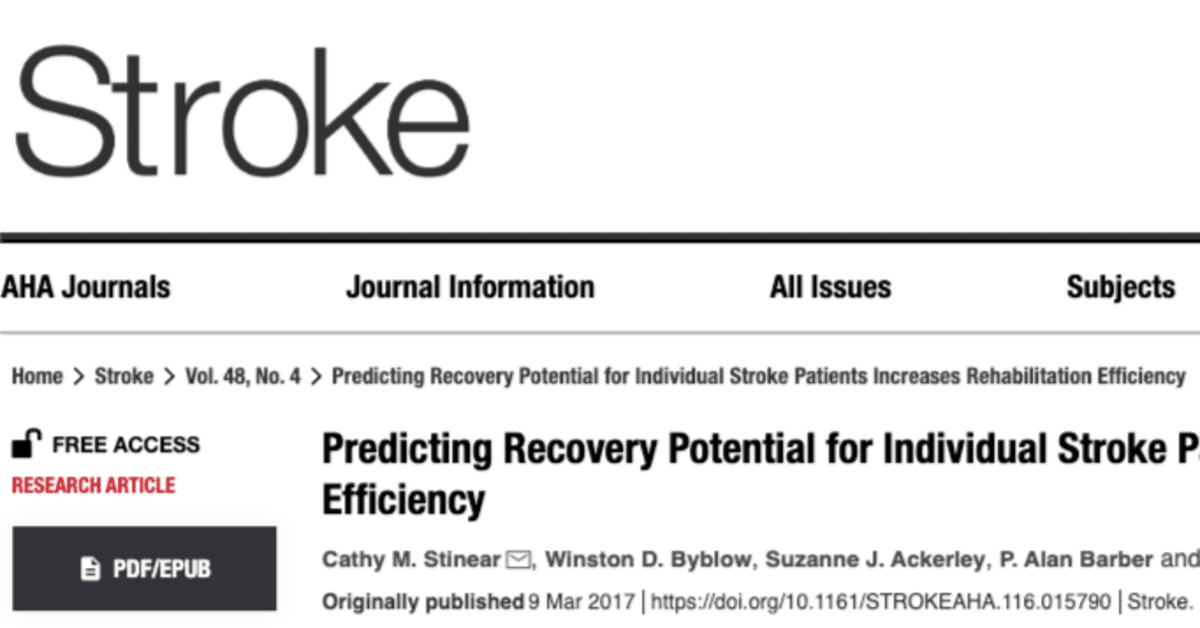
脳卒中患者の回復可能性を予測することで、リハビリテーションの効率は向上する
DOI: 10.1161/STROKEAHA.116.015790.
論文中で使用されている,Predict Recovery Potential (PREP) algorithmの論文は,DOI: 10.1016/S1474-4422(10)70247-7.
Keywords: length of stay; prognosis; stroke; upper extremity.
Abstract
Background and purpose: Several clinical measures and biomarkers are associated with motor recovery after stroke, but none are used to guide rehabilitation for individual patients. The objective of this study was to evaluate the implementation of upper limb predictions in stroke rehabilitation, by combining clinical measures and biomarkers using the Predict Recovery Potential (PREP) algorithm.
背景と目的:脳卒中後の運動機能回復にはいくつかの臨床指標やバイオマーカーが関連しているが、個々の患者のリハビリテーションの指針として用いられているものはない。本研究の目的は、PREP(Predict Recovery Potential)アルゴリズムを用い、臨床指標とバイオマーカーを組み合わせることにより、脳卒中リハビリテーションにおける上肢予測の実施を評価することである。
Methods: Predictions were provided for patients in the implementation group (n=110) and withheld from the comparison group (n=82). Predictions guided rehabilitation therapy focus for patients in the implementation group. The effects of predictive information on clinical practice (length of stay, therapist confidence, therapy content, and dose) were evaluated. Clinical outcomes (upper limb function, impairment and use, independence, and quality of life) were measured 3 and 6 months poststroke. The primary clinical practice outcome was inpatient length of stay. The primary clinical outcome was Action Research Arm Test score 3 months poststroke.
方法 予測は実施群(n=110)には提供され、比較群(n=82)には提供されなかった。予測は実施群の患者のリハビリテーション治療の焦点の指針となった。予測情報が臨床実践(入院期間、セラピストの自信、治療内容、投与量)に及ぼす影響を評価した。臨床転帰(上肢機能、障害、使用、自立、QOL)は、脳卒中後3ヵ月と6ヵ月に測定された。主要臨床アウトカムは入院期間であった。主要臨床転帰は、脳卒中後3ヵ月のAction Research Arm Testスコアであった。
Results: Length of stay was 1 week shorter for the implementation group (11 days; 95% confidence interval, 9-13 days) than the comparison group (17 days; 95% confidence interval, 14-21 days; P=0.001), controlling for upper limb impairment, age, sex, and comorbidities. Therapists were more confident (P=0.004) and modified therapy content according to predictions for the implementation group (P<0.05). The algorithm correctly predicted the primary clinical outcome for 80% of patients in both groups. There were no adverse effects of algorithm implementation on patient outcomes at 3 or 6 months poststroke.
結果 入院期間は、上肢障害、年齢、性別、合併症を考慮すると、実施群(11日;95%信頼区間、9~13日)は比較群(17日;95%信頼区間、14~21日;P=0.001)より1週間短かった。セラピストはより自信を持ち(P=0.004)、実施群の予測に従って治療内容を修正した(P<0.05)。アルゴリズムは、両群とも80%の患者の主要臨床転帰を正しく予測した。脳卒中後3ヵ月および6ヵ月の時点での患者の転帰に、アルゴリズムの導入による悪影響はみられなかった。
Conclusions: PREP algorithm predictions modify therapy content and increase rehabilitation efficiency after stroke without compromising clinical outcome.
結論 PREPアルゴリズムによる予測は、臨床転帰を損なうことなく、脳卒中後の治療内容を修正し、リハビリテーションの効率を高める。
この記事が気に入ったらサポートをしてみませんか?