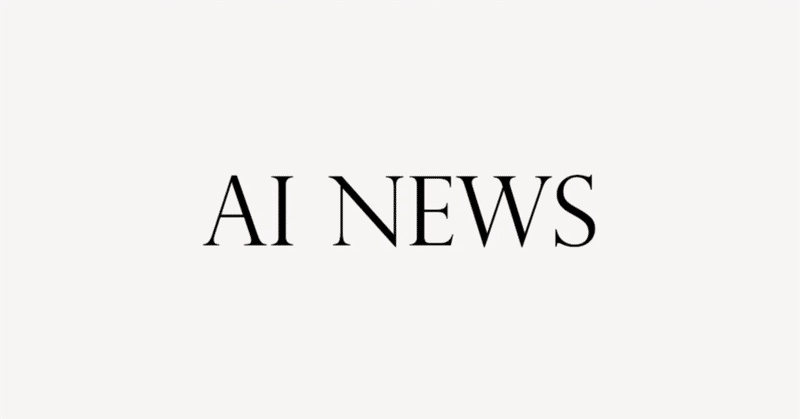
【AIニュース】英語解説を日本語で読む【2023年5月14日|@TheAIGRID】
NVIDIAは最新の生成AIの進歩を紹介するために20以上の研究論文を公開しました。その中で注目すべき4つの論文を紹介します。
公開日:2023年5月14日
※動画を再生してから読むのがオススメです。
NVIDIA just released around 20 different research papers in which they detail the very next advancements in generative AI and how it's going to impact us.
NVIDIAは約20種類の研究論文を発表し、その中で、生成AIの次の進歩について詳しく説明し、それが私たちにどのような影響を与えるかについて述べています。
Now, in this video, we're going to cover four of the key ones that they talk about and why these ones are very, very interesting.
このビデオでは、その中から4つの重要なものを取り上げ、これらのものが非常に興味深いものである理由を説明するつもりです。
So stay tuned because honestly, you're going to be surprised at what NVIDIA has been able to do, especially with the advancements in AI.
正直なところ、特にAIの進歩によってNVIDIAができるようになったことに驚かれることでしょうから、ご期待ください。
So one of NVIDIA's first groundbreaking papers is essentially learning physically simulated tennis skills from broadcast videos.
NVIDIAの最初の画期的な論文の1つは、基本的に放送されたビデオから物理的にシミュレートされたテニススキルを学習するものです。
In this video, and in the research paper that they've done, they essentially talk about how it's possible now for you to use broadcast videos.
この動画や研究論文では、放送用のビデオを使用して、現在することが可能になったと述べています。
For example, perhaps the NBA or maybe just a simple tennis match.
例えば、NBAやテニスの試合などです。
You can use this in order to simply mimic those skills or bodily movements, and map that accurately onto a 3D character.
そのような技術や体の動きを模倣して、3Dキャラクターに正確にマッピングすることができます。
Now, you have to understand that this isn't just something where they're simply just using some fake motion capture data.
ただ、これは単にモーションキャプチャーの偽データを使っているわけではない、ということを理解しておいてください。
This is really accurate, really precise, and really good data that is then being applied to this 3D rendered character that you can see.
これは、本当に正確で精密で優れたデータであり、それを3Dレンダリングされたキャラクターに適用しているのです。
So we know that motion capture is very expensive to produce at scale, and we know that some companies that want to use motion capture currently cannot do so because, of course, it might be outside of their budget range.
モーションキャプチャは大規模に制作すると非常に高価であり、現在モーションキャプチャを使用したいと考えている企業の中には、もちろん予算範囲を超えるために使用できない企業もあります。
Now, of course, what's also an issue as well is that motion capture data is usually quite large, so this sometimes does have its issues.
また、モーションキャプチャーのデータは非常に大きいので、問題がある場合もあります。
So, as you know, right here, the more actually recorded footages that you do have, let's say, for example, when we look at games of athletes that are actually playing in their current sport, it's always going to be much more natural and much better than just simply recording some motion capture footage.
