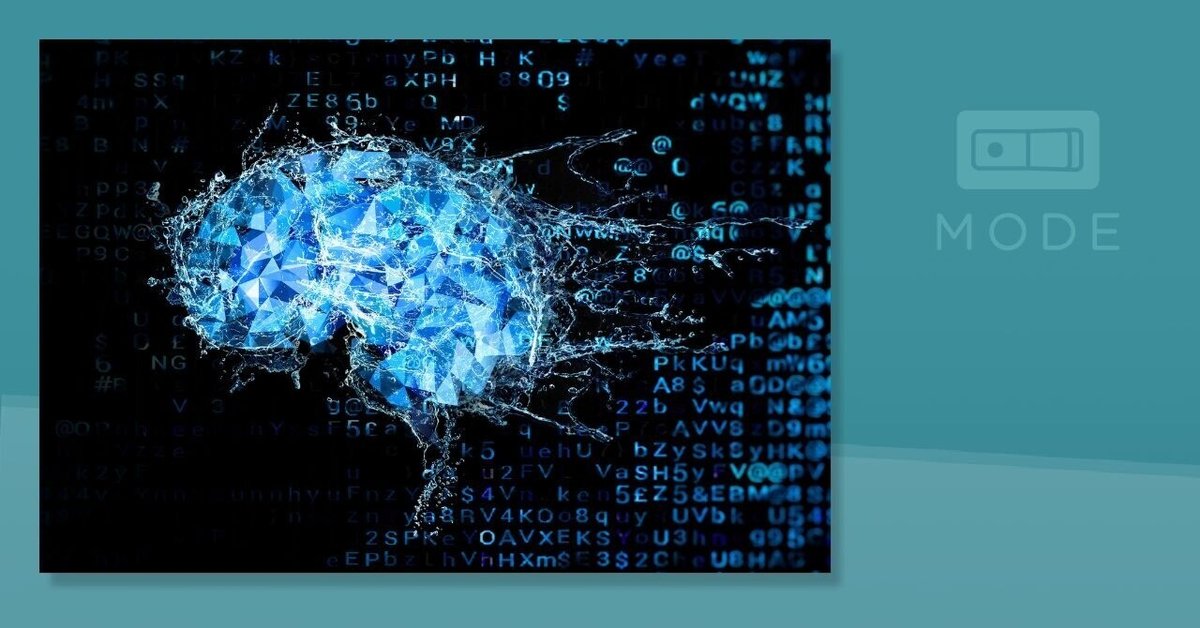
Maximizing Business Returns through Strategic AI Implementation
日本語版:
Author:Mark Shotton, Head of US Business Development, MODE, Inc.
In the ever-evolving business landscape, grasping the intricacies of artificial intelligence (AI) is crucial for success. AI, particularly in its generative and traditional forms, is pivotal in shaping business strategies, improving efficiencies and helps your business achieve a true digital transformation. However, many businesses hesitate to fully embrace AI due to uncertainties about its capabilities and limitations. Perceived as risky and expensive, AI presents challenges for decision-makers. Yet, educating oneself about AI, managing risks, and collaborating with experts can provide a clear framework for aligning AI goals with desired business outcomes, representing a wise investment.
AI's potential to enhance operational efficiencies, predictive maintenance, and realize cost and time savings is increasingly recognized. Working with a knowledgeable team is essential, as AI's effectiveness hinges on the quality of the data it's trained on. Ensuring data security, reliability, and originality is vital. AI is not just a tool; it's a paradigm shift in business thinking, essential for digital transformation.
Research from Harvard Business Review indicates that 67% of senior IT leaders are prioritizing generative AI within the next 18 months, with 33% considering it a top priority. This trend underscores the importance of understanding these AI forms to make informed decisions.
So, let’s dive into two forms of AI to better understand their business use cases, nuances, and hopefully help decision makers feel comfortable about embracing this technology that is here to stay. As Benjamin Franklin once said, “An investment in knowledge pays the best interest”.
Traditional AI
Traditional artificial intelligence, also known as Narrow AI or Weak AI, is a subset of artificial intelligence that focuses on performing specific tasks intelligently. It refers to systems designed to respond to a particular set of inputs. These systems have the capability to learn from data and make decisions or predictions based on that data. Traditional AI systems typically operate in a deterministic manner, following explicit rules and instructions set by human programmers. They are rule-based and rely on well-defined algorithms that are derived from structured data. Its main characteristics include:
Rule-Based Systems: These systems follow explicitly programmed instructions to make decisions and solve problems.
Limited Adaptability: Post-deployment, these systems do not generally learn from new data, relying on their original programming.
Specific Applications: Traditional AI is suitable for expert systems, search algorithms, and game AI, where outcomes are predictable.
Deterministic Output: Outputs are consistent and predictable when provided with the same input.
Some familiar examples of Traditional Ai applications
Virtual assistants: like Alexa or Siri
Computer games that predict moves
Searches for e-commerce using algorithms.
Generative AI
Generative AI, a more recent development in AI technology, is adept at creating new content or ideas based on existing data. It has specific applications and is a subset of AI that excels at solving particular tasks in business. The primary difference between Generative AI and Traditional AI lies in their objectives and functioning. While Traditional AI aims to perform specific tasks based on predefined rules and patterns, Generative AI goes beyond this limitation and strives to create entirely new data that resembles human-created content. It is increasingly being integrated with IoT (Internet of Things) systems and platforms, bringing numerous benefits. Generative AI offers a privacy-preserving approach by generating synthetic data that maintains the statistical properties of the original dataset while ensuring individual privacy. This approach enables data sharing and collaboration while safeguarding sensitive information. Some characteristics include:
Learning from Data: These systems evolve by learning from large datasets, generating outputs that are not pre-programmed.
