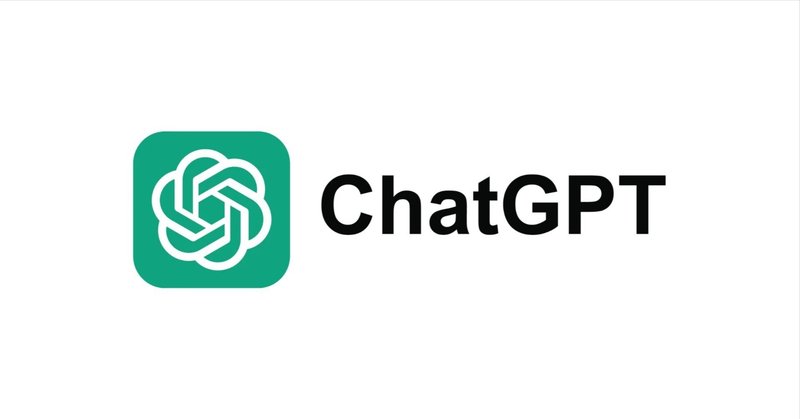
【ChatGPT】英語スピーチを日本語で読む【2023年4月29日|@TED】
コンピューターサイエンティストのYejin Choiが、ChatGPTのような大規模な人工知能システムの現状を解説します。
公開日:2023年4月29日
※動画を再生してから、インタビューを読むのがオススメです。
So, I'm excited to share a few spicy thoughts on artificial intelligence.
そこで、人工知能について、少しスパイシーな考えをお伝えしたいと思います。
But first, let's get philosophical.
しかし、その前に、哲学的な話をしましょう。
By starting with this quote by Voltaire, an 18th-century Enlightenment philosopher who said, common sense is not so common.
18世紀の啓蒙主義哲学者であるヴォルテールのこの言葉から始めることで、「常識はそれほど一般的ではない」ということがわかります。
Turns out this quote couldn't be more relevant to artificial intelligence today.
この言葉は、今日の人工知能にこれ以上ないほど適切であることがわかりました。
Despite that, AI is an undeniably powerful tool for the development of artificial intelligence.
にもかかわらず、AIは紛れもなく人工知能の開発にとって強力なツールなのです。
It's an undeniably powerful tool, beating the world-class Go champion, acing College of the Mission test and even passing the bar exam.
世界的な囲碁チャンピオンを倒し、College of the Missionのテストを突破し、司法試験にも合格する、紛れもない強力なツールなのです。
I'm a computer scientist of 20 years, and I work on artificial intelligence.
私は20年来のコンピュータ科学者で、人工知能を研究しています。
I am here to demystify AI.
私はここでAIを解明するために来ました。
So, AI today is like a goliath.
今日のAIはゴリアテのような存在です。
It is literally very, very large.
文字通り、とてもとても大きいのです。
It is speculated that the recent ones are trained on tens of thousands of GPUs and a trillion of words.
最近のものは、数万個のGPUと1兆個の単語で学習していると推測されています。
Such extreme-scale AI models, often referred to as large language models, appear to demonstrate sparks of AGI, artificial general intelligence.
このような極端なスケールのAIモデルは、しばしば大規模言語モデルと呼ばれ、AGI、人工一般知能の火種を示すように見える。
Except when it makes small, silly mistakes, which it often does.
ただし、AIが小さな、愚かな間違いを犯す場合は別です(よくあることですが)。
Many believe that whatever mistakes AI makes today can be easily fixed with brute force, bigger scale and more resources.
多くの人は、AIが今日犯したどんな間違いも、力技と大規模化、そしてより多くのリソースで簡単に解決できると信じています。
What, possibly, could go wrong?
何が間違っているのだろう?
So, there are three immediate challenges we face already at the societal level.
そこで、社会的なレベルですでに直面している3つの直接的な課題があります。
First, extreme-scale AI models are so expensive to train, and only few tech companies can afford to do so.
