facial images without downsampling. We demonstrate the flexibility of our architecture by training the proposed model with images of resolutions ranging from 256 x 256 to 4K. In addition to being the first method for facial landmark detection on high resolution images, our approach achieves superior performance over traditional (holistic) state-of-the-art architectures across ALL resolutions, leading to a general-purpose, extremely flexible, high quality landmark detector.
12. 顔のセグメンテーション(2)
12-1. Towards Learning Structure via Consensus for Face Segmentation and Parsing
Authors: Iacopo Masi, Joe Mathai, Wael AbdAlmageed
Units: USC Information Sciences Institute, Marina del Rey, CA, USA
Face segmentation is the task of densely labeling pixels on the face according to their semantics. While current methods place an emphasis on developing sophisticated architectures, use conditional random fields for smoothness, or rather employ adversarial training, we follow an alternative path towards robust face segmentation and parsing. Occlusions, along with other parts of the face, have a proper structure that needs to be propagated in the model during training. Unlike state-of-the-art methods that treat face segmentation as an independent pixel prediction problem, we argue instead that it should hold highly correlated outputs within the same object pixels. We thereby offer a novel learning mechanism to enforce structure in the prediction via consensus, guided by a robust loss function that forces pixel objects to be consistent with each other. Our face parser is trained by transferring knowledge from another model, yet it encourages spatial consistency while fitting the labels. Different than current practice, our method enjoys pixel-wise predictions, yet paves the way for fewer artifacts, less sparse masks, and spatially coherent outputs.
12-2. Dynamic Face Video Segmentation via Reinforcement Learning
Authors: Yujiang Wang, Mingzhi Dong, Jie Shen, Yang Wu, Shiyang Cheng, Maja Pantic
Units: Imperial College London, Samsung AI Center Cambridge, University College London, Nara Institue of Science and Technology
For real-time semantic video segmentation, most recent works utilised a dynamic framework with a key scheduler to make online key/non-key decisions. Some works used a fixed key scheduling policy, while others proposed adaptive key scheduling methods based on heuristic strategies, both of which may lead to suboptimal global performance. To overcome this limitation, we model the online key decision process in dynamic video segmentation as a deep reinforcement learning problem and learn an efficient and effective scheduling policy from expert information about decision history and from the process of maximising global return. Moreover, we study the application of dynamic video segmentation on face videos, a field that has not been investigated before. By evaluating on the 300VW dataset, we show that the performance of our reinforcement key scheduler outperforms that of various baselines in terms of both effective key selections and running speed. Further results on the Cityscapes dataset demonstrate that our proposed method can also generalise to other scenarios. To the best of our knowledge, this is the first work to use reinforcement learning for online key-frame decision in dynamic video segmentation, and also the first work on its application on face videos.
13. 顔編集(3)
13-1. Interpreting the Latent Space of GANs for Semantic Face Editing
Authors: Yujun Shen, Jinjin Gu, Xiaoou Tang, Bolei Zhou
Units: The Chinese University of Hong Kong, The Chinese University of Hong Kong, Shenzhen
Despite the recent advance of Generative Adversarial Networks (GANs) in high-fidelity image synthesis, there lacks enough understanding of how GANs are able to map a latent code sampled from a random distribution to a photo-realistic image. Previous work assumes the latent space learned by GANs follows a distributed representation but observes the vector arithmetic phenomenon. In this work, we propose a novel framework, called InterFaceGAN, for semantic face editing by interpreting the latent semantics learned by GANs. In this framework, we conduct a detailed study on how different semantics are encoded in the latent space of GANs for face synthesis. We find that the latent code of well-trained generative models actually learns a disentangled representation after linear transformations. We explore the disentanglement between various semantics and manage to decouple some entangled semantics with subspace projection, leading to more precise control of facial attributes. Besides manipulating gender, age, expression, and the presence of eyeglasses, we can even vary the face pose as well as fix the artifacts accidentally generated by GAN models. The proposed method is further applied to achieve real image manipulation when combined with GAN inversion methods or some encoder-involved models. Extensive results suggest that learning to synthesize faces spontaneously brings a disentangled and controllable facial attribute representation.
