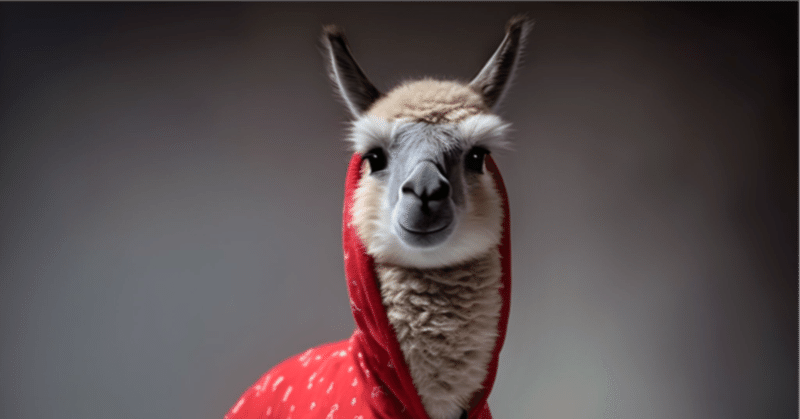
RedPajama-INCITE のInstructモデルの活用事例
以下の記事をもとに、「RedPajama-INCITE」のInstructモデルの活用事例をまとめました。
1. RedPajama-INCITE のInstructモデル
「RedPajama-INCITE-3B / 7B」では、「Base」「Instruct」「Chat」の3種類のモデルがリリースされました。
「Base」は、Instructチューニング前のベースモデル、「Instruct」はFew-Shotアプリケーション用にInstructチューニングしたモデル、「Chat」はチャットアプリケーション用にInstructチューニングしたモデルになります。
「HELM」の評価で、「RedPajama-INCITE-Instruct-3B-v1」は「Llama-7B」(Instructチューニングなし) に僅差で下回り、「RedPajama-INCITE-Instruct-7B-v0.1」は「Llama-7B」を上回っています。
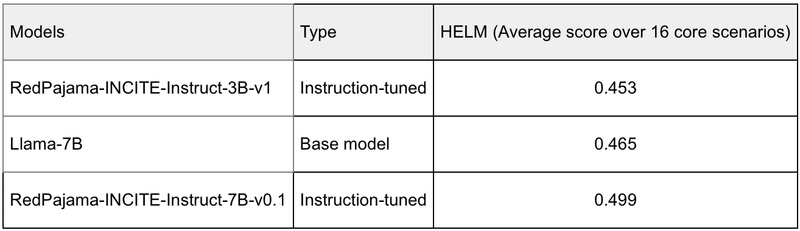
2. RedPajama-INCITE のInstructモデルの活用事例
実世界でのLLMの活用事例の多くは、「Few-Shotプロンプト」が使われています。リーガルAIのスタートアップ「Standd」は、弁護士が非構造化データから、法律業務や事業展開に関するインサイトの生成を支援します。彼らは、「RedPajama 7B」を使って、さまざまなタスクに「Few-Shotプロンプト」を使用しています。
以下はその一例になります。
2-1. 重要な文章のハイライト
通常文字がプロンプト、太字がLLMが生成したレスポンスになります。
You are a sentence highlighter. You highlight sentences that can be used to answer a specific question. To highlight a sentence you return the full text of a sentence in a bulleted list. If none should be highlighted return None.
Question: Who is the cow?
Paragraph: My name is Stephen. I am a cow. My favorite drink is soda.
Highlights:
- My name is Stephen.
- I am a cow.
Question: Who is the duck?
Paragraph: Today was a weird day. The sun was hot and I am tired.
Highlights:
None
Question: What is the largest deer?
Paragraph: The moose (in North America) or elk (in Eurasia) (Alces alces) is a member of the New World deer subfamily and is the only species in the genus Alces. It is the largest and heaviest extant species in the deer family. Most adult male moose have distinctive broad, palmate ("open-hand shaped") antlers; most other members of the deer family have antlers with a dendritic ("twig-like") configuration. Moose typically inhabit boreal forests and temperate broadleaf and mixed forests of the Northern Hemisphere in temperate to subarctic climates. Hunting and other human activities have caused a reduction in the size of the moose's range over time. It has been reintroduced to some of its former habitats. Currently, most moose occur in Canada, Alaska, New England (with Maine having the most of the lower 48 states), New York State, Fennoscandia, the Baltic states, Poland, Kazakhstan, and Russia.
Highlights:
- The moose (in North America) or elk (in Eurasia) (Alces alces) is a member of the New World deer subfamily and is the only species in the genus Alces
- It is the largest and heaviest extant species in the deer family.
Question: What architecture is RedPajama based on?
Paragraph: RedPajama-INCITE-Base-3B-v1 is trained over the RedPajama v1 dataset, with the same architecture as the popular Pythia model suite. We chose to start with the Pythia architecture to understand the value of training with the much larger RedPajama dataset with respect to the current leading open-source dataset, the Pile. Training on Summit leveraged the DeeperSpeed codebase developed by EleutherAI. We are excited to see that at 800B tokens, RedPajama-Base-INCITE-3B has better few-shot performance (measured in HELM, as the average score over 16 core scenarios) and better zero-shot performance (measured in Eleuther’s LM evaluation harness) compared with open models of similar size, including the well-regarded GPT-Neo and Pythia-2.8B (trained with 420B and 300B tokens, respectively, with the Pile). On HELM, it outperforms these models by 3-5 points. On a subset of tasks from lm-evaluation-harness, outperforms these open models by 2-7 points.
