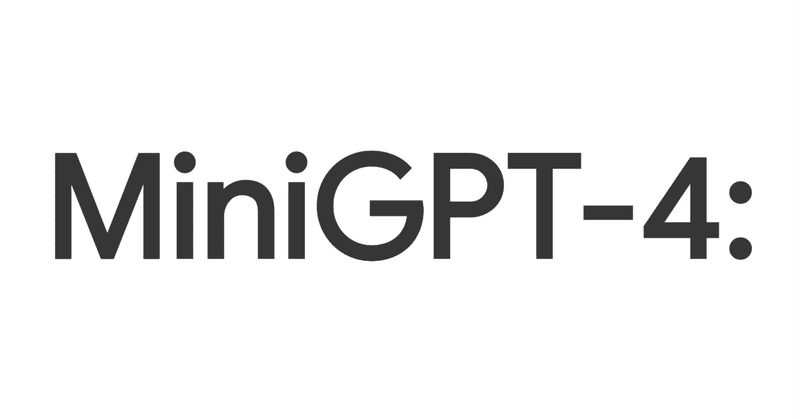
【MiniGPT-4】英語解説を日本語で読む【2023年4月21日|@AINewsOfficial】
MiniGPT-4の解説です。
公開日:2023年4月21日
※動画を再生してから、インタビューを読むのがオススメです。
Artificial intelligence has taken another leap forward with the introduction of MiniGPT-4, which marries a frozen visual encoder with a large language model called Vakuna.
人工知能は、凍結型ビジュアルエンコーダーと大型言語モデル「Vakuna」を組み合わせたMiniGPT-4の登場により、さらに飛躍的な進歩を遂げました。
This groundbreaking model demonstrates a host of new visual tasks, plus an amazing level of emergent understanding that could redefine the landscape of AI applications, so keep watching to discover its three next-generation visual understanding abilities as we show you how you can use it for free.
この画期的なモデルは、いろいろな新しい視覚タスクを示すだけでなく、すごいレベルの新しい理解力が現れて、AIアプリケーションの風景を再定義できるかもしれません。だから、見ていてくださいね。次世代の3つの視覚理解能力を紹介しますし、無料で使える方法もお伝えします。
MiniGPT-4's base capabilities are impressive, doing much more than simply describing what it's seeing by even being able to create apps from handwritten sketches and explain visual abnormalities.
MiniGPT-4の基本能力は印象的で、手書きのスケッチからアプリを作成したり、視覚の異常を説明したりと、単に見ているものを説明する以上のことができます。
However, it's the emerging abilities of the model that truly set it apart.
しかし、このモデルの真価を発揮するのは、新たな能力です。
With its advanced, multimodal understanding, MiniGPT-4 can even write stories and poems inspired by images, solve problems, or teach users how to do what's being seen in images.
MiniGPT-4は、その高度なマルチモーダル理解力により、画像からインスピレーションを得た物語や詩を書いたり、問題を解決したり、画像に映っていることの方法をユーザーに教えたりすることもできます。
Despite its strengths, MiniGPT-4 does have limitations, such as language hallucination and sometimes inadequate perception capacities.
MiniGPT-4は、その長所にもかかわらず、言語の幻覚や時には不十分な知覚能力など、限界があります。
These issues can be addressed through more high quality training data, aligning with advanced large language models, and using stronger visual perception models.
これらの問題は、より質の高いトレーニングデータ、高度な大規模言語モデルとの整合性、より強力な視覚認識モデルの使用によって解決することができます。
As the field of artificial intelligence continues to advance, breakout models like this are pushing the boundaries of what is possible in multimodal generation, but by aligning visual information with text modality, MiniGPT-4 demonstrates the potential for even more advanced, versatile AI applications in the future.