ですので、ご存知の通り、ここで実際に録画された映像がたくさんあるほど、例えば、現役のスポーツ選手たちの試合を見る場合など、モーションキャプチャ映像を単純に録画するよりもはるかに自然で優れています。
And essentially, what this paper talks about, and what this video talks about - which we'll leave a link to in the description - is how they're able to essentially get that data from the video.
この論文やこの動画が語っているのは、基本的には、そのビデオからどのようにしてデータを取得できるのかということで、説明のリンクを残しておきます。
They then essentially translate that onto a 3D character, in which they can then accurately represent exactly what was done in the video.
そして、そのデータを3Dキャラクターに変換し、ビデオで行われたことを正確に表現することができるのです。
To the extent that there's a lot that these characters can actually do in terms of their ability to hit the ball into several different locations at certain times, and hit it into a certain spot at a certain time.
このキャラクターが実際にできることは、ある時間に複数の異なる場所にボールを打ち込んだり、ある時間にある場所にボールを打ち込んだりすることができることです。
So it's something that is definitely refined.
だから、確実に洗練されているものなんです。
It's not something that seems quite basic.
かなり基本的なことだと思われるものではありません。
I know that many different projects out there are quite basic, but this definitely seems very, very refined.
世の中にあるさまざまなプロジェクトは非常に基本的なものだと思いますが、これは間違いなく非常に洗練されたものだと思います。
The system that they've developed seems very, very good when it does come to refining some of the jagged movements.
彼らが開発したシステムは、ギザギザの動きを精緻化するのに非常に適しているようです。
They talk about how there are many different issues with this, but they've managed to refine those and fix those.
いろいろな問題があるようですが、それらを改善し、修正することができたと語っています。
Now, some of you might be wondering, what other tools are there like this out there?
さて、このようなツールは他にあるのだろうかと思われる方もいらっしゃるかもしれません。
Now, there's one tool that recently did come to mind, which is one that Dynamics did release.
最近思いついたツールとしては、Dynamicsがリリースしたものがあります。
Something that is quite similar essentially that tries to wipe out the need for necessary mocap animations, and it's actually very, very interesting.
これは、モーキャップアニメーションの必要性を排除しようとするもので、非常によく似たものです。
There are many different demos that you can actually see online, and of course, this is in beta stage where you can actually sign up to the waitlist.
オンラインではさまざまなデモを見ることができますし、もちろんこれはベータ版の段階で、ウェイティングリストに登録することができます。
So this is definitely something as well that is going to be going on in the motion capture space, another area that is being disrupted by AI.
モーションキャプチャーの分野でも、AIによって破壊されつつある分野であることは間違いないでしょう。
But I do have to be honest with you, this research paper by NVIDIA, it does show a very fine-tuned version, I think, of what Wonder Dynamics is trying to do.
しかし、正直なところ、このNVIDIAの研究論文は、ワンダーダイナミクスがやろうとしていることを非常に細かく調整したものだと思います。
So I think that if this is fully released at skill, it will most certainly change the way things are captured, especially in video games and in any other industries where you do need that kind of data.
ですから、もしこれがスキルとして完全にリリースされれば、特にビデオゲームや、そのようなデータを必要とする他の産業において、物事の捉え方が変わることは間違いないでしょうね。
Now, NVIDIA, like he said once again, are coming with more stuff.
さて、NVIDIAは、彼がもう一度言ったように、もっといろいろなものを出してきます。
Now, this one, I gotta be honest with you, is very, very impressive.
これは、正直に言って、とても印象的なものです。
Okay, this is what we call key-locked ranked one editing for text-to-image personalization.
これは、テキストから画像へのパーソナライゼーションのための、キーロックされたランク1編集と呼ばれているものです。
That's what NVIDIA has titled this.
NVIDIAはこのように名付けています。
And essentially, this is what they call profusion.
そして、本質的には、これは彼らが「プロフュージョン」と呼ぶものです。
So it says,We present profusion, a new text-to-image personalization method with only 100 kilobytes model size, trained for roughly four minutes.' This can creatively portray personalized objects and it allows significant changes in their appearance.
