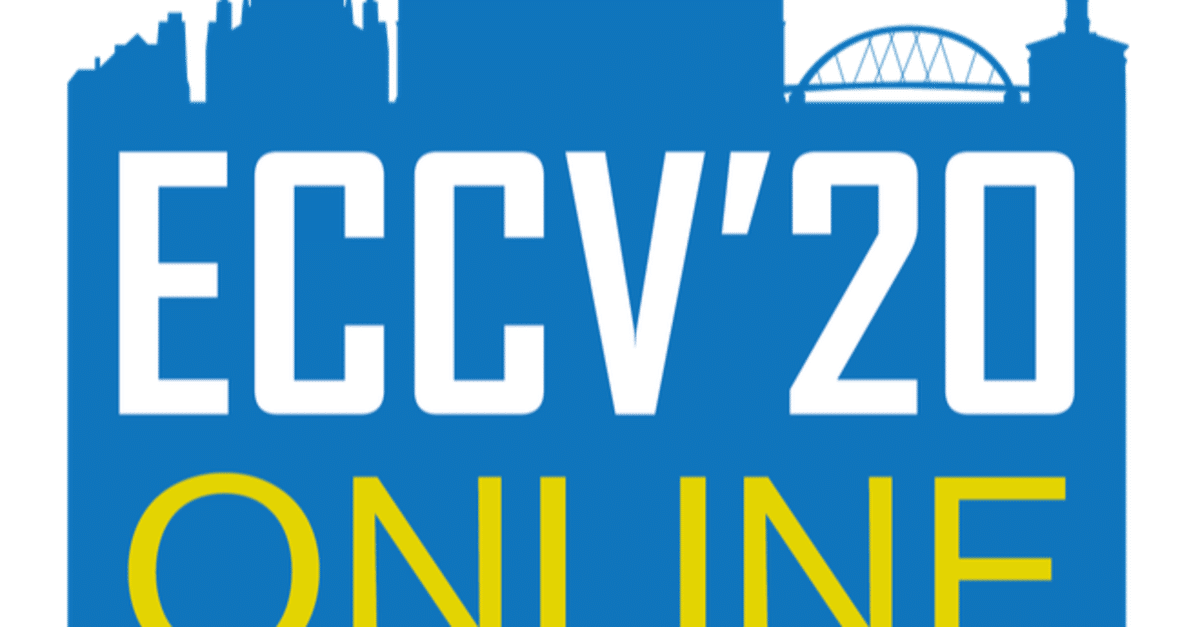
ECCV2020 - 顔関連の論文リスト
ECCV2020(European Conference on Computer Vision 2020)は画像解析分野におけるヨーロッパのトップカンファレンスです。
この記事では、このカンファレンスで発表された顔関連の論文をリストアップしています。
また、一部の論文はAI-SCHOLARで紹介記事を投稿していますので、そのリンクも合わせて掲載しています。ぜひご覧ください。
1. 顔の位置合わせ(2)
1-1. “Look Ma, no landmarks!” – Unsupervised, model-based dense face alignment
Authors: Tatsuro Koizumi, William A. P. Smith
Units: Canon Inc., University of York
Abstract:
In this paper, we show how to train an image-to-image network to predict dense correspondence between a face image and a 3D morphable model using only the model for supervision. We show that both geometric parameters (shape, pose and camera intrinsics) and photometric parameters (texture and lighting) can be inferred directly from the correspondence map using linear least squares and our novel inverse spherical harmonic lighting model. The least squares residuals provide an unsupervised training signal that allows us to avoid artefacts common in the literature such as shrinking and conservative underfitting. Our approach uses a network that is 10x smaller than parameter regression networks, significantly reduces sensitivity to image alignment and allows known camera calibration or multi-image constraints to be incorporated during inference. We achieve results competitive with state-of-the-art but without any auxiliary supervision used by previous methods.
1-2. Towards Fast, Accurate and Stable 3D Dense Face Alignment
Authors: Jianzhu Guo, Xiangyu Zhu, Yang Yang, Fan Yang, Zhen Lei, Stan Z. Li
Units: CBSR&NLPR, Institute of Automation, Chinese Academy of Sciences, School of Artificial Intelligence, University of Chinese Academy of Sciences, College of Software, Beihang University, School of Engineering, Westlake University
Abstract:
Existing methods of 3D dense face alignment mainly concentrate on accuracy, thus limiting the scope of their practical applications.
In this paper, we propose a novel regression framework which makes
a balance among speed, accuracy and stability. Firstly, on the basis of
a lightweight backbone, we propose a meta-joint optimization strategy
to dynamically regress a small set of 3DMM parameters, which greatly
enhances speed and accuracy simultaneously. To further improve the stability on videos, we present a virtual synthesis method to transform one
still image to a short-video which incorporates in-plane and out-of-plane
face moving. On the premise of high accuracy and stability, our model
runs at over 50fps on a single CPU core and outperforms other state-ofthe-art heavy models simultaneously. Experiments on several challenging
datasets validate the efficiency of our method. The code and models will
be available at https://github.com/cleardusk/3DDFA_V2.
2. 顔の年齢分析(2)
2-1. Hierarchical Face Aging through Disentangled Latent Characteristics
Authors: Peipei Li, Huaibo Huang, Yibo Hu, Xiang Wu, Ran He, Zhenan Sun
Units: Center for Research on Intelligent Perception and Computing, NLPR, CASIA, Center for Excellence in Brain Science and Intelligence Technology, CAS, School of Artificial Intelligence, University of Chinese Academy of Sciences, Artificial Intelligence Research, CAS, Jiaozhou, Qingdao, China
Abstract:
Current age datasets lie in a long-tailed distribution, which
brings difficulties to describe the aging mechanism for the imbalance
ages. To alleviate it, we design a novel facial age prior to guide the aging mechanism modeling. To explore the age effects on facial images, we
propose a Disentangled Adversarial Autoencoder (DAAE) to disentangle the facial images into three independent factors: age, identity and
extraneous information. To avoid the “wash away” of age and identity
information in face aging process, we propose a hierarchical conditional generator by passing the disentangled identity and age embeddings
to the high-level and low-level layers with class-conditional BatchNorm.
Finally, a disentangled adversarial learning mechanism is introduced to
boost the image quality for face aging. In this way, when manipulating
the age distribution, DAAE can achieve face aging with arbitrary ages.
Further, given an input face image, the mean value of the learned age
posterior distribution can be treated as an age estimator. These indicate
that DAAE can efficiently and accurately estimate the age distribution in
a disentangling manner. DAAE is the first attempt to achieve facial age
analysis tasks, including face aging with arbitrary ages, exemplar-based
face aging and age estimation, in a universal framework. The qualitative
and quantitative experiments demonstrate the superiority of DAAE on
five popular datasets, including CACD2000, Morph, UTKFace, FG-NET
and AgeDB.
2-2. Adaptive Variance Based Label Distribution Learning For Facial Age Estimation
Authors: Xin Wen, Biying Li, Haiyun Guo, Zhiwei Liu, Guosheng Hu, Ming Tang, Jinqiao Wang
Units: National Laboratory of Pattern Recognition, Institute of Automation,
Chinese Academy of Sciences, School of Artificial Intelligence, University of Chinese Academy of Sciences, ObjectEye Inc., NEXWISE Co., Ltd, Anyvision
Abstract:
Estimating age from a single facial image is a classic and
challenging topic in computer vision. One of its most intractable issues
is label ambiguity, i.e., face images from adjacent age of the same person
are often indistinguishable. Some existing methods adopt distribution
learning to tackle this issue by exploiting the semantic correlation between age labels. Actually, most of them set a fixed value to the variance
of Gaussian label distribution for all the images. However, the variance is
closely related to the correlation between adjacent ages and should vary
across ages and identities. To model a sample-specific variance, in this paper, we propose an adaptive variance based distribution learning (AVDL)
method for facial age estimation. AVDL introduces the data-driven optimization framework, meta-learning, to achieve this. Specifically, AVDL
performs a meta gradient descent step on the variable (i.e. variance)
to minimize the loss on a clean unbiased validation set. By adaptively
learning proper variance for each sample, our method can approximate
the true age probability distribution more effectively. Extensive experiments on FG-NET and MORPH II datasets show the superiority of our
proposed approach to the existing state-of-the-art methods.