人工知能の分野が進歩し続ける中、このようなブレイクアウトモデルは、マルチモーダル世代で可能なことの限界に挑戦していますが、MiniGPT-4は、視覚情報をテキストモダリティに合わせることで、将来的にさらに高度で汎用性のあるAIアプリケーションの可能性を示しています。
Furthermore, this new model has showcased its remarkable capabilities through various demonstrations, such as generating detailed image descriptions, identifying amusing aspects within images, uncovering unusual content from images, retrieving facts about people, movies, or art from an image, and even writing raps or creating advertisements for products seen in an image.
さらに、この新モデルは、画像の詳細な説明文の生成、画像の面白さの特定、画像から珍しい内容の発見、画像から人物や映画、芸術に関する情報の検索、さらには画像に写っている商品のラップや広告の作成など、さまざまなデモンストレーションを通じて、その驚くべき能力を披露しています。
All of these examples help to illustrate the powerful potential of MiniGPT-4 and its future successors in diverse applications.
これらの例は、MiniGPT-4とその後継機が、さまざまな用途で活躍する強力な可能性を示しています。
Created by a group of PhD students at King Abdullah University of Science and Technology in Saudi Arabia, MiniGPT-4's bicono-large language model is based on llama and reportedly attains 90% of ChatGPT's quality as assessed by GPT-4.
サウジアラビアのキング・アブドラ科学技術大学の博士課程学生グループによって開発されたMiniGPT-4は、ラマをベースとした2つの大型言語モデルで、GPT-4で評価されたChatGPTの90%の品質を達成したと言われています。
The model incorporates the pre-trained vision component from bootstrapping language image pre-training and introduces a single projection layer to align the encoded visual features with the vicuna language model while keeping all other vision and language components frozen.
このモデルは、ブートストラップ言語画像事前訓練で訓練された視覚コンポーネントを組み込み、単一の投影レイヤーを導入して、他のすべての視覚および言語コンポーネントを凍結したまま、符号化された視覚特徴をビキューナ言語モデルに合わせます。
The creators of MiniGPT-4 employed a two-stage training approach to optimize the model's performance.
MiniGPT-4の開発者は、モデルのパフォーマンスを最適化するために、2段階のトレーニングアプローチを採用しました。
The first stage involved pre-training the model on a vast collection of aligned image text pairs, while the second stage consisted of fine-tuning the pre-trained model with a smaller, high-quality image text dataset using a designed conversational template.
第1段階では、膨大な数の画像テキストペアを用いてモデルを事前学習し、第2段階では、設計された会話テンプレートを用いて、より小規模で高品質な画像テキストデータセットを用いて事前学習したモデルを微調整する。
This method improved generation reliability and usability while maintaining computational efficiency.
この方法により、計算効率を維持しながら、生成の信頼性と使いやすさを向上させることができました。
By being designed as a scaled-down version of the immensely powerful GPT-4, MiniGPT-4 aims to explore the advanced multimodal generation capabilities of its larger counterpart.
MiniGPT-4は、非常に強力なGPT-4の縮小版として設計されており、より大きなGPT-4の高度なマルチモーダル生成能力を追求することを目的としています。
The development process used vicuna as the language decoder plus a vision transformer's architecture with a pre-trained Q-former as the visual encoder.
開発プロセスでは、言語デコーダーにvicuna、視覚エンコーダーに事前学習済みのQ-formerを搭載したビジョントランスのアーキテクチャを採用しました。
A linear projection layer was used to bridge the gap between the visual encoder and the large language model, allowing for seamless integration of visual features with the model.
視覚エンコーダーと大規模な言語モデルとの間のギャップを埋めるために、線形投影レイヤーが使用され、視覚的特徴とモデルとのシームレスな統合が可能になりました。
As promised, here are the three next-gen abilities unveiled by MiniGPT-4.
約束通り、MiniGPT-4が発表した3つの次世代能力を紹介しよう。
Number one, writing stories and poems inspired by images.
その1、画像に触発されて物語や詩を書く。
With its advanced multimodal generation capabilities, MiniGPT-4 can generate creative content inspired by visual inputs, unlocking an exciting world of artificial intelligence-driven storytelling.
