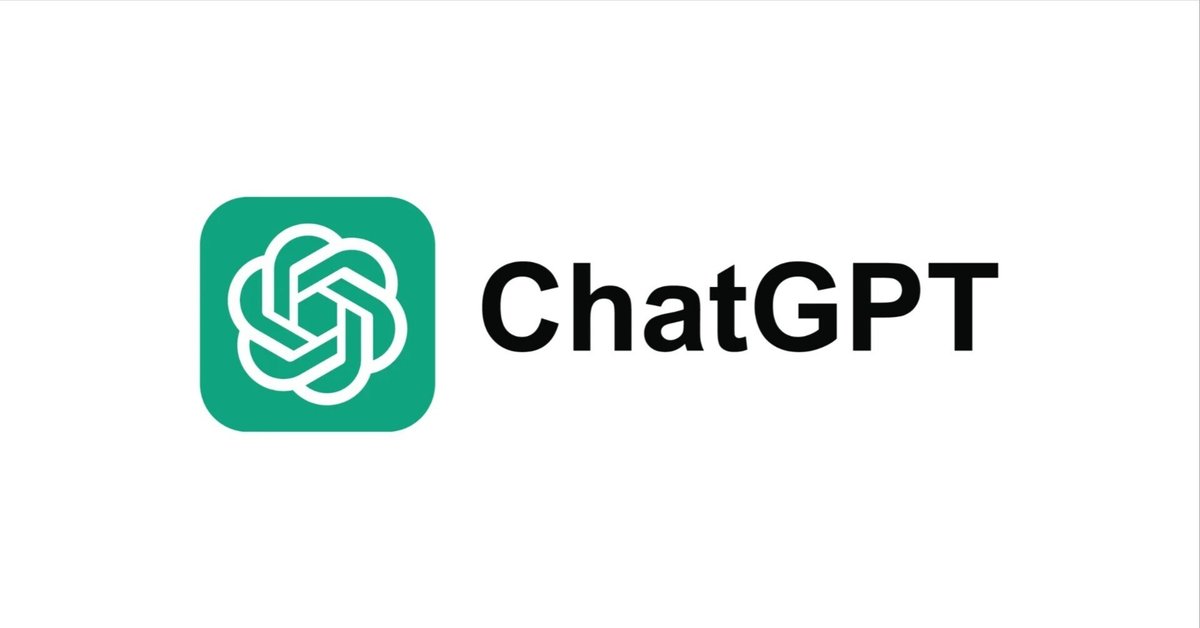
【ChatGPT】英語解説を日本語で読む【2023年4月22日|@PensionCraft】
ChatGPTの解説です。
公開日:2023年4月22日
※動画を再生してから、インタビューを読むのがオススメです。
The uses of large language models like ChatGPT are exploding, and it seems like we see new use cases every day.
ChatGPTのような大規模な言語モデルの用途は爆発的に広がっており、毎日新しいユースケースを目にするような気がします。
But as investors, the key question is whether we can use these models to improve our returns.
しかし、投資家として重要なのは、これらのモデルを利用してリターンを向上させることができるかどうかということです。
In this video, we look at some recent research that's been published, which has some very interesting results in that regard, and we'll also consider whether we can practically apply this to our own portfolios.
このビデオでは、この点に関して非常に興味深い結果が発表された最近の研究を見て、これを自分のポートフォリオに実際に適用できるかどうかも考えてみます。
If you do enjoy our content, don't forget, please do subscribe to our channel.
もし私たちのコンテンツを楽しんでいただけたら、忘れずにチャンネル登録をお願いします。
That way, you won't miss any new content, and you could also click on like for this video.
そうすれば、新しいコンテンツを見逃すことはありませんし、このビデオの「いいね!」をクリックすることもできます。
So let's look at whether ChatGPT can be used to forecast stock returns in a bit more detail.
それでは、ChatGPTが株式リターンの予測に使えるかどうか、もう少し詳しく見ていきましょう。
Now what we'll be talking about is sentiment analysis, and the basic idea here is that we take news headlines.
