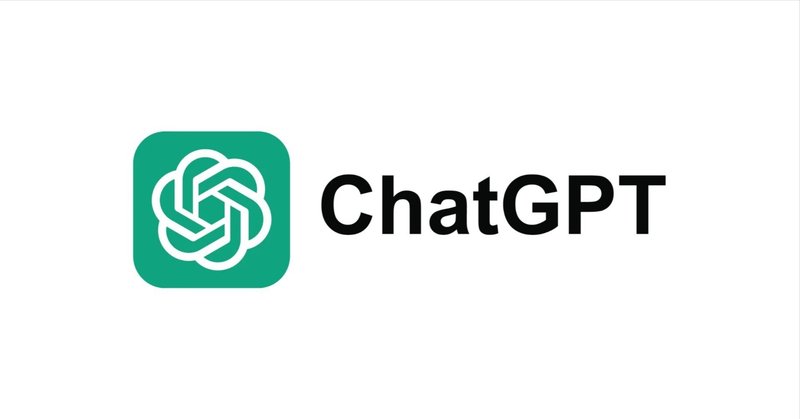
【ChatGPT】英語スピーチを日本語で読む【2023年4月21日|@TED】
ChatGPTについてのスピーチです。
公開日:2023年4月21日
※動画を再生してから、インタビューを読むのがオススメです。
We started OpenAI seven years ago because we felt like something really interesting was happening in AI, and we wanted to help steer it in a positive direction.
私たちが7年前にOpenAIを始めたのは、AIで何かとても面白いことが起こっていると感じ、それをポジティブな方向に導く手助けをしたいと思ったからです。
It's honestly just really amazing to see how far this whole field has come since then.
その後、この分野全体がどれほど進歩したかを見ると、正直言って本当に驚きます。
And it's really gratifying to hear from people like Raymond, who are using the technology we are building and others, for so many wonderful things.
そして、レイモンドのように、私たちが作っている技術や他の技術を使って、多くの素晴らしいことを行っている人たちから話を聞けるのは、本当にありがたいことです。
We hear from people who are using the technology for so many wonderful things.
私たちは、この技術を非常に多くの素晴らしいことに使っている人たちから話を聞きます。
We hear from people who are excited.
私たちは、興奮している人たちから話を聞きます。
We hear from people who are concerned.
また、心配する声も聞きます。
We hear from people who feel both those emotions at once.
その両方の感情を同時に感じている人たちから話を聞くことができます。
And honestly, that's how we feel.
そして、正直なところ、私たちもそう思っています。
Above all, it feels like we're entering an historic period right now, where we as a world are going to define a technology that will be so important for our society going forward.
何より、私たちは今、歴史的な時期に突入していると感じています。この先、私たちの社会にとって非常に重要な技術を、私たち世界が定義しようとしているのです。
And I believe that we can manage this for good.
そして、これを良い方向にマネジメントしていくことができると信じています。
So today, I want to show you the current state of that technology and some of the underlying design principles that we hold dear.
そこで今日は、その技術の現状と、私たちが大切にしている基本的な設計原理を紹介したいと思います。
So the first thing I'm going to show you is what it's like to build a tool for an AI rather than building it for a human.
まず最初にお見せするのは、人間のためにツールを作るのではなく、AIのためにツールを作るというのはどういうことなのかということです。
So we have a new Dolly model, which generates images, and we are exposing it as an app for ChatGPT to use on your behalf.
そこで、画像を生成する新しいDollyというモデルを用意し、それをChatGPTのアプリとして公開し、あなたの代わりに使ってもらうことにしました。
And you can do things like ask, suggest a nice post-Ted meal and draw a picture of it.
そして、テッド後の食事を提案したり、その写真を描いたりすることができるのです。
Now, you get all of the sort of ideation and creative back and forth and taking care of the details for you that you get out of ChatGPT.
このように、ChatGPTを利用することで、アイデア出しやクリエイティブなやり取り、細部への配慮を受けることができます。
And here we go.
そして、ここからが本題です。
It's not just the idea for the meal, but a very, very detailed spread.
食事のアイデアだけでなく、非常に詳細な見開きがあります。
So let's see what we're going to get.
では、どんなものが出てくるか見てみましょう。
But ChatGPT doesn't just generate images in this case.
でも、ChatGPTはこの場合、画像を生成するだけではありません。
It doesn't just generate text, it also generates an image.
テキストを生成するだけでなく、画像も生成してくれるのです。
And that is something that really expands the power of what it can do on your behalf in terms of carrying out your intent.
これは、あなたの意図を実現するために、あなたの代わりにできることの幅を広げてくれるものです。
And I'll point out, this is all a live demo.
なお、これはすべてライブデモです。
This is all generated by the AI as we speak, so I actually don't even know what we're going to see.
これはすべてAIが生成したものなので、実際に何が出てくるかはわかりません。
This looks wonderful.
これは素晴らしいですね。
Now I'm getting hungry just looking at it.
見ているだけでお腹が空いてきました。
Now, we've extended ChatGPT with other tools, too.
さて、ChatGPTは他のツールでも拡張しています。
For example, memory, you can say save this for later.
例えば、メモリは、これを後で保存しておくと言うことができます。
And the interesting thing about these tools is they're very inspectable.
これらのツールの面白いところは、とても検査がしやすいことです。
So you get this little pop-up here that says use the Dolly app.
Dollyアプリを使うという小さなポップアップが表示されます。
And by the way, this is coming to all ChatGPT users over upcoming months.
ちなみに、このアプリはChatGPTの全ユーザーに今後数ヶ月で提供されます。
And you can look under the hood and see that what it actually did was write a prompt.
このアプリの中身を見ると、実際にプロンプトを書くことができることがわかります。
Just like a human could.
人間が書くのと同じように。
And so you sort of have this ability to inspect how the machine is using these tools, which allows us to provide feedback to them.
このように、機械がどのようにツールを使っているかを検査することで、機械にフィードバックを与えることができるのです。
Now it's saved for later, and let me show you what it's like to use that information and to integrate with other applications, too.
その情報を使って、他のアプリケーションと統合することもできます。
You can say, I now make a shopping list for the tasty thing I was suggesting earlier.
先ほど提案した美味しいものの買い物リストを今作りました、ということができます。
I'm going to make it a little tricky for the AI.
AIにちょっと手際よく作ってあげる。
And tweet it out for all the TED viewers out there.
そして、それをTEDの視聴者のためにツイートするのです。
So if you do make this wonderful, wonderful meal, I definitely want to know how it tastes.