3. 顔認識(12)
3-1. Semi-Siamese Training for Shallow Face Learning
Authors: Hang Du, Hailin Shi, Yuchi Liu, Jun Wang, Zhen Lei, Dan Zeng, Tao Mei
Units: Shanghai University, JD AI Research, NLPR, Institute of Automation, Chinese Academy of Sciences
Abstract:
Most existing public face datasets, such as MS-Celeb-1M
and VGGFace2, provide abundant information in both breadth (large
number of IDs) and depth (sufficient number of samples) for training.
However, in many real-world scenarios of face recognition, the training
dataset is limited in depth, i.e. only two face images are available for each
ID. We define this situation as Shallow Face Learning, and find it problematic with existing training methods. Unlike deep face data, the shallow face data lacks intra-class diversity. As such, it can lead to collapse of feature dimension and consequently the learned network can easily suffer from degeneration and over-fitting in the collapsed dimension. In this
paper, we aim to address the problem by introducing a novel training
method named Semi-Siamese Training (SST). A pair of Semi-Siamese
networks constitute the forward propagation structure, and the training
loss is computed with an updating gallery queue, conducting effective
optimization on shallow training data. Our method is developed without
extra-dependency, thus can be flexibly integrated with the existing loss
functions and network architectures. Extensive experiments on various
benchmarks of face recognition show the proposed method significantly
improves the training, not only in shallow face learning, but also for
conventional deep face data.
3-2. BroadFace: Looking at Tens of Thousands of People at Once for Face Recognition
Authors: Yonghyun Kim, Wonpyo Park, Jongju Shin
Units: Kakao Enterprise, Kakao Corp.
Abstract:
The datasets of face recognition contain an enormous number of identities and instances. However, conventional methods have difficulty in reflecting the entire distribution of the datasets because a minibatch of small size contains only a small portion of all identities. To overcome this difficulty, we propose a novel method called BroadFace, which is a learning process to consider a massive set of identities, comprehensively. In BroadFace, a linear classifier learns optimal decision boundaries among identities from a large number of embedding vectors accumulated over past iterations. By referring more instances at once, the optimality of the classifier is naturally increased on the entire datasets. Thus, the encoder is also globally optimized by referring the weight matrix of the classifier. Moreover, we propose a novel compensation method to increase the number of referenced instances in the training stage. BroadFace can be easily applied on many existing methods to accelerate a learning process and obtain a significant improvement in accuracy without extra computational burden at inference stage. We perform extensive ablation studies and experiments on various datasets to show the effectiveness of BroadFace, and also empirically prove the validity of our compensation method. BroadFace achieves the state-of-the-art results with significant improvements on nine datasets in 1:1 face verification and 1:N face identification tasks, and is also effective in image retrieval.
こちらの論文は、AI-SCHOLARで論文紹介の記事を投稿しています。是非ご覧ください。
3-3. Sub-center ArcFace: Boosting Face Recognition by Large-scale Noisy Web Faces
Authors: Jiankang Deng, Jia Guo, Tongliang Liu, Mingming Gong, Stefanos Zafeiriou
Units: Imperial College, InsightFace, University of Sydney, 4University of Melbourne
Abstract:
Margin-based deep face recognition methods (e.g. SphereFace,
CosFace, and ArcFace) have achieved remarkable success in unconstrained face recognition. However, these methods are susceptible to the massive label noise in the training data and thus require laborious human effort to clean the datasets. In this paper, we relax the intra-class constraint of ArcFace to improve the robustness to label noise. More specifically, we design K sub-centers for each class and the training sample only needs to be close to any of the K positive sub-centers instead of the
only one positive center. The proposed sub-center ArcFace encourages
one dominant sub-class that contains the majority of clean faces and
non-dominant sub-classes that include hard or noisy faces. Extensive
experiments confirm the robustness of sub-center ArcFace under massive real-world noise. After the model achieves enough discriminative power, we directly drop non-dominant sub-centers and high-confident noisy samples, which helps recapture intra-compactness, decrease the influence from noise, and achieve comparable performance compared to ArcFace trained on the manually cleaned dataset. By taking advantage of the large-scale raw web faces (Celeb500K), sub-center Arcface achieves state-of-the-art performance on IJB-B, IJB-C, MegaFace, and FRVT.
Authors: Arslan Ali, Matteo Testa, Tiziano Bianchi, Enrico Magli
Units: Department of Electronics and Telecommunications
Abstract:
We present BioMetricNet: a novel framework for deep unconstrained face verification which learns a regularized metric to compare facial features. Differently from popular methods such as FaceNet, the proposed approach does not impose any specific metric on facial features; instead, it shapes the decision space by learning a latent representation in which matching and non-matching pairs are mapped onto clearly separated and well-behaved target distributions. In particular, the network jointly learns the best feature representation, and the best metric that follows the target distributions, to be used to discriminate face images. In this paper we present this general framework, first of its kind for facial verification, and tailor it to Gaussian distributions. This choice enables the use of a simple linear decision boundary that can be tuned to achieve the desired trade-off between false alarm and genuine acceptance rate, and leads to a loss function that can be written in closed form. Extensive analysis and experimentation on publicly available datasets such as Labeled Faces in the wild (LFW), Youtube faces (YTF), Celebrities in Frontal-Profile in the Wild (CFP), and challenging datasets like cross-age LFW (CALFW), cross-pose LFW (CPLFW), In-the-wild Age Dataset (AgeDB) show a significant performance improvement and confirms the effectiveness and superiority of BioMetricNet over existing state-of-theart methods.
こちらの論文は、AI-SCHOLARで論文紹介の記事を投稿しています。是非ご覧ください。
3-5. Generate to Adapt: Resolution Adaption Network for Surveillance Face Recognition
Authors: Han Fang, Weihong Deng, Yaoyao Zhong, and Jiani Hu
Units: Beijing University of Posts and Telecommunications
Abstract:
Although deep learning techniques have largely improved
face recognition, unconstrained surveillance face recognition is still an
unsolved challenge, due to the limited training data and the gap of domain distribution. Previous methods mostly match low-resolution and
high-resolution faces in different domains, which tend to deteriorate the
original feature space in the common recognition scenarios. To avoid this
problem, we propose resolution adaption network (RAN) which contains
Multi-Resolution Generative Adversarial Networks (MR-GAN) followed
by a feature adaption network. MR-GAN learns multi-resolution representations and randomly selects one resolution to generate realistic
low-resolution (LR) faces that can avoid the artifacts of down-sampled
faces. A novel feature adaption network with translation gate is developed to fuse the discriminative information of LR faces into backbone
network, while preserving the discrimination ability of original face representations. The experimental results on IJB-C TinyFace, SCface, QMULSurvFace datasets have demonstrated the superiority of our method compared with state-of-the-art surveillance face recognition methods, while showing stable performance on the common recognition scenarios.