これからお話しするのはセンチメント分析で、基本的な考え方は、ニュースのヘッドラインを取り上げることです。
These could come from any kind of news service, and then we feed those news headlines into some kind of model.
ニュースの見出しはどのようなニュースサービスからでも構いませんが、その見出しをある種のモデルに送り込みます。
That model then converts that news headline into a buy or sell signal, depending on whether it thinks it's a positive news story or a negative news story.
このモデルは、ニュースの見出しをポジティブなニュースかネガティブなニュースかによって、買いシグナルまたは売りシグナルに変換します。
And if the models write about the sentiment and it captures the market reaction properly, that's the key thing.
そして、モデルがセンチメントを書き、それが市場の反応を適切に捉えることができれば、それが重要なポイントになります。
Then we could actually make money based on those recommendations.
そうすれば、その推奨に基づいて実際にお金を稼ぐことができるのです。
What do these models look like?
このようなモデルはどのようなものでしょうか?
Well, some of them have been around for some time.
いくつかのモデルは以前から存在しています。
One example that you can see beside me here is from Raven pack, and what that tries to do is to take a huge amount of unstructured data, so this could come from things like news flow, but also social media, textual data, transcripts from earnings reports, and it turns those into a sentiment analysis which hopefully gives you a signal as to whether to buy or sell a stock.
この横にあるのはRaven Packの例ですが、これは膨大な量の非構造化データ(ニュースフロー、ソーシャルメディア、テキストデータ、決算報告書のトランスクリプトなど)を取り込み、それらをセンチメント分析に変換して、株の売買のシグナルを与えるというものです。
Now Raven's pack analysis is very much crafted to work only for financial data.
Ravenのパック分析は、金融データのみに対応するように非常によくできています。
Large language models, on the other hand, are kind of general learning models which are unsupervised.
一方、大規模言語モデルは、教師なしで学習する一般的なモデルです。
You just show them a set of data, and then they can learn to produce the right outputs based on a prompt which you give them.
データセットを見せるだけで、与えられたプロンプトに基づいて正しい出力を生成するように学習することができます。
Now, recently those have exploded in complexity, and one measure of that complexity is the number of parameters in the model.
最近、これらのモデルは爆発的に複雑化しており、その複雑さを表す一つの指標として、モデルのパラメータ数が挙げられます。
Now in 2018, the state-of-the-art model was from Google AI, and that was BERT LARGE.
2018年、最先端のモデルはGoogle AIによるもので、「BERT LARGE」でした。
That had 340 million parameters in the model, and the competitors with that model would come from OpenAI.
あれはモデルのパラメータが3億4千万もあり、そのモデルを持つ競合はOpenAIからやってくることになります。
So the first version of GPT, that was the Generative P-Train Transformer model, had 110 million parameters.
GPTの最初のバージョン、つまりGenerative P-Train Transformerモデルは、1億1000万個のパラメーターを持っていました。
GPT-2 had 1.5 billion parameters.
GPT-2は15億のパラメータを持っていました。
So as you add complexity to these models, their analysis becomes more sophisticated, and they can pick up more long-term relationships between words in natural language.
つまり、モデルに複雑性を持たせることで、分析がより洗練され、自然言語における単語間の長期的な関係をより多く拾い上げることができるようになるのです。
And if you're wondering, BERT stands for bi-directional encoder representations from transformers, hence the acronyms.
また、バートとは、トランスフォーマーによる双方向エンコーダ表現の略で、このような頭文字をとっています。
Now the prompts that you feed into these large language models are absolutely critical.
このような大規模な言語モデルに入力するプロンプトは、非常に重要です。
So for example, here you can see I'm using OpenAI's ChatGPT, and I told it to write a short limerick about active versus passive investing.
例えば、ここではOpenAIのChatGPTを使っていますが、アクティブ投資とパッシブ投資に関する短いリメリックを書くように指示しました。
Now you can see it's done pretty well.
これで、かなりうまくいったことがお分かりいただけると思います。
It's picked up the rhyming scheme, so it goes A-A-B-B-A. But what's a bit wonky about this, if you try reading it out to yourself, is that it doesn't have the right number of syllables.