Highlights:
- RedPajama-INCITE-Base-3B-v1 is trained over the RedPajama v1 dataset, with the same architecture as the popular Pythia model suite.
- We chose to start with the Pythia architecture to understand the value of training with the much larger RedPajama dataset with respect to the current leading open-source dataset, the Pile.
- Training on Summit leveraged the DeeperSpeed codebase developed by EleutherAI.
【翻訳】
あなたは文章を強調表示する人です。 特定の質問に答えるために使用できる文を強調表示します。 文を強調表示するには、文の全文を箇条書きリストで返します。 何も強調表示する必要がない場合は、None を返します。
Question: 牛は誰ですか?
Paragraph: 私の名前はスティーブンです。 私は牛です。 私の好きな飲み物はソーダです。
Highlights:
- 私の名前はスティーブンです。
- 私は牛です。
Question: アヒルは誰ですか?
Paragraph: 今日は変な日でした。 日差しが暑くて疲れました。
Highlights:
なし
Question: 最大の鹿は何ですか?
Paragraph: ヘラジカ (北アメリカ) またはヘラジカ (ユーラシア) (Alces alces) は、新世界シカ亜科のメンバーであり、Alces 属の唯一の種です。 現存するシカ科の中で最大かつ最も重い種です。 成体の雄のヘラジカのほとんどは、特徴的な幅広の掌状 (「開いた手の形」) の角を持っています。 シカ科の他のほとんどのメンバーは、樹枝状 (「小枝のような」) 構造の角を持っています。 ヘラジカは通常、北半球の温帯から亜寒帯気候の北方林、温帯広葉樹林および混交林に生息しています。 狩猟やその他の人間の活動により、ヘラジカの生息範囲は時間の経過とともに減少してきました。 以前の生息地の一部に再導入されました。 現在、ヘラジカのほとんどはカナダ、アラスカ、ニューイングランド(メイン州が下位48州のほとんどを占める)、ニューヨーク州、フェノスカンディア、バルト三国、ポーランド、カザフスタン、ロシアで発生している。
Highlights:
- ヘラジカ (北アメリカ) またはヘラジカ (ユーラシア) (Alces alces) は、新世界シカ亜科のメンバーであり、Alces 属の唯一の種です。
- 現存するシカ科の中で最大かつ最も重い種です。
Question: RedPajama はどのようなアーキテクチャに基づいていますか?
Paragraph: RedPajama-INCITE-Base-3B-v1 は、人気のある Pythia モデル スイートと同じアーキテクチャを備えた RedPajama v1 データセット上でトレーニングされます。 現在の主要なオープンソース データセットである Pile と比較して、はるかに大規模な RedPajama データセットを使用したトレーニングの価値を理解するために、Pythia アーキテクチャから始めることにしました。 Summit のトレーニングでは、EleutherAI が開発した DeeperSpeed コードベースを活用しました。 800B トークンで、RedPajama-Base-INCITE-3B の少数ショット パフォーマンス (16 コア シナリオの平均スコアとして HELM で測定) とゼロショット パフォーマンス (Eleuther の LM 評価ハーネスで測定) が優れていることを確認して興奮しています。 ) 評判の高い GPT-Neo および Pythia-2.8B (Pile でそれぞれ 420B および 300B トークンでトレーニングされた) を含む、同様のサイズのオープン モデルと比較しました。 HELM では、これらのモデルよりも 3 ~ 5 ポイント優れています。 lm-evaluation-harness のタスクのサブセットでは、これらのオープン モデルより 2 ~ 7 ポイント優れています。
Highlights:
- RedPajama-INCITE-Base-3B-v1 は、人気のある Pythia モデル スイートと同じアーキテクチャを備えた RedPajama v1 データセット上でトレーニングされます。
- 現在の主要なオープンソース データセットである Pile よりもはるかに大規模な RedPajama データセットを使用したトレーニングの価値を理解するために、Pythia アーキテクチャから始めることにしました。
- Summit のトレーニングでは、EleutherAI が開発した DeeperSpeed コードベースを活用しました。
2-2. 地理の質問応答
Please answer the following question:
Question: What is the capital of Canada?
Answer: Ottawa
Question: What is the currency of Switzerland?
Answer: Swiss franc
Question: In which country is Wisconsin located?
Answer: United States
【翻訳】
次の質問に答えてください。
Question: カナダの首都はどこですか?
Answer: オタワ
Question: スイスの通貨は何ですか?
Answer: スイスフラン
Question: ウィスコンシン州はどこの国にありますか?
Answer: アメリカ
2-3. 感情分析
Label the tweets as either 'positive', 'negative', 'mixed', or 'neutral':
Tweet: I can say that there isn't anything I would change.
Label: positive
Tweet: I'm not sure about this.
Label: neutral
Tweet: I liked some parts but I didn't like other parts.
Label: mixed
Tweet: I think the background image could have been better.
Label: negative
Tweet: I really like it.
Label: positive
【翻訳】
ツイートに「positive」、「negative」、「mixed」、「neutral」のいずれかのラベルを付けます。
Tweet: 変更するものは何もないと言えます。
Label: positive
Tweet: これについてはわかりません。
Label: neutral
Tweet: いくつかの部分は気に入りましたが、他の部分は好きではありませんでした。
Label: mixed
Tweet: 背景画像はもっと良かったかもしれないと思います。
Label: negative
Tweet: とても気に入っています。
Label: positive
この記事が気に入ったらサポートをしてみませんか?