モデルサイズがわずか100キロバイトで、およそ4分間トレーニングされた新しいテキストから画像へのパーソナライゼーション手法であるprofusionを紹介します」と書かれています。これは、パーソナライズされたオブジェクトを創造的に描写することができ、その外観を大きく変えることができるのです。
Now, trust me when I say you're about to be shocked at how good this is at training personalized images based on literally sometimes even just one image.
文字通り、たった1枚の画像をもとにパーソナライズされた画像を学習させるというこの手法の優秀さに、皆さんは衝撃を受けることでしょう。
Then, you're allowed to customize that with a text prompt.
そして、それをテキストプロンプトでカスタマイズすることができるのです。
So let's take a few looks at some of the examples because this is by far one of the most game-changing things that I've seen when it comes to image generation in AI.
では、いくつかの例を見てみましょう。これは、AIの画像生成に関して、私が見た中で最も画期的なものの1つです。
So the first example we have here is profusion being able to generate eight appealing images in several seconds where essentially they transform the nature of what's going on in that image.
最初の例として、Profusionがいくつかの秒で8枚の魅力的な画像を生成することができ、その画像で起こっていることの本質を変えることができます。
They're able to take the actual table and then present it covered in snow.
実際のテーブルを、雪に覆われた状態で見せることができるのです。
Now, you can see right here that this isn't just fluff.
このように、この画像は単なるフワフワしたものではないことがお分かりいただけると思います。
This is actually really high-quality data that we can see in this image that looks really legible and really good.
この画像で見ることができるのは、本当に高品質なデータで、本当に読みやすく、とても良いものです。
Now, this is what I'm saying when I say that this is going to be more widely used.
このように、このデータはもっと広く使われるようになると思います。
I believe than maybe some applications like Midjourney because this personalization is essentially what really drives the utilization of these kind of models.
Midjourneyのようなアプリケーションよりも、このパーソナライゼーションが、この種のモデルの活用の原動力になると思うからです。
Because if you can personalize something, then it definitely does have more use.
なぜなら、パーソナライズができれば、より多くの人に使ってもらえるからです。
Now, of course, as well, you can see that there is a large level of consistency with these images.
もちろん、これらの画像には大きな一貫性があることもおわかりいただけると思います。
This is essentially what a lot of people have wanted in Midjourney for quite some time, and profusion actually excels at this and does it very, very well.
これは、多くの人がMidjourneyに求めていたことであり、profusionはこの点に優れていて、非常にうまくできています。
Now, there's also some other incredible examples of where you're able to train.
このほかにも、驚くようなトレーニングの例がいくつかあります。
The examples you can see on the left-hand side, the examples of the teddy and of course the teapot.
左側にあるテディとティーポットの例です。
Then essentially what they've done is they've transformed the single concept into another image, and this looks very, very good.
このように、1つのコンセプトを別のイメージに変換しているのですが、これが非常によく見えるのです。
So we can see here that this is really cool.
つまり、これはとてもクールだということがわかります。
But what you don't see in this example, if you look closer, you can see that they've actually combined two of the trained images together.
しかし、この例では見えませんが、よく見ると、実際に2つの学習済み画像を組み合わせていることがわかります。
On the right-hand side, we can see inference combined concepts.
右側には、推論で結合された概念が表示されています。
So, a teddy sitting by the fire with the teapot and a teddy sailing on a teapot in a lake.
テディがティーポットと一緒に火のそばに座っている」と「テディがティーポットの上に乗って湖を航行している」です。
And that's really, really cool.
これは本当に素晴らしいことです。
I do believe that this has many different applications, and I'm pretty sure Adobe is going to be scrambling to get this kind of software embedded into Firefly.
この技術は多くの異なるアプリケーションがあり、私はAdobeがこの種のソフトウェアをFireflyに組み込むために奔走するだろうと確信しています。
This is something that I don't think I've seen anywhere apart from Dreambooth.
これは、Dreambooth以外では見たことがないものだと思います。
But with this level of consistency and of course quality, we haven't seen this just yet.
しかし、このレベルの一貫性ともちろん品質で、私たちはまだ見たことがありません。
So this is honestly truly groundbreaking stuff from NVIDIA.