3-6. Caption-Supervised Face Recognition: Training a State-of-the-Art Face Model without Manual Annotation
Authors: Qingqiu Huang, Lei Yang, Huaiyi Huang, Tong Wu, Dahua Lin
Units: The Chinese University of Hong Kong, Tsinghua Univerisity
Abstract:
The advances over the past several years have pushed the performance of face recognition to an amazing level. This great success, to a large extent, is built on top of millions of annotated samples. However, as we endeavor to take the performance to the next level, the reliance on annotated data becomes a major obstacle. We desire to explore an alternative approach, namely using captioned images for training, as an attempt to mitigate this difficulty. Captioned images are widely available on the web, while the captions often contain the names of the subjects in the images. Hence, an effective method to leverage such data would significantly reduce the need of human annotations. However, an important challenge along this way needs to be tackled: the names in the captions are often noisy and ambiguous, especially when there are multiple names in the captions or multiple people in the photos. In this work, we propose a simple yet effective method, which trains a face recognition model by progressively expanding the labeled set via both selective propagation and caption-driven expansion. We build a large-scale dataset of captioned images, which contain 6.3M faces from 305K subjects. Our experiments show that using the proposed method, we can train a state-of-the-art face recognition model without manual annotation (99.65% in LFW). This shows the great potential of caption-supervised face recognition.
こちらの論文は、AI-SCHOLARで論文紹介の記事を投稿しています。是非ご覧ください。
3-7. Improving Face Recognition by Clustering Unlabeled Faces in the Wild
Authors: Aruni RoyChowdhury, Xiang Yu, Kihyuk Sohn, Erik Learned-Miller, Manmohan Chandraker
Units: University of Massachusetts Amherst, NEC Labs America
Abstract:
While deep face recognition has benefited significantly from large-scale labeled data, current research is focused on leveraging unlabeled data to further boost performance, reducing the cost of human annotation. Prior work has mostly been in controlled settings, where the labeled and unlabeled data sets have no overlapping identities by construction. This is not realistic in large-scale face recognition, where one must contend with such overlaps, the frequency of which increases with the volume of data. Ignoring identity overlap leads to significant labeling noise, as data from the same identity is split into multiple clusters. To address this, we propose a novel identity separation method based on extreme value theory. It is formulated as an out-of-distribution detection algorithm, and greatly reduces the problems caused by overlapping-identity label noise. Considering cluster assignments as pseudo-labels, we must also overcome the labeling noise from clustering errors. We propose a modulation of the cosine loss, where the modulation weights correspond to an estimate of clustering uncertainty. Extensive experiments on both controlled and real settings demonstrate our method's consistent improvements over supervised baselines, e.g., 11.6% improvement on IJB-A verification.
3-8. Exclusivity-Consistency Regularized Knowledge Distillation for Face Recognition
Authors: Xiaobo Wang, Tianyu Fu, Shengcai Liao, Shuo Wang, Zhen Lei, Tao Mei
Units: JD AI Research, Inception Institute of Artificial Intelligence (IIAI), CBSR&NLPR, Institute of Automation, Chinese Academy of Science, School of Artificial Intelligence, University of Chinese Academy of Science
Abstract:
Knowledge distillation is an effective tool to compress large
pre-trained Convolutional Neural Networks (CNNs) or their ensembles
into models applicable to mobile and embedded devices. The success
of which mainly comes from two aspects: the designed student network
and the exploited knowledge. However, current methods usually suffer
from the low-capability of mobile-level student network and the unsatisfactory knowledge for distillation. In this paper, we propose a novel
position-aware exclusivity to encourage large diversity among different
filters of the same layer to alleviate the low-capability of student network. Moreover, we investigate the effect of several prevailing knowledge
for face recognition distillation and conclude that the knowledge of feature consistency is more flexible and preserves much more information
than others. Experiments on a variety of face recognition benchmarks
have revealed the superiority of our method over the state-of-the-arts.
3-9. Explainable Face Recognition
Authors: Jonathan R. Williford, Brandon B. May, Jeffrey Byrne
Units: Systems & Technology Research
Abstract:
Explainable face recognition (XFR) is the problem of explaining the matches returned by a facial matcher, in order to provide insight into why a probe was matched with one identity over another. In this paper, we provide the first comprehensive benchmark and baseline evaluation for XFR. We define a new evaluation protocol called the “inpainting game”, which is a curated set of 3648 triplets (probe, mate, nonmate) of 95 subjects, which differ by synthetically inpainting a chosen facial characteristic like the nose, eyebrows or mouth creating an inpainted nonmate. An XFR algorithm is tasked with generating a network attention map which best explains which regions in a probe image match with a mated image, and not with an inpainted nonmate for each triplet. This provides ground truth for quantifying what image regions contribute to face matching. Finally, we provide a comprehensive benchmark on this dataset comparing five state-of-the-art XFR algorithms on three facial matchers. This benchmark includes two new algorithms called subtree EBP and Density-based Input Sampling for Explanation (DISE) which outperform the state-of-the-art XFR by a wide margin.
こちらの論文は、AI-SCHOLARで論文紹介の記事を投稿しています。是非ご覧ください。
3-10. Manifold Projection for Adversarial Defense on Face Recognition
Authors: Jianli Zhou, Chao Liang, Jun Chen
Units: National Engineering Research Center for Multimedia Software, School of Computer Science, Key Laboratory of Multimedia and Network Communication Engineering
Abstract:
Although deep convolutional neural network based face recognition system has achieved remarkable success, it is susceptible to adversarial images: carefully constructed imperceptible perturbations can easily mislead deep neural networks. A recent study has shown that in addition to regular off-manifold adversarial images, there are also adversarial images on the manifold. In this paper, we propose Adversarial Variational AutoEncoder (A-VAE), a novel framework to tackle both types of attacks. We hypothesize that both off-manifold and on-manifold attacks move the image away from the high probability region of image manifold. We utilize variational autoencoder (VAE) to estimate the lower bound of the log-likelihood of image and explore to project the input images back into the high probability regions of image manifold again. At inference time, our model synthesizes multiple similar realizations of a given image by random sampling, then the nearest neighbor of the given image is selected as the final input of the face recognition model. As a preprocessing operation, our method is attack-agnostic and can adapt to a wide range of resolutions. The experimental results on LFW demonstrate that our method achieves state-of-the-art defense success rate against conventional off-manifold attacks such as FGSM, PGD, and C&W under both grey-box and white-box settings, and even on-manifold attack.
3-11. Jointly De-biasing Face Recognition and Demographic Attribute Estimation
Authors: Sixue Gong, Xiaoming Liu, Anil K. Jain
Units: Michigan State University
Abstract:
We address the problem of bias in automated face recognition and demographic attribute estimation algorithms, where errors are lower on certain cohorts belonging to specific demographic groups. We present a novel de-biasing adversarial network (DebFace) that learns to extract disentangled feature representations for both unbiased face recognition and demographics estimation. The proposed network consists of one identity classifier and three demographic classifiers (for gender, age, and race) that are trained to distinguish identity and demographic attributes, respectively. Adversarial learning is adopted to minimize correlation among feature factors so as to abate bias influence from other factors. We also design a new scheme to combine demographics with identity features to strengthen robustness of face representation in different demographic groups. The experimental results show that our approach is able to reduce bias in face recognition as well as demographics estimation while achieving state-of-the-art performance.
3-12. Improving Face Recognition from Hard Samples via Distribution Distillation Loss
Authors: Yuge Huang, Pengcheng Shen, Ying Tai, Shaoxin Li, Xiaoming Liu, Jilin Li, Feiyue Huang, Rongrong Ji
Units: Youtu Lab, Tencent, Michigan State University, Xiamen University
Abstract:
Large facial variations are the main challenge in face recognition. To this end, previous variation-specific methods make full use of task-related prior to design special network losses, which are typically not general among different tasks and scenarios. In contrast, the existing generic methods focus on improving the feature discriminability to minimize the intra-class distance while maximizing the inter-class distance, which perform well on easy samples but fail on hard samples. To improve the performance on hard samples, we propose a novel Distribution Distillation Loss to narrow the performance gap between easy and hard samples, which is simple, effective and generic for various types of facial variations. Specifically, we first adopt state-of-the-art classifiers such as Arcface to construct two similarity distributions: a teacher distribution from easy samples and a student distribution from hard samples. Then, we propose a novel distribution-driven loss to constrain the student distribution to approximate the teacher distribution, which thus leads to smaller overlap between the positive and negative pairs in the student distribution. We have conducted extensive experiments on both generic large-scale face benchmarks and benchmarks with diverse variations on race, resolution and pose. The quantitative results demonstrate the superiority of our method over strong baselines, e.g., Arcface and Cosface.