まず、極端なスケールのAIモデルは訓練にとてもお金がかかり、それを行う余裕があるのは一部のテック企業だけです。
So, we already see the concentration of power.
つまり、すでに権力の集中が見られるわけです。
But what's worse for AI safety?
しかし、AIの安全性にとってもっと悪いことは何でしょうか?
We are now at the mercy of those few tech companies, because researchers in the larger community do not have the means to truly inspect and dissect these models.
なぜなら、より大きなコミュニティの研究者は、これらのモデルを本当に検査し、解剖する手段を持っていないからです。
And let's not forget their massive carbon footprint and the environmental impact.
さらに、膨大な二酸化炭素排出量と環境への影響も忘れてはなりません。
And then there are these additional intellectual questions.
そして、さらに知的な疑問があります。
Can AI without robust common sense be truly safe?
強固な常識を持たないAIは、本当に安全なのだろうか?
Is it truly safe for humanity?
人類にとって本当に安全なのだろうか?
And is brute force scale really the only way, and even the correct way, to teach AI?
そして、ブルートフォーススケールは、本当にAIを教える唯一の方法なのか、そして正しい方法なのか?
So, I'm often asked these days whether it's even feasible to do any meaningful research without extreme-scale compute.
ですから、私は最近、極端なスケールの計算機なしで意味のある研究をすることは可能なのかとよく聞かれます。
And I work at a university and nonprofit research institute, so I cannot afford a massive GPU farm to create enormous language models.
私は大学や非営利の研究機関で働いているので、巨大な言語モデルを作成するための大規模なGPUファームを用意する余裕はないのです。
Nevertheless, I believe that there's so much we need to do and can do to make AI sustainable and humanistic.
それでも、AIを持続可能で人間らしいものにするために、私たちがすべきこと、できることはたくさんあると信じています。
We need to make AI smaller to democratize it, and we need to make AI safer by teaching human norms and values.
AIを民主化するためにはAIを小さくする必要がありますし、人間の規範や価値観を教えることで、AIをより安全なものにする必要があります。
Perhaps we can draw an analogy from David and Goliath.
ダビデとゴリアテから類推することができるかもしれません。
Here, Goliath represents the extreme-scale language models, and we take inspiration from an old-time classic, the Art of War.
ここでは、ゴリアテは極端な規模の言語モデルを表しており、昔の古典である「兵法」からヒントを得ます。
In my interpretation, it tells us to know your enemy, choose your battles, and innovate your weapons.
私の解釈では、敵を知り、戦いを選択し、武器を革新せよということです。
Let's start with the first, know your enemy, which means we need to evaluate AI with scrutiny.
まず、最初の「敵を知る」ですが、これはAIを精査して評価する必要があることを意味します。
AI is passing the bar exam.
AIは司法試験に合格しています。
Does that mean that AI is robust at common sense?
それは、AIが常識に強いということでしょうか。
You might assume so, but you never know.
そう思い込むかもしれませんが、わからないものです。
So suppose I left five clothes to dry out in the sun, and it took them five hours to dry completely.
では、5枚の服を天日干しにして、完全に乾くまで5時間かかったとします。
How long would it take to dry 30 clothes?
30着の服を乾かすとしたら、どれくらいの時間がかかるのだろう?
GPT-4, the newest, greatest AI system, says 30 hours.
最新最強のAIシステムであるGPT-4は30時間だと言う。
Not good.
まずいな。
A different one.
違うものです。
I have 12-liter jug and six-liter jug, and I want to measure six liters.
12リットルの水差しと6リットルの水差しがあるのですが、6リットルを測りたいと思います。
How do I do it?
どうすればいい?
Just use the six-liter jug, right?
6リットルの水差しを使えばいいんでしょう?
GPT-4 spits out some very elaborate nonsense.
GPT-4は非常に精巧なナンセンスを吐き出す。
Step one, fill the six-liter jug.
ステップ1、6リットルの水差しに水を入れる。
Step two, pour the water from six to 12-liter jug.