13-2. MaskGAN: Towards Diverse and Interactive Facial Image Manipulation
Authors: Cheng-Han Lee, Ziwei Liu, Lingyun Wu, Ping Luo
Units: SenseTime Research, The Chinese University of Hong Kong, The University of Hong Kong
Facial image manipulation has achieved great progress in recent years. However, previous methods either operate on a predefined set of face attributes or leave users little freedom to interactively manipulate images. To overcome these drawbacks, we propose a novel framework termed MaskGAN, enabling diverse and interactive face manipulation. Our key insight is that semantic masks serve as a suitable intermediate representation for flexible face manipulation with fidelity preservation. MaskGAN has two main components: 1) Dense Mapping Network (DMN) and 2) Editing Behavior Simulated Training (EBST). Specifically, DMN learns style mapping between a free-form user modified mask and a target image, enabling diverse generation results. EBST models the user editing behavior on the source mask, making the overall framework more robust to various manipulated inputs. Specifically, it introduces dual-editing consistency as the auxiliary supervision signal. To facilitate extensive studies, we construct a large-scale high-resolution face dataset with fine-grained mask annotations named CelebAMask-HQ. MaskGAN is comprehensively evaluated on two challenging tasks: attribute transfer and style copy, demonstrating superior performance over other state-of-the-art methods. The code, models, and dataset are available at this https URL.
13-3. Cascade EF-GAN: Progressive Facial Expression Editing With Local Focuses
Authors: Rongliang Wu, Gongjie Zhang, Shijian Lu, Tao Chen
Units: Nanyang Technological University, Fudan University
Recent advances in Generative Adversarial Nets (GANs) have shown remarkable improvements for facial expression editing. However, current methods are still prone to generate artifacts and blurs around expression-intensive regions, and often introduce undesired overlapping artifacts while handling large-gap expression transformations such as transformation from furious to laughing. To address these limitations, we propose Cascade Expression Focal GAN (Cascade EF-GAN), a novel network that performs progressive facial expression editing with local expression focuses. The introduction of the local focus enables the Cascade EF-GAN to better preserve identity-related features and details around eyes, noses and mouths, which further helps reduce artifacts and blurs within the generated facial images. In addition, an innovative cascade transformation strategy is designed by dividing a large facial expression transformation into multiple small ones in cascade, which helps suppress overlapping artifacts and produce more realistic editing while dealing with large-gap expression transformations. Extensive experiments over two publicly available facial expression datasets show that our proposed Cascade EF-GAN achieves superior performance for facial expression editing.
14. 暗所下の顔認識(1)
14-1. Learning Physics-Guided Face Relighting Under Directional Light
Authors: Thomas Nestmeyer, Jean-Francois Lalonde, Iain Matthews, Andreas Lehrmann
Units: MPI for Intelligent Systems, Universite Laval, Epic Games, Borealis AI
Relighting is an essential step in realistically transferring objects from a captured image into another environment. For example, authentic telepresence in Augmented Reality requires faces to be displayed and relit consistent with the observer's scene lighting. We investigate end-to-end deep learning architectures that both de-light and relight an image of a human face. Our model decomposes the input image into intrinsic components according to a diffuse physics-based image formation model. We enable non-diffuse effects including cast shadows and specular highlights by predicting a residual correction to the diffuse render. To train and evaluate our model, we collected a portrait database of 21 subjects with various expressions and poses. Each sample is captured in a controlled light stage setup with 32 individual light sources. Our method creates precise and believable relighting results and generalizes to complex illumination conditions and challenging poses, including when the subject is not looking straight at the camera.
15. Face Reenactment (2)
15-1. FReeNet: Multi-Identity Face Reenactment
Authors: Jiangning Zhang, Xianfang Zeng, Mengmeng Wang, Yusu Pan, Liang Liu, Yong Liu, Yu Ding, Changjie Fan
Units: Zhejiang University, Fuxi AI Lab, NetEase
This paper presents a novel multi-identity face reenactment framework, named FReeNet, to transfer facial expressions from an arbitrary source face to a target face with a shared model. The proposed FReeNet consists of two parts: Unified Landmark Converter (ULC) and Geometry-aware Generator (GAG). The ULC adopts an encode-decoder architecture to efficiently convert expression in a latent landmark space, which significantly narrows the gap of the face contour between source and target identities. The GAG leverages the converted landmark to reenact the photorealistic image with a reference image of the target person. Moreover, a new triplet perceptual loss is proposed to force the GAG module to learn appearance and geometry information simultaneously, which also enriches facial details of the reenacted images. Further experiments demonstrate the superiority of our approach for generating photorealistic and expression-alike faces, as well as the flexibility for transferring facial expressions between identities.