4. 3Dマスクモデリング(7)
4-1. Beyond 3DMM Space: Towards Fine-grained 3D Face Reconstruction
Authors: Xiangyu Zhu, Fan Yang, Di Huang, Chang Yu, Hao Wang, Jianzhu Guo, Zhen Lei, Stan Z. Li
Units: CBSR & NLPR, Institute of Automation, Chinese Academy of Sciences, School of Artificial Intelligence, University of Chinese Academy of Sciences, College of Software, Beihang University, Beijing Advanced Innovation Center for BDBC, Beihang University, School of Engineering, Westlake University
Abstract:
Recently, deep learning based 3D face reconstruction methods have shown promising results in both quality and efficiency. However, most of their training data is constructed by 3D Morphable Model, whose space spanned is only a small part of the shape space. As a result, the reconstruction results lose the fine-grained geometry and look different from real faces. To alleviate this issue, we first propose a solution to construct large-scale fine-grained 3D data from RGB-D images, which are expected to be massively collected as the proceeding of hand-held depth camera. A new dataset Fine-Grained 3D face (FG3D) with 200k samples is constructed to provide sufficient data for neural network training. Secondly, we propose a Fine-Grained reconstruction Network (FGNet) that can concentrate on shape modification by warping the network input and output to the UV space. Through FG3D and FGNet, we successfully generate reconstruction results with fine-grained geometry. The experiments on several benchmarks validate the effectiveness of our method compared to several baselines and other state-of-the-art methods. The proposed method and code will be available at https://github.com/XiangyuZhu-open/Beyond3DMM.
4-2. Inequality-Constrained and Robust 3D Face Model Fitting
Authors: Evangelos Sariyanidi, Casey J. Zampella, Robert T. Schultz, Birkan Tunc
Units: Center for Autism Research, Children’s Hospital of Philadelphia, University of Pennsylvania
Abstract:
Fitting 3D morphable models (3DMMs) on faces is a wellstudied problem, motivated by various industrial and research applications. 3DMMs express a 3D facial shape as a linear sum of basis functions. The resulting shape, however, is a plausible face only when the basis coefficients take values within limited intervals. Methods based on unconstrained optimization address this issue with a weighted L2 penalty on coefficients; however, determining the weight of this penalty is difficult, and the existence of a single weight that works universally is questionable. We propose a new formulation that does not require the tuning of any weight parameter. Specifically, we formulate 3DMM fitting as an inequality-constrained optimization problem, where the primary constraint is that basis coefficients should not exceed the interval that is learned when the 3DMM is constructed. We employ additional constraints to exploit sparse landmark detectors, by forcing the facial shape to be within the error bounds of a reliable detector. To enable operation “in-the-wild”, we use a robust objective function, namely Gradient Correlation. Our approach performs comparably with deep learning (DL) methods on “in-the-wild” data that have inexact ground truth, and better than DL methods on more controlled data with exact ground truth. Since our formulation does not require any learning, it enjoys a versatility that allows it to operate with multiple frames of arbitrary sizes. This study’s results encourage further research on 3DMM fitting with inequality-constrained optimization methods, which have been unexplored compared to unconstrained methods.
4-3. JNR: Joint-based Neural Rig Representation for Compact 3D Face Modeling
Authors: Noranart Vesdapunt, Mitch Rundle, HsiangTao Wu, Baoyuan Wang
Units: Microsoft Cloud and AI
Abstract:
In this paper, we introduce a novel approach to learn a 3D face model using a joint-based face rig and a neural skinning network. Thanks to the joint-based representation, our model enjoys some significant advantages over prior blendshape-based models. First, it is very compact such that we are orders of magnitude smaller while still keeping strong modeling capacity. Second, because each joint has its semantic meaning, interactive facial geometry editing is made easier and more intuitive. Third, through skinning, our model supports adding mouth interior and eyes, as well as accessories (hair, eye glasses, etc.) in a simpler, more accurate and principled way. We argue that because the human face is highly structured and topologically consistent, it does not need to be learned entirely from data. Instead we can leverage prior knowledge in the form of a human-designed 3D face rig to reduce the data dependency, and learn a compact yet strong face model from only a small dataset (less than one hundred 3D scans). To further improve the modeling capacity, we train a skinning weight generator through adversarial learning. Experiments on fitting high-quality 3D scans (both neutral and expressive), noisy depth images, and RGB images demonstrate that its modeling capacity is on-par with state-of-the-art face models, such as FLAME and Facewarehouse, even though the model is 10 to 20 times smaller. This suggests broad value in both graphics and vision applications on mobile and edge devices.
4-4. Personalized Face Modeling for Improved Face Reconstruction and Motion Retargeting
Authors: Bindita Chaudhuri, Noranart Vesdapunt, Linda Shapiro, Baoyuan Wang
Units: University of Washington, Microsoft Cloud and AI
Abstract:
Traditional methods for image-based 3D face reconstruction and facial motion retargeting fit a 3D morphable model (3DMM) to the face, which has limited modeling capacity and fail to generalize well to in-the-wild data. Use of deformation transfer or multilinear tensor as a personalized 3DMM for blendshape interpolation does not address the fact that facial expressions result in different local and global skin deformations in different persons. Moreover, existing methods learn a single albedo per user which is not enough to capture the expression-specific skin reflectance variations. We propose an end-to-end framework that jointly learns a personalized face model per user and per-frame facial motion parameters from a large corpus of in-the-wild videos of user expressions. Specifically, we learn user-specific expression blendshapes and dynamic (expression-specific) albedo maps by predicting personalized corrections on top of a 3DMM prior. We introduce novel constraints to ensure that the corrected blendshapes retain their semantic meanings and the reconstructed geometry is disentangled from the albedo. Experimental results show that our personalization accurately captures fine-grained facial dynamics in a wide range of conditions and efficiently decouples the learned face model from facial motion, resulting in more accurate face reconstruction and facial motion retargeting compared to state-of-the-art methods.
4-5. Self-Supervised Monocular 3D Face Reconstruction by Occlusion-Aware Multi-view Geometry Consistency
Authors: Jiaxiang Shang, Tianwei Shen, Shiwei Li, Lei Zhou, Mingmin Zhen, Tian Fang, Long Quan
Units: Hong Kong University of Science and Technology, Everest Innovation Technology
Abstract:
Recent learning-based approaches, in which models are trained
by single-view images have shown promising results for monocular 3D
face reconstruction, but they suffer from the ill-posed face pose and
depth ambiguity issue. In contrast to previous works that only enforce
2D feature constraints, we propose a self-supervised training architecture by leveraging the multi-view geometry consistency, which provides
reliable constraints on face pose and depth estimation. We first propose
an occlusion-aware view synthesis method to apply multi-view geometry
consistency to self-supervised learning. Then we design three novel loss
functions for multi-view consistency, including the pixel consistency loss,
the depth consistency loss, and the facial landmark-based epipolar loss.
Our method is accurate and robust, especially under large variations of
expressions, poses, and illumination conditions. Comprehensive experiments on the face alignment and 3D face reconstruction benchmarks
have demonstrated superiority over state-of-the-art methods. Our code
and model are released in https://github.com/jiaxiangshang/MGCNet.