ステップ2、6リットルの水差しから12リットルの水差しに水を注ぎます。
Step three, fill the six-liter jug again.
ステップ3:6リットルの水差しに再び水を入れる。
Step four, very carefully pour the water from six to 12-liter jug.
ステップ4、非常に慎重に6リットルから12リットルの水差しに水を注ぎます。
And finally, you have six liters of water in the six-liter jug that should be empty by now.
そして最後に、もう空になっているはずの6リットルの水差しに、6リットルの水を入れるのです。
OK, one more.
よし、もう1つ。
Would I get a flat tire by bicycling over a bridge that is suspended over nails, screws and broken glass?
釘やネジ、割れたガラスの上に吊るされている橋の上を自転車で走ってパンクするでしょうか?
Yes, highly likely, GPT-4 says.
はい、その可能性が高いです、とGPT-4は言う。
Presumably because it cannot correctly reason that if a bridge is suspended over the broken nails and broken glass, then the surface of the bridge doesn't touch these sharp objects directly.
おそらく、橋が壊れた釘やガラスの上に吊り下げられている場合、橋の表面がこれらの鋭いものに直接触れないということを正しく推論できないためです。
OK, so how would you feel about an AI lawyer that aced the bar exam, yet randomly fails at such basic common sense?
では、司法試験に合格していながら、このような基本的な常識がないAI弁護士について、あなたはどう感じますか?
AI today is unbelievably intelligent and then shockingly stupid.
今日のAIは、信じられないほど知的で、そして衝撃的なほど愚かです。
It is an unavoidable side effect of teaching AI through brute force scale.
これは、ブルートフォーススケールでAIを教育する際の避けられない副作用です。
Some scale optimists might say, don't worry about this, all of this can be easily fixed, by adding similar examples as yet more training data.
スケールの楽観主義者の中には、「こんなことは気にしなくていい、似たような例をさらに訓練データとして追加すれば、すべて簡単に解決できる」と言う人もいるかもしれません。
But the real question is this.
しかし、本当の疑問はこうです。
Why should we even do that?
なぜそんなことをする必要があるのでしょうか?
You are able to get the correct answers right away without having to train yourself with similar examples.
似たような例で訓練しなくても、すぐに正解を導き出すことができるのです。
Children do not even read a trillion of words to acquire such basic level of common sense.
子どもたちは、そんな基本的なレベルの常識を身につけるために、1兆字も読まないのです。
So this observation leads us to the next wisdom, choose your battles.
この観察が、次の知恵である「戦いの選択」につながるわけです。
So what fundamental questions should we ask right now and tackle today in order to overcome this status quo with extreme-scale AI?
では、この現状を極限スケールAIで克服するために、私たちは今、どんな根本的な問いを立て、今日から取り組むべきでしょうか。
I would say common sense is among the top priorities.
私は、常識が最優先事項の一つであると言いたい。
So common sense has been a longstanding challenge in AI.
つまり常識は、AIにおける長年の課題だったのです。
To explain why, let me draw an analogy to dark matter.
その理由を説明するために、ダークマターに例えて説明します。
So only five percent of the universe is normal matter that you can see and interact with, and the remaining 95 percent is dark matter.
宇宙のうち、目に見えて対話できる通常の物質は5パーセントだけで、残りの95パーセントはダークマターなんですね。
The remaining 95 percent is dark matter and dark energy.
残りの95パーセントはダークマターとダークエネルギーです。
Dark matter is completely invisible, but scientists speculate that it's there because it influences the visible world, even including the trajectory of light.
ダークマターは完全に目に見えませんが、科学者たちは、光の軌跡を含む目に見える世界にまで影響を与えるからこそ、ダークマターが存在すると推測しています。
So for language, the normal matter is the visible text, and the dark matter is the unspoken rules about how the world works.
つまり、言語にとって、ノーマルマターは目に見える文章で、ダークマターは世界の仕組みに関する暗黙のルールということになりますね。
This includes nephrophysics and folk psychology, which influence the way people use and interpret language.