韻を踏んでいるので、A-A-B-B-Aとなっています。しかし、この文章を自分で読んでみると、音節の数が適切でないことがわかります。
Particularly on line number four, it's got 11 syllables which just sounds really awkward.
特に4行目では、11音節になってしまっていて、とても不自然に聞こえます。
So I tried to correct it by telling it how many syllables should be in each line, and you can see it still didn't really figure it out.
そこで、各行の音節数を指示して修正してみましたが、それでもまだよくわからないことがわかります。
So because this is a general language model, it's not tuned for a particular thing like limericks.
つまり、これは一般的な言語モデルであり、叙情詩のような特定のものに対してチューニングされているわけではありません。
It doesn't perform incredibly well on every task.
また、すべてのタスクで非常に優れた性能を発揮するわけではありません。
It does pretty well on a very broad range of tasks.
しかし、非常に広範なタスクでうまく機能します。
Don't get me wrong, it is incredible.
しかし、誤解を恐れずに言えば、これはすごいことなのです。
So here I told it to write an R script to convert a zoo time series from prices to returns, and it did so, and the script actually works.
そこで、動物園の時系列を価格からリターンに変換するRスクリプトを書くように指示したところ、そのスクリプトは実際に動作したのです。
Not only that, but it actually came up with an explanation of how the code works.
それだけでなく、そのスクリプトがどのように機能するかについての説明も書いてくれました。
So these models are now incredibly sophisticated, but they still do sometimes go wrong.
このように、モデルは非常に洗練されたものになっていますが、それでも時々うまくいかないことがあります。
But the key thing is whether you can craft a prompt to produce the right output, and in fact, there's a whole new job category, prompt engineer, in crafting those prompts for these large language models.
しかし、重要なのは、正しい出力を生み出すプロンプトを作成できるかどうかです。実際、このような大規模な言語モデルのプロンプトを作成するプロンプトエンジニアという新しい職種が存在するのです。
So how can these large language models be used to do sentiment analysis for stocks?
では、このような大規模言語モデルを用いて、株式のセンチメント分析を行うにはどうしたらよいのでしょうか。
The results I'm about to show you are from this paper which has been published in April 2023, and what it does is to compare predictive accuracy for various models, and that includes the RavenPack news analytics tool which we just saw, but also increasingly complex versions of GPT going from GPT 1 to GPT 2 and ChatGPT which uses the GPT 3.5 version, but also two flavors of the BERT model.
これからお見せする結果は、2023年4月に発表されたこの論文によるものです。この論文では、様々なモデルの予測精度を比較しています。その中には、先ほど見たRavenPackニュース分析ツール、GPT1、GPT2、GPT3.5バージョンを使用するChatGPT、さらにBERTモデルの2種類のバージョンと、ますます複雑になっていくGPTがあります。
Now the approach is not to feed a whole news story about a stock to the model, but instead just to focus on news headlines.
現在では、銘柄に関するニュース記事全体をモデルに供給するのではなく、ニュースのヘッドラインにのみ焦点を当てるというアプローチがとられています。
Those are presented to the model using a very standardized prompt as we'll see, and then it has to figure out whether this headline is positive or negative for stock sentiment.
この見出しは、これから説明するように、非常に標準化されたプロンプトを使ってモデルに提示され、この見出しが株価のセンチメントにとってポジティブかネガティブかを判断する必要があるのです。
Now I think it's useful to look at an example headline, one which they give in the paper, which is looking at a story about Oracle, the database provider, and a less well-known company which is Rimini Street.
新聞に掲載されている見出しの例を見てみましょう。データベースプロバイダーのオラクルと、あまり知られていないリミニストリートという会社についての記事です。
Now Rimini Street provides support products and services around these software products from Oracle and SAP, and in this example, we'll be looking at this headline and here's the entire story that goes with it.
リミニストリートは、オラクルやSAPのソフトウェア製品に関するサポート製品やサービスを提供しています。この例では、このヘッドラインとそれに付随するストーリー全体を見てみましょう。
Now really it was a question about intellectual property which allegedly Rimini had leaked to other clients and which belonged to Oracle.
