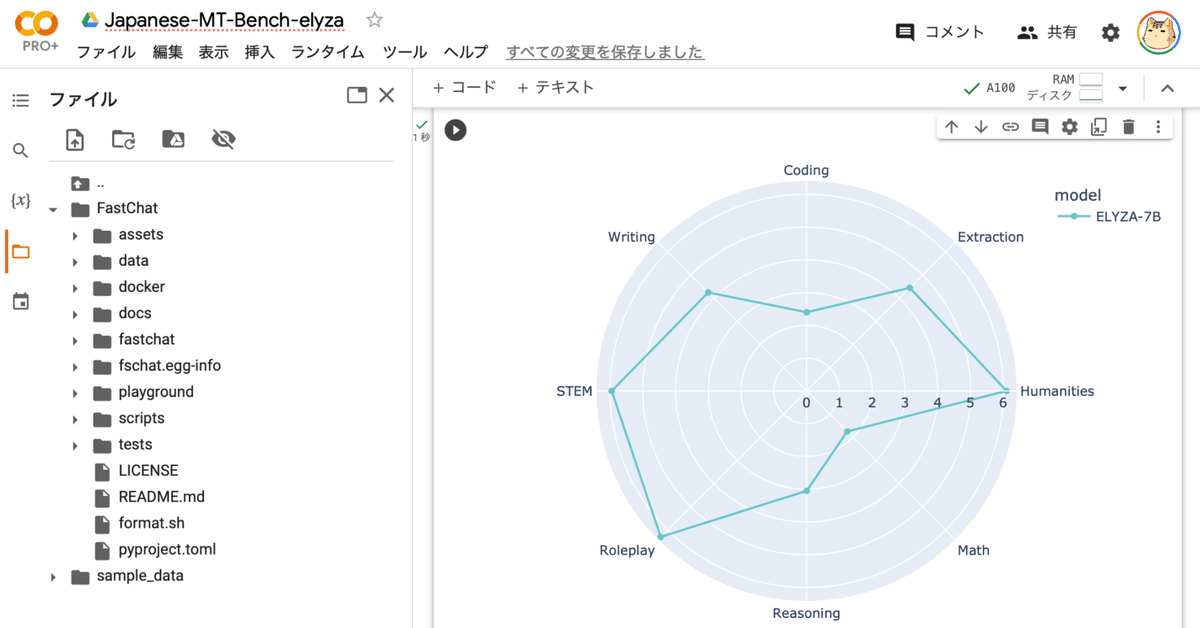
MT-Bench による日本語LLMの評価
「MT-Bench」による日本語LLMの評価の手順をまとめました。
【注意】Google Colab Pro/Pro+ のA100で動作確認しています。
前回
1. Japanese MT-Bench
「LMSys」の「MT-Bench」の質問は英語のため、英語による指示の効き目の評価しかできません。そこで「Stability AI」が提供する「Japanese MT-Bench」の質問ファイルと参照回答ファイルを使います。
今回は「MT-Bench」本体として(「Stability-AI/FastChat」ではなく)「lm-sys/FastChat」を使っています。
2. Japanese MT-Benchのデータの準備
「Japanese MT-Bench」のデータの準備の手順は、次のとおりです。
(1) 以下のフォルダ構成を準備。
「gpt-4.jsonl」をreference_answerフォルダ下に、「question_full.jsonl」を「question.jsonl」に名前変更してjapanese_mt_benchフォルダ下に配置します。
・japanese_mt_benchフォルダ
・reference_answerフォルダ
・gpt-4.jsonl : 参照回答ファイル (80問)
gpt-4.jsonをコピーして配置。
・question.jsonl : 質問ファイル (80問)
question_full.jsonlを名前変更して配置。
(2) japanese_mt_benchフォルダをzip圧縮。
「japanese_mt_bench.zip」が生成されます。
3. セットアップ
Colabでのセットアップ手順は、次のとおりです。
(1) パッケージのインストール。
!git clone https://github.com/lm-sys/FastChat
%cd FastChat
!pip install -e ".[model_worker,webui]"
!pip install openai anthropic
!pip install plotly kaleido
(2) 環境変数の準備。
以下のコードの <OpenAI_APIのトークン> にはOpenAI APIのトークンを指定します。(有料)
# 環境変数の準備
import os
os.environ["OPENAI_API_KEY"] = "<OpenAI_APIのトークン>"
(3) mt-benchのフォルダ (llm_judge) に移動。
%cd fastchat/llm_judge/
(4) 「japanese_mt_bench.zip」を「fastchat/llm_judge/data」に配置。
(5) 「japanese_mt_bench.zip」の解凍。
!unzip data/japanese_mt_bench.zip -d data
4. 質問に対する回答の生成
「gen_model_answer.py」でMT-Benchの質問に対する回答を生成します。
(1) 「gen_model_answer.py」の実行。
「--model-path elyza/ELYZA-japanese-Llama-2-7b-fast-instruct」と「--bench-name japanese_mt_bench」を指定しています。