15-2. Learning Identity-Invariant Motion Representations for Cross-ID Face Reenactment
Authors: Po-Hsiang Huang, Fu-En Yang, Yu-Chiang Frank Wang
Units: Graduate Institute of Communication Engineering, National Taiwan University, ASUS Intelligent Cloud Services
Human face reenactment aims at transferring motion patterns from one face (from a source-domain video) to an-other (in the target domain with the identity of interest).While recent works report impressive results, they are notable to handle multiple identities in a unified model. In this paper, we propose a unique network of CrossID-GAN to perform multi-ID face reenactment. Given a source-domain video with extracted facial landmarks and a target-domain image, our CrossID-GAN learns the identity-invariant motion patterns via the extracted landmarks and such information to produce the videos whose ID matches that of the target domain. Both supervised and unsupervised settings are proposed to train and guide our model during training.Our qualitative/quantitative results confirm the robustness and effectiveness of our model, with ablation studies confirming our network design.
16. 顔レンダリング (1)
16-1. Rotate-and-Render: Unsupervised Photorealistic Face Rotation From Single-View Images
Authors: Hang Zhou, Jihao Liu, Ziwei Liu, Yu Liu, Xiaogang Wang
Units: The Chinese University of Hong Kong, SenseTime Research
Though face rotation has achieved rapid progress in recent years, the lack of high-quality paired training data remains a great hurdle for existing methods. The current generative models heavily rely on datasets with multi-view images of the same person. Thus, their generated results are restricted by the scale and domain of the data source. To overcome these challenges, we propose a novel unsupervised framework that can synthesize photo-realistic rotated faces using only single-view image collections in the wild. Our key insight is that rotating faces in the 3D space back and forth, and re-rendering them to the 2D plane can serve as a strong self-supervision. We leverage the recent advances in 3D face modeling and high-resolution GAN to constitute our building blocks. Since the 3D rotation-and-render on faces can be applied to arbitrary angles without losing details, our approach is extremely suitable for in-the-wild scenarios (i.e. no paired data are available), where existing methods fall short. Extensive experiments demonstrate that our approach has superior synthesis quality as well as identity preservation over the state-of-the-art methods, across a wide range of poses and domains. Furthermore, we validate that our rotate-and-render framework naturally can act as an effective data augmentation engine for boosting modern face recognition systems even on strong baseline models.
17. Face Generation (3)
17-1. One-Shot Domain Adaptation for Face Generation
Authors: Chao Yang, Ser-Nam Lim
Units: Facebook AI
In this paper, we propose a framework capable of generating face images that fall into the same distribution as that of a given one-shot example. We leverage a pre-trained StyleGAN model that already learned the generic face distribution. Given the one-shot target, we develop an iterative optimization scheme that rapidly adapts the weights of the model to shift the output's high-level distribution to the target's. To generate images of the same distribution, we introduce a style-mixing technique that transfers the low-level statistics from the target to faces randomly generated with the model. With that, we are able to generate an unlimited number of faces that inherit from the distribution of both generic human faces and the one-shot example. The newly generated faces can serve as augmented training data for other downstream tasks. Such setting is appealing as it requires labeling very few, or even one example, in the target domain, which is often the case of real-world face manipulations that result from a variety of unknown and unique distributions, each with extremely low prevalence. We show the effectiveness of our one-shot approach for detecting face manipulations and compare it with other few-shot domain adaptation methods qualitatively and quantitatively.
17-2. Exploring Unlabeled Faces for Novel Attribute Discovery
Authors: Hyojin Bahng, Sunghyo Chung, Seungjoo Yoo, Jaegul Choo
Units: Korea University, Kakao Corp.
Despite remarkable success in unpaired image-to-image translation, existing systems still require a large amount of labeled images. This is a bottleneck for their real-world applications; in practice, a model trained on labeled CelebA dataset does not work well for test images from a different distribution -- greatly limiting their application to unlabeled images of a much larger quantity. In this paper, we attempt to alleviate this necessity for labeled data in the facial image translation domain. We aim to explore the degree to which you can discover novel attributes from unlabeled faces and perform high-quality translation. To this end, we use prior knowledge about the visual world as guidance to discover novel attributes and transfer them via a novel normalization method. Experiments show that our method trained on unlabeled data produces high-quality translations, preserves identity, and be perceptually realistic as good as, or better than, state-of-the-art methods trained on labeled data.