NVIDIAの製品は、本当に画期的なものです。
Now, what's also cool is that you can see some of the other examples here, where there's a teddy dressed in a blue suit looking at a gourmet meal.
また、青いスーツを着たテディがグルメな食事を見ているといった他の例も見ることができます。
Looks very accurate.
とても正確そうですね。
Then of course, there's a dog wearing a sombrero, a definitely another accurate output.
もちろん、ソンブレロをかぶった犬も、間違いなく正確な出力です。
Now, one thing that many people are wondering is how does this compare against the other models that are in the space that do exactly this.
さて、多くの人が気になるのは、このようなことをする他のモデルと比較してどうなのかということでしょう。
So, you can see right here that they actually showcase other models and exactly how they handle the same exact prompt with the same exact thoughts.
ここでは、他のモデルが同じ正確なプロンプトと同じ正確な思考をどのように扱っているかを実際に示しています。
And I think it's clear that by far, NVIDIA's model is clearly just the best.
そして、NVIDIAのモデルが圧倒的に優れていることは明らかだと思います。
I mean, although they have used several prompts to get this, the other ones just didn't seem to actually get the task, and they honestly do fail, but not spectacularly.
つまり、彼らはこれを得るためにいくつかのプロンプトを使いましたが、他のものは実際にタスクを理解していないようで、正直言って失敗していますが、目を見張るほどではありません。
But NVIDIA is just that step ahead.
しかし、NVIDIAはその一歩先を行っているのです。
Now, this right here is what I wanted to show you all because this is what I think the future of AI image generation is going to be.
さて、ここで皆さんにお見せしたいのは、これこそがAI画像生成の未来だと思うからです。
Where you have this one-shot personalization.
一発勝負のパーソナライゼーションです。
Now, some people might just have one image of something and they want to essentially have consistency of that image and then essentially manipulate that even further.
ある人は、あるものの画像を1枚だけ持っていて、その画像の一貫性を保ちつつ、さらにそれを操作したいと思うかもしれません。
And with NVIDIA's Perfusion, you can see right here that this is exactly what we have.
NVIDIAのPerfusionを使えば、まさにこのようなことが可能になります。
So, this is something that is truly game-changing.
これは、まさに画期的なことなのです。
NVIDIA's Perfusion is one of the models that I think they're going to talk more about at the event, and it's definitely something that you should be paying attention to.
NVIDIAのPerfusionは、イベントで詳しく述べられると思われるモデルの1つであり、ぜひ注目すべきものです。
Because once it does get embedded into, I believe, potentially their NVIDIA Castle, we could then be seeing it rolled out into many other different applications.
なぜなら、それがNVIDIAのCastleに組み込まれる可能性があるとすれば、その後、さまざまなアプリケーションに展開されることになるでしょう。
Because NVIDIA is going to be allowing many different, you know, companies and softwares to be able to use their Picasso cloud service.
NVIDIAは、さまざまな企業やソフトウェアがPicassoクラウドサービスを利用できるようにする予定だからです。
So, definitely something to look out for.
ですから、間違いなく注目すべきものです。
Now, this is very interesting.
さて、これは非常に興味深いことです。
This, once again, is showing where NVIDIA leads the way.
これは、NVIDIAがリードしていることを改めて示しています。
This is live 3D portraits from real-time Radiance fields for single image portrait view synthesis.
これは、リアルタイムのラディアンスフィールドからのライブ3Dポートレートで、単一画像のポートレートビュー合成を行います。
Or, in simpler terms, they present a One-Shot method to infer and render photorealistic 3D representations from a single image in real time.
もっと簡単に言うと、1枚の画像からフォトリアリスティックな3D表現をリアルタイムで推測し、レンダリングするワンショット方式を提示しています。
So, they can just pretty much get an image of something and then essentially get a 3D realistic, photorealistic representation of that image.
つまり、何かの画像を取得し、その画像の3Dリアルなフォトリアリスティック表現を得ることができるのです。
Now, right here you can see the inputs versus the outputs.
さて、ここに入力と出力が表示されています。
And for those of you who haven't seen the competition's level of detail, you won't understand why this is truly groundbreaking.
