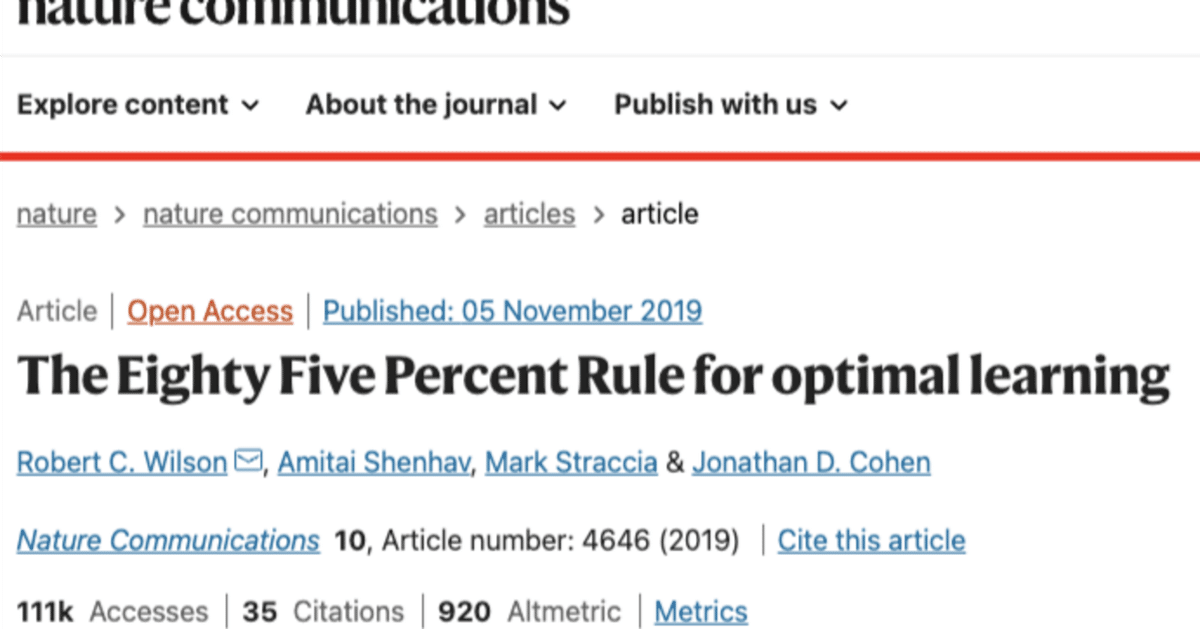
最適な学習のための85%ルール
https://doi.org/10.1038/s41467-019-12552-4
リハビリテーションや理学療法分野において85%の設定を具体的にどう考えるか?は課題だと思いますが,これまでの難易度設定に対する考え方を修正することも視野に入るなと思った論文.
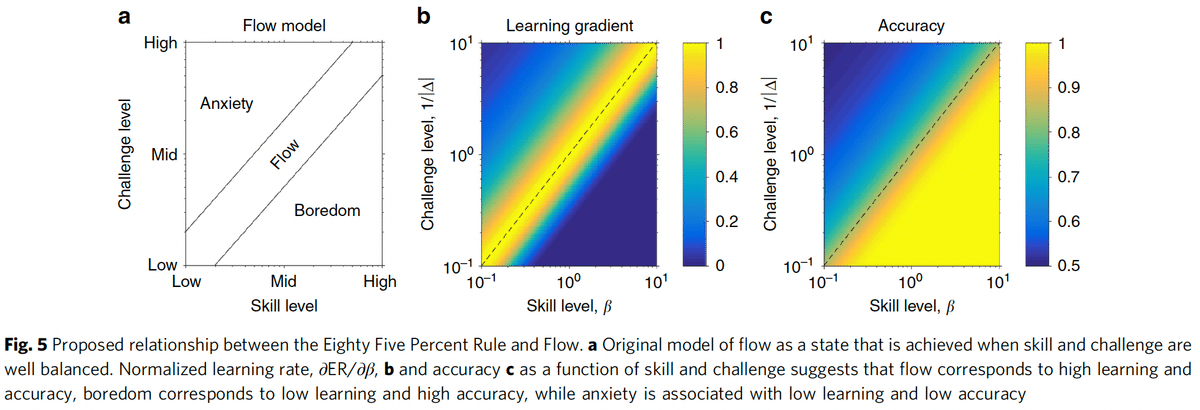
Abstract
Researchers and educators have long wrestled with the question of how best to teach their clients be they humans, non-human animals or machines. Here, we examine the role of a single variable, the difficulty of training, on the rate of learning. In many situations we find that there is a sweet spot in which training is neither too easy nor too hard, and where learning progresses most quickly. We derive conditions for this sweet spot for a broad class of learning algorithms in the context of binary classification tasks. For all of these stochastic gradient-descent based learning algorithms, we find that the optimal error rate for training is around 15.87% or, conversely, that the optimal training accuracy is about 85%. We demonstrate the efficacy of this ‘Eighty Five Percent Rule’ for artificial neural networks used in AI and biologically plausible neural networks thought to describe animal learning.
要旨
研究者や教育者は、人間、人間以外の動物、機械など、クライアントにどのように教えるのがベストなのかという問題に長い間取り組んできた。ここでは、トレーニングの難易度という一つの変数が学習率に果たす役割を検証する。多くの状況において、トレーニングが簡単すぎず難しすぎず、学習が最も早く進むスイートスポットが存在することがわかった。このスイートスポットの条件を、二値分類タスクの文脈における幅広いクラスの学習アルゴリズムについて導出する。これら全ての確率的勾配降下ベースの学習アルゴリズムにおいて、最適な学習エラー率は約15.87%であり、逆に最適な学習精度は約85%であることが分かる。この「85%ルール」の有効性を、AIに用いられる人工ニューラルネットワークと、動物の学習を表現すると考えられる生物学的に妥当なニューラルネットワークに対して実証する。
この記事が気に入ったらサポートをしてみませんか?