リミニが他のクライアントに漏らしたとされる知的財産が、オラクルに帰属していたことが問題視されたのです。
And Oracle managed to get a $630,000 fine against Rimini Street in court.
そして、オラクルは裁判でリミニストリートに対して63万ドルの罰金を科すことに成功しました。
So personally, I think that this would be positive for Oracle.
ですから、個人的には、これはオラクルにとってプラスになるのではないかと思っています。
So the standardized prompt that the research paper used to feed these headlines to the model is as follows.
そこで、研究論文がこれらのヘッドラインをモデルに送り込むために用いた標準化プロンプトは、次のようなものです。
Firstly, it tells the model to forget all of your previous instructions.
まず、モデルに「これまでの指示をすべて忘れろ」と指示します。
Presumably, that wipes the slate clean in terms of previous prompts.
おそらく、これまでのプロンプトを白紙に戻すのだろう。
Then it says pretend you're a financial expert.
そして、「あなたは金融の専門家です」と言います。
Not only that, but you're a financial expert with stock recommendation experience.
それだけでなく、あなたは株の推奨経験を持つ金融の専門家です。
And then it tells ChatGPT how to respond to the headline.
そして、ChatGPTに見出しにどう答えるかを指示します。
In the first line of the response, it either says yes, no, or unknown, according to whether the headline is good news for the stock, bad news for the stock, or uncertain.
最初の行で、その見出しが株にとって良いニュースなのか、悪いニュースなのか、不確かなものなのかによって、「はい」「いいえ」「不明」のいずれかを答えます。
Then, after that one-word, one-line response, it asks the model to elaborate on its answer, and it has to do that with a short and concise sentence on the next line, explaining its decision.
そして、その1語1行の回答の後に、その回答を詳しく説明するようモデルに求めます。その際、次の行に短く簡潔な文章で、その判断を説明する必要があります。
And then we tell the model which stock we're talking about.
そして、どの銘柄についての話なのかをモデルに伝えます。
So, in this case, is the headline good or bad for the stock price of Oracle, and we also specify whether it's over the short, medium, or long term.
つまり、この場合、ヘッドラインはオラクルの株価にとって良いのか悪いのか、そして、それが短期、中期、長期のどれに当たるのかも指定します。
In this case, it's over the short term.
この場合、短期的なものです。
And then finally, the model's given the actual headline.
そして最後に、実際のヘッドラインがモデルに与えられます。
So, the bits in red are the bits which are substituted for different stocks, for different terms, and for different headlines.
つまり、赤字の部分は、銘柄や用語、見出しの違いによって置き換えられる部分です。
But all of the rest of the text, which is in white, would be the same for every stock and for every headline.
しかし、白で書かれた残りのテキストは、どの銘柄でもどの見出しでも同じです。
So here's ChatGPT's response to that prompt: The answer is yes.
では、そのプロンプトに対するChatGPTの回答です: 答えは「イエス」です。
In other words, the headline is good for the stock, and its explanation is actually quite sophisticated.
つまり、この見出しはその銘柄にとって良い見出しであり、その説明は実は非常に洗練されています。
It's actually saying that the fine against Rimini Street could boost investor confidence because it shows Oracle can protect its intellectual property, and that indirectly could increase demand for Oracle's products and services.
つまり、Rimini Streetに対する罰金は、Oracleが知的財産を保護できることを示すため、投資家の信頼を高め、間接的にOracleの製品とサービスに対する需要を高める可能性があるということです。
And in fact, I think that's quite a good answer.
そして実際、これは非常に良い回答だと思います。
Compare that with a response from Ravenpack, which came up with a minus 0.52 negative sentiment for Oracle.
Ravenpackの回答を見てみると、オラクルに対するネガティブな感情はマイナス0.52となりました。
So, you can see that the ability of the language model to infer interesting things, relationships between the words in the headline, allows it to see through the immediate problem, which is that this is a court settlement, but to see that that could be construed as positive for the stock.