そして、競合の詳細なレベルを見たことがない人には、なぜこれが本当に画期的なことなのか理解できないかもしれません。
Because it's very hard to infer 3D data from an image.
なぜなら、画像から3Dデータを推測するのは非常に難しいからです。
Because there are many different complex things at stake when you're trying to depict what goes where.
何がどこにあるのかを描き出すには、さまざまな複雑な要素が絡んでくるからです。
And it's something that you can easily get wrong.
しかも、簡単に間違ってしまうものなのです。
But this is something that NVIDIA has mastered.
しかし、これはNVIDIAが得意とするところです。
And this research paper/video that they talk about goes over in detail how they've managed to do this and all the different techniques that they've used.
この研究論文/ビデオでは、NVIDIAがどのようにしてこれを実現したのか、またどのようなテクニックを使ったのかについて詳しく説明しています。
I mean, it's honestly quite interesting as to how they managed to do this.
つまり、彼らがどうやってこれを実現したのか、正直なところ非常に興味深いのです。
Because this wasn't something a couple of years ago that you thought you'd be able to get with this level of accuracy.
数年前までは、これほどの精度を出せるとは思ってもみなかったからです。
And like I said before, as AI continues to develop at this rapid pace, we're going to start seeing innovations which we weren't thought possible develop at a ridiculous level.
そして、先ほども言ったように、AIがこの急速なペースで発展し続けるにつれて、私たちは、可能だと思われていなかったイノベーションがとんでもないレベルで発展するのを見るようになるでしょう。
Now, this was something that I found really, really interesting.
さて、これは私が本当に、本当に面白いと思ったことです。
And where you can see in real time, you're able to get these different effects.
リアルタイムで見ると、このように異なる効果を得ることができるのです。
You can see that with the input compared to the output novel view, you're able to get a completely different angle on what this speaker is saying.
入力と出力の新規ビューを比較すると、この話者が言っていることに完全に異なる角度を得ることができます。
And you can see right here that we have a driving video, then we have an input single image.
また、ここでは、走行中のビデオと、入力された1枚の画像を見ています。
And then, of course, we have the 2D talking head and the 3D lifting with their method.
そして、もちろん、2Dのトーキングヘッドと3Dのリフティングがあります。
Now, this can be comparable to did in some aspect.
これは、ある面ではdidに匹敵するものです。
If you don't know what that is, essentially, it's where people convert an image to something moving.
ご存じない方もいらっしゃるかもしれませんが、要するに、画像を動きのあるものに変換するものです。
But this is on a completely numb level, you can see right here.
しかし、これは完全に無感覚なレベルなんです。
That you can see in videos at the top left compared to the others in the other areas.
左上の動画で、他の領域のものと比べてわかることです。
And we can see clearly that NVIDIA's is far more accurate, with far more detail.
そして、NVIDIAの方がはるかに正確で、はるかに詳細であることがはっきりとわかります。
And this is clearly superior to anything else that is currently being developed.
そして、これは現在開発されている他のどの製品よりも明らかに優れています。
So, I would say hat stuff to NVIDIA for doing this.
ですから、私はNVIDIAがこのようなことをしたことに、脱帽ですと言いたいです。
Constantly with every single blog, with every single research paper that I read compared to the other research papers that I read literally just a couple weeks before, we're seeing NVIDIA push the boundaries on what's truly capable.
ブログのたびに、研究論文を読むたびに、ほんの2週間前に読んだ他の研究論文と比較して、NVIDIAが真に可能なことの限界を押し広げているのを目の当たりにしています。
Now, what's also cool is that essentially, as we talked about previously, in real time, you can see that this is a live demo of someone essentially using a phone to RGB video to 3D.
さて、さらに素晴らしいのは、前回お話したように、リアルタイムで、誰かが携帯電話を使ってRGBビデオを3Dにするライブデモを見ることができることです。
Now, of course, this is running on an RTX 490, which is essentially one of their top-tier graphics cards.
もちろん、これはRTX 490で動作しており、実質的に最上位のグラフィックスカードの1つです。
But this also goes to show the potential applications of this.
