CTranslate2でrinna instructionをquantizeして動かす
これまでquantizeはllama.cppを使っていたが、GPUを使えなかったのでCTranslate2を使ってみた。
CTranslate2はtransfomer modelのメモリを削減、高速化を行うライブラリ
CTranslate2 is a C++ and Python library for efficient inference with Transformer models.
C++で実装されており、pythonからC++を呼び出している。document通りinstallすると、GPUも利用してくれる。
いろんなモデルが対応している
Decoder-only models: GPT-2, GPT-J, GPT-NeoX, OPT, BLOOM, MPT, LLaMa, CodeGen, GPTBigCode, Falcon
install-and-usage
install
pip install ctranslate2
pip install sentencepiece transformers
記事を書いているときのバージョン
ctranslate2 3.15.1
sentencepiece 0.1.99
transformers 4.30.1
使い方
translator = ctranslate2.Translator(translation_model_path)
translator.translate_batch(tokens)
generator = ctranslate2.Generator(generation_model_path)
generator.generate_batch(start_tokens)
https://github.com/OpenNMT/CTranslate2#installation-and-usage
cuda 12はサポートしていないので注意
https://github.com/OpenNMT/CTranslate2/issues/1250
モデルの変換
サポートしているquantizeは以下
int8
int8_float16
int16
float16
https://opennmt.net/CTranslate2/quantization.html
int8_float16指定でfloat16でモデルをロード、quantizeするのでvram少ないときはこれが良さそう
https://github.com/OpenNMT/CTranslate2/blob/master/python/ctranslate2/converters/transformers.py#L1572
以下で変換
model="rinna/japanese-gpt-neox-3.6b-instruction-sft"
output_dir="/rinnna"
type="int8_float16"
ct2-transformers-converter --model model$ --output_dir $output_dir --type $type
model.binが生成される
cd $output_dir
ls
config.json
model.bin
vocabulary.txt
textの生成
modelのロード
model=output_dir
generator = ctranslate2.Generator(model, device="auto")
tokenizer = transformers.AutoTokenizer.from_pretrained("rinna/japanese-gpt-neox-3.6b-instruction-sft", use_fast=False)
生成にはgenerator classのメソッドを使う
(documentのexampleには、add_special_tokens=Falseがついてないのでつけるのを忘れないようにする。)
tokens = tokenizer.convert_ids_to_tokens(tokenizer.encode(prompt, add_special_tokens=False))
results = generator.generate_batch(
[tokens],
max_length=512,
sampling_topk=10,
sampling_temperature=0.7,
include_prompt_in_result=True,
beam_size=4,
end_token=[tokenizer.eos_token_id, tokenizer.pad_token_id],
return_end_token=True,
repetition_penalty=1.5
)
text = tokenizer.decode(results[0].sequences_ids[0])
print(text)
generateの引数は以下を参照
引数いくつかpickup
beam_size
beam_size=1でgreedy search
include_prompt_in_result
長い入力のパフォーマンスを上げるにはFalseを設定するのが良い
Falseの場合、promptをdecoderの内部状態の初期化に使われる
Trueの場合、promptが結果に含まれるように生成を制約
固定で与えるprefixは、static_promptとして与えることができ、cacheしてくれる
static_prompt – If the model expects a static prompt (a.k.a. system prompt) it can be set here to simplify the inputs and optionally cache the model state for this prompt to accelerate future generations.
cache_static_prompt – Cache the model state after the static prompt and reuse it for future generations using the same static prompt.
実際に生成してみる
環境はcolab T4 ハイメモリ
> ユーザー: 日本のおすすめの観光地を教えてください。<NL>システム: どの地域の観光地が知りたいですか?<NL>ユーザー: 渋谷の観光地を教えてください。<NL>
システム: ああ、わかりました!</s>
> ユーザー: 日本のおすすめの観光地を教えてください。<NL>システム: どの地域の観光地が知りたいですか?<NL>ユーザー: 渋谷の観光地を教えてください。<NL>
システム:わかりました、まずは「ハチ公像」から始めましょう!</s>
生成も問題なさそう
生成に使うリソースは、RAM2.3GB、GPU3.8GB
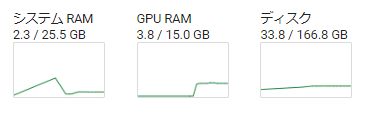
所感
3Bモデルというのもあるが、だいぶ早い
GPUもRAMも抑えられてて良い感じ
promptを長くするとPADしか生成されないことがあった
おそらく原因はこれかなぁ…(issueではT5の既存バグらしい)
https://github.com/OpenNMT/CTranslate2/issues/1074
sentenseのembedding用のmethodはなさそう
generatorのforward_batchでlogitが出力できるので、一旦これでembeddingしてみる