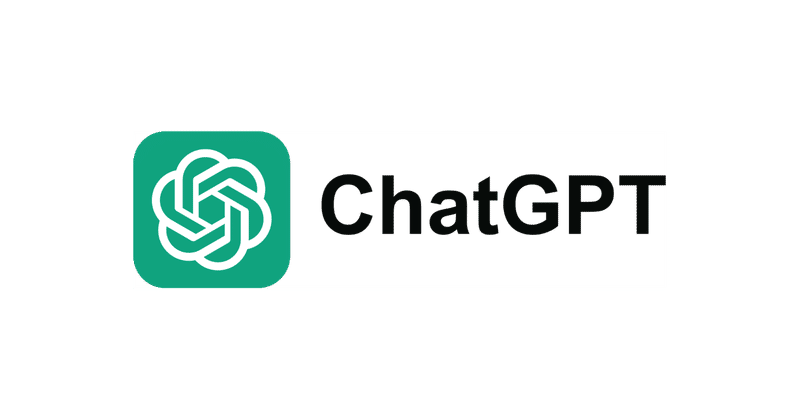
【ChatGPT】英語解説を日本語で読む【2023年4月22日|@Lex Clips】
ChatGPTの解説です。
公開日:2023年4月22日
※動画を再生してから、インタビューを読むのがオススメです。
So, well before ChatGPT, I would tell my students, just ask what would Manoli say right now.
だから、ChatGPTの前に、生徒たちに「マノリは今、何を言っているんだろう」と聞いていたんです。
And you guys all have a pretty good emulator of me right now.
皆さんは今、私の良いエミュレーターを持っていますね。
And I don't know if you know the programming paradigm of the rubber duckling, where you basically explain to the rubber duckling that's just sitting there exactly what you did with your code and why you have a bug.
ラバーダックリングというプログラミングのパラダイムをご存じでしょうか、そこに座っているラバーダックリングに、自分のコードで何をしたのか、なぜバグがあるのかを説明するのです。
And just by the act of explaining, you'll kind of figure it out.
そうすれば、説明することで、なんとなくわかってくるものです。
I woke up one morning from a dream where I was giving a lecture in this amphitheater and one of my friends was basically giving me some deep evolutionary insight on how cancer genomes and cancer cells evolve.
ある朝、私は夢から目覚めました。円形劇場で講義をしていて、友人の一人が、がんゲノムやがん細胞がどのように進化していくかについて、進化論的な深い洞察を私に教えてくれるのです。
And I woke up with a very elaborate discussion that I was giving and a very elaborate set of insights that he had that I was projecting onto my friend in my sleep.
そして私は、私が行っていた非常に精巧な議論と、彼が持っていた非常に精巧な洞察のセットを、寝ている間に友人に投影している状態で目を覚ましました。
And obviously this was my dream, so my own neurons were capable of doing that.
これは明らかに私の夢でしたから、私自身のニューロンにはその能力がありました。
But they only did that under the prompt of, you are now Piyush Gupta, you are a professor in cancer genomics, you're an expert in that field, what do you say?
しかし、「あなたは今、ピユシュ・グプタで、がんゲノム学の教授で、その分野の専門家です、何を言っているのですか?
So I feel that we all have that inside us, that we have that capability of basically saying, I don't know what the right thing is, but let me ask my virtual ex, what would you do?
私たちは皆、自分の中にそういう能力を持っていて、「何が正しいかわからないけど、仮想の元恋人に聞いてみよう、あなたならどうする?」
And virtual ex would say, be kind, I'm like, oh, yes, or something like that.
そして、バーチャル元彼は、「親切にしなさい」と言うのですが、私は、「ああ、そうだな」と思いました。
And even though I myself might not be able to do it unprompted, and my favorite prompt is think step by step.
そして、私自身は促されないとできないかもしれないけれど、私の好きな促しは、ステップバイステップで考えることです。
And I'm like, you know, this also works on my 10 year old.
そして、これは私の10歳の子どもにも効果があるんです。
When he tries to solve a math equation all in one step, I know exactly what mistake you'll make.
彼が数学の方程式を一通り解こうとしたとき、私はあなたがどんな間違いをするのかよく分かっています。
But if I prompt it with, oh, please think step by step, then it sort of gets you in a mindset.
でも、「一歩ずつ考えてね」と促すと、ある種のマインドセットになるんです。
And I think it's also part of the way that ChatGPT was actually trained, this whole sort of human in the loop reinforcement learning has probably reinforced these types of behaviors, whereby having this feedback loop, you kind of aligned AI better to the prompting opportunities by humans.
ChatGPTが実際に訓練された方法の一部だと思いますが、ループ内の人間による強化学習によって、この種の行動が強化されたのでしょう。このフィードバックループがあることで、人間による促しの機会に対してAIをよりうまく合わせることができるのです。
Yeah, prompting human like reasoning steps, the step by step kind of thinking.
そう、人間による推論のステップや、段階的な思考のようなものを促すのです。
Yeah, but it does seem to be, I suppose it just puts a mirror to our own capabilities.
でも、これは私たち自身の能力を映し出す鏡のようなものだと思います。
And so we can be truly impressed by our own cognitive capabilities, because the variety of what you can try is we don't usually have this kind of, we can't play with our own mind rigorously through Python code, right?