!python gen_model_answer.py --model-path elyza/ELYZA-japanese-Llama-2-7b-fast-instruct --model-id ELYZA-japanese-Llama-2-7b-fast-instruct --bench-name japanese_mt_bench
回答は「data/japanese_mt_bench/model_answer/ELYZA-japanese-Llama-2-7b-fast-instruct.jsonl」に出力されます。期待通りの回答が確認します。
評価するモデルは、FastChatが対応している必要があります。
5. 回答に対する評価の生成
「gen_judgment.py」で、回答に対するGPT-4による評価を生成します。
(1) 「gen_judgment.py」の実行。
「--model-list ELYZA-japanese-Llama-2-7b-fast-instruct」と「--bench-name japanese_mt_bench」を指定しています。
!python gen_judgment.py --model-list ELYZA-japanese-Llama-2-7b-fast-instruct --parallel 2 --bench-name japanese_mt_bench
Stats:
{
"bench_name": "japanese_mt_bench",
"mode": "single",
"judge": "gpt-4",
"baseline": null,
"model_list": [
"ELYZA-japanese-Llama-2-7b-fast-instruct"
],
"total_num_questions": 80,
"total_num_matches": 160,
"output_path": "data/japanese_mt_bench/model_judgment/gpt-4_single.jsonl"
}
Press Enter to confirm...
(2) 「Press Enter to confirm...」が表示されたら設定を確認してENTER。
評価は「data/japanese_mt_bench/model_judgment/gpt-4_single.jsonl」に出力されます。期待通りの評価内容か確認します。
6. MT-Benchのスコアの表示
「show_result.py」で、MT-Benchのスコアを表示します。
(1) 「show_result.py」を以下のように編集。
「japanese_mt_bench」でマルチターンの評価を行います。
if args.bench_name == "mt_bench":
print("\n########## Second turn ##########")
↓
if args.bench_name == "japanese_mt_bench":
print("\n########## Second turn ##########")
(2) 「show_result.py」の実行。
「--model-list ELYZA-japanese-Llama-2-7b-fast-instruct」と「--bench-name japanese_mt_bench」を指定しています。