つまり、言語モデルが見出しの単語間の関係という興味深いものを推論する能力によって、これが法廷での和解であるという直接的な問題を見抜き、それが株価にとってプラスに解釈される可能性があることを見抜くことができるのです。
Now, if you're enjoying this video and you want to learn more about investing, remember we do offer a membership where you can join our community and learn with like-minded investors online.
このビデオをご覧になり、投資についてもっと学びたいと思われたなら、私たちのコミュニティに参加して、同じ志を持つ投資家たちとオンラインで学ぶことができるメンバーシップを提供していることを思い出してください。
It's very friendly.
とてもフレンドリーです。
There's lots of members-only content, members-only videos, and you get to chat to each other using a chat application and ask questions anytime you like.
会員限定のコンテンツや会員限定のビデオもたくさんありますし、チャットアプリケーションを使ってお互いにチャットしたり、いつでも好きなときに質問したりすることができます。
Now, if you do want to learn more about that, just click on the link in the description beneath me or just go to pensioncraft.com.
もっと詳しく知りたい方は、私の下にある説明のリンクをクリックするか、pensioncraft.comにアクセスしてみてください。
So, how well did the models perform?
さて、モデルの性能はどうだったのでしょうか?
Well, whenever you're measuring performance, you have to be very careful that you do things out of sample.
さて、パフォーマンスを測定するときはいつも、サンプルの範囲外で行うことに十分注意しなければなりません。
Otherwise, the model could actually know what happens to the stock price.
そうでなければ、株価がどうなったかをモデルが実際に知ってしまう可能性があるからです。
Now, fortunately, the version of ChatGPT which the researchers used had no training after the date of September 2021.
さて、幸運なことに、研究者が使用したChatGPTのバージョンには、2021年9月以降のトレーニングがありませんでした。
So, by only using headlines from October 2021 to December 2022, the researchers could ensure that the model wouldn't have access to the answer.
そのため、2021年10月から2022年12月までのヘッドラインのみを使用することで、モデルが答えを知ることができないようにすることができました。
And in terms of the stocks which they used, they had to have a news story published about them, a headline which they took from that Raven Pack Dow Jones news story feed, and they used stocks from the US exchanges.
また、使用する銘柄については、その銘柄に関するニュース記事が掲載され、その見出しがRaven Pack Dow Jonesのニュース記事フィードから取得されている必要があり、米国の取引所の銘柄を使用しました。
So, that's the New York Stock Exchange, NASDAQ, and the American Exchange.
つまり、ニューヨーク証券取引所、ナスダック、アメリカン証券取引所です。
Now let's assume that we had an absolutely perfect model.
ここで、完全に完璧なモデルがあったと仮定しましょう。
What would we expect it to look like?
どのようなモデルになるのでしょうか?
Well, beside me here, you can see a distribution of returns chopped up into two buckets.
私の横には、2つのバケツに分けられたリターンの分布が見えます。
So let's say we receive a positive headline about a stock, as judged by the model, and that would go into the good news category.
例えば、モデルによって判断されたある銘柄のポジティブな見出しを受け取ったとします。これはグッドニュースのカテゴリーに入ります。
Well, if the model was absolutely perfect, then on the following day, the return would always be positive if it was right about the good news story, and conversely, if it thought it was a bad news story, then it would have 100% accuracy in predicting negative returns.
もしモデルが完璧であれば、翌日のリターンは、良いニュースが正しければ必ずプラスになり、逆に悪いニュースだと思えば、100%の精度でマイナスのリターンを予測することになります。
Of course, in the real world, it never looks like that.
もちろん、現実の世界では、決してそんなことはありません。
In reality, you'd have something like this that would also be a fantastic model because notice that the average return on good news is higher than the average return on bad news.
なぜなら、良いニュースの平均リターンは、悪いニュースの平均リターンよりも高いからです。
That's the best that we can really hope for.
これが、私たちが本当に望むことのできる最高の状態です。
There'll always be some false positives and false negatives, and this is what the results looked like.
偽陽性と偽陰性は常に存在しますし、結果はこのようなものでした。
So we've got each of the models in the columns here.