しかし、このデモは、RTX 490の潜在的な用途を示すものでもあります。
Imagine maybe you wanted to see different angles of something, or maybe you wanted to see someone essentially in a more 3D way.
例えば、何かを別の角度から見たいと思ったとき、あるいは誰かをより立体的に見たいと思ったとき、これを想像してみてください。
This is going to present more lifelike and more realistic video calls.
よりリアルで臨場感のあるビデオ通話を実現することができるのです。
So I'm not exactly sure what these applications are going to be.
ですから、どのような用途になるかは、まだよくわかりません。
Of course, right now, it's just pure speculation.
もちろん、今はまだ純粋な憶測に過ぎません。
But it just goes to show that being able to generate this amount of depth and detail from a simple 2D image is truly incredible.
しかし、単純な2D画像からこれだけの奥行きとディテールを生み出すことができるのは、本当にすごいことだということがわかります。
Now, this one is really cool.
さて、こちらは本当にクールです。
Essentially, if you're someone who plays games, you know that hair is something that games do really struggle with.
ゲームをされる方ならご存知だと思いますが、ゲームでは髪の毛の表現が非常に難しいのです。
This is because of the complex computations needed to essentially calculate how each hair strand is going to move.
これは、髪の毛の1本1本がどのように動くかを計算するために、複雑な計算が必要になるためです。
But NVIDIA has come up with a solution.
しかし、NVIDIAはその解決策を思いつきました。
So, in this new paper, they essentially talk about a method that can simulate tens of thousands of hairs in high resolution and in real time.
この新しい論文では、何万本もの髪の毛を高解像度でリアルタイムにシミュレートできる方法について述べています。
This is done using neural physics and an AI technique that teaches a neural network how to predict how an object would move in the real world.
これは、神経物理学と、現実世界で物体がどのように動くかを予測する方法をニューラルネットワークに教えるAI技術を使って行われています。
So essentially, what they're doing is using these neural networks to essentially teach them how these hairs would look.
つまり、ニューラルネットワークを使って、毛髪がどのように見えるかを教えているのです。
And remember, this is going to be in real time.
しかも、これはリアルタイムで行われます。
So this is going to be really cool to see how it actually plays out in real game.
ですから、実際のゲームではどのように動くのか、とても楽しみです。
Then, of course, we had the neural textures versus the BCX textures.
そしてもちろん、ニューラルテクスチャとBCXテクスチャの比較も行いました。
So essentially, what we have here is once again using neural networks to essentially compress textures that usually are quite high in file size but at no other cost.
つまり、ニューラルネットワークを使って、通常はファイルサイズが大きいテクスチャを圧縮しているのですが、それ以外のコストはかかりません。
So there's no cost in GPU size, there's no cost in essentially size.
つまり、GPUサイズや本質的なサイズにコストはかかりません。
You can see that they're both around three megabytes in file size.
どちらもファイルサイズは3メガバイト程度であることがお分かりいただけると思います。
But at the same time, you can see that the one on the left, NVIDIA's new neural textures, actually produces up to 16 times more quality with the same actual file size.
しかし同時に、左側のNVIDIAの新しいニューラル・テクスチャは、同じファイルサイズで最大16倍もの品質を生み出していることがわかります。
This is very, very interesting because it means essentially you're going to get higher quality at the same power level.
これは非常に興味深いことで、同じパワーレベルでより高いクオリティが得られることを意味します。
So this is really, really game-changing for NVIDIA.
つまり、これはNVIDIAにとって、本当に、本当に画期的なことなのです。
Now, this annual conference on computer graphics is going to be happening in August.
さて、このコンピュータグラフィックスに関する年次会議は、8月に開催される予定です。
That's where they're going to be presenting all of their ideas, especially some of those that are interconnected with AI.
そこで、NVIDIAのすべてのアイデア、特にAIと相互に関連するアイデアが発表される予定です。
And it's going to be interesting to see what other stuff NVIDIA has to talk about, especially expanding upon some of the topics that we talked about today.
NVIDIAが今後どのような話題を取り上げるのか、特に今日話したトピックをさらに拡大することが興味深いでしょう。
この記事が気に入ったらサポートをしてみませんか?