4-6. Eyeglasses 3D shape reconstruction from a single face image
Authors: Yating Wang, Quan Wang, Feng Xu
Units: BNRist and school of software, Tsinghua University, SenseTime Group Limited
Abstract:
A complete 3D face reconstruction requires to explicitly model the eyeglasses on the face, which is less investigated in the literature. In this paper, we present an automatic system that recovers the 3D shape of eyeglasses from a single face image with an arbitrary head pose. To achieve this goal, we first trains a neural network to jointly perform glasses landmark detection and segmentation, which carry the sparse and dense glasses shape information respectively for 3D glasses pose estimation and shape recovery. To solve the ambiguity in 2D to 3D reconstruction, our system fully explores the prior knowledge including the relative motion constraint between face and glasses and the planar and symmetric shape prior feature of glasses. From the qualitative and quantitative experiments, we see that our system reconstructs promising 3D shapes of eyeglasses for various poses.
4-7. Synthesizing Coupled 3D Face Modalities by Trunk-Branch Generative Adversarial Networks
Authors: Baris Gecer, Alexander Lattas, Stylianos Ploumpis, Jiankang Deng, Athanasios Papaioannou, Stylianos Moschoglou, Stefanos Zafeiriou
Units: Imperial College, FaceSoft.io
Abstract:
Generating realistic 3D faces is of high importance for computer graphics and computer vision applications. Generally, research on 3D face generation revolves around linear statistical models of the facial surface. Nevertheless, these models cannot represent faithfully either the facial texture or the normals of the face, which are very crucial for photorealistic face synthesis. Recently, it was demonstrated that Generative Adversarial Networks (GANs) can be used for generating high-quality textures of faces. Nevertheless, the generation process either omits the geometry and normals, or independent processes are used to produce 3D shape information. In this paper, we present the first methodology that generates high-quality texture, shape, and normals jointly, which can be used for photo-realistic synthesis. To do so, we propose a novel GAN that can generate data from different modalities while exploiting their correlations. Furthermore, we demonstrate how we can condition the generation on the expression and create faces with various facial expressions. The qualitative results shown in this paper are compressed due to size limitations, full-resolution results and the accompanying video can be found in the supplementary documents. The code and models are available at the project page: https://github.com/barisgecer/TBGAN.
5. 顔検出(2)
5-1. ProgressFace: Scale-Aware Progressive Learning for Face Detection
Authors: Jiashu Zhu, Dong Li, Tiantian Han, Lu Tian, Yi Shan
Units: Xilinx Inc.
Abstract:
Scale variation stands out as one of key challenges in face detection. Recent attempts have been made to cope with this issue by incorporating image / feature pyramids or adjusting anchor sampling / matching strategies. In this work, we propose a novel scale-aware progressive training mechanism to address large scale variations across faces. Inspired by curriculum learning, our method gradually learns large-to-small face instances. The preceding models learned with easier samples (i.e., large faces) can provide good initialization for succeeding learning with harder samples (i.e., small faces), ultimately deriving a better optimum of face detectors. Moreover, we propose an auxiliary anchor-free enhancement module to facilitate the learning of small faces by supplying positive anchors that may be not covered according to the criterion of IoU overlap. Such anchor-free module will be removed during inference and hence
no extra computation cost is introduced. Extensive experimental results
demonstrate the superiority of our method compared to the state-of-thearts on the standard FDDB and WIDER FACE benchmarks. Especially,
our ProgressFace-Light with MobileNet-0.25 backbone achieves 87.9%
AP on the hard set of WIDER FACE, surpassing largely RetinaFace with
the same backbone by 9.7%. Code and our trained face detection models
are available at https://github.com/jiashu-zhu/ProgressFace.
5-2. Design and Interpretation of Universal Adversarial Patches in Face Detection
Authors: Xiao Yang, Fangyun Wei, Hongyang Zhang, Jun Zhu
Units: Dept. of Comp. Sci. & Tech., BNRist Center, Institute for AI, Tsinghua University, Microsoft Research Asia, TTIC
Abstract:
We consider universal adversarial patches for faces — small visual elements whose addition to a face image reliably destroys the performance of face detectors. Unlike previous work that mostly focused on the algorithmic design of adversarial examples in terms of improving the success rate as an attacker, in this work we show an interpretation of such patches that can prevent the state-of-the-art face detectors from detecting the real faces. We investigate a phenomenon: patches designed to suppress real face detection appear face-like. This phenomenon holds generally across different initialization, locations, scales of patches, backbones and face detection frameworks. We propose new optimizationbased approaches to automatic design of universal adversarial patches for varying goals of the attack, including scenarios in which true positives are suppressed without introducing false positives. Our proposed algorithms perform well on real-world datasets, deceiving state-of-the-art face detectors in terms of multiple precision/recall metrics and transferability.
6. 高解像度(1)
6-1. Blind Face Restoration via Deep Multi-scale Component Dictionaries
Authors: Xiaoming Li, Chaofeng Chen, Shangchen Zhou, Xianhui Lin, Wangmeng Zuo, Lei Zhang
Units: Faculty of Computing, Harbin Institute of Technology, Department of Computer Science, The University of Hong Kong, School of Computer Science and Engineering, Nanyang Technological University, DAMO Academy, Alibaba Group, Peng Cheng Lab, Department of Computing, The Hong Kong Polytechnic University
Abstract:
Recent reference-based face restoration methods have received
considerable attention due to their great capability in recovering highfrequency details on real low-quality images. However, most of these
methods require a high-quality reference image of the same identity, making them only applicable in limited scenes. To address this issue, this
paper suggests a deep face dictionary network (termed as DFDNet) to
guide the restoration process of degraded observations. To begin with,
we use K-means to generate deep dictionaries for perceptually significant
face components (i.e., left/right eyes, nose and mouth) from high-quality
images. Next, with the degraded input, we match and select the most similar component features from their corresponding dictionaries and transfer the high-quality details to the input via the proposed dictionary feature transfer (DFT) block. In particular, component AdaIN is leveraged to
eliminate the style diversity between the input and dictionary features
(e.g., illumination), and a confidence score is proposed to adaptively fuse
the dictionary feature to the input. Finally, multi-scale dictionaries are
adopted in a progressive manner to enable the coarse-to-fine restoration. Experiments show that our proposed method can achieve plausible
performance in both quantitative and qualitative evaluation, and more
importantly, can generate realistic and promising results on real degraded
images without requiring an identity-belonging reference. The source code
and models are available at https://github.com/csxmli2016/DFDNet.
6-2. Face Super-Resolution Guided by 3D Facial Priors
Authors: Xiaobin Hu, Wenqi Ren, John LaMaster, Xiaochun Cao, Xiaoming Li, Zechao Li, Bjoern Menze, Wei Liu
Units: Informatics, Technische Universit¨at M¨unchen, SKLOIS, IIE, CAS, Harbin Institute of Technology, NJUST, Tencent AI Lab, Peng Cheng Laboratory, Cyberspace Security Research Center
Abstract:
State-of-the-art face super-resolution methods employ deep
convolutional neural networks to learn a mapping between low- and highresolution facial patterns by exploring local appearance knowledge. However, most of these methods do not well exploit facial structures and
identity information, and struggle to deal with facial images that exhibit large pose variations. In this paper, we propose a novel face superresolution method that explicitly incorporates 3D facial priors which
grasp the sharp facial structures. Our work is the first to explore 3D
morphable knowledge based on the fusion of parametric descriptions of
face attributes (e.g., identity, facial expression, texture, illumination, and
face pose). Furthermore, the priors can easily be incorporated into any
network and are extremely efficient in improving the performance and
accelerating the convergence speed. Firstly, a 3D face rendering branch is
set up to obtain 3D priors of salient facial structures and identity knowledge. Secondly, the Spatial Attention Module is used to better exploit
this hierarchical information (i.e., intensity similarity, 3D facial structure, and identity content) for the super-resolution problem. Extensive
experiments demonstrate that the proposed 3D priors achieve superior
face super-resolution results over the state-of-the-arts.