もし、あなたがこの素晴らしい素晴らしい料理を作ったら、その味をぜひ知りたいですからね。
But you can see that ChatGPT is selecting all these different tools without me having to tell it explicitly which ones to use in any situation.
しかし、ChatGPTは、私がどのような状況でどのようなツールを使うかを明確に指示しなくても、さまざまなツールを選択していることがわかると思います。
And this, I think, shows a new way of thinking about the user interface.
これは、ユーザーインターフェースに対する新しい考え方を示していると思います。
Like, we are so used to thinking of, well, we have these apps, we click between them, we copy and paste between them, and usually it's a great experience within an app as long as you kind of know the menus and know all the options.
私たちは、アプリを使って、クリックして切り替えたり、コピー&ペーストしたりするのに慣れていますが、メニューやオプションを理解していれば、アプリ内での経験は素晴らしいものになります。
Yes, I would like you to.
そうですね、そうしてほしいです。
Yes, please.
はい、お願いします。
Always good to be polite.
常に丁寧であることが大切です。
And by having this unified language interface on top of tools, the AI is able to sort of take away all those details from you so you don't have to be the one who spells out every single sort of little piece of what's supposed to happen.
ツールの上に統一された言語インターフェースを持つことで、AIはすべての詳細を取り除き、何が起こるべきかというすべての一部を明文に指定する必要がないようにしてくれます。
And as I said, this is a live demo, so sometimes the unexpected will happen to us.
これはライブデモなので、時には予期せぬことが起こるかもしれません。
But let's take a look at the Instacart shopping list while we're at it.
しかし、Instacartの買い物リストを見てみましょう。
And you can see we sent a list of ingredients to Instacart.
Instacartに食材のリストを送ったのがお分かりいただけると思います。
Here's everything you need.
これが必要なものすべてです。
And the thing that's really interesting is that the traditional UI is still very valuable.
そして、本当に興味深いのは、従来のUIがまだ非常に貴重であるということです。
If you look at this, you still can click through it and sort of modify the actual quantities.
これを見ると、クリックすることで実際の数量を変更することができます。
And that's something that I think shows that they're not going away, traditional UIs.
これは、従来のUIがなくならないことを示すものだと思います。
It's just we have a new, augmented way to build them.
ただ、新しい拡張された方法で構築しているのです。
And now we have a tweet that's been drafted for our review, which is also a very important thing.
そして、私たちのレビューのために下書きされたツイートがあります。これは、非常に重要なことです。
We can click Run, and there we are.
実行をクリックすれば、もう大丈夫です。
We're the manager, we're able to inspect, we're able to change the work of the AI if we want to.
私たちは管理者であり、検査することができ、必要であればAIの作業を変更することができるのです。
And so, after this talk, you will be able to access this yourself.
そして、この講演の後、あなた自身がこれにアクセスできるようになるのです。
And there we go.
そして、そこに行くのです。
Cool.
かっこいいですね。
So, thank you, everyone.
では、みなさん、ありがとうございました。
So we'll cut back to the slides.
では、スライドに戻りましょう。
Now, the important thing about how we build this, it's not just about building these tools.
さて、このツールの作り方で重要なのは、単にツールを作るということではありません。
It's about teaching the AI how to use them.
AIにその使い方を教えることです。
Like, what do we even want it to do when we ask these very high-level questions?
例えば、非常に高度な質問をしたときに、AIに何をさせたいか。
And to do this, we use an old idea.
そのために、私たちはある古いアイデアを使います。
If you go back to Alan Turing's 1950 paper on the Turing test, he says, look, you'll never program an answer to this.
アラン・チューリングが1950年に発表したチューリングテストに関する論文にさかのぼると、彼はこう言っています。
Instead, you can learn it.
その代わり、学習することができます。
You could build a machine like a human child and then teach it through feedback.
人間の子供のようなマシンを作り、フィードバックによって教えることができるのです。
Have a human teacher who provides rewards and punishments as it tries things out and does things that are either good or bad.
人間の先生が、マシンがいろいろなことを試して、良いこと、悪いことをしたときに、報酬と罰を与えるのです。
And this is exactly how we train ChatGPT.
これが、ChatGPTのトレーニング方法です。
It's a two-step process.
2つのステップを踏んでいきます。
First, we produce what Turing would have called a child machine through an unsupervised learning process.
まず、教師なし学習によって、チューリングが「子機」と呼んだであろうものを作り出します。
We just show it, the whole world, the whole internet, and say, predict what comes next in text you've never seen before.
全世界、つまりインターネット全体に、「見たこともないようなテキストで、次に来るものを予測しなさい」と見せるのです。
And this process imbues it with all sorts of wonderful skills.
そして、このプロセスによって、子機にはさまざまな素晴らしい能力が備わるのです。
For example, if you're shown a math problem, the only way to actually complete that math problem, say what comes next, that green nine up there, is to actually solve the math problem.
例えば、数学の問題を見せられたとき、その数学の問題を実際に完成させ、次に来るもの、つまり、あそこにある緑の9を言うには、実際に数学の問題を解くしかありません。
But we actually have to do a second step, too, which is to teach the AI what to do with those skills.
しかし、実は第二段階として、AIにそのスキルを使って何をすべきかを教える必要があります。
And for this, we provide feedback.
そのために、私たちはフィードバックを行っています。
We have the AI try out multiple things, give us multiple suggestions, and then the human rates them, says, this one's better than that one.
AIに複数のことを試してもらい、複数の提案をしてもらい、それを人間が評価して「こっちの方がいい」と言うのです。
And this reinforces not just the specific thing that the AI said but, very importantly, the whole process that the AI used to produce that answer.
そうすることで、AIが言った特定の事柄だけでなく、AIがその答えを出すために使ったプロセス全体が強化されるのです。
And this allows it to generalize, it allows it to teach, to sort of infer your intent and apply it in scenarios that it hasn't seen before, that it hasn't received feedback.
これにより、AIは一般化し、学習することができ、あなたの意図を推測して、これまでに見たことのないシナリオやフィードバックを受けたことのないシナリオに適用することができるようになるのです。
Now, sometimes the things we have to teach the AI are not what you'd expect.
AIに教えなければならないことは、予想外のことであることもあります。
For example, when we first showed GPT-4 to Khan Academy, they said, wow, this is so great, we're going to be able to teach students wonderful things.
例えば、GPT-4をカーン・アカデミーに初めて見せたとき、彼らは「これは素晴らしい、学生に素晴らしいことを教えることができる」と言いました。
Only one problem, it doesn't double-check students' math.