高度なマルチモーダル生成機能を持つMiniGPT-4は、視覚入力からインスピレーションを得た創造的なコンテンツを生成し、人工知能主導のストーリーテリングのエキサイティングな世界を解き放ちます。
Number two, solving problems shown in images.
その2 画像に示された問題を解決する。
MiniGPT-4 goes beyond mere visual recognition and can identify issues within images, providing solutions and insights.
MiniGPT-4は、単なる視覚認識にとどまらず、画像内の問題を特定し、解決策や洞察を提供することができます。
This capability opens the door for more practical and problem-solving artificial intelligence applications in real-world scenarios.
この機能は、実世界のシナリオにおいて、より実用的で問題解決型の人工知能アプリケーションの扉を開くものです。
Number three, teaching users how to perform tasks based on results in photos, like how to cook a meal from an image of a dish.
その3、料理の画像から料理を作る方法など、写真の結果に基づいたタスクの実行方法をユーザーに教える。
MiniGPT-4 takes image recognition to the next level by not only identifying food items in photos, but also guiding users through the cooking process.
MiniGPT-4は、写真に写っている食材を識別するだけでなく、料理のプロセスをユーザーに案内することで、画像認識を次のレベルに引き上げました。
This ability demonstrates the potential for AI to become a much more interactive and helpful presence in our everyday lives.
このように、AIは私たちの日常生活において、よりインタラクティブで便利な存在になる可能性を示しているのです。
With AI continuing to evolve and improve at an accelerating pace, MiniGPT-4's next-generation abilities are likely just the tip of the iceberg, with more emergent abilities to come as researchers and developers continue to explore and expand the capabilities of AI models like these.
AIが加速度的に進化・改善する中、MiniGPT-4の次世代能力は氷山の一角に過ぎず、研究者や開発者がこのようなAIモデルの能力を探求・拡大し続けることで、さらなる新たな能力が生まれる可能性があります。
More groundbreaking innovations and applications will become public much sooner than most people would expect.
もっと画期的なイノベーションとアプリケーションは、多くの人が予想するよりもずっと早く公開されることでしょう。
As we witness the impressive capabilities of MiniGPT-4 in the world of vision language models, another groundbreaking innovation from NVIDIA is making waves in the realm of artificial intelligence-generated content, with next-generation text-to-video using Stable Diffusion, which showcases the seamless fusion of language and visuals to create captivating video content.
ビジョン言語モデルの世界でMiniGPT-4の素晴らしい能力を目の当たりにする一方で、NVIDIAの別の画期的なイノベーションは、人工知能が生成するコンテンツの領域で波紋を広げている。安定拡散を用いた次世代のテキストトゥビデオでは、言語とビジュアルをシームレスに融合して魅力的なビデオコンテンツを作成できることが紹介されている。
In NVIDIA's recent demonstration, they revealed their high-resolution video synthesis using a latent diffusion model that converts text inputs into high-quality video outputs with minimal latency, showing the potential to transform industries such as advertising, entertainment, and education by providing a more accessible and efficient way to create compelling visual narratives with its ability for personalization.
NVIDIAの最近のデモでは、テキスト入力を最小限の待ち時間で高品質のビデオ出力に変換する潜在拡散モデルを使用した高解像度ビデオ合成が公開され、パーソナライゼーション能力により説得力のあるビジュアルナラティブを作成する、より身近で効率的な方法を提供し、広告、娯楽、教育などの産業を変革する可能性を示しました。
The model even facilitates temporally aligned image synthesis across multiple frames, setting new benchmarks in video generation.
このモデルは、複数のフレームにまたがって時間的に整列した画像を合成することも可能で、動画生成の新たなベンチマークとなるものです。
The model has even been trained to generate high-resolution car ride videos spanning several minutes, making it particularly relevant for autonomous driving research.