17-3. Disentangled and Controllable Face Image Generation via 3D Imitative-Contrastive Learning
Authors: Yu Deng, Jiaolong Yang, Dong Chen, Fang Wen, Xin Tong
Units: Tsinghua University, Microsoft Research Asia
We propose an approach for face image generation of virtual people with disentangled, precisely-controllable latent representations for identity of non-existing people, expression, pose, and illumination. We embed 3D priors into adversarial learning and train the network to imitate the image formation of an analytic 3D face deformation and rendering process. To deal with the generation freedom induced by the domain gap between real and rendered faces, we further introduce contrastive learning to promote disentanglement by comparing pairs of generated images. Experiments show that through our imitative-contrastive learning, the factor variations are very well disentangled and the properties of a generated face can be precisely controlled. We also analyze the learned latent space and present several meaningful properties supporting factor disentanglement. Our method can also be used to embed real images into the disentangled latent space. We hope our method could provide new understandings of the relationship between physical properties and deep image synthesis.
18. Face Hallucination (2)
18-1. Cross-Spectral Face Hallucination via Disentangling Independent Factors
Authors: Boyan Duan, Chaoyou Fu, Yi Li, Xingguang Song, Ran He
Units: NLPR & CEBSIT & CRIPAC, CASIA, University of Chinese Academy of Sciences, Central Media Technology Institute, Huawei Technology Co., Ltd.
The cross-sensor gap is one of the challenges that have aroused much research interests in Heterogeneous Face Recognition (HFR). Although recent methods have attempted to fill the gap with deep generative networks, most of them suffer from the inevitable misalignment between different face modalities. Instead of imaging sensors, the misalignment primarily results from facial geometric variations that are independent of the spectrum. Rather than building a monolithic but complex structure, this paper proposes a Pose Aligned Cross-spectral Hallucination (PACH) approach to disentangle the independent factors and deal with them in individual stages. In the first stage, an Unsupervised Face Alignment (UFA) module is designed to align the facial shapes of the near-infrared (NIR) images with those of the visible (VIS) images in a generative way, where UV maps are effectively utilized as the shape guidance. Thus the task of the second stage becomes spectrum translation with aligned paired data. We develop a Texture Prior Synthesis (TPS) module to achieve complexion control and consequently generate more realistic VIS images than existing methods. Experiments on three challenging NIR-VIS datasets verify the effectiveness of our approach in producing visually appealing images and achieving state-of-the-art performance in HFR.
18-2. Copy and Paste GAN: Face Hallucination From Shaded Thumbnails
Authors: Yang Zhang, Ivor Tsang, Yawei Luo, Changhui Hu, Xiaobo Lu, Xin Yu
Units: School of Automation, Southeast University, Key Laboratory of Measurement and Control of Complex Systems of Engineering, Ministry of Education, Southeast University, Centre for Artificial Intelligence, University of Technology Sydney, School of Computer Science and Technology, Huazhong University of Science and Technology, School of Automation, Nanjing University of Posts and Telecommunications, Australian Centre for Robotic Vision, Australian National University
Existing face hallucination methods based on convolutional neural networks (CNN) have achieved impressive performance on low-resolution (LR) faces in a normal illumination condition. However, their performance degrades dramatically when LR faces are captured in low or non-uniform illumination conditions. This paper proposes a Copy and Paste Generative Adversarial Network (CPGAN) to recover authentic high-resolution (HR) face images while compensating for low and non-uniform illumination. To this end, we develop two key components in our CPGAN: internal and external Copy and Paste nets (CPnets). Specifically, our internal CPnet exploits facial information residing in the input image to enhance facial details; while our external CPnet leverages an external HR face for illumination compensation. A new illumination compensation loss is thus developed to capture illumination from the external guided face image effectively. Furthermore, our method offsets illumination and upsamples facial details alternately in a coarse-to-fine fashion, thus alleviating the correspondence ambiguity between LR inputs and external HR inputs. Extensive experiments demonstrate that our method manifests authentic HR face images in a uniform illumination condition and outperforms state-of-the-art methods qualitatively and quantitatively.
