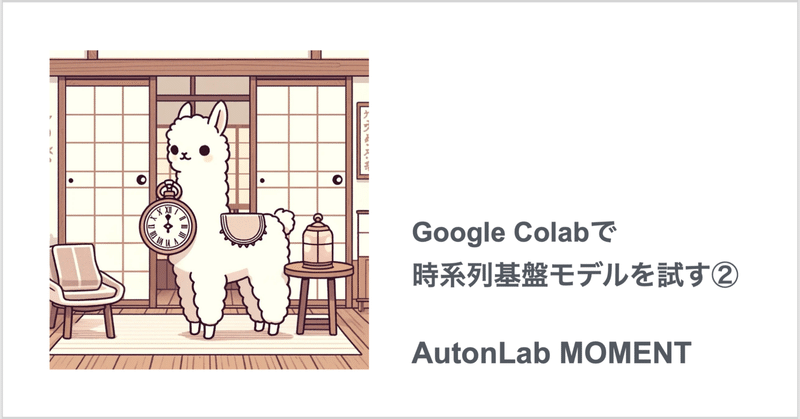
Google Colabで時系列基盤モデルを試す②:AutonLab MOMENT
はじめに
前回のGoogle Timesfmに引き続き、HuggingFaceにある商用可能なライセンスの時系列基盤モデルを4つ試し、比較していきたいと思います。
利用するデータはETTh1という電力変圧器温度に関する多変量時系列データセットです。事前学習にこのデータが含まれる可能性があるため、モデルの絶対的な評価に繋がらないことに注意してください。
ダウンロード数:4.59k
モデルサイズ:200m
ライセンス:Apache-2.0
ダウンロード数:5.79k
モデルサイズ:385m
ライセンス:MIT
ibm-granite/granite-timeseries-ttm-v1
ダウンロード数:10.1k
モデルサイズ:805k (小さい!!)
ライセンス:Apache-2.0
ダウンロード数:256k (多い!!)
モデルサイズ:709m
ライセンス:Apache-2.0
6月2日時点でダウンロード数が少ない順に実施していきます。
今回はAutonLabのMOMENT-1です。AutonLabはカーネギーメロン大学の組織の1つのようです。
MOMENT-1は、Granite, chronos-t5と同様にDecoder-Encoder Architectureです。chronos-t5と同様、言語モデルのT5をベースにしています。
同時に、学習に使用したデータをTimeseries-PILEとして公開しています。
MOMENT-1はそのチュートリアルでETTh1のデータが使用されているので、ETTh1が事前学習で利用されていることはないと思います。
1. 推論
ライブラリの準備
# library install
!pip install git+https://github.com/moment-timeseries-foundation-model/moment.git
データ準備
前回同様ETTh1.csvを使います。取得はこちらから可能です。
import pandas as pd
# データ読み込み
# https://github.com/zhouhaoyi/ETDataset/blob/main/ETT-small/ETTh1.csv
df = pd.read_csv("ETTh1.csv")
print(len(df))
df.head(2)
データは以下のような形式です。OT(Oil Temperature)が目的変数となります。

データ加工
モデルに与える長さを512、予測する長さを96と今回はおきます。Fine Tuningに使うため、後ろから予測するデータをとっておきます。
前回とはモデルに与えるTensorの形が異なることに注意してください。
import torch
context_length = 512
forecast_horizon = 96
# データセット分割
df_train = df.iloc[-(context_length+forecast_horizon):-forecast_horizon]
df_test = df.iloc[-forecast_horizon:]
# 形式の変更
train_tensor = torch.tensor(df_train[["HUFL", "HULL", "MUFL", "MULL", "LUFL", "LULL", "OT"]].values, dtype=torch.float)
train_tensor = train_tensor.t().unsqueeze(0)
test_tensor = torch.tensor(df_test[["HUFL", "HULL", "MUFL", "MULL", "LUFL", "LULL", "OT"]].values, dtype=torch.float)
test_tensor = test_tensor.t().unsqueeze(0)
モデルの取得
task_nameには、実施するタスクに合わせてforecasting(予測)、classification(分類)、reconstruction(異常検出/補完/事前トレーニング)、embedding(埋め込み表現)を指定します。
forecastingタスクの場合、追加で予測するデータ長であるforecast_horizonを指定します。forecast_horizonに関しては特に記載がなかったです。後述しますが、forecast_horizonはどんな値でも良いはずです。ただ、さすがに入力の長さを超えると予測精度も下がることが考えられるのでtimesfmと同様に入力長以下に抑えることが望ましいと思います。
from momentfm import MOMENTPipeline
model = MOMENTPipeline.from_pretrained(
"AutonLab/MOMENT-1-large",
model_kwargs={
'task_name': 'forecasting',
'forecast_horizon': forecast_horizon
},
)
model.init()
model.to("cuda:0")
model pipelineは以下のような構成です。
MOMENTPipeline(
(normalizer): RevIN()
(tokenizer): Patching()
(patch_embedding): PatchEmbedding(
(value_embedding): Linear(in_features=8, out_features=1024, bias=False)
(position_embedding): PositionalEmbedding()