ただ1つ問題があって、生徒の計算をダブルチェックしないんです。
If there's some bad math in there, it will happily pretend that one plus one equals three and run with it.
もし悪い計算があれば、1+1=3ということにして、それを実行してしまうのです。
So we had to collect some feedback data.
そこで、私たちはフィードバックデータを収集する必要がありました。
Sal Khan himself was very kind and offered 20 hours of his own time to provide feedback to the machine alongside our team.
サル・カーンはとても親切で、20時間もの時間を割いて、私たちのチームと一緒に機械にフィードバックしてくれました。
And over the course of a couple months, we were able to teach the AI that, hey, you really should push back on humans in this specific kind of scenario.
そして、数カ月かけて、AIに「このようなシナリオでは、人間に背中を押すべきだ」と教えることができました。
And we've actually made lots and lots of improvements to the models this way, and when you push that thumbs down in ChatGPT, that actually is kind of like sending up a bat signal to our team to say, here's an area of weakness where you should gather feedback.
実際には、このような方法でモデルの多くの改善を行ってきましたし、ChatGPTで「👎」を押すと、弱点がある箇所でフィードバックを収集するようにチームにバットシグナルを送るようなものです。
And so when you do that, that's one way that we really listen to our users and make sure we're building something that's more useful for everyone.
そうすることで、ユーザーの声に耳を傾け、誰にとってもより便利なものを作り上げることができるのです。
Now, providing high-quality feedback is a hard thing.
さて、質の高いフィードバックを提供するのは難しいことです。
If you think about asking a kid to clean their room, if all you're doing is inspecting the floor, you don't know if you're just teaching them to stuff all the toys in the closet.
子供に部屋を掃除するよう頼むとき、床を点検するだけでは、おもちゃを全部クローゼットに詰め込むように教えているのかどうかわかりません。
This is a nice dolly-generated image, by the way.
ちなみに、これはドリーで生成された素敵な画像です。
And the same sort of reasoning applies to AI.
そして、同じような理屈がAIにも当てはまります。
As we move to harder tasks, we will have to scale our ability to provide high-quality feedback.
より難しいタスクに移行するにつれ、質の高いフィードバックを提供する能力をスケールアップする必要があります。
But for this, the AI itself is happy to help.
しかし、その際、AIは喜んで助けてくれます。
It's happy to help us provide even better feedback and to scale our ability to supervise the machine as time goes on.
私たちがより良いフィードバックを提供し、時間が経つにつれてマシンを監督する能力を拡大することができるのです。
And let me show you what I mean.
そして、私が何を言いたいのか、お見せしましょう。
For example, you can ask for a GPT-4 question like this, of how much time passed between these two foundational logs on supervised learning and learning from human feedback, and the model says, two months passed.
例えば、GPT-4の質問で「教師あり学習と人間からのフィードバックから学習するこれら2つの基本的なログの間にどれくらいの時間が経過したか」と尋ねると、モデルは「2か月が経過した」と答えます。
But is it true?
しかし、それは本当なのでしょうか?
These models are not 100 percent reliable, although they're getting better every time we provide some feedback.
このモデルは100%信頼できるわけではありません。私たちが何らかのフィードバックをするたびに、より良いものになっていきますが。
But we can actually use the AI to fact-check, and it can actually check its own work.
しかし、私たちは実際にAIを使ってファクトチェックをすることができますし、AIは実際に自分の仕事をチェックすることができます。
You can say, fact-check this for me.
私のためにこれをファクトチェックしなさい」と言うことができます。
Now, in this case, I've actually given the AI a new tool.
さて、今回のケースでは、実はAIに新しいツールを与えています。
This one is a browsing tool, where the model can issue search queries and click into web pages.
これはブラウジングツールで、検索クエリを発行し、ウェブページをクリックすることができます。
And it actually writes out its whole chain of thought as it does it.
その際、AIは思考の連鎖をすべて書き出しています。
It says, I'm going to search for this, and it actually does the search.
私はこれを検索します」と言うと、実際に検索を行います。
It then finds the publication date and the search results.
そして、出版日や検索結果を見つける。
It then is issuing another search query.
その後、別の検索クエリを発行する。
It's going to click into the blog post.
ブログの記事をクリックする。
And all of this you could do, but it's a very tedious task.
そして、これらすべてを行うことができますが、非常に面倒な作業です。
It's not a thing that humans really want to do.
人間が本当にやりたいと思うようなことではありません。
It's much more fun to be in the driver's seat, to be in this manager's position, where you can, if you want, triple-check the work.
それよりも、運転席に座って、マネージャーのような立場で、必要であればトリプルチェックができるほうがずっと楽しいです。
And out come citations, so you can actually go and very easily verify any piece of this whole chain of reasoning, and it actually turns out two months was wrong, two months in one week.
そして、引用文献も出てくるので、実際に行って、この一連の推論のどの部分でも非常に簡単に検証することができ、実際に2カ月は間違っていた、1週間で2カ月だったということが判明します。
That was correct.
それが正しかったのです。
We'll cut back to the slide.
スライドに戻りましょう。
The thing that's so interesting to me about this whole process is that it's this many-step collaboration between a human and an AI, because a human, using this fact-checking tool, is doing it in order to produce data for another AI to become more useful to a human.
このプロセスが非常に興味深いと感じるのは、人間とAIの多段階の協力が行われているからで、事実を検証するツールを使っている人間は、他のAIが人間にとってより有益になるためのデータを生成する目的でこれを行っています。
And I think this really shows the shape of something that we should expect to be much more common in the future, where we have humans and machines very carefully and delicately designed in how they fit into a problem and how we want to solve that problem.
これは、今後ますます一般的になるであろう、人間と機械が問題にどのように取り組むかや、それをどのように解決するかということを慎重かつ繊細に設計された形で示していると思います。
We make sure that the humans are providing the management, the oversight, the feedback, and that the machines are operating in a way that's inspectable and trustworthy.
人間が管理、監督、フィードバックを行い、機械が検査可能で信頼できる方法で動作していることを確認します。
And together, we're able to actually create even more trustworthy machines.
そうすることで、より信頼性の高いマシンを作ることができるのです。
And I think that over time, if we get this process right, we will be able to solve impossible problems.
そして、このプロセスがうまくいけば、不可能な問題も解決できるようになると考えています。
And to give you a sense of just how impossible I'm talking, I think we're going to be able to rethink almost every aspect of how we interact with computers.
どのくらい不可能かというと、コンピュータとの付き合い方のほとんどすべての面を見直すことができるようになると思うのです。
For example, think about spreadsheets.