ここに各モデルを列挙しています。
We've got GPT, ChatGPT, the most sophisticated GPT model.
GPT、ChatGPT、最も洗練されたGPTモデルです。
We've got the more basic versions of GPT - that's GPT-1, GPT-2.
GPTの基本バージョンであるGPT-1、GPT-2があります。
We've got the BERT model and we've got Raven.
BERTモデルもありますし、Ravenもあります。
So what this score table does is it looks to see whether the model said that there was good news, unknown or bad news and then looks at the average return on the following day, and that's for the stock that was in the prompt.
このスコア・テーブルでは、モデルが「良いニュース」「不明なニュース」「悪いニュース」のいずれを伝えたかを調べ、翌日の平均リターンを調べますが、これはプロンプトに表示された銘柄のものです。
Notice that for GPT, when there was a good news story, the average return was plus 0.13 percent.
GPTの場合、良いニュースがあったときの平均リターンはプラス0.13%であることに注目してください。
When there was a bad news story, it was minus 0.13 percent.
悪いニュースがあったときは、マイナス0.13%でした。
So it did that job of separating the good and bad returns and interpreting sentiment.
つまり、良いリターンと悪いリターンを分離し、センチメントを解釈する役割を果たしたのです。
However, if you look at any of the other models, they do a very poor job.
しかし、他のモデルを見ると、非常に悪い仕事しかしていません。
The average returns for GPT-1 and GPT-2 were quite similar, depending on whether it was good news or bad news according to the model.
GPT-1とGPT-2の平均リターンは、モデルによると良いニュースか悪いニュースかによって、かなり似ていました。
So what this shows is that the more sophisticated GPT model with more parameters and more inferential ability could actually do a pretty good job of discriminating positive and negative sentiment from headlines.
つまり、より多くのパラメータと推論能力を持つより洗練されたGPTモデルは、ヘッドラインからポジティブな感情とネガティブな感情を識別するために、実際にはかなり良い仕事をすることができるということを示しているのです。
If we actually implement that into a trading strategy - and the authors aren't very clear on how they generated these lines - what they do is they take all of the stocks for which there was a headline.
実際にこれを取引戦略に導入するとしたら、著者らはこの線をどのように生成したのかあまり明確にしていませんが、何をするかというと、ヘッドラインがあった銘柄をすべて取り上げるのです。
If the model thinks the headline is positive, then they buy that stock, hold it to the next day, and then sell.
ヘッドラインがポジティブだとモデルが判断した場合、その銘柄を買い、翌日まで保有し、その後売却します。
If the model, on the other hand, thinks the news is negative, then they'd short the stock which profits from a fall and then close out the short the next day.
一方、ネガティブなニュースだと判断した場合は、下落によって利益を得る銘柄を空売りし、翌日には空売りを解消します。
So in other words, they'd have a long-short portfolio which is regenerated every day, and they'd simply repeat that over this period, and you can see the long-short strategy does very well.
つまり、毎日再生されるロングショートのポートフォリオがあり、それをこの期間繰り返すだけで、ロングショート戦略が非常にうまくいっていることがわかるのです。
If you have a short-only strategy where they simply short the stocks where there's a negative sentiment story according to the model, again, the performance there is quite good, but notice that the performance is not steady, so there was a period of outperformance at the beginning of the period, then the results were kind of flat, didn't really go anywhere, and then outperformance again at the end of the period.
モデルによると、株価に対してネガティブなセンチメントがある銘柄をショートするだけの戦略をお持ちでしたら、そのパフォーマンスはかなり良いですが、ご注意いただきたいのは、そのパフォーマンスが安定していないということです。期間の初めには、優れたパフォーマンスがありましたが、その後、結果はあまり変わらず、平坦でした。そして、期間の終わりに再び優れたパフォーマンスが現れました。
There's also a long-only strategy, which is for stocks that had good news which they bought, and I'm not clear what all news is - they didn't explain that in the paper - but this was a period when stocks were falling in 2022.