(dropout): Dropout(p=0.1, inplace=False)
)
(encoder): T5Stack(
(embed_tokens): Embedding(32128, 1024)
(block): ModuleList(
(0): T5Block(
(layer): ModuleList(
(0): T5LayerSelfAttention(
(SelfAttention): T5Attention(
(q): Linear(in_features=1024, out_features=1024, bias=False)
(k): Linear(in_features=1024, out_features=1024, bias=False)
(v): Linear(in_features=1024, out_features=1024, bias=False)
(o): Linear(in_features=1024, out_features=1024, bias=False)
(relative_attention_bias): Embedding(32, 16)
)
(layer_norm): T5LayerNorm()
(dropout): Dropout(p=0.1, inplace=False)
)
(1): T5LayerFF(
(DenseReluDense): T5DenseGatedActDense(
(wi_0): Linear(in_features=1024, out_features=2816, bias=False)
(wi_1): Linear(in_features=1024, out_features=2816, bias=False)
(wo): Linear(in_features=2816, out_features=1024, bias=False)
(dropout): Dropout(p=0.1, inplace=False)
(act): NewGELUActivation()
)
(layer_norm): T5LayerNorm()
(dropout): Dropout(p=0.1, inplace=False)
)
)
)
(1-23): 23 x T5Block(
(layer): ModuleList(
(0): T5LayerSelfAttention(
(SelfAttention): T5Attention(
(q): Linear(in_features=1024, out_features=1024, bias=False)
(k): Linear(in_features=1024, out_features=1024, bias=False)
(v): Linear(in_features=1024, out_features=1024, bias=False)
(o): Linear(in_features=1024, out_features=1024, bias=False)
)
(layer_norm): T5LayerNorm()
(dropout): Dropout(p=0.1, inplace=False)
)
(1): T5LayerFF(
(DenseReluDense): T5DenseGatedActDense(
(wi_0): Linear(in_features=1024, out_features=2816, bias=False)
(wi_1): Linear(in_features=1024, out_features=2816, bias=False)
(wo): Linear(in_features=2816, out_features=1024, bias=False)
(dropout): Dropout(p=0.1, inplace=False)
(act): NewGELUActivation()
)
(layer_norm): T5LayerNorm()
(dropout): Dropout(p=0.1, inplace=False)
)
)
)
)
(final_layer_norm): T5LayerNorm()
(dropout): Dropout(p=0.1, inplace=False)
)
(head): ForecastingHead(
(flatten): Flatten(start_dim=-2, end_dim=-1)
(dropout): Dropout(p=0.1, inplace=False)
(linear): Linear(in_features=65536, out_features=96, bias=True)
)
)
forecast_horizonとして96を指定したのでout_features=96のForecastingHeadがheadとしてついています。
実は、事前学習時にはreconstruction用のヘッドで学習されており、forecastingとclassification用のヘッドは新たにランダムに初期化される形となります。なのでforecast_horizonはどの値でも良さそうと考えました。
また、このためforecastingとclassificationタスクはFineTuningを行わないと意味がありませんが一応ランダムに初期化されていることを確認するために推論も実施してみようと思います。
推論
import torch
# 推論
forecast = model(train_tensor.to("cuda:0"))
forecast_tensor = forecast.forecast.cpu()
推論結果の出力
予測結果としてOT(Oil Temperature)がみたいのでchannel_idxとして6を指定します。