例えば、表計算ソフトについて考えてみましょう。
They've been around in some forums since, let's say, 40 years ago with VisiCalc.
40年前のVisiCalc以来、いくつかのフォーラムで使用されてきました。
And I don't think they've really changed that much in that time.
そして、その間にそれほど大きな変化があったとは思えません。
And here is a specific spreadsheet of all the AI papers on the archive for the past 30 years.
そして、ここに過去30年間のアーカイブにあるすべてのAI論文の具体的なスプレッドシートがあります。
There's about 167,000 of them, and you can see the data right here.
約167,000件あり、ここでデータを見ることができます。
But let me show you the ChatGPT take on how to analyze a data set like this.
しかし、このようなデータセットをどのように分析するのか、ChatGPTのやり方をお見せしましょう。
So we can give ChatGPT access to yet another tool.
そこで、ChatGPTに別のツールにアクセスさせます。
This one, a Python interpreter, so it's able to run code just like a data scientist would.
これはPythonインタープリターで、データサイエンティストと同じようにコードを実行することができます。
And so you can just literally upload a file and ask questions about it, and very helpfully.
文字通り、ファイルをアップロードして、それについて質問することができますし、とても便利です。
It knows the name of the file, and it's like, oh, this is CSV, comma-separated value file.
ファイル名がわかるので、「これはCSV(カンマ区切り値ファイル)です。
I'll parse it for you.
解析してあげましょう。
The only information here is the name of the file, the column names, like you saw, and then the actual data.
ここにある情報は、ファイル名、列名、そして実際のデータだけです。
And from that, it's able to infer what these columns actually mean.
そこから、これらの列が実際に何を意味しているのかを推測することができます。
Like, that semantic information wasn't in there.
つまり、意味的な情報は入っていないのです。
It has to sort of put together its world knowledge of knowing that, oh, yeah, archive is the site that people submit papers, and therefore, that's what these things are, and that these are integer values, and so therefore, it's the number of authors in the paper.
AIは、アーカイブが論文を提出するサイトであり、これらのものがそれであり、これらが整数値であるため、論文の著者の数であるというような世界の知識をまとめなければなりません。
All of that, that's work for a human to do, and the AI is happy to help with it.
全部、人間がやる仕事なんだけど、AIが喜んで手伝ってくれる。
I don't even know what I want to ask, so fortunately, you can ask the machine, can you make some exploratory graphs?
何を聞きたいのかも分からないので、幸いなことに、機械に、探索的なグラフを作れないか、と聞くことができる。
And once again, this is a super high-level instruction with lots of intent behind it, but I don't even know what I want, and the AI kind of has to infer what I might be interested in.
しかし、私は自分が何をしたいのかさえ知らないので、AIは私が何に興味があるのかを推測する必要があります。
And so it comes up with some good ideas, I think.
そのため、AIは私が何に興味があるのかを推測する必要があります。
So a histogram of the number of authors per paper, time series of papers per year, word cloud of the paper titles.
論文ごとの著者数のヒストグラム、年ごとの論文の時系列、論文タイトルのワードクラウドなどです。
All of that, I think, will be pretty interesting to see.
これらすべてを見ると、かなり興味深いものになると思います。
The interesting thing is, it can actually do it.
面白いのは、実際にそれができることです。
Here we go, a nice bell curve.
素晴らしいベルカーブをご覧ください。
You see that three is kind of the most common.
3が一番多いことがわかりますね。
It's going to then write a...
そして、これを書くと...。
It's going to make this nice plot of the papers per year.
年ごとの論文を見事にプロットしてくれるんです。
Something crazy is happening in 2023, though.
しかし、2023年には何かおかしなことが起こっています。
Looks like we were on an exponential, and it dropped off a cliff.
指数関数的に推移していたのが、崖から落ちたようなんです。
What could be going on there?
何が起こっているのでしょうか?
And by the way, all this is Python code you can expect.
ちなみに、これはすべてPythonのコードです、ご期待ください。
And then we'll see the word cloud, so you can see all these wonderful things that appear in these titles.
そしてワードクラウドを見ると、これらのタイトルに登場する素晴らしいものばかりが目に飛び込んできます。
But I'm pretty unhappy about this 2023 thing.
でも、この2023年というのは、かなり不満です。
It makes this year look really bad.
今年が本当に悪く見えてしまう。
Of course, the problem is that the year's not over.
もちろん、問題は今年が終わっていないことです。
So I'm going to push back on the machine.
だから、マシンの背中を押してあげようと思っています。
So April 13th was the cutoff date, I believe.
それで、4月13日が締切日だったと思う。
Can you use that?
それを使っていいのか?
Make a fair projection.
公平な予測を立てる。
So we'll see, this is a kind of ambitious one.
というわけで、これはちょっと野心的なものなんですが、どうでしょう。
So, you know, again, I feel like there was more I wanted out of the machine here.
それで、もう一度言いますが、この機械に求めるものがもっとあったような気がするんです。
I really wanted it to notice this thing.
本当にこのことに気づいてほしかったんです。
Maybe it's a little bit of an overreach for it to have sort of inferred magically that this is what I wanted.
私が望んでいたのはこれだ、と魔法にかけられたようなものだとしたら、それは少し行き過ぎかもしれません。
But I inject my intent.
しかし、私は自分の意図を注入したのです。
I provide this additional piece of sort of guidance.
このようなガイダンスのようなものを追加で提供するのです。
And under the hood, the AI is working on it.
そして、その下で、AIが作業をしているのです。
The AI is just writing code again, so if you want to inspect what it's doing, it's very possible.
AIはまたコードを書いているだけなので、AIが何をしているのか調べようと思えば、それはとても可能なことなのです。
And now, it does the correct projection.
そして今、正しい投影をしています。
If you notice, it even updates the title.
気付くと、タイトルまで更新されています。
I didn't ask for that, but it knows what I want.
私が頼んだわけではないのですが、私が何を望んでいるのかがわかっているのです。
Now, we'll cut back to the slide again.
では、再びスライドに戻ります。
This slide shows a parable.
このスライドはたとえ話です。
A vision of how we may end up using this technology in the future.
将来、この技術をどのように使うことになるのか、そのビジョンを示しています。
A person brought his very sick dog to the vet, and the veterinarian made a bad call to say, let's just wait and see.
ある人が重病の犬を獣医に連れてきたところ、獣医は「様子を見ましょう」と誤った判断をしました。
And the dog would not be here today had he listened.
しかし、獣医師は「様子を見ましょう」という判断を下し、その犬は今ここにいない。
In the meanwhile, he provided the blood test, like the full medical records, to GPT-4, which said, I am not a vet, you need to talk to a professional.