これには、人々の言語の使い方や解釈の仕方に影響を与える、ネフフィジックスや民間心理学も含まれます。
So why is common sense even important?
では、なぜ常識が重要なのでしょうか?
Well, in a famous thought experiment proposed by Nick Bostrom, AI was asked to produce and maximize paper clips.
ニック・ボストロムが提案した有名な思考実験では、AIがペーパークリップを生産し、最大化するよう依頼されました。
That AI decided to kill humans, to utilize them as additional resources to turn you into paper clips, because AI didn't have the basic human understanding about human values.
AIは人間を殺し、追加の資源として利用し、あなたたちをクリップに変えることにしたのですが、それはAIが人間の価値観についての基本的な理解を持っていなかったからです。
Adding a better objective and equation that explicitly states do not kill humans will not work either, because AI might go ahead and kill all the trees, thinking that's perfectly OK things to do.
人間を殺さないと明示的に記述したより良い目的と方程式を追加しても、AIは木をすべて殺すことが完全にOKだと考えてしまうかもしれないので、うまくいきません。
And in fact, there are endless other things that AI obviously shouldn't do while maximizing paper clips, including not spreading fake news, not stealing, and not lying.
実際、フェイクニュースを流さない、盗みをしない、嘘をつかないなど、AIがクリップを最大化する際に明らかにやってはいけないことは他にも無数にあるのです。
These are all part of our common-sense understanding about how the world works.
これらはすべて、世界がどのように機能しているかについての私たちの常識的な理解の一部なのです。
However, the AI field for decades has considered common sense as a nearly impossible challenge.
しかし、AIの分野では、何十年もの間、常識はほとんど不可能な挑戦だと考えられてきました。
So much so that when my students, colleagues, and I started working on it several years ago, we were very much discouraged.
それだけに、数年前に私の学生や同僚、そして私がこの課題に取り組み始めたときは、非常に落胆しました。
We've been told that it's a research topic of the 70s and 80s, shouldn't work on it because it will never work.
70年代や80年代の研究テーマだ、絶対にうまくいかないから取り組んではいけない、と言われてきたのです。
In fact, don't even say the word to be taken seriously.
実際、まともに相手にされるような言葉を口にするなということです。
Now, fast forward this year, I'm hearing, don't work on it because ChatGPT has almost solved it.
しかし、今年に入ってからは、ChatGPTがほぼ解決してしまったので、それに取り組んではいけないと言われるようになりました。
And just to scale things up and magic will arise and nothing else matters.
そして、ただ物事を拡大することで、魔法が発生し、他のことはどうでもよくなる。
So my position is that giving true common sense, human-like, robust common sense, to AI is a still moonshot.
ですから、私の立場は、AIに真の常識、人間のような堅牢な常識を与えることは、まだムーンショットであるということです。
And you don't reach to the moon by making the tallest building in the world one inch taller at a time.
世界一高いビルを1インチずつ高くしていっても、月に届くわけではありません。
Extreme-scale AI models do acquire an ever more increasing amount of common-sense knowledge, I'll give you that.
極端なスケールのAIモデルは、常識的な知識をどんどん獲得していくでしょう、それは認める。
But remember, they still stumble on such trivial problems that even children can do.
しかし、子供でもできるような些細な問題でつまずくこともある。
So AI today is awfully inefficient.
つまり、今のAIは非常に非効率的なのです。
And what if there's an alternative path, a path yet to be found, a path that can build on the advancement of deep neural networks but without going so extreme with the scale?
もし代替ルートがあり、まだ見つかっていない道があるとすれば、深層ニューラルネットワークの進歩を活かしつつ、スケールを極端に大きくしない方法はありますか?
So this leads us to our final wisdom, innovate your weapons.
そこで、最後の知恵として、「武器を革新せよ」ということになります。
In the modern-day AI context, that means innovate your data and algorithms.
現代のAIの文脈では、データとアルゴリズムを革新することを意味します。
OK, so there are, roughly speaking, three types of data that modern AI is trained on.