Creativity and Novelty: Generative AI can create original content like text, images, showcasing a level of creativity akin to human ingenuity.
Diverse Applications: Refers to a wide range of uses and industries where this technology can be applied, showcasing its flexibility and adaptability.
Non-Deterministic Outputs: The same input can lead to different outputs, highlighting its dynamic nature.
Examples of Generative Ai applications
Text generation: Language models that can generate human-like text, including giving updates on mechanical systems, identifying anomalies, given a specific prompt.
Reports: Generate automated reports to optimize inventory levels, preventative maintenance or check if equipment is working correctly.
Alert notifications: Get live updates with time series data.
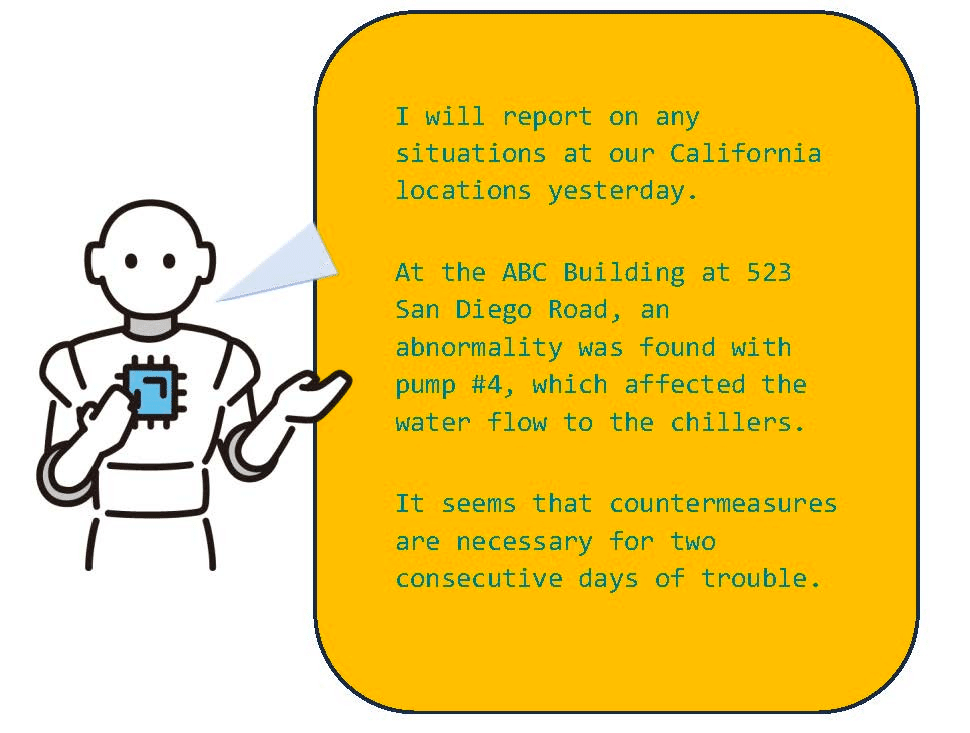
Industry Applications
Generative AI combined with IoT has seen significant application across various sectors:
Construction: Using smartphones for reporting on jobsites, monitor sites or equipment.
Manufacturing: Used in predictive maintenance, quality control, and process optimization.
Healthcare: Revolutionizing operational efficiencies with updates on remote monitoring, keeping track of assets or generating reports.
Agriculture: Employed for precision farming, crop monitoring, and yield optimization.
Other Industries: Includes energy, retail, smart cities, and environmental monitoring.
The Importance of Technology Partners
The market appears to be segmented into established AI companies, IoT platform providers, cloud service providers, and specialized generative AI startups, each contributing uniquely to the IoT generative AI landscape. Working with these partners individually will not only add up in costs, but may have data collecting and integration issues, and possibilities that may lead to compromised outcomes. Having the correct methods and IoT systems to gather useful data and insights, properly securing the data in the cloud for ongoing use with AI and working with a team that is knowledgeable about how all these systems work together is the key to reaping the rewards of a generative Ai platform.
Key Differences between Traditional Ai and Generative AI
Learning Ability: Generative AI adapts and learns from data, unlike traditional AI.
Output Nature: Traditional AI offers predictable outputs, while generative AI can produce diverse and novel outputs.
Flexibility: Generative AI is more adaptable to new data and scenarios.
Application Scope: Traditional AI is suited for structured tasks, whereas generative AI excels in creative and dynamic environments.
Conclusion
The differentiation between generative and traditional AI marks a significant progression in AI technology. Traditional AI thrives in structured environments, while generative AI introduces a level of creativity and adaptability previously unattainable. Generative AI can create something new from the information you gave it. For businesses, the possibilities are endless, and it can give you a return on your investment (ROI) unseen before.Done correctly, generative AI is safe and more attainable than you may think. As AI continues to evolve, the distinction between these two forms may become less pronounced, leading to the emergence of more sophisticated and versatile AI systems.
We have only just started the journey of AI innovation and business applications. Going forward, recognizing the unique capabilities of these different forms on AI will allow you to harness their full potential without compromising data.