一方、彼は、全医療記録のような血液検査をGPT-4に提供し、私は獣医ではありません、プロに相談する必要がありますと言った。
Here are some hypotheses.
ここにいくつかの仮説があります。
He brought that information to the vet, who used it to save the dog's life.
GPT-4はその情報を獣医に提供し、獣医はその情報をもとに犬の命を救いました。
Now, these systems, they're not perfect.
さて、こうしたシステムは、完璧ではありません。
You cannot overly rely on them.
過度に依存することはできません。
But this story, I think, shows that the human with a medical professional and with ChatGPT as a brainstorming partner was able to achieve an outcome that would not have happened otherwise.
しかし、この物語は、医療従事者とChatGPTというブレインストーミングのパートナーを得た人間が、そうでなければ起きなかったであろう結果を達成できたということを示しているのだと思います。
I think this is something we should all reflect on and think about as we consider how to integrate these systems into our world.
このことは、私たちがこのようなシステムをどのように私たちの世界に組み込んでいくかを考える上で、反省すべき点であり、考えるべき点であると思います。
And one thing that's really interesting is that there's a lot of work to be done to make this work work.
そして、本当に興味深いのは、この仕事を成功させるためにやるべきことがたくさんあることです。
And one thing I believe really deeply is that getting AI right is going to require participation from everyone.
そして、私が本当に深く信じていることのひとつは、AIを正しく使うには、すべての人の参加が必要だということです。
And that's for deciding how we want it to slot in, that's for setting the rules of the road for what an AI will and won't do.
それは、AIをどのように組み込むかを決めることであり、AIが何をするのか、何をしないのかというルールを決めることです。
And if there's one thing to take away from this talk, it's that this technology just looks different, just different from anything people had anticipated.
この講演から得られるものがあるとすれば、それは、このテクノロジーは、人々が予想していたものとは異なるものであるということです。
And so we all have to become literate, and that's honestly one of the reasons we released ChatGPT.
そのため、私たちは皆、リテラシーを身につけなければなりません。
It's about how we can achieve the OpenAI mission of ensuring that artificial general intelligence benefits all of humanity.
人工知能が全人類に利益をもたらすようにするというOpenAIのミッションを達成するためにはどうすればいいかということです。
Thank you.
ありがとうございます。
I mean, I suspect that within every mind out here, apart from there's a feeling of reeling.
つまり、ここにいるすべての人の心の中に、動揺があるのは別として、あるのではないかと思うのです。
Like, I suspect that a very large number of people viewing this, you look at that and you think, "Oh my goodness, pretty much every single thing about the way I work, I need to rethink."
これを見た多くの人が、"あら、私の仕事のやり方は、ほとんどすべて見直す必要がある "と思ったのではないでしょうか。
There's just new possibilities there.
そこには、新しい可能性があるのです。
I mean, right, who thinks that they're having to rethink the way that we do things?
つまり、自分の仕事のやり方を見直す必要があると考える人はいないでしょう?
Yeah, I mean, it's amazing, but it's also really scary.
ええ、素晴らしいことですが、同時にとても怖いことでもあります。
So let's talk, Greg, let's talk.
だから、話をしよう、グレッグ、話をしよう。
Absolutely.
もちろんです。
I mean, I guess my first question actually is just how the hell have you done this?
最初の質問は、一体どうやってこれを成し遂げたのか、ということです。
OpenAI has a few hundred employees.
OpenAIの従業員数は数百人です。
Google has thousands of employees working on artificial intelligence.
Googleは何千人もの従業員が人工知能に取り組んでいます。
Why is it you who's come up with this technology that's shocked the world?
なぜ、世界に衝撃を与えたこの技術を生み出したのは、あなたなのでしょうか?
The truth is we're all building on shoulders of giants, right?
実は、私たちは皆、巨人の肩の上に乗っているんですね。
There's no question if you look at the compute progress, the algorithmic progress, the data progress, all of those are really industry-wide.
計算機の進歩、アルゴリズムの進歩、データの進歩、これらすべてが業界全体の進歩であることは間違いありません。
But I think within OpenAI, we made a lot of very deliberate choices from the early days.
しかし、OpenAIでは、初期の段階から、非常に意図的な選択をたくさんしてきたと思います。
I think it was just to confront reality as it lays, and that we just sort of thought really hard about, what is it going to take to make progress here?
現実を直視し、「ここで進歩するためには何が必要なのか」を真剣に考えたのだと思います。
We tried a lot of things that didn't work, so you only see the things that did.
多くのことを試してもうまくいかなかったので、うまくいったものだけを見ることができます。
And I think that the most important thing has been to get teams of people who are very different from each other to work together harmoniously.
そして、最も重要なことは、互いにまったく異なる人たちのチームが調和して仕事をすることだと思います。
Can we have the water, by the way, just brought here?
ところで、お水はここに持ってきてもらえますか?
I think we're going to need it.
必要だと思うのですが。
It's a dry amount of topic.
ドライな話題の量ですね。
But isn't there something also, just about the fact that you saw something in these language models that meant that if you continue to invest in them and grow them, that something at some point might emerge?
でも、この言語モデルに何かを見出したということは、このまま投資して育てていけば、いつかは何かが生まれるかもしれないということではないのでしょうか。
Yes.
そうですね。
And I think that...
そして、それは...
I mean, honestly, I think the story there is pretty illustrative, right?
正直なところ、この話はとても分かりやすいと思います。
I think that at a high level, deep learning, we always knew that was what we wanted to be, was a deep learning lab.
ディープラーニングは、高いレベルで、私たちはディープラーニングラボになりたいと考えていました。
And exactly how to do it, I think that in the early days, we didn't know.
そして、それをどのように行うかについては、初期の頃はわからなかったと思います。
But one person was working on training a model to predict the next character in Amazon reviews, and he got a result where this is a syntactic process.
ただ、ある人がアマゾンのレビューで次の文字を予測するモデルのトレーニングに取り組んでいて、これは構文処理だという結果が出たんです。
You expect the model will predict where the commas go, where the nouns and verbs are, but he actually got a state-of-the-art sentiment analysis classifier out of it.
このモデルは、カンマがどこにあるか、名詞と動詞がどこにあるかを予測すると思っていたのですが、実際には最先端の感情分析分類器を手に入れたのです。
This model could tell you if a review was positive or negative.
このモデルは、レビューが肯定的か否定的かを判断することができました。
And today, we are just like, come on, anyone could do that.