このモデルは、数分間の高解像度の車載映像も生成できるように訓練されており、特に自律走行に関する研究に適しています。
These videos have a resolution of 512 by 1024 pixels, establishing the state of the art in most benchmarks.
これらの動画の解像度は512×1024ピクセルで、ほとんどのベンチマークで最先端を確立しています。
Furthermore, NVIDIA's researchers have demonstrated the conversion of an existing Stable Diffusion model into a video model.
さらに、NVIDIAの研究者は、既存の安定拡散モデルをビデオモデルに変換することを実証しました。
This process involves fine-tuning the Stable Diffusion model with video data for a short period, followed by adding temporal layers behind each existing spatial layer of the network.
このプロセスでは、安定拡散モデルを短期間のビデオデータで微調整し、その後、ネットワークの既存の各空間レイヤーの後ろに時間レイヤーを追加します。
These layers are then trained with video data.
これらの層は、ビデオデータでトレーニングされます。
Time-stable upscalers are also trained to generate videos with a resolution of 1280 by 2048 pixels from text prompts.
また、テキストプロンプトから1280×2048ピクセルの解像度のビデオを生成するために、時間的に安定したアップスケーラーをトレーニングします。
By utilizing Stable Diffusion as the foundation for the video model, the researchers can build upon existing capabilities and techniques without having to develop a new model from scratch.
映像モデルの基盤として安定拡散を利用することで、研究者はゼロから新しいモデルを開発することなく、既存の機能や技術を利用することができます。
For instance, despite its training dataset containing only real-world videos, the AI model is also capable of generating art videos, thanks to the underlying Stable Diffusion model.
例えば、学習データセットには現実世界の動画しか含まれていないにもかかわらず、このAIモデルは、基礎となる安定拡散モデルにより、アート動画を生成することも可能です。
The generated videos range from 3.8 to 4.7 seconds in length, depending on the frame rate.
生成された動画の長さは、フレームレートに応じて3.8秒から4.7秒です。
NVIDIA's team has also demonstrated that their DreamBooth technology can work with the video-specific Stable Diffusion model, producing videos containing objects not found in the original training data.
NVIDIAのチームは、DreamBooth技術が動画に特化したStable Diffusionモデルと連携し、元のトレーニングデータにはないオブジェクトを含む動画を生成できることも実証しています。
This capability presents exciting opportunities for content creators who can use DreamBooth to personalize their video content.
この機能は、DreamBoothを使用してビデオコンテンツをパーソナライズすることができるコンテンツクリエーターにエキサイティングな機会を提供します。
By integrating temporal layers into the image-latent diffusion model fine-tuned with DreamBooth, the model can output extremely specific content, even when using nuanced text prompting, offering what is arguably the most impressive text-to-video generation to date.
DreamBoothで微調整された画像-静止拡散モデルに時間的なレイヤーを組み込むことで、微妙なテキストプロンプトを使用した場合でも、極めて特殊なコンテンツを出力することができ、これまでで最も素晴らしいテキスト-ビデオ生成機能を提供します。
The model also has the potential to generate slightly longer videos by applying the learned temporal layers convolutionally in time, although with a slight reduction in quality.
また、学習した時間的レイヤーを時間的に畳み込むように適用することで、若干の品質低下はあるものの、より長い動画を生成することも可能です。
Additionally, the model can simulate specific driving scenarios by training a bounding box-conditioned image-only latent diffusion model, enabling the creation of plausible videos from a user-defined scene.
さらに、このモデルは、バウンディングボックスを条件とする画像のみの潜在拡散モデルを学習させることで、特定の運転シナリオをシミュレートすることができ、ユーザーが定義したシーンからもっともらしい動画を作成することが可能です。
Furthermore, the model can generate multiple realistic rollouts from the same starting frame, demonstrating its versatility and potential applications across various domains.
さらに、このモデルは、同じスタートフレームから複数のリアルなロールアウトを生成することができ、その汎用性と様々な領域への応用の可能性を示しています。
この記事が気に入ったらサポートをしてみませんか?