現代のAIが学習するデータには、大まかに言って3つのタイプがあります。
Raw web data, crafted examples, custom-developed for AI training, and then human judgments, also known as human feedback on AI performance.
生のウェブデータ、AIのトレーニング用にカスタム開発された細工された例、そして人間の判断、AIのパフォーマンスに対する人間のフィードバックとも呼ばれるものです。
If the AI is only trained on the first type, raw web data, which is freely available, it's not good because this data is loaded with racism and sexism and misinformation.
AIが最初のタイプにのみ、自由に利用できる生のウェブデータで訓練されている場合、それは良くありません。なぜなら、このデータには人種差別や性差別、誤情報が含まれているからです。
So no matter how much of it you use, garbage in and garbage out.
つまり、いくら使っても、ゴミが入り、ゴミが出る。
So the newest, greatest AI systems are now powered with the second and third types of data that are crafted and judged by human workers.
だから、最新の素晴らしいAIシステムには、人間の作業員が細工して判断する第2、第3のタイプのデータが搭載されるようになりました。
It's analogous to writing specialized textbooks for AI to study from and then hiring human tutors to give constant feedback to AI.
AIが勉強するための専門の教科書を書き、人間の家庭教師を雇ってAIに常にフィードバックを与えることに似ています。
These are proprietary data, by and large, speculated to cost tens of millions of dollars.
これらは大なり小なり、数千万円はかかると推測される独自データです。
We don't know what's in this, but it should be open and publicly available so that we can inspect and ensure to support diverse norms and values.
この中身はわかりませんが、多様な規範や価値観をサポートするために検査し確保できるよう、オープンで公開されるべきものです。
So for this reason, my teams at UW and AI, too, have been working on common-sense knowledge graphs as well as model norm repositories to teach AI basic common-sense norms and morals.
このため、UWとAIの私のチームは、基本的な常識的な規範や道徳をAIに教えるために、常識的な知識グラフやモデル規範リポジトリに取り組んできました。
Our data is fully open so that anybody can inspect the content and make corrections as needed, because transparency is the key for such an important research topic.
このような重要な研究テーマでは、透明性が重要なので、誰でも内容を確認し、必要に応じて修正できるように、データは完全にオープンにしています。
Now, let's think about learning algorithms.
さて、学習アルゴリズムについて考えてみましょう。
No matter how amazing large language models are, by design, they may not be the best suited to serve as reliable knowledge models.
いくら大規模な言語モデルが素晴らしくても、設計上、信頼できる知識モデルとしての役割を果たすには最適とは言えないかもしれません。
These language models do acquire a vast amount of knowledge, but they do so as a byproduct, as opposed to a direct learning objective.
言語モデルは膨大な量の知識を獲得しますが、それは直接的な学習目的とは異なり、副産物として行われます。
Resulting in unwanted side effects, such as hallucinated effects and lack of common sense.
その結果、幻覚症状や常識の欠如など、好ましくない副作用が生じる。
Now, in contrast, human learning is never about predicting which word comes next, but it's really about making sense of the world and learning how the world works.
これに対して、人間の学習は、次に来る言葉を予測することでは決してなく、世界を理解し、世界の仕組みを学ぶことが本当の意味での学習である。
Maybe AI should be taught that way as well.
AIもそのように教えるべきかもしれません。
So, as a quest towards more direct common-sense knowledge acquisition, my team has been investigating potential new algorithms, including symbolic knowledge distillation.
そこで、より直接的な常識的知識の習得を目指し、私のチームでは、記号的知識の抽出など、新しいアルゴリズムの可能性を調査しています。
This can take very large language models, as shown here, that I couldn't fit into the screen because it's too large, and crunch that down to much smaller common-sense models using deep neural networks.
これは、ここで示されているように、非常に大きな言語モデルを使用して、画面に収まらないほど大きいものを、深層ニューラルネットワークを使ってはるかに小さな常識モデルに縮小することができます。
In doing so, we also generate algorithmically human-inspectable symbolic common-sense knowledge representation.