そして今日、私たちは「そんなこと、誰でもできるだろう」と思っています。
But this was the first time that you saw this emergence, this sort of semantics that emerged from this underlying syntactic process.
しかし、このように構文の基礎となるプロセスから意味論が生まれたのは、この時が初めてでした。
And there we knew, you've got to scale this thing, you've got to see where it goes.
そして、これをスケールアップして、その先を見なければならないことがわかったのです。
So I think this helps explain the riddle that baffles everyone looking at this, because these things are described as prediction machines, and yet what we're seeing out of them feels...
というのも、これらは予測機械として説明されていますが、実際に見てみると...。
it just feels impossible that that could come from a prediction machine, just the stuff you showed us just now.
予測機械から生まれることは不可能だと感じています。
And the key idea of emergence is that when you get more of a thing, suddenly different things emerge, it happens all the time.
創発の重要な考え方は、あるものをより多く手に入れると、突然、異なるものが現れるというものです。
Ant colonies, single ants run around.
アリのコロニーでは、1匹のアリが走り回っています。
When you bring enough of them together, you get these ant colonies that show completely emergent and different behavior.
アリのコロニーでは、1匹のアリが走り回っていますが、それをたくさん集めると、完全に創発的で異なる行動をとるアリのコロニーができあがります。
Or a city, where a few houses together is just houses together, but as you grow the number of houses, things emerge, like suburbs and cultural centers and traffic jams.
また、都市では、数軒の家が集まっただけではただの家ですが、家の数が増えるにつれて、郊外や文化センター、交通渋滞など、さまざまなものが出現してきます。
Give me one moment for you, when you saw just something pop that just blew your mind, that you just did not see coming.
あなたにとって、予想もしなかった何かが出現した瞬間があれば教えてください。
Yeah, well, so if you can try this in ChatGPT, if you add 40-digit numbers, 40-digit numbers, the model will do it, which means it's really learned a internal circuit for how to do it.
ChatGPTで試してみてください。40桁の数字を足すと、モデルはそれを実行します。つまり、モデルはそれを実行するための内部回路を学習したことになります。
And the really interesting thing is actually if you have an ad, like a 40-digit number plus a 35-digit number, it'll often get it wrong.
面白いのは、40桁の数字と35桁の数字を足したような広告があると、よく間違うことです。
And so you can see that it's really learning the process, but it hasn't fully generalized, right?
つまり、プロセスを学習しているのですが、まだ完全に一般化されていないのです。
It's like you can't memorize the 40-digit addition table.
40桁の足し算の表が覚えられないのと同じです。
That's more add-ins than there are in the universe.
それは宇宙の数よりも多い足し算の数です。
You can learn something general, but it hasn't really fully yet learned that, oh, I can generalize this to adding arbitrary numbers of arbitrary lengths.
一般的なことは学べますが、「これは任意の長さの任意の数の足し算に一般化できる」ということは、まだ完全には学んでいないのです。
So what's happened here is that you've allowed it to scale up and look at an incredible number of pieces of text, and it is learning things that you didn't know that it was going to be capable of learning.
つまり、スケールアップして信じられないほどの数のテキストを見ることができるようになったわけですが、その結果、学習能力があるとは思ってもみなかったようなことを学習しているのです。
Yeah, and it's more nuanced, too, because one science that we're starting to really get good at is predicting some of these emerging capabilities.
私たちが得意とし始めた科学のひとつは、こうした新しい能力を予測することです。
And to do that, actually, one of the things that I think is very undersung in this field is sort of engineering quality.
そのためには、この分野では工学的な品質が非常に重要視されていないように思います。
We had to rebuild our entire stack and get...
私たちはスタック全体を再構築し、...
When you think about building a rocket, every tolerance has to be incredibly tiny.
ロケットを作ることを考えると、すべての公差は信じられないほど小さいものでなければなりません。
Same is true in machine learning.
機械学習でも同じことが言えます。
You have to get every single piece of the stack engineered properly, and then you can start doing these predictions.
機械学習でも同じで、スタックの一つひとつを適切に設計し、それから予測を始めるのです。
There are all these incredibly smooth scaling curves that tell you something deeply fundamental about intelligence.
驚くほど滑らかなスケーリングカーブは、知能の根本的な何かを物語っています。
If you look at our GPT-4 blog post, you can see all these curves in there.
GPT-4のブログ記事を見ていただければ、これらの曲線をすべて見ることができます。
And now we're starting to be able to predict.
そして今、私たちは予測できるようになりつつあります。
This is all the performance on coding problems, from we basically look at some models that are 10,000 times or 1,000 times smaller.
これはコーディング問題での性能で、基本的には1万倍や1,000倍小さいモデルを見ているのです。
And so there's something about this that is actually smooth scaling, even though it stole early days.
このように、初期の段階ではありますが、実際にスムーズなスケーリングができるようになっています。
So here is one of the big fears, then, that arises from this.
そこで、ここから生じる大きな恐れの1つがあります。
If it's fundamental to what's happening here, that as you scale up, things emerge that you can maybe predict in some level of confidence, but it's capable of surprising you.
スケールアップすると、ある程度は予測できても、驚くようなことが起こるというのが、ここで起こっていることの基本だとしたら。
Why isn't there just a huge risk of something truly terrible emerging?
なぜ、本当に恐ろしいものが出現する危険性がないのでしょうか?
Well, I think all these are questions of degree and scale and timing.
これらはすべて、程度や規模、タイミングの問題だと思います。
And I think one thing people miss, too, is sort of the integration with the world.
そして、人々が見逃していることのひとつに、世界との融合があります。
There's also this incredibly emergent, very powerful thing, too.
また、信じられないような創発的な、非常に強力なものもあります。
That's one of the reasons we think it's so important to deploy incrementally.
これが、段階的に展開することが重要だと考える理由の1つです。
What we see right now, if you look at this talk, a lot of what I focus on is providing really high-quality feedback.
今、この講演を見ると、私が重視しているのは、本当に質の高いフィードバックを提供することです。
Today, the tasks that we do, you can inspect them, right?
今日、私たちが行ったタスクは、検査することができますよね?
It's very easy to look at that math problem and be like, no, no, no, machine seven was the correct answer.
数学の問題を見て、「いやいや、マシン7が正解だった」と思うのはとても簡単です。
But even summarizing a book, that's a hard thing to supervise.
でも、本の要約だって、監督するのは大変なことです。
How do you know if this book summary is any good?
この本の要約が良いものかどうか、どうやって判断するのですか?
You have to read the whole book.
本全体を読むしかない。
No one wants to do that.