7. なりすまし防止(2)
7-1. CelebA-Spoof: Large-Scale Face Anti-Spoofing Dataset with Rich Annotations
Authors: Yuanhan Zhang, ZhenFei Yin, Yidong Li, Guojun Yin, Junjie Yan, Jing Shao, Ziwei Liu
Units: Beijing Jiaotong University, SenseTime Group Limited, The Chinese University of Hong Kong
Abstract:
As facial interaction systems are prevalently deployed, security and reliability of these systems become a critical issue, with substantial research efforts devoted. Among them, face anti-spoofing emerges as
an important area, whose objective is to identify whether a presented
face is live or spoof. Though promising progress has been achieved, existing works still have difficulty in handling complex spoof attacks and
generalizing to real-world scenarios. The main reason is that current
face anti-spoofing datasets are limited in both quantity and diversity. To
overcome these obstacles, we contribute a large-scale face anti-spoofing
dataset, CelebA-Spoof, with the following appealing properties: 1)
Quantity: CelebA-Spoof comprises of 625,537 pictures of 10,177 subjects,
significantly larger than the existing datasets. 2) Diversity: The spoof
images are captured from 8 scenes (2 environments * 4 illumination conditions) with more than 10 sensors. 3) Annotation Richness: CelebA-Spoof contains 10 spoof type annotations, as well as the 40 attribute annotations inherited from the original CelebA dataset. Equipped with CelebA-Spoof, we carefully benchmark existing methods in a unified multi-task framework, Auxiliary Information Embedding Network (AENet), and
reveal several valuable observations. Our key insight is that, compared
with the commonly-used binary supervision or mid-level geometric representations, rich semantic annotations as auxiliary tasks can greatly boost the performance and generalizability of face anti-spoofing across a wide range of spoof attacks. Through comprehensive studies, we show that
CelebA-Spoof serves as an effective training data source. Models trained
on CelebA-Spoof (without fine-tuning) exhibit state-of-the-art performance on standard benchmarks such as CASIA-MFSD. The datasets are available at https://github.com/Davidzhangyuanhan/CelebA-Spoof.
こちらの論文は、AI-SCHOLARで論文紹介の記事を投稿しています。是非ご覧ください。
7-2. On Disentangling Spoof Trace for Generic Face Anti-Spoofing
Authors: Yaojie Liu, Joel Stehouwer, Xiaoming Liu
Units: Michigan State University
Abstract:
Prior studies show that the key to face anti-spoofing lies in the subtle image pattern, termed "spoof trace", e.g., color distortion, 3D mask edge, Moire pattern, and many others. Designing a generic anti-spoofing model to estimate those spoof traces can improve not only the generalization of the spoof detection, but also the interpretability of the model's decision. Yet, this is a challenging task due to the diversity of spoof types and the lack of ground truth in spoof traces. This work designs a novel adversarial learning framework to disentangle the spoof traces from input faces as a hierarchical combination of patterns at multiple scales. With the disentangled spoof traces, we unveil the live counterpart of the original spoof face, and further synthesize realistic new spoof faces after a proper geometric correction. Our method demonstrates superior spoof detection performance on both seen and unseen spoof scenarios while providing visually convincing estimation of spoof traces. Code is available at this https URL.
こちらの論文は、AI-SCHOLARで論文紹介の記事を投稿しています。是非ご覧ください。
8. 顔画像生成(3)
8-1. CONFIG: Controllable Neural Face Image Generation
Authors: Marek Kowalski, Stephan J. Garbin, Virginia Estellers, Tadas Baltrušaitis, Matthew Johnson, Jamie Shotton
Units: Microsoft
Abstract:
Our ability to sample realistic natural images, particularly faces, has advanced by leaps and bounds in recent years, yet our ability to exert fine-tuned control over the generative process has lagged behind. If this new technology is to find practical uses, we need to achieve a level of control over generative networks which, without sacrificing realism, is on par with that seen in computer graphics and character animation. To this end we propose ConfigNet, a neural face model that allows for controlling individual aspects of output images in semantically meaningful ways and that is a significant step on the path towards finely-controllable neural rendering. ConfigNet is trained on real face images as well as synthetic face renders. Our novel method uses synthetic data to factorize the latent space into elements that correspond to the inputs of a traditional rendering pipeline, separating aspects such as head pose, facial expression, hair style, illumination, and many others which are very hard to annotate in real data. The real images, which are presented to the network without labels, extend the variety of the generated images and encourage realism. Finally, we propose an evaluation criterion using an attribute detection network combined with a user study and demonstrate state-of-the-art individual control over attributes in the output images.
8-2. High Resolution Zero-Shot Domain Adaptation of Synthetically Rendered Face Images
Authors: Stephan J. Garbin, Marek Kowalski, Matthew Johnson, Jamie Shotton
Units: Microsoft
Abstract:
Generating photorealistic images of human faces at scale remains a prohibitively difficult task using computer graphics approaches.
This is because these require the simulation of light to be photorealistic,
which in turn requires physically accurate modelling of geometry, materials, and light sources, for both the head and the surrounding scene.
Non-photorealistic renders however are increasingly easy to produce. In
contrast to computer graphics approaches, generative models learned
from more readily available 2D image data have been shown to produce
samples of human faces that are hard to distinguish from real data. The
process of learning usually corresponds to a loss of control over the shape
and appearance of the generated images. For instance, even simple disentangling tasks such as modifying the hair independently of the face,
which is trivial to accomplish in a computer graphics approach, remains
an open research question. In this work, we propose an algorithm that
matches a non-photorealistic, synthetically generated image to a latent
vector of a pretrained StyleGAN2 model which, in turn, maps the vector
to a photorealistic image of a person of the same pose, expression, hair,
and lighting. In contrast to most previous work, we require no synthetic
training data. To the best of our knowledge, this is the first algorithm of
its kind to work at a resolution of 1K and represents a significant leap
forward in visual realism.
8-3. Speech-driven Facial Animation using Cascaded GANs for Learning of Motion and Texture
Authors: Dipanjan Das, Sandika Biswas, Sanjana Sinha, Brojeshwar Bhowmick
Units: Embedded Systems and Robotics, TCS Research
Abstract:
Speech-driven facial animation methods should produce accurate and realistic lip motions with natural expressions and realistic texture portraying target-specific facial characteristics. Moreover,the methods should also be adaptable to any unknown faces and speech quickly during inference. Current state-of-the-art methods fail to generate realistic animation from any speech on unknown faces due to their poor generalization over different facial characteristics, languages, and accents. Some of these failures can be attributed to the end-to-end learning of the complex relationship between the multiple modalities of speech and the video. In this paper, we propose a novel strategy where we partition the problem and learn the motion and texture separately. Firstly, we train a GAN network to learn the lip motion in a canonical landmark using DeepSpeech features and induce eye-blinks before transferring the motion to the person-specific face. Next, we use another GAN based texture generator network to generate high fidelity face corresponding to the motion on person-specific landmark. We use meta-learning to make the texture generator GAN more flexible to adapt to the unknown subject’s traits of the face during inference. Our method gives significantly improved facial animation than the state-of-the-art methods and generalizes well across the different datasets, different languages, and accents, and also works reliably well in presence of noises in the speech.
9. 顔の偽造検出(1)
9-1. Thinking in Frequency: Face Forgery Detection by Mining Frequency-aware Clues
Authors: Yuyang Qian, Guojun Yin, Lu Sheng, Zixuan Chen, Jing Shao
Units: Microsoft
Abstract:
As realistic facial manipulation technologies have achieved remarkable progress, social concerns about potential malicious abuse of these technologies bring out an emerging research topic of face forgery detection. However, it is extremely challenging since recent advances are able to forge faces beyond the perception ability of human eyes, especially in compressed images and videos. We find that mining forgery patterns with the awareness of frequency could be a cure, as frequency provides a complementary viewpoint where either subtle forgery artifacts or compression errors could be well described. To introduce frequency into the face forgery detection, we propose a novel Frequency in Face Forgery Network (F3-Net), taking advantages of two different but complementary frequency-aware clues, 1) frequency-aware decomposed image components, and 2) local frequency statistics, to deeply mine the forgery patterns via our two-stream collaborative learning framework. We apply DCT as the applied frequency-domain transformation. Through comprehensive studies, we show that the proposed F3-Net significantly outperforms competing state-of-the-art methods on all compression qualities in the challenging FaceForensics++ dataset, especially wins a big lead upon low-quality media.
