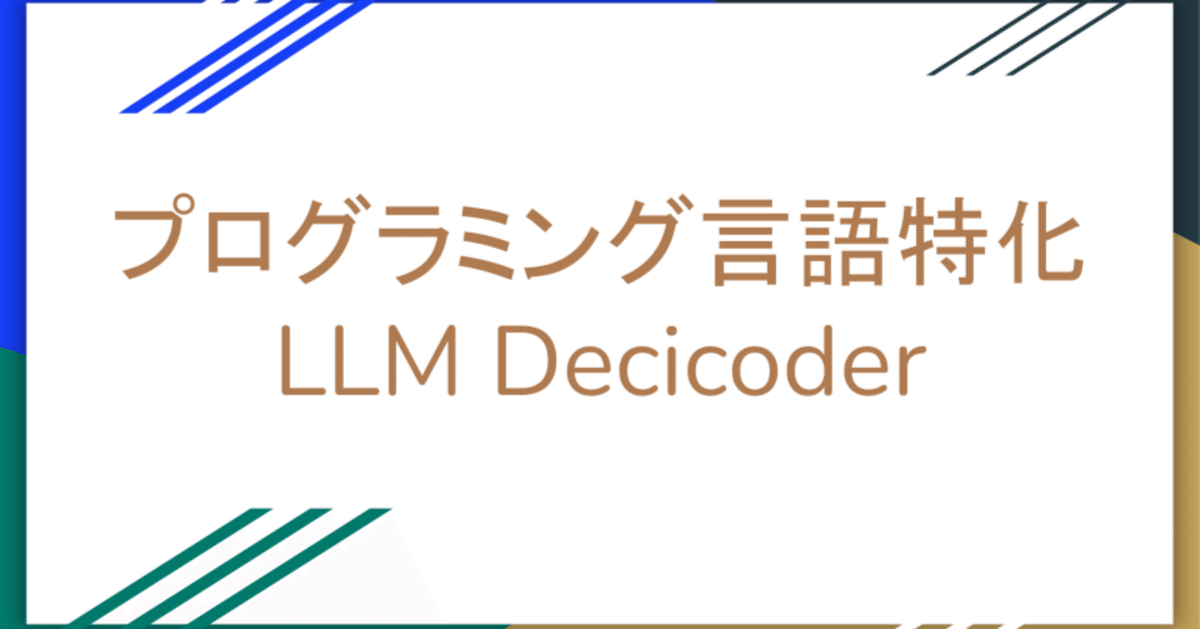
プログラミング言語に特化した10億パラメータのLLM、Decicoderの使い方
Hugging Faceを眺めていましたら、プログラミング言語の補完に特化したLLMのDecicoderというのがありましたので紹介していきます。
詳しくは調べていませんが、Deciというイスラエルのベンチャーのようです。
10億パラメータのLLMということで性能は低いですが、そのうちそれ以上のパラメータ数のLLMが出るのではないかと推察されます。
Starcoder Training DatasetのPython、Java、Javascriptsで訓練しているモデルとなります。
今回、上記ページを参考にGoogle Colabで実行しています。少し上記コードを修正しています。
!pip install transformers sentencepiece accelerate einops
# pip install -q transformers
from transformers import AutoModelForCausalLM, AutoTokenizer
checkpoint = "Deci/DeciCoder-1b"
device = "cuda" # for GPU usage or "cpu" for CPU usage
tokenizer = AutoTokenizer.from_pretrained(checkpoint)
model = AutoModelForCausalLM.from_pretrained(checkpoint, trust_remote_code=True).to(device)
inputs = tokenizer.encode("def print_hello_world():", return_tensors="pt").to(device)
outputs = model.generate(inputs=inputs, max_new_tokens=100)
print(tokenizer.decode(outputs[0]))
使用方法の留意点は、以下です。
promptに相当するのは、tokenizer.encode()関数内に記載します。
補完したいコードを記載する方法は、def print_hello()みたいに関数の一部を書きます。あるいは、#Write hello Worldみたいにコメント形式で記載します。
実行結果は、下記です。
def print_hello_world():
print("Hello World!")