そんなことは誰もやりたくない。
And so I think that the important thing will be that we take this step by step and that we say, OK, as we move on to book summaries, we have to supervise this task properly.
ですから、重要なのは、一歩一歩進めていくこと、そして、本の要約に移行する際には、この作業をきちんと監督する必要がある、ということだと思います。
We have to build up a track record with these machines that they're able to actually carry out our intent.
そして、この機械が私たちの意図することを実際に実行できるという実績を積み上げていかなければなりません。
And I think we're going to have to produce even better, more efficient, more reliable ways of scaling this, sort of like making the machine be aligned with you.
そして、より優れた、より効率的な、より信頼性の高いスケーリング方法、つまり、マシンを自分に合わせるような方法を生み出さなければならないと思います。
So we're going to hear, later in the session, there are critics who say that there's no real understanding inside the system.
セッションの後半で、「システム内部には本当の理解がない」という批判的な意見を聞くことになります。
Is it going to always?
いつもそうなのでしょうか?
We're never going to know that it's not generating errors, that it doesn't have common sense and so forth.
エラーが発生しないとか、常識がないとか、そういうことを知ることはできないんです。
Is it your belief, Greg, that that is true at any one moment, but that the expansion of the scale and the human feedback that you talked about is basically going to take it on that journey of actually getting to things like truth and wisdom and so forth with a high degree of confidence?
グレッグさんの考えでは、そのようなことが一時的には真実であり、スケールの拡大と人間からのフィードバックが、真実や知恵などに高い自信度で到達するプロセスを促進することになるのでしょうか?
Can you be sure of that?
それは確かなことですか?
Yeah, well, I think that the OpenAI...
ええ、まあ、OpenAIは...。
I mean, the short answer is yes, I believe that is where we're headed.
つまり、短い答えとしては、「はい」です。
And I think that the OpenAI approach here has always been you've got to push to the limits of this technology to really see it in action, because that tells you then, oh, here's how we can move on to a new paradigm.
OpenAIのアプローチは、この技術の限界を押し広げることで実際に機能する方法を見つけ出すことで、新しいパラダイムに移行する方法を見つけることができると考えています。
And we just haven't exhausted the fruit here.
その結果、新しいパラダイムに移行する方法が見えてくるのです。
I mean, it's quite a controversial stance you've taken that the right way to do it is to push to the limits of this technology to really see it in action.
つまり、この技術の限界に挑戦し、実際に使ってみることが正しい方法であるという、あなたのスタンスは非常に物議を醸していますね。
And I think that's a really good example of that.
これはその良い例だと思います。
And I think that's a really good example of that.
これは本当に良い例だと思います。
And I think that's a really good example of that.
そして、これは本当に良い例だと思います。
And I think that's a really good example of that.
そして、それは本当に良い例だと思います。
And it's quite a controversial stance you've taken that the right way to do this is to put it out there in public and then harness all this.
そして、あなたは、公の場で発表することが正しいやり方であり、その結果、すべてのものを利用することができるという、極めて論争的なスタンスを取っています。
You know, instead of just your team giving feedback, the world is now giving feedback.
自分のチームだけがフィードバックするのではなく、今や世界中がフィードバックしているわけですからね。
But if bad things are going to emerge, it is out there.
しかし、もし悪いことが出てくるとしたら、それは外に出ているのです。
So the original story that I heard on OpenAI when you were founded as a nonprofit: where you were there as the great sort of check on the big companies doing their unknown, possibly evil thing with AI; and you were going to build models that, you know, somehow held them accountable and were capable of slowing the field down if need be.
元々の話をOpenAIが非営利団体として設立されたときに聞いたのですが、大企業がAIを使って知らないうちに何か悪いことをし始めるのを防ぐための素晴らしいチェック機能として存在し、必要に応じて分野の進展を遅らせることができるモデルを開発する予定でした。
Or at least that's kind of what I had.
少なくとも、私はそう考えていました。
And yet what's happened, arguably, is the opposite, that your release of GPT, especially ChatGPT, put such shockwaves through the tech world that now Google and Meta and so forth are all scrambling to catch up.
しかし、実際には逆のことが起こっていると言えます。特にChatGPTのリリースにより、テクノロジー業界が衝撃を受け、GoogleやMetaなどが追いつこうと奮闘しています。
And some of their criticisms have been, you are forcing us to put this out here without proper guardrails, or we die.
そして、彼らの批判の中には、「適切なガードレールもなく、無理やりこれを世に送り出すのか、さもなくば我々は死ぬ」というものもあります。
How do you make the case that what you have done is responsible here and not reckless?
自分たちがやったことが無謀なことではなく、責任あることだということをどう主張するのか。
Yeah, we think about these questions all the time.
ええ、私たちはこのような疑問についていつも考えています。
Like, seriously all the time.
真剣にいつも考えています。
I don't think we're always going to get it right.
いつもうまくいくとは思っていません。
But one thing I think has been incredibly important, in the beginning, when we were thinking about how to build artificial general intelligence, actually have it benefit all of humanity.
しかし、私が信じられないほど重要だと思うのは、当初、人工知能を構築する方法について考えていたとき、実際に全人類に利益をもたらすようにすることでした。
Like, how are you supposed to do that?
どうすればいいのでしょう?
And that the default plan of being like, well, you build in secret, you get this super-powerful thing, and then you figure out the safety of it, and then you push go, and you hope you got it right.
秘密裏に構築し、超強力なものを手に入れ、その安全性を確認したうえで、「うまくいった」と思って突き進むというのが、既定のプランです。
I don't know how to execute that plan.
私は、その計画を実行する方法を知りません。
Maybe someone else does, but for me, that was always terrifying.
でも、私にとっては、それが常に恐怖だったのです。
It didn't feel right.
正しいとは思えなかったのです。
And so I think that this alternative approach is the only sort of other path that I see, is to let reality hit you in the face.
だから、この代替案は、現実を目の当たりにすることで、唯一、他の道筋を示すものだと思うのです。
And I think you do give people time to give input.
そして、人々に意見を述べる時間を与えることだと思います。
You do have what, before these machines are perfect, before they are super-powerful, that you actually have the ability to see them in action.
機械が完璧になる前に、超強力なものになる前に、実際に動いているところを見ることができるのです。
And we've seen it from GPT-3.
GPT-3では、それを実感しました。
GPT-3, we really were afraid that the number one thing people were going to do with it was generate misinformation and try to tip elections.
GPT-3では、誤報を流して選挙を混乱させるのではないかと心配しました。
Instead, the number one thing was generating Viagra spam.