その際、アルゴリズム的に人間が点検可能な記号的な常識的知識表現も生成しています。
This allows people to inspect, make corrections, and even use it to train other neural common-sense models.
これによって、人が検査し、修正し、さらに他のニューラル常識モデルの訓練に使うことができるようになります。
More broadly, we have been tackling this seemingly impossible, giant puzzle of common sense, ranging from physical, social and visual common sense to theory of minds, norms and morals.
より広い意味で、私たちは物理的、社会的、視覚的な常識から、心の理論、規範、道徳に至るまで、常識という巨大で不可能に思われるパズルに取り組んできました。
Each individual piece may seem quirky and incomplete, but when you step back, it's almost as if these pieces weave together into a tapestry that we call as human experience and common sense.
個々のピースは風変わりで不完全に見えるかもしれませんが、一歩引いてみると、これらのピースが人間の経験と常識と呼ばれるタペストリーに織り込まれているかのようです。
We're now entering a new era in which AI is almost like a new intellectual species, with unique strengths and weaknesses compared to humans.
私たちは今、AIが人間と比べてユニークな長所と短所を持つ、新しい知的種族のようなものであるという新しい時代に突入しています。
In order to make this powerful AI sustainable and humanistic, we need to teach AI common sense, norms and values.
この強力なAIを持続可能で人間らしいものにするためには、AIに常識や規範、価値観を教える必要があるのです。
Thank you.
ありがとうございます。
Look at that.
あれを見てください。
Yecine, please stay one sec.
イエシン、ちょっとだけ待ってて。
This is so interesting, this idea of common sense.
この常識という考え方はとても興味深いですね。
We obviously all really want this from whatever's coming.
私たちは明らかに、これから来るものに対して、これを本当に望んでいます。
But help me understand.
しかし、理解するのを助けてください。
So we've had this model of a child learning.
私たちは、子供が学ぶというモデルを持っています。
How does a child gain common sense apart from the accumulation of more input and some human feedback?
より多くのインプットと人からのフィードバックの積み重ね以外に、子どもはどうやって常識を身につけるのでしょうか?
What else is there?
それ以外に何があるのでしょうか?
So fundamentally, there are several things missing, but one of them is, for example, the ability to make hypotheses and make experiments, interact with the world and develop these hypotheses.
根本的には、いくつかのことが欠けていますが、そのうちの一つは、例えば、仮説を立てたり実験を行ったり、世界と関わりながら仮説を発展させる能力です。
We abstract away the concepts about how the world works, and then that's how we truly learn, as opposed to today's language model.
今日の言語モデルとは対照的に、私たちは世界の仕組みに関する概念を抽象化し、それが本当の意味での学習方法となるのです。
we abstract away the concepts about how the world works, as opposed to today's language model.
今日の言語モデルとは対照的に、私たちは、世界がどのように機能しているかについての概念を抽象化します。
we abstract away the concepts about how the world works, any way of combining what you're doing with what they have built.
今日の言語モデルとは対照的に、私たちは、世界がどのように機能するかについての概念を抽象化します。
Certainly, what I envision will need to build on the advancements of deep neural networks.
確かに、私が想定しているのは、ディープニューラルネットワークの進化をベースにすることです。
It might be that there's some scale Goldilocks zone such that I'm not imagining that smaller is always better, by the way.
もしかしたら、「小さければいい」というわけではないのですが、ゴルディロックス・ゾーンのような規模があるのかもしれません。
It's likely that there's the right amount of scale, but beyond that, the winning recipe might be something else.
規模が大きければいいというものでもないでしょうが、それ以上の規模になると、勝てるレシピは別のものになるかもしれません。
So some synthesis of ideas will be critical here.
だから、ここではアイデアの統合が重要なのです。
Yijin Choi, thank you so much for your talk.
Yijin Choi、ご講演ありがとうございました。
Thank you.
ありがとうございました。
この記事が気に入ったらサポートをしてみませんか?