また、ロングオンリー戦略もあり、これは良いニュースがあった銘柄を買うというもので、全てのニュースが何なのかは不明ですが、論文では説明されていませんでしたが、これは2022年に株価が下落していた時期です。
So the fact that it did generate a positive return is actually quite interesting.
しかし、これは2022年に株価が下落していた時期のことで、プラスのリターンが得られたという事実は、実に興味深いものです。
Notice, however, that this is without transaction costs, and as we'll see later, that's very significant.
しかし、これは取引コストを除いた場合であり、後で見るように、これは非常に重要なことです。
So the authors conclude that the superiority of ChatGPT in predicting stock market returns can be attributed to its advanced language understanding capabilities, which allow it to capture the nuances and subtleties within news headlines.
つまり、ChatGPTが株式市場のリターンを予測するのに優れているのは、高度な言語理解能力によって、ニュースの見出しに含まれるニュアンスや微妙なニュアンスを捉えることができるからだと著者たちは結論付けています。
But I think the more interesting point here is that it actually interprets things in the same way as market participants because remember that's the goal here.
しかし、より興味深いのは、ChatGPTが市場参加者と同じように物事を解釈しているという点です。
In order to predict what the stock market does, you have to get
株式市場の動きを予測するためには、次のようなことが必要です。
But I think the more interesting point here is that it actually interprets things in the same way as market participants, because remember, that's the goal here.
しかし、ここでもっと興味深いのは、実際に市場参加者と同じように物事を解釈している点だと思います。
In order to predict what the stock market does, you have to get into the mind of investors, so I think it's truly remarkable that the model can do that, particularly as it's not specifically trained to do so.
株式市場の動きを予測するためには、投資家の心理に入り込む必要があります。このモデルがそれを可能にしたことは、本当に驚くべきことだと思います。
It is a general language learning model, but their conclusion is that this enables the model to generate more reliable sentiment scores, leading to better predictions of daily stock market returns.
一般的な言語学習モデルですが、その結果、より信頼性の高いセンチメントスコアを生成できるようになり、日々の株式市場のリターンをより良く予測できるようになったというのが、彼らの結論です。
So you're probably thinking, "Can I make money with this?"
だから、"これで儲けられるのか?"と思っていることでしょう。
But personally, I think it would be impractical with the model as it's implemented here, and that's because of churn.
しかし、個人的には、今回実装されたモデルでは現実的ではないと思います。
Remember what the model was doing: it was looking at a headline today, deciding whether to buy or sell, and then selling or closing out a short the following day.
このモデルは、今日のヘッドラインを見て、買うか売るかを決め、翌日には売るか空売りを解消する、ということをやっていました。
So there could be a huge turnover of stock in this portfolio.
ですから、このポートフォリオでは、株式の入れ替わりが激しくなる可能性があるのです。
Now, of course, you could restrict the number of stocks you apply this to, and there are various workarounds, but that churn, the bid-off has spread, is going to eat a large amount of the profits for this strategy.
もちろん、これを適用する銘柄を制限することもできますし、さまざまな回避策がありますが、この回転、つまりビッドオフが広がっていることが、この戦略の利益の大部分を食ってしまうことになるのです。
And if you remember, the outperformance came in two fairly concentrated bursts at the beginning and the end of the period.
そして思い出してみてください、アウトパフォームは期初と期末の2回、かなり集中したバーストで発生しました。
So if this thing doesn't outperform, you'll still be continually churning and losing money on the bid-off of spread.
つまり、もしこれがアウトパフォームしなかったとしても、スプレッドのビッドオフで継続的にチャーンして損をすることになるのです。
Also, the strategy which worked best was a long short strategy, which is kind of like a market neutral hedge fund strategy.
また、最もうまくいった戦略はロング・ショート戦略で、これはマーケット・ニュートラルなヘッジファンド戦略のようなものです。
So for a hedge fund to implement this could be practical, I think, because they can go short quite easily on single stocks.
ヘッジファンドがこれを実行するのは、単一銘柄のショートをかなり簡単に行えるので、実用的だと思います。
For retail investors, it's not easy to do that.