19. Face Morphable Model (2)
19-1. A Morphable Face Albedo Model
Authors: William A.P. Smith, Alassane Seck, Hannah Dee, Bernard Tiddeman, Joshua Tenenbaum, Bernhard Egger
Units: University of York, ARM Ltd, Aberystwyth University, MIT - BCS, CSAIL & CBMM
In this paper, we bring together two divergent strands of research: photometric face capture and statistical 3D face appearance modelling. We propose a novel lightstage capture and processing pipeline for acquiring ear-to-ear, truly intrinsic diffuse and specular albedo maps that fully factor out the effects of illumination, camera and geometry. Using this pipeline, we capture a dataset of 50 scans and combine them with the only existing publicly available albedo dataset (3DRFE) of 23 scans. This allows us to build the first morphable face albedo model. We believe this is the first statistical analysis of the variability of facial specular albedo maps. This model can be used as a plug in replacement for the texture model of the Basel Face Model (BFM) or FLAME and we make the model publicly available. We ensure careful spectral calibration such that our model is built in a linear sRGB space, suitable for inverse rendering of images taken by typical cameras. We demonstrate our model in a state of the art analysis-by-synthesis 3DMM fitting pipeline, are the first to integrate specular map estimation and outperform the BFM in albedo reconstruction.
19-2. Learning Formation of Physically-Based Face Attributes
Authors: Ruilong Li, Karl Bladin, Yajie Zhao, Chinmay Chinara, Owen Ingraham, Pengda Xiang, Xinglei Ren, Pratusha Prasad, Bipin Kishore, Jun Xing, Hao Li
Units: USC Institute for Creative Technologies, University of Southern California, Pinscreen
Based on a combined data set of 4000 high resolution facial scans, we introduce a non-linear morphable face model, capable of producing multifarious face geometry of pore-level resolution, coupled with material attributes for use in physically-based rendering. We aim to maximize the variety of face identities, while increasing the robustness of correspondence between unique components, including middle-frequency geometry, albedo maps, specular intensity maps and high-frequency displacement details. Our deep learning based generative model learns to correlate albedo and geometry, which ensures the anatomical correctness of the generated assets. We demonstrate potential use of our generative model for novel identity generation, model fitting, interpolation, animation, high fidelity data visualization, and low-to-high resolution data domain transferring. We hope the release of this generative model will encourage further cooperation between all graphics, vision, and data focused professionals while demonstrating the cumulative value of every individual's complete biometric profile.
20. 3D Facial Motion Estimation (1)
20-1. DeepFaceFlow: In-the-wild Dense 3D Facial Motion Estimation
Authors: Mohammad Rami Koujan, Anastasios Roussos, Stefanos Zafeiriou
Units: College of Engineering, Mathematics and Physical Sciences, University of Exeter, Department of Computing, Imperial College London, Institute of Computer Science, Foundation for Research and Technology-Hellas (FORTH-ICS), FaceSoft.io
Dense 3D facial motion capture from only monocular in-the-wild pairs of RGB images is a highly challenging problem with numerous applications, ranging from facial expression recognition to facial reenactment. In this work, we propose DeepFaceFlow, a robust, fast, and highly-accurate framework for the dense estimation of 3D non-rigid facial flow between pairs of monocular images. Our DeepFaceFlow framework was trained and tested on two very large-scale facial video datasets, one of them of our own collection and annotation, with the aid of occlusion-aware and 3D-based loss function. We conduct comprehensive experiments probing different aspects of our approach and demonstrating its improved performance against state-of-the-art flow and 3D reconstruction methods. Furthermore, we incorporate our framework in a full-head state-of-the-art facial video synthesis method and demonstrate the ability of our method in better representing and capturing the facial dynamics, resulting in a highly-realistic facial video synthesis. Given registered pairs of images, our framework generates 3D flow maps at ~60 fps.
21. 3D Face Dataset (1)
21-1. FaceScape: A Large-Scale High Quality 3D Face Dataset and Detailed Riggable 3D Face Prediction
Authors: Haotian Yang, Hao Zhu, Yanru Wang, Mingkai Huang, Qiu Shen, Ruigang Yang, Xun Cao
Units: Nanjing University, Baidu Research, University of Kentucky, Inceptio Inc., National Engineering Laboratory for Deep Learning Technology and Applications
In this paper, we present a large-scale detailed 3D face dataset, FaceScape, and propose a novel algorithm that is able to predict elaborate riggable 3D face models from a single image input. FaceScape dataset provides 18,760 textured 3D faces, captured from 938 subjects and each with 20 specific expressions. The 3D models contain the pore-level facial geometry that is also processed to be topologically uniformed. These fine 3D facial models can be represented as a 3D morphable model for rough shapes and displacement maps for detailed geometry. Taking advantage of the large-scale and high-accuracy dataset, a novel algorithm is further proposed to learn the expression-specific dynamic details using a deep neural network. The learned relationship serves as the foundation of our 3D face prediction system from a single image input. Different than the previous methods, our predicted 3D models are riggable with highly detailed geometry under different expressions. The unprecedented dataset and code will be released to public for research purpose.