!python show_result.py --model-list ELYZA-japanese-Llama-2-7b-fast-instruct --bench-name japanese_mt_bench
Mode: single
Input file: data/japanese_mt_bench/model_judgment/gpt-4_single.jsonl
########## First turn ##########
score
model turn
ELYZA-japanese-Llama-2-7b-fast-instruct 1 5.0625
########## Second turn ##########
score
model turn
ELYZA-japanese-Llama-2-7b-fast-instruct 2 3.275
########## Average ##########
score
model
ELYZA-japanese-Llama-2-7b-fast-instruct 4.16875
7. レーダーチャートのプロット
レーダーチャートをプロットします。
(1) 評価をDataFrameとして読み込み。
import json
import pandas as pd
import plotly.express as px
import plotly.graph_objects as go
CATEGORIES = ["Coding", "Extraction", "Humanities", "Math", "Reasoning", "Roleplay", "STEM", "Writing"]
def get_model_df():
cnt = 0
q2result = []
fin = open("data/japanese_mt_bench/model_judgment/gpt-4_single.jsonl", "r")
for line in fin:
obj = json.loads(line)
obj["category"] = CATEGORIES[(int(obj["question_id"])-1)//10]
q2result.append(obj)
df = pd.DataFrame(q2result)
return df
df = get_model_df()
(2) スコアの計算。
all_models = df["model"].unique()
print(all_models)
scores_all = []
for model in all_models:
for cat in CATEGORIES:
res = df[(df["category"]==cat) & (df["model"]==model) & (df["score"] >= 0)]
score = res["score"].mean()
scores_all.append({"model": model, "category": cat, "score": score})
(3) レーダーチャートの表示。
target_models = ["ELYZA-japanese-Llama-2-7b-fast-instruct"]
scores_target = [scores_all[i] for i in range(len(scores_all)) if scores_all[i]["model"] in target_models]