import matplotlib.pyplot as plt
channel_idx = 6
time_index = 0
history = train_tensor[time_index, channel_idx, :].detach().numpy()
true = test_tensor[time_index, channel_idx, :].detach().numpy()
pred = forecast_tensor[time_index, channel_idx, :].detach().numpy()
plt.figure(figsize=(12, 4))
# Plotting the first time series from history
plt.plot(range(len(history)), history, label='History (512 timesteps)', c='darkblue')
# Plotting ground truth and prediction
num_forecasts = len(true)
offset = len(history)
plt.plot(range(offset, offset + len(true)), true, label='Ground Truth (96 timesteps)', color='darkblue', linestyle='--', alpha=0.5)
plt.plot(range(offset, offset + len(pred)), pred, label='Forecast (96 timesteps)', color='red', linestyle='--')
plt.title(f"ETTh1 (Hourly) -- (idx={time_index}, channel={channel_idx})", fontsize=18)
plt.xlabel('Time', fontsize=14)
plt.ylabel('Value', fontsize=14)
plt.legend(fontsize=14)
plt.show()
結果は以下のようになります。Headがランダムに初期化されているので予測はされません。
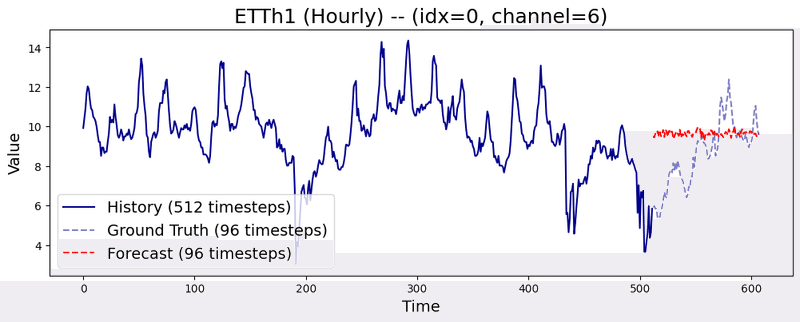
2. Fine Tuning
Headのみを学習していくことになります。
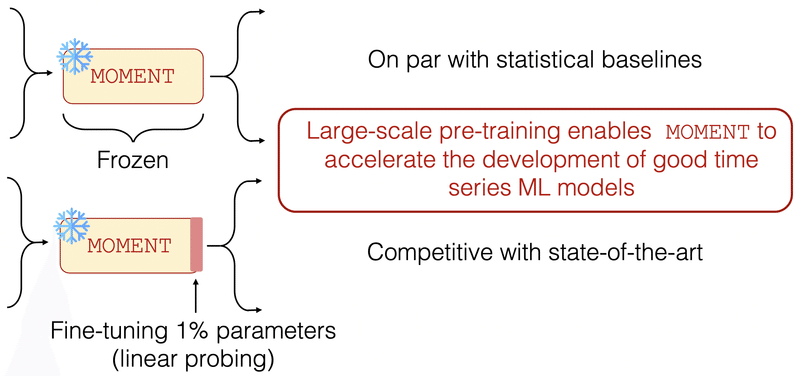
Data Loaderの準備
公式のチュートリアルでは事前に用意されたデータを呼び出すためのInformerDatasetというクラスを使っていますが、自前で用意したデータでFineTuningできるように書き換えています。
また、df_testの最後のcontext_length+forecast_horizon分は最後の推論用に取り除いておきます。
import numpy as np
import torch
import torch.cuda.amp
from torch.utils.data import DataLoader, Dataset
from torch.optim.lr_scheduler import OneCycleLR
from tqdm import tqdm
from momentfm.utils.utils import control_randomness
from momentfm.utils.forecasting_metrics import get_forecasting_metrics
class ETThDataset(Dataset):
def __init__(self, data, random_seed=42, forecast_horizon=192, seq_len=512):
self.data = data
self.random_seed = random_seed
self.forecast_horizon = forecast_horizon
self.seq_len = seq_len
def __len__(self):
return len(self.data) - self.seq_len - self.forecast_horizon
def __getitem__(self, idx):
# ランダムシードを設定
torch.manual_seed(self.random_seed)
# シーケンスの開始位置をランダムに選択
start_idx = torch.randint(0, len(self.data) - self.seq_len - self.forecast_horizon, (1,)).item()
# 入力シーケンスを取得
x = torch.tensor(self.data.iloc[start_idx:start_idx+self.seq_len, :].values, dtype=torch.float32)
x = x.t()
# 目標シーケンスを取得
y = torch.tensor(self.data.iloc[start_idx+self.seq_len:start_idx+self.seq_len+self.forecast_horizon, -1].values, dtype=torch.float32)
y = y.unsqueeze(0)
# input_mask
input_mask = np.ones(self.seq_len)
return x, y, input_mask
# ETTh1.csvを読み込む
df = pd.read_csv("ETTh1.csv")
# 日時の除去
df = df.drop(columns=["date"])