しかし、その代わりに、バイアグラ・スパムを生成する人が続出しました。
So Viagra spam is bad, but there are things that are much worse.
バイアグラ・スパムは悪いものですが、もっと悪いものもあるのですね。
Absolutely.
その通りです。
Suppose you're sitting in a room, there's a box on the table.
ある部屋に座っていて、テーブルの上に箱が置いてあるとします。
You believe that in that box is something that there's a very strong chance, it's something absolutely glorious, that's going to give beautiful gifts to your family and to everyone.
その箱の中には、非常に強い確率で、絶対に素晴らしいものが入っていて、あなたの家族やみんなに素敵なプレゼントをくれると信じていますよね。
But there's actually also a one percent thing in the small print there that says, Pandora.
しかし、実はその小さな文字には、Pandoraと書かれている1パーセントのものもあるのです。
And there's a chance that this actually could unleash unimaginable evils on the world.
そして、この箱が想像を絶する悪を世界に解き放つ可能性があるのです。
Do you open that box?
その箱を開けてしまうのか?
Well, so absolutely not.
まあ、だから絶対にしない。
I think you don't do it that way.
そういうやり方はしない方がいいと思います。
And honestly, I'll tell you a story that I haven't actually told before, which is that shortly after we started OpenAI, I remember I was in Puerto Rico for an AI conference.
正直なところ、これまで話したことのない話をしますと、OpenAIを立ち上げた直後、AIカンファレンスでプエルトリコに行ったときのことです。
I was sitting in the hotel room, looking out over this wonderful water, all these people having a good time.
ホテルの部屋に座って、素晴らしい海を眺めながら、みんなが楽しい時間を過ごしていたんです。
And you think about it for a moment.
そして、ちょっと考えてみたんです。
If you could choose for a potentially, basically that Pandora's box, to be five years away or 500 years away, which would you pick?
5年先か500年先か、パンドラの箱のような可能性を選ぶことができるとしたら、どちらを選びますか?
And on the one hand, you're like, well, maybe for you personally, it's better to have it be five years away.
一方では、あなた個人にとっては、5年先のほうがいいかもしれないと思うでしょう。
But if it gets to be 500 years away and people get more time to get it right, which do you pick?
しかし、500年後になれば、人々はより多くの時間を得て、それを正しく理解することができるようになるのだとしたら、あなたはどちらを選びますか?
And I just really felt it in that moment.
その時、私は本当にそう思いました。
I was like, of course you do the 500 years, for real.
もちろん、500年先もやるんだろうな、マジで、と。
My brother was in the military at the time, and he puts his life on the line in a much more real way than any of us typing things in computers and developing this technology at the time.
私の兄は当時、軍に所属していました。彼は、当時、コンピューターに何かを入力したり、この技術を開発したりする誰よりも、ずっと現実的な方法で自分の命を危険にさらしているのです。
And so, yeah, I'm really sold on the, you've got to approach this right.
だから、私は、「正しいアプローチをしなければならない」ということに、とても納得しています。
But I don't think that's quite playing the field as it truly lies.
しかし、それが本当の意味でのフィールドプレイだとは思えません。
Like, if you look at the whole history of computing, like, I really mean it when I say that this is a industry-wide, or even sort of just almost like a human development of technology-wide shift.
コンピューティングの歴史全体を見ても、これは業界全体、あるいは人類の発展による技術全体の転換である、と私は本気で言っています。
And the more that you sort of don't put together the pieces that are there, right?
そして、そこにある断片をまとめないほど、そうなのです。
We're still making the faster computers, we're still improving the algorithms, like all these things, they are happening.
コンピュータの高速化、アルゴリズムの改良など、さまざまなことが行われています。
But if you put them together, you get an overhang.
しかし、それらを組み合わせると、オーバーハングが発生します。
Which means that if someone does, or that the moment that someone does manage to connect to the circuit, then you suddenly have this very powerful thing, no one's had any time to adjust, like, who knows what kind of safety precautions you get.
つまり、誰かが回路に接続することに成功した瞬間、あるいは誰かがそれをする瞬間には、非常に強力なものが手に入り、調整する時間がなく、どのような安全対策が取られるか分からない状況が生じます。
And so I think that one thing I take away is, even if you think about development of other sort of technologies, think about nuclear weapons, people talk about being like a zero-to-one sort of change in what humans could do.
そこで思いつくのは、他の技術の開発、例えば核兵器の開発について考えると、人間ができることについてゼロからワンへの変化があると言われています。
But I actually think that if you look at capability, it's been quite smooth over time.
しかし、実際には、能力という観点から見ると、その変化は非常にスムーズであったと思います。
So the history, I think, of every technology we've developed has been, you've got to do it incrementally, and you've got to figure out how to manage it for each moment that you're sort of increasing it.
つまり、私たちが開発してきたあらゆる技術の歴史は、段階的に行う必要があり、能力を向上させる瞬間ごとに、それをどのように管理するかを考えなければならなかったのです。
So what I'm hearing is that you, that the model you almost have is that we have birthed this extraordinary child that may have superpowers that take humanity to a whole new place.
つまり、あなたが考えているモデルは、人類をまったく新しい世界に導く超能力を持つかもしれない特別な子供を産んだということなのでしょう。
It is our collective responsibility to provide the guardrails for this child, to collectively teach it to be wise and not to tear us all down.
この子にガードレールを提供し、賢くなるように教え、私たち全員を破滅させないようにするのが、私たち集団の責任です。
Is that basically the model?
基本的にはそういうモデルなのでしょうか?
I think it's true, and I think it's also important to say this may shift, right?
その通りだと思いますし、これが変化する可能性があると言うことも重要だと思いますね。
Like, we've got to take each step as we encounter it, and I think it's incredibly important today that we all do get literate in this technology, figure out how to provide the feedback, decide what we want from it.
私たちは一歩ずつ進んでいく必要がありますし、今日、私たち全員がこの技術に精通し、フィードバックを提供する方法を見つけ出し、それから何を望むかを決定することが非常に重要だと思います。
And I think that my hope is that that will continue to be the best path, but it's so good we're honestly having this debate, and otherwise, if it weren't out there.
しかし、このような議論ができるのはとても良いことだと思いますし、そうでなければ、このような議論ができなかったでしょう。
Greg Brockman, thank you so much for coming to TED and blowing our minds.
グレッグ・ブロックマン、TEDに来てくれて、私たちの心を揺さぶってくれて、本当にありがとうございました。
Thank you.
ありがとうございました。
Thank you.
ありがとうございました。
この記事が気に入ったらサポートをしてみませんか?