# sort by target_models
scores_target = sorted(scores_target, key=lambda x: target_models.index(x["model"]), reverse=True)
df_score = pd.DataFrame(scores_target)
df_score = df_score[df_score["model"].isin(target_models)]
rename_map = {"ELYZA-japanese-Llama-2-7b-fast-instruct": "ELYZA-7B",}
for k, v in rename_map.items():
df_score.replace(k, v, inplace=True)
fig = px.line_polar(df_score, r = 'score', theta = 'category', line_close = True, category_orders = {"category": CATEGORIES},
color = 'model', markers=True, color_discrete_sequence=px.colors.qualitative.Pastel)
fig.show()
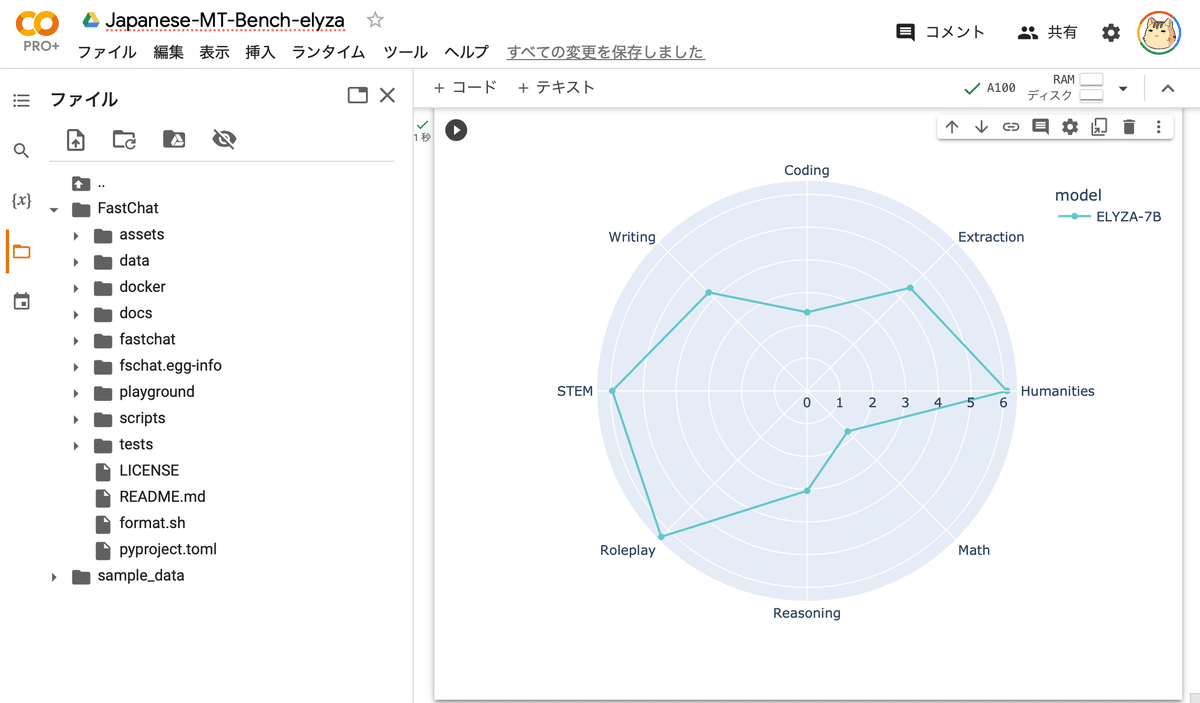
8. 複数のLLMの比較
model-listに複数のモデルIDを指定することで、複数のLLMを比較できます。
・質問に対する回答の生成
!python gen_api_answer.py --model gpt-3.5-turbo --bench-name japanese_mt_bench
・回答に対する評価の生成
!python gen_judgment.py --model-list gpt-3.5-turbo ELYZA-japanese-Llama-2-7b-fast-instruct --bench-name japanese_mt_bench
・MT-Benchのスコアの表示
!python show_result.py --model-list gpt-3.5-turbo ELYZA-japanese-Llama-2-7b-fast-instruct --bench-name japanese_mt_bench
Mode: single
Input file: data/japanese_mt_bench/model_judgment/gpt-4_single.jsonl
########## First turn ##########
score
model turn
gpt-3.5-turbo 1 8.1125
ELYZA-japanese-Llama-2-7b-fast-instruct 1 5.0375
########## Second turn ##########
score
model turn
gpt-3.5-turbo 2 7.5875
ELYZA-japanese-Llama-2-7b-fast-instruct 2 3.2500
########## Average ##########
score
model
gpt-3.5-turbo 7.85000
ELYZA-japanese-Llama-2-7b-fast-instruct 4.14375
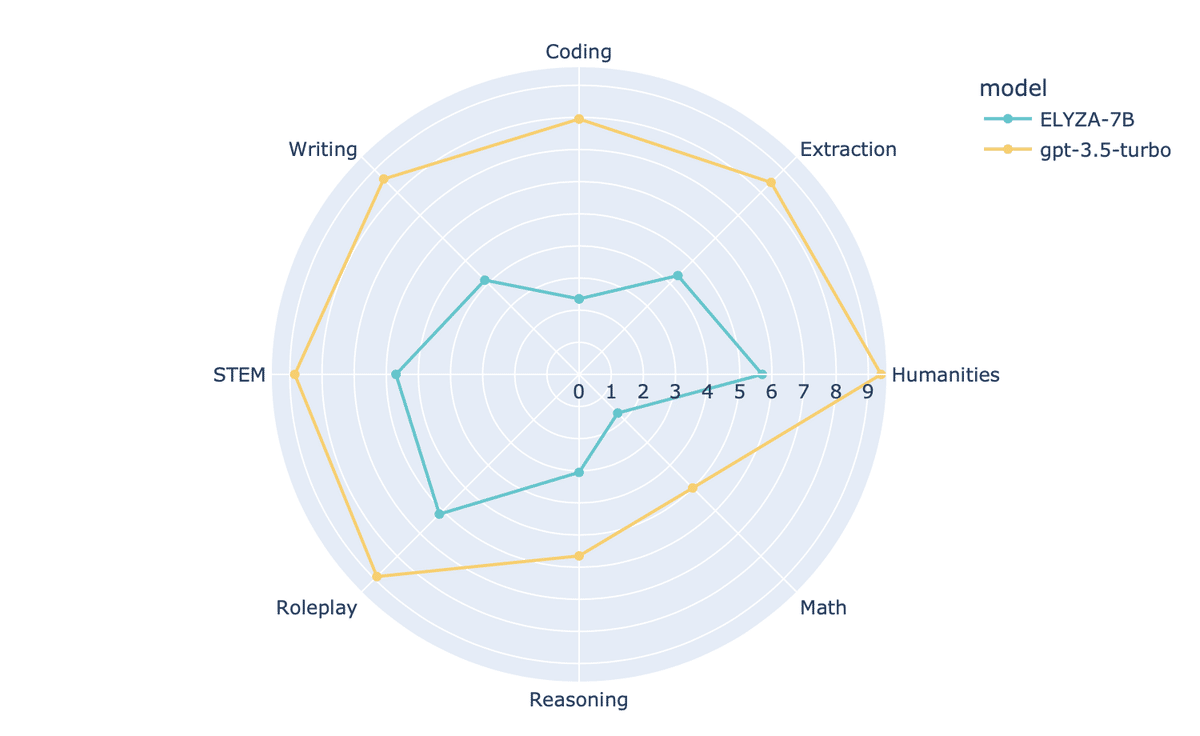
関連
この記事が気に入ったらサポートをしてみませんか?