# データを前8割をトレーニング用、後ろ2割をテスト用に分割
train_size = int(0.8 * len(df))
print(train_size)
df_train = df.iloc[:train_size]
df_test = df.iloc[train_size:-(context_length+forecast_horizon)]
# ETTDatasetを作成
train_dataset = ETThDataset(df_train, forecast_horizon=96)
test_dataset = ETThDataset(df_test, forecast_horizon=96)
# DataLoaderの定義
batch_size = 64
train_loader = DataLoader(train_dataset, batch_size=batch_size, shuffle=True)
test_loader = DataLoader(test_dataset, batch_size=batch_size, shuffle=True)
諸々の設定
今後のGraniteやchronos-t5と条件を揃えるため、epochは1とします。
# lossやoptim、scheduler等諸々定義
criterion = torch.nn.MSELoss()
optimizer = torch.optim.Adam(model.parameters(), lr=1e-4)
device = torch.device("cuda" if torch.cuda.is_available() else "cpu")
cur_epoch = 0
max_epoch = 1
# Move the model to the GPU
model = model.to(device)
# Move the loss function to the GPU
criterion = criterion.to(device)
# Enable mixed precision training
scaler = torch.cuda.amp.GradScaler()
# Create a OneCycleLR scheduler
max_lr = 1e-4
total_steps = len(train_loader) * max_epoch
scheduler = OneCycleLR(optimizer, max_lr=max_lr, total_steps=total_steps, pct_start=0.3)
# Gradient clipping value
max_norm = 5.0
Fine Tuning実施
Google ColabのT4で7分ほどかかりました。
# FineTuning開始
while cur_epoch < max_epoch:
losses = []
for timeseries, forecast, input_mask in tqdm(train_loader, total=len(train_loader)):
# Move the data to the GPU
timeseries = timeseries.float().to(device)
input_mask = input_mask.to(device)
forecast = forecast.float().to(device)
with torch.cuda.amp.autocast():
output = model(timeseries, input_mask)
loss = criterion(output.forecast, forecast)
# Scales the loss for mixed precision training
scaler.scale(loss).backward()
# Clip gradients
scaler.unscale_(optimizer)
torch.nn.utils.clip_grad_norm_(model.parameters(), max_norm)
scaler.step(optimizer)
scaler.update()
optimizer.zero_grad(set_to_none=True)
losses.append(loss.item())
losses = np.array(losses)
average_loss = np.average(losses)
print(f"Epoch {cur_epoch}: Train loss: {average_loss:.3f}")
# Step the learning rate scheduler
scheduler.step()
cur_epoch += 1
# Evaluate the model on the test split
trues, preds, histories, losses = [], [], [], []
model.eval()
with torch.no_grad():
for timeseries, forecast, input_mask in tqdm(test_loader, total=len(test_loader)):
# Move the data to the GPU
timeseries = timeseries.float().to(device)
input_mask = input_mask.to(device)
forecast = forecast.float().to(device)
with torch.cuda.amp.autocast():
output = model(timeseries, input_mask)
loss = criterion(output.forecast, forecast)