22. Face Completion (1)
22-1. Learning Oracle Attention for High-Fidelity Face Completion
Authors: Tong Zhou, Changxing Ding, Shaowen Lin, Xinchao Wang, Dacheng Tao
Units: South China University of Technology, Stevens Institute of Technology, UBTECH Sydney AI Centre, School of Computer Science, Faculty of Engineering, The University of Sydney
High-fidelity face completion is a challenging task due to the rich and subtle facial textures involved. What makes it more complicated is the correlations between different facial components, for example, the symmetry in texture and structure between both eyes. While recent works adopted the attention mechanism to learn the contextual relations among elements of the face, they have largely overlooked the disastrous impacts of inaccurate attention scores; in addition, they fail to pay sufficient attention to key facial components, the completion results of which largely determine the authenticity of a face image. Accordingly, in this paper, we design a comprehensive framework for face completion based on the U-Net structure. Specifically, we propose a dual spatial attention module to efficiently learn the correlations between facial textures at multiple scales; moreover, we provide an oracle supervision signal to the attention module to ensure that the obtained attention scores are reasonable. Furthermore, we take the location of the facial components as prior knowledge and impose a multi-discriminator on these regions, with which the fidelity of facial components is significantly promoted. Extensive experiments on two high-resolution face datasets including CelebA-HQ and Flickr-Faces-HQ demonstrate that the proposed approach outperforms state-of-the-art methods by large margins.
23. Other (2)
23-1. Can Facial Pose and Expression Be Separated With Weak Perspective Camera?
Authors: Evangelos Sariyanidi, Casey J. Zampella, Robert T. Schultz, Birkan Tunc
Units: Center for Autism Research, Children’s Hospital of Philadelphia, University of Pennsylvania
Separating facial pose and expression within images requires a camera model for 3D-to-2D mapping. The weak perspective (WP) camera has been the most popular choice; it is the default, if not the only option, in state-of-the-art facial analysis methods and software. WP camera is justified by the supposition that its errors are negligible when the subjects are relatively far from the camera, yet this claim has never been tested despite nearly 20 years of research. This paper critically examines the suitability of WP camera for separating facial pose and expression. First, we theoretically show that WP causes pose-expression ambiguity, as it leads to estimation of spurious expressions. Next, we experimentally quantify the magnitude of spurious expressions. Finally, we test whether spurious expressions have detrimental effects on a common facial analysis application, namely Action Unit (AU) detection. Contrary to conventional wisdom, we find that severe pose-expression ambiguity exists even when subjects are not close to the camera, leading to large false positive rates in AU detection. We also demonstrate that the magnitude and characteristics of spurious expressions depend on the point distribution model used to model the expressions. Our results suggest that common assumptions about WP need to be revisited in facial expression modeling, and that facial analysis software should encourage and facilitate the use of the true camera model whenever possible.
23-2. Cross-Modal Deep Face Normals With Deactivable Skip Connections
Authors: Victoria Fernandez Abrevaya, Adnane Boukhayma, Philip H. S. Torr, Edmond Boyer
Units: Inria, Univ. Grenoble Alpes, CNRS, Grenoble INP, LJK, Inria, Univ. Rennes, CNRS, IRISA, M2S, University of Oxford
We present an approach for estimating surface normals from in-the-wild color images of faces. While data-driven strategies have been proposed for single face images, limited available ground truth data makes this problem difficult. To alleviate this issue, we propose a method that can leverage all available image and normal data, whether paired or not, thanks to a novel cross-modal learning architecture. In particular, we enable additional training with single modality data, either color or normal, by using two encoder-decoder networks with a shared latent space. The proposed architecture also enables face details to be transferred between the image and normal domains, given paired data, through skip connections between the image encoder and normal decoder. Core to our approach is a novel module that we call deactivable skip connections, which allows integrating both the auto-encoded and image-to-normal branches within the same architecture that can be trained end-to-end. This allows learning of a rich latent space that can accurately capture the normal information. We compare against state-of-the-art methods and show that our approach can achieve significant improvements, both quantitative and qualitative, with natural face images.
この記事が気に入ったらサポートをしてみませんか?