こちらの論文は、AI-SCHOLARで論文紹介の記事を投稿しています。是非ご覧ください。
10. 顔の分析(1)
10-1. Edge-aware Graph Representation Learning and Reasoning for Face Parsing
Authors: Gusi Te, Yinglu Liu, Wei Hu, Hailin Shi, Tao Mei
Units: Wangxuan Institute of Computer Technology, Peking University, JD AI Research
Abstract:
Face parsing infers a pixel-wise label to each facial component, which has drawn much attention recently. Previous methods have shown their efficiency in face parsing, which however overlook the correlation among different face regions. The correlation is a critical clue about the facial appearance, pose, expression etc., and should be taken into account for face parsing. To this end, we propose to model and reason the region-wise relations by learning graph representations, and leverage the edge information between regions for optimized abstraction. Specifically, we encode a facial image onto a global graph representation where a collection of pixels ("regions") with similar features are projected to each vertex. Our model learns and reasons over relations between the regions by propagating information across vertices on the graph. Furthermore, we incorporate the edge information to aggregate the pixel-wise features onto vertices, which emphasizes on the features around edges for fine segmentation along edges. The finally learned graph representation is projected back to pixel grids for parsing. Experiments demonstrate that our model outperforms state-of-the-art methods on the widely used Helen dataset, and also exhibits the superior performance on the large-scale CelebAMask-HQ and LaPa dataset. The code is available at this https URL.
11. 顔の正面化(1)
Authors: Yuxiang Wei, Ming Liu, Haolin Wang, Ruifeng Zhu, Guosheng Hu, Wangmeng Zuo
Units: School of Computer Science and Technology, Harbin Institute of Technology, University of Burgundy Franche-Comté, Anyvision, University of the Basque Country, Peng Cheng Lab
Abstract:
Despite recent advances in deep learning-based face frontalization methods, photo-realistic and illumination preserving frontal face
synthesis is still challenging due to large pose and illumination discrepancy during training. We propose a novel Flow-based Feature Warping
Model (FFWM) which can learn to synthesize photo-realistic and illumination preserving frontal images with illumination inconsistent supervision. Specifically, an Illumination Preserving Module (IPM) is proposed to learn illumination preserving image synthesis from illumination inconsistent image pairs. IPM includes two pathways which collaborate to
ensure the synthesized frontal images are illumination preserving and
with fine details. Moreover, a Warp Attention Module (WAM) is introduced to reduce the pose discrepancy in the feature level, and hence to
synthesize frontal images more effectively and preserve more details of
profile images. The attention mechanism in WAM helps reduce the artifacts caused by the displacements between the profile and the frontal
images. Quantitative and qualitative experimental results show that our
FFWM can synthesize photo-realistic and illumination preserving frontal
images and performs favorably against the state-of-the-art results. Our
code is available at https://github.com/csyxwei/FFWM.
12. 顔の属性編集(3)
12-1. CooGAN: A Memory-Efficient Framework for High-Resolution Facial Attribute Editing
Authors: Xuanhong Chen, Bingbing Ni, Naiyuan Liu, Ziang Liu, Yiliu Jiang, Loc Truong, Qi Tian
Units: Shanghai Jiao Tong University, Huawei HiSilicon, Huawei
Abstract:
In contrast to great success of memory-consuming face editing methods at a low resolution, to manipulate high-resolution (HR) facial images, i.e., typically larger than 7682 pixels, with very limited memory is still challenging. This is due to the reasons of 1) intractable huge demand of memory; 2) inefficient multi-scale features fusion. To address these issues, we propose a NOVEL pixel translation framework called Cooperative GAN (CooGAN) for HR facial image editing. This framework features a local path for fine-grained local facial patch generation (i.e., patch-level HR, LOW memory) and a global path for global lowresolution (LR) facial structure monitoring (i.e., image-level LR, LOW memory), which largely reduce memory requirements. Both paths work in a cooperative manner under a local-to-global consistency objective (i.e., for smooth stitching). In addition, we propose a lighter selective transfer unit for more efficient multi-scale features fusion, yielding higher fidelity facial attributes manipulation. Extensive experiments on CelebAHQ well demonstrate the memory efficiency as well as the high image generation quality of the proposed framework.
12-2. SSCGAN: Facial Attribute Editing via Style Skip Connections
Authors: Wenqing Chu, Ying Tai, Chengjie Wang, Jilin Li, Feiyue Huang, Rongrong Ji
Units: Youtu Lab, Tencent, Xiamen University
Abstract:
Existing facial attribute editing methods typically employ an encoder-decoder architecture where the attribute information is expressed as a conditional one-hot vector spatially concatenated with the image or intermediate feature maps. However, such operations only learn the local semantic mapping but ignore global facial statistics. In this work, we focus on solving this issue by editing the channel-wise global information denoted as the style feature. We develop a style skip connection based generative adversarial network, referred to as SSCGAN which enables accurate facial attribute manipulation. Specifically, we inject the target attribute information into multiple style skip connection paths between the encoder and decoder. Each connection extracts the style feature of the latent feature maps in the encoder and then performs a residual learning based mapping function in the global information space guided by the target attributes. In the following, the adjusted style feature will be utilized as the conditional information for instance normalization to transform the corresponding latent feature maps in the decoder. In addition, to avoid the vanishing of spatial details (e.g. hairstyle or pupil locations), we further introduce the skip connection based spatial information transfer module. Through the global-wise style and local-wise spatial information manipulation, the proposed method can produce better results in terms of attribute generation accuracy and image quality. Experimental results demonstrate the proposed algorithm performs favorably against the state-of-the-art methods.
12-3. ByeGlassesGAN: Identity Preserving Eyeglasses Removal for Face Images
Authors: Yu-Hui Lee, Shang-Hong Lai
Units: Department of Computer Science, National Tsing Hua University, Microsoft AI R&D Center
Abstract:
In this paper, we propose a novel image-to-image GAN framework for eyeglasses removal, called ByeGlassesGAN, which is used to automatically detect the position of eyeglasses and then remove them from face images. Our ByeGlassesGAN consists of an encoder, a face decoder, and a segmentation decoder. The encoder is responsible for extracting
information from the source face image, and the face decoder utilizes
this information to generate glasses-removed images. The segmentation
decoder is included to predict the segmentation mask of eyeglasses and
completed face region. The feature vectors generated by the segmentation
decoder are shared with the face decoder, which facilitates better reconstruction results. Our experiments show that ByeGlassesGAN can provide visually appealing results in the eyeglasses-removed face images even for semi-transparent color eyeglasses or glasses with glare. Furthermore, we demonstrate significant improvement in face recognition accuracy for face images with glasses by applying our method as a pre-processing step in our face recognition experiment.
13. 顔の表情認識(1)
13-1. Margin-Mix: Semi–Supervised Learning for Face Expression Recognition
Authors: Corneliu Florea, Mihai Badea, Laura Florea, Andrei Racoviteanu, Constantin Vertan
Units: Image Processing and Analysis Laboratory, Unversity Politehnica of Bucharest
Abstract:
In this paper, as we aim to construct a semi-supervised learning algorithm, we exploit the characteristics of the Deep Convolutional Networks to provide, for an input image, both an embedding descriptor and a prediction. The unlabeled data is combined with the labeled one in order to provide synthetic data, which describes better the input space. The network is asked to provide a large margin between clusters, while new data is self-labeled by the distance to class centroids, in the embedding space. The method is tested on standard benchmarks for semi–supervised learning, where it matches state of the art performance and on the problem of face expression recognition where it increases the accuracy by a noticeable margin.