しかし、個人投資家の場合、それを実行するのは容易ではありません。
So we'd have to probably go for the long only strategy, which, if you remember, didn't perform as well.
そのため、ロングオンリーの戦略を取ることになるのですが、これはあまり良いパフォーマンスではなかったことを覚えています。
Another problem is the fact that everybody now knows about this model and how well it performs.
もう一つの問題は、このモデルについて、そしてそのパフォーマンスの高さについて、今や誰もが知っているという事実です。
So alpha, remember, is outperformance if you can beat the market due to some special insight into the market.
アルファとは、市場に対する特別な洞察力によって市場を打ち負かすことができる場合のアウトパフォームのことです。
And this is an insight which anybody can buy from OpenAI very cheaply.
そしてこの洞察は、誰でもOpenAIから非常に安く買うことができます。
So this thing is now in the hands of investors almost everywhere.
つまり、これは今やほとんどすべての投資家の手中にあるのです。
And what usually happens when news spreads about a strategy is that the performance of that strategy starts to diminish.
そして、ある戦略についてニュースが広まると、通常、その戦略のパフォーマンスが低下し始めるのです。
That's because the trades start to get crowded, everyone does the same thing, and some people will do it more quickly than others, so they'll probably eat their lunch.
それは、取引が混雑し始め、皆が同じことをし、ある人は他の人よりも早くそれを行うので、おそらくランチを食べることになるからです。
So this gradual diminishing of the outperformance is called alpha decay, and it's something that hedge funds are used to all the time.
このようにアウトパフォームが徐々に低下していくことをアルファ・ディケイと呼びますが、これはヘッジファンドがいつも使っていることです。
If they come up with a great strategy, gradually the outperformance of that strategy often wanes with time, and so they have to come up with a new strategy.
もし素晴らしい戦略を思いついたとしても、時間とともにその戦略のアウトパフォームが徐々に低下していくことが多いので、新しい戦略を考えなければならないのです。
So personally, I'm not convinced that this thing is going to work for retail investors such as you and I. If anybody's going to monetize it, it'll probably be hedge funds which have lots of resources they can throw at this problem, and they're probably doing this kind of thing anyway.
ですので、個人的には、この戦略が私たちのような個人投資家にとってうまくいくとは確信が持てません。もし誰かがこれを利益につなげるとしたら、おそらく多くのリソースをこの問題に投入できるヘッジファンドでしょう。彼らは、おそらくすでにこのようなことを行っているでしょう。
So I think the results of the paper are really fascinating because they show what the model can do is get inside the mind of investors and predict what this sentiment will be in response to a headline in the same way as a human would.
この論文では、モデルが投資家の心の中に入り込み、人間がするのと同じように、ヘッドラインに対してどのような感情を抱くかを予測することができることを示しており、本当に魅力的だと思います。
Now, that is truly remarkable.
これは、本当に驚くべきことです。
In terms of practical implementation of the strategy, I think it's not really practical for retail investors.
この戦略の実用化という点では、個人投資家にとってはあまり実用的ではないと思います。
Maybe you could use it if you have variations on it, or you could use macroeconomic information which you'd feed to the model for longer term forecasts, but I don't think it's practical as it's presented in this paper.
バリエーションがあれば使えるかもしれませんし、マクロ経済情報を使って長期的な予測をモデルに与えることもできますが、この論文で紹介されているような実用性はないと思います。
Now, don't forget, our offer: if you do want to join our membership and get access to all of the goodies like our trackers to track macroeconomic variables but also market variables, and also members-only videos and be able to chat to other members of the community, then just look at the description in the link below or go to pensioncraft.com.
もし、あなたがメンバーシップに参加して、マクロ経済変数だけでなく市場変数も追跡できるトラッカーや、メンバー限定のビデオ、コミュニティの他のメンバーとのチャットなど、すべての特典にアクセスしたい場合は、以下のリンクの説明を見るか、pensioncraft.comにアクセスしてください。
And as always, thank you for listening.
また、いつもご清聴ありがとうございます。
この記事が気に入ったらサポートをしてみませんか?