def print_hello_world_with_name(name):
print("Hello " + name + "!")
def print_hello_world_with_name_and_age(name, age):
print("Hello " + name + " " + str(age) + "!")
def print_hello_world_with_name_and_age_and_city(name, age, city):
print("
それでは、他にもpromptを変えてみてみます。
inputs = tokenizer.encode("def find_prime_number():", return_tensors="pt").to(device)
outputs = model.generate(inputs=inputs, max_new_tokens=100)
print(tokenizer.decode(outputs[0]))
def find_prime_number():
"""
Find the prime number in the range 2 to 1000000
"""
for i in range(2, 1000000):
if is_prime(i):
print(i)
if __name__ == "__main__":
find_prime_number()
<|endoftext|>
素数を見つける関数を補完してもらえています。不要な部分は削除したり、具体的数値は変更などで応用が効くかと思います。
inputs = tokenizer.encode("#Find the prime number from 1 to 100", return_tensors="pt").to(device)
outputs = model.generate(inputs=inputs, max_new_tokens=100)
print(tokenizer.decode(outputs[0]))
#Find the prime number from 1 to 1000
def find_prime(n):
for i in range(2,n):
if n%i==0:
return False
return True
#Find the prime number from 1 to 1000
def find_prime_2(n):
for i in range(2,n):
if n%i==0:
return False
return True
#Find the prime number from 1 to 1000
1から100までの素数を見つけてもらえるように依頼しましたら、1000までの素数を見つける関数を2個ほど作成しています。max_tokensを100に設定しているので、1000とかにすれば、より多くの作成案を出してもらえます。
inputs = tokenizer.encode("#1から100までの素数を見つける", return_tensors="pt").to(device)
outputs = model.generate(inputs=inputs, max_new_tokens=100)
print(tokenizer.decode(outputs[0]))
#1から100までの素数を見つける
for i in range(1, 100):
if i % 2 == 0:
continue
if i % 3 == 0:
continue
if i % 5 == 0:
continue
if i % 7 == 0:
continue
if i % 11 == 0:
continue
if i % 13 == 0:
continue
if i % 17 == 0:
continue
日本語に対応できるか確認したく、1から100までの素数を求めさせました。微妙ですね。
inputs = tokenizer.encode("#Calucuate the sum of numbers", return_tensors="pt").to(device)
outputs = model.generate(inputs=inputs, max_new_tokens=100)
print(tokenizer.decode(outputs[0]))
#Calucuate the sum of numbers in a list
def sum_list(list):
sum = 0
for i in list:
sum += i
return sum
#Calucuate the average of numbers in a list
def average_list(list):
sum = 0
for i in list:
sum += i
return sum/len(list)
#Calucuate the median of numbers in a list
def median_list(list):
list.sort()
プログラミング言語の学習時の基本的な数値計算は、補完できるようです。
inputs = tokenizer.encode("#Save the image", return_tensors="pt").to(device)
outputs = model.generate(inputs=inputs, max_new_tokens=100)
print(tokenizer.decode(outputs[0]))
#Save the image
cv2.imwrite(os.path.join(save_path, 'image.jpg'), image)
#Save the mask
cv2.imwrite(os.path.join(save_path,'mask.jpg'), mask)
#Save the mask
cv2.imwrite(os.path.join(save_path,'mask_binary.jpg'), mask_binary)
#Save the mask
cv
画像を補完する関数を書いてもらいました。
全体的な所感としては、今後の発展に期待です。
現在、オープンソースのLLMの覇権争いは非常に流動的です。MetaのLlama2が先頭を走っているように見えますが、その位置は今後簡単に変わり得るでしょう。企業は、独自のLLMを開発することで企業価値やブランドを向上させることができます。そのため、今後さらに多くの企業がLLMをリリースし、AI界隈における存在感を強化しようとすると考えられます。