14. 顔の表情編集(2)
14-1. Toward Fine-grained Facial Expression Manipulation
Authors: Jun Ling, Han Xue, Li Song, Shuhui Yang, Rong Xie, Xiao Gu
Units: Institute of Image Communication and Network Engineering, Shanghai Jiao Tong University, MoE Key Lab of Artificial Intelligence, AI Institute, Shanghai Jiao Tong University
Abstract:
Facial expression manipulation aims at editing facial expression with a given condition. Previous methods edit an input image under the guidance of a discrete emotion label or absolute condition (e.g., facial action units) to possess the desired expression. However, these methods either suffer from changing condition-irrelevant regions or are inefficient for fine-grained editing. In this study, we take these two objectives into consideration and propose a novel method. First, we replace continuous absolute condition with relative condition, specifically, relative action units. With relative action units, the generator learns to only transform regions of interest which are specified by non-zero-valued relative AUs. Second, our generator is built on U-Net but strengthened by multi-scale feature fusion (MSF) mechanism for high-quality expression editing purposes. Extensive experiments on both quantitative and qualitative evaluation demonstrate the improvements of our proposed approach compared to the state-of-the-art expression editing methods. Code is available at https://github.com/junleen/Expression-manipulator.
14-2. Learning to Generate Customized Dynamic 3D Facial Expressions
Authors: Rolandos Alexandros Potamias, Jiali Zheng, Stylianos Ploumpis, Giorgos Bouritsas, Evangelos Ververas, Stefanos Zafeiriou
Units: Department of Computing, Imperial College London
Abstract:
Recent advances in deep learning have significantly pushed the state-of-the-art in photorealistic video animation given a single image. In this paper, we extrapolate those advances to the 3D domain, by studying 3D image-to-video translation with a particular focus on 4D facial expressions. Although 3D facial generative models have been widely explored during the past years, 4D animation remains relatively unexplored. To this end, in this study we employ a deep mesh encoder-decoder like architecture to synthesize realistic high resolution facial expressions by using a single neutral frame along with an expression identification. In addition, processing 3D meshes remains a non-trivial task compared to data that live on grid-like structures, such as images. Given the recent progress in mesh processing with graph convolutions, we make use of a recently introduced learnable operator which acts directly on the mesh structure by taking advantage of local vertex orderings. In order to generalize to 4D facial expressions across subjects, we trained our model using a high resolution dataset with 4D scans of six facial expressions from 180 subjects. Experimental results demonstrate that our approach preserves the subject's identity information even for unseen subjects and generates high quality expressions. To the best of our knowledge, this is the first study tackling the problem of 4D facial expression synthesis.
15. バイアス関連(1)
15-1. Towards causal benchmarking of bias in face analysis algorithms
Authors: Guha Balakrishnan, Yuanjun Xiong, Wei Xia, Pietro Perona
Units: Massachusetts Institute of Technology, California Institute of Technology, Amazon Web Services
Abstract:
Measuring algorithmic bias is crucial both to assess algorithmic fairness, and to guide the improvement of algorithms. Current bias measurement methods in computer vision are based on observational datasets, and so conflate algorithmic bias with dataset bias. To address this problem we develop an experimental method for measuring algorithmic bias of face analysis algorithms, which directly manipulates the attributes of interest, e.g., gender and skin tone, in order to reveal causal links between attribute variation and performance change. Our method is based on generating synthetic image grids that differ along specific attributes while leaving other attributes constant. Crucially, we rely on the perception of human observers to control for synthesis inaccuracies when measuring algorithmic bias. We validate our method by comparing it to a traditional observational bias analysis study in gender classification algorithms. The two methods reach different conclusions. While the observational method reports gender and skin color biases, the experimental method reveals biases due to gender, hair length, age, and facial hair. We also show that our synthetic transects allow for more straightforward bias analysis on minority and intersectional groups.
16. 顔の匿名化(1)
16-1. Password-conditioned Anonymization and Deanonymization with Face Identity Transformers
Authors: Xiuye Gu, Weixin Luo, Michael S. Ryoo, Yong Jae Lee
Units: Stanford University, UC Davis, ShanghaiTech, Stony Brook University
Abstract:
Cameras are prevalent in our daily lives, and enable many useful systems built upon computer vision technologies such as smart cameras and home robots for service applications. However, there is also an increasing societal concern as the captured images/videos may contain privacy-sensitive information (e.g., face identity). We propose a novel face identity transformer which enables automated photo-realistic password-based anonymization and deanonymization of human faces appearing in visual data. Our face identity transformer is trained to (1) remove face identity information after anonymization, (2) recover the original face when given the correct password, and (3) return a wrong—but photo-realistic—face given a wrong password. With our carefully designed password scheme and multi-task learning objective, we achieve both anonymization and deanonymization using the same single network. Extensive experiments show that our method enables multimodal password conditioned anonymizations and deanonymizations, without sacrificing privacy compared to existing anonymization methods.
17. その他(2)
17-1. Neural Voice Puppetry: Audio-driven Facial Reenactment
Authors: Justus Thies, Mohamed Elgharib, Ayush Tewari, Christian Theobalt, Matthias Nießner
Units: Technical University of Munich, Max Planck Institute for Informatics, Saarland Informatics Campus
Abstract:
We present Neural Voice Puppetry, a novel approach for audio-driven facial video synthesis. Given an audio sequence of a source person or digital assistant, we generate a photo-realistic output video of a target person that is in sync with the audio of the source input. This audio-driven facial reenactment is driven by a deep neural network that employs a latent 3D face model space. Through the underlying 3D representation, the model inherently learns temporal stability while we leverage neural rendering to generate photo-realistic output frames. Our approach generalizes across different people, allowing us to synthesize videos of a target actor with the voice of any unknown source actor or even synthetic voices that can be generated utilizing standard text-tospeech approaches. Neural Voice Puppetry has a variety of use-cases, including audio-driven video avatars, video dubbing, and text-driven video synthesis of a talking head. We demonstrate the capabilities of our method in a series of audio- and text-based puppetry examples, including comparisons to state-of-the-art techniques and a user study.
17-2. Learning to Predict Salient Faces: A Novel Visual-Audio Saliency Model
Authors: Yufan Liu, Minglang Qiao, Mai Xu, Bing Li, Weiming Hu, Ali Borji
Units: National Laboratory of Pattern Recognition, CASIA, University of Chinese Academy of Sciences, CEBSIT, Beihang University & Hangzhou Innovation Institute, Beihang University, MarkableAI Inc.
Abstract:
Recently, video streams have occupied a large proportion of
Internet traffic, most of which contain human faces. Hence, it is necessary to predict saliency on multiple-face videos, which can provide
attention cues for many content based applications. However, most of
multiple-face saliency prediction works only consider visual information
and ignore audio, which is not consistent with the naturalistic scenarios. Several behavioral studies have established that sound influences
human attention, especially during the speech turn-taking in multipleface videos. In this paper, we thoroughly investigate such influences by
establishing a large-scale eye-tracking database of Multiple-face Video in
Visual-Audio condition (MVVA). Inspired by the findings of our investigation, we propose a novel multi-modal video saliency model consisting of three branches: visual, audio and face. The visual branch takes the RGB frames as the input and encodes them into visual feature maps. The audio and face branches encode the audio signal and multiple
cropped faces, respectively. A fusion module is introduced to integrate
the information from three modalities, and to generate the final saliency
map. Experimental results show that the proposed method outperforms
11 state-of-the-art saliency prediction works. It performs closer to human
multi-modal attention.
この記事が気に入ったらサポートをしてみませんか?