losses.append(loss.item())
trues.append(forecast.detach().cpu().numpy())
preds.append(output.forecast.detach().cpu().numpy())
histories.append(timeseries.detach().cpu().numpy())
losses = np.array(losses)
average_loss = np.average(losses)
model.train()
trues = np.concatenate(trues, axis=0)
preds = np.concatenate(preds, axis=0)
histories = np.concatenate(histories, axis=0)
metrics = get_forecasting_metrics(y=trues, y_hat=preds, reduction='mean')
print(f"Epoch {cur_epoch}: Test MSE: {metrics.mse:.3f} | Test MAE: {metrics.mae:.3f}")
3. 推論(Fine Tuning後)
最後に、先に試したのと同じ区間で推論を再度実施してみます。
データ準備
import torch
context_length = 512
forecast_horizon = 96
# データセット分割
df_train = df.iloc[-(context_length+forecast_horizon):-forecast_horizon]
df_test = df.iloc[-forecast_horizon:]
# 形式の変更
train_tensor = torch.tensor(df_train[["HUFL", "HULL", "MUFL", "MULL", "LUFL", "LULL", "OT"]].values, dtype=torch.float)
train_tensor = train_tensor.t().unsqueeze(0)
test_tensor = torch.tensor(df_test[["HUFL", "HULL", "MUFL", "MULL", "LUFL", "LULL", "OT"]].values, dtype=torch.float)
test_tensor = test_tensor.t().unsqueeze(0)
推論
import torch
# 推論
model.eval()
forecast = model(train_tensor.to("cuda:0"))
forecast_tensor = forecast.forecast.cpu()
推論結果の出力
import matplotlib.pyplot as plt
channel_idx = 6
time_index = 0
history = train_tensor[time_index, channel_idx, :].detach().numpy()
true = test_tensor[time_index, channel_idx, :].detach().numpy()
pred = forecast_tensor[time_index, channel_idx, :].detach().numpy()
plt.figure(figsize=(12, 4))
# Plotting the first time series from history
plt.plot(range(len(history)), history, label='History (512 timesteps)', c='darkblue')
# Plotting ground truth and prediction
num_forecasts = len(true)
offset = len(history)
plt.plot(range(offset, offset + len(true)), true, label='Ground Truth (96 timesteps)', color='darkblue', linestyle='--', alpha=0.5)
plt.plot(range(offset, offset + len(pred)), pred, label='Forecast (96 timesteps)', color='red', linestyle='--')
plt.title(f"ETTh1 (Hourly) -- (idx={time_index}, channel={channel_idx})", fontsize=18)
plt.xlabel('Time', fontsize=14)
plt.ylabel('Value', fontsize=14)
plt.legend(fontsize=14)
plt.show()
結果は以下のようになりました。
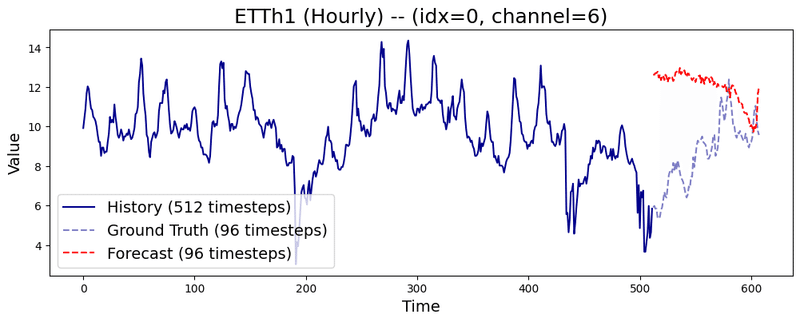
4. 結果
結果としては、学習はしたような気はするものの学習が足りてないような予測となりました。(ほとんど公式のチュートリアルから持ってきたものの、コードに誤りがあればご連絡いただきたいです。)
FTの結果は期待通りとはいかなかったもののHeadを付け替えて予測や分類、Embedding、異常検知と幅広いタスクに対応できるのが特徴的でtimesfmにはなかった点だと思います。
時系列のEmbeddingというのは今まで考えたことがなかったので、今度どこかで試してみたいと思います。
次回
参照
この記事が気に入ったらサポートをしてみませんか?