だからこそ、私たち自身の認知能力に本当に感銘を受けることができます。なぜなら、普段私たちはPythonコードを使って自分の心を厳密に操作することはできないので、試すことができるバリエーションは限られているからですよね。
Yeah.
そうです。
So it allows us to really play with all of human wisdom and knowledge, or at least knowledge at our fingertips, and then mess with that little mind that can think and speak in all kinds of ways.
だから、人間の知恵や知識、少なくとも指先の知識で遊ぶことができ、さらに、あらゆる方法で考えたり話したりできる小さな心を弄ぶことができるのです。
What's unique is that, as I mentioned earlier, every one of us was trained by different subset of human culture.
ユニークなのは、先ほど述べたように、私たち一人ひとりが、人類の文化の異なる部分集合によって訓練されたことです。
And ChatGPT was trained on all of it.
そして、ChatGPTはその全てに訓練を受けています。
And the difference there is that it probably has the ability to emulate almost every one of us.
そして、そこでの違いは、おそらく私たちのほとんどすべてを模倣する能力を持っているということです。
The fact that you can figure out where that is in cognitive behavioral space just by a few prompts is pretty impressive.
認知行動空間において、いくつかのプロンプトを出すだけで、それがどこにあるのかがわかるというのは、とても印象的です。
But the fact that that exists somewhere is absolutely beautiful.
しかし、それがどこかに存在するという事実は、非常に美しいものです。
And the fact that it's encoded in an orthogonal way from the knowledge, I think is also beautiful.
そして、それが知識とは直交する形で符号化されているという事実も、私は美しいと思います。
The fact that somehow through this extreme overparameterization of AI models, it was able to somehow figure out that context, knowledge, and form are separable.
AIモデルの極端なオーバーパラメーター化によって、コンテキスト、知識、フォームが分離可能であることを理解することができたのです。
And that you can sort of describe scientific knowledge in a haiku in the form of, I don't know, Shakespeare or something.
そして、科学的な知識を俳句の中で、シェイクスピアか何かの形で表現することができるのです。
That tells you something about the decoupling and the decouplability of these types of aspects of human psyche.
このことは、人間の精神のこの種の側面の分離と分離可能性について、何かを物語っています。
And that's part of the science of this whole thing.
そして、それがこの全体の科学の一部なのです。
So these large language models are days old in terms of this kind of leap that they've taken.
ですから、これらの大規模な言語モデルは、このような飛躍を遂げたという点では、何日も前のものなのです。
And it would be interesting to do this kind of analysis on them of the separation of context, form, and knowledge.
文脈、形式、知識の分離について、このような分析をするのは興味深いことです。
Where exactly does that happen?
それはいったいどこで起こるのでしょうか?
There's already sort of initial investigations, but it's very hard to figure out where.
すでに初期調査のようなものは行われていますが、その場所を特定するのは非常に困難です。
Is there a particular set of parameters that are responsible for a particular piece of knowledge or a particular context or a particular style of speaking?
特定の知識、特定の文脈、特定の話し方には、特定のパラメータのセットが必要なのでしょうか?
So with convolutional neural networks, interpretability had many good advances because we can kind of understand them.
そのため、畳み込みニューラルネットワークでは、解釈のしやすさが格段に向上しました。
There's a structure to them.
ニューラルネットワークには構造があります。
There's a locality to them.
局所性がある。
And we can kind of understand the different layers, have different sort of ranges that they're looking at.
また、異なるレイヤーを理解することができ、そのレイヤーが見ている範囲の違いも理解できます。
So we can look at activation features and basically see where does that correspond to.
そのため、活性化の特徴を見て、それがどこに対応しているのかを確認することができます。
With large language models, it's perhaps a little more complicated, but I think it's still achievable in the sense that we could kind of ask, well, what kind of prompts does this generate?
大規模な言語モデルの場合は、もう少し複雑ですが、「このモデルはどのようなプロンプトを生成するか?
If I sort of drop out this part of the network, then what happens?
ネットワークのこの部分を削除したら、どうなるのか?
And sort of start getting at a language to even describe these types of aspects of human behavior or psychology, if you wish, from the spoken part in the language part.
そして、人間の行動や心理のこのような側面を記述するための言語を、音声部分から言語部分へと広げていくことができるのです。
And the advantage of that is that it might actually teach us something about humans as well.
そして、その利点は、実際に人間について何かを教えてくれるかもしれないということです。
We might not have words to describe these types of aspects right now.
私たちは今、このような側面を表現する言葉を持っていないかもしれません。
But when somebody speaks in a particular way, it might remind us of a friend that we know from here and there and there.
しかし、誰かが特定の方法で話すと、あちらこちらで知り合った友人を思い出すかもしれません。
And if we had better language for describing that, these concepts might become more apparent in our own human psyche.
もし、それを説明するためのより良い言葉があれば、こうした概念は私たち自身の人間心理の中でより明白になるかもしれません。
And then we might be able to encode them better in machines themselves.
そして、その概念を機械にうまく組み込むことができるようになるかもしれません。
I mean, but probably you and I would have certain interests with the base model would open Echo is the base model, which is before the alignment of the reinforcement learning with human feedback and before the AI safety-based kind of censorship of the model.
つまり、しかし、おそらくあなたと私は、ベースモデルは、人間のフィードバックと強化学習の整合性の前であり、モデルのAIの安全ベースの一種の検閲の前であるベースモデルとしてエコーを開くだろうとの特定の関心を持っています。
この記事が気に入ったらサポートをしてみませんか?