ヒトの呼吸器系における SARS-CoV-2 排出のダイナミクス
SARS-CoV-2 shedding dynamics in human respiratory tract
ヒトの呼吸器系における SARS-CoV-2 排出のダイナミクス
Abstract
It is crucial to understand how Severe Acute Respiratory Syndrome Coronavirus 2 (SARS-CoV-2) sheds in human respiratory tract, but this question is still elusive to understand due to technical limitations.
重症急性呼吸器症候群コロナウイルス 2 (SARS-CoV-2) がヒトの呼吸器系でどのように排出されるかを理解することは重要ですが、技術的な制限により、この問題は依然として理解しにくいものです。
Here we integrated published human metagenomic data of SARS-CoV-2 and developed a novel algorithm named as RedeCoronaVS to systematically dissect SARS-CoV-2 shedding modes with single-cell data as reference.
ここでは、公開されている SARS-CoV-2 のヒトメタゲノムデータを統合し、単一細胞データを参照として SARS-CoV-2 排出モードを体系的に分析するための、RedeCoronaVS という新しいアルゴリズムを開発しました。
We identify that SARS-CoV-2 particles are the dominant mode of viral shedding in the very early infection phase (≤24 hours after hospitalization).
感染のごく初期段階 (入院後 24 時間以内) では、SARS-CoV-2 粒子がウイルス排出の主なモードであることがわかりました。
Within the first week after hospitalization, SARS-CoV-2 replicons within host cells dominate SARS-CoV-2 shedding together with viral particles.
入院後 1 週間以内に、宿主細胞内の SARS-CoV-2 レプリコンがウイルス粒子とともに SARS-CoV-2 排出を支配します。
One week later, viral fragments become the dominant mode in patients with mild or moderate symptoms, but viral replicons still dominate in some patients with severe symptoms.
1 週間後、軽度または中等度の症状の患者ではウイルス断片が支配的になりますが、重度の症状の患者の中にはウイルス レプリコンが依然として支配的である人もいます。
In addition to epithelial cells, SARS-CoV-2 replicons in neutrophils, macrophages, and plasma cells also show important roles and are associate with sampling time and disease severity.
上皮細胞に加えて、好中球、マクロファージ、形質細胞内の SARS-CoV-2 レプリコンも重要な役割を果たしており、サンプル採取時間と病気の重症度に関連しています。
Introduction
Coronavirus Disease 2019 (COVID-19), representing a newly emerging infectious disease, is a respiratory illness known for rapid transmission, acute onset, diverse modes of transmission, and the potential for multi-organ infections.
新型コロナウイルス感染症(COVID-19)は、新興感染症として知られ、急速な感染、急性発症、多様な感染様式、多臓器感染の可能性で知られる呼吸器疾患です。
Despite relaxed control policies in many countries, the ongoing emergence of new SARS-CoV-2 variants poses a risk of recurrent infections for susceptible populations.
多くの国で緩和された管理政策にもかかわらず、SARS-CoV-2の新たな変異体の出現が続いており、感受性の高い集団に再発感染のリスクが生じています。
Therefore, understanding the viral shedding patterns of COVID-19 patients is important for formulating precise control strategies.
したがって、COVID-19患者のウイルス排出パターンを理解することは、正確な管理戦略を策定する上で重要です。
Currently, research on methods for detecting viral shedding in COVID-19 patients mainly falls into three categories: quantitative reverse transcription polymerase chain reaction (qRT-PCR) and droplet digital polymerase chain reaction (ddPCR) for measuring viral RNA copies; antigen-detecting (rapid) diagnostic tests targeting viral proteins, primarily the spike protein; and methods for measuring infectious viral particles, such as virus isolation and plaque assays or 50% tissue culture infectious dose (TCID50).
現在、COVID-19 患者のウイルス排出を検出する方法の研究は、主に次の 3 つのカテゴリに分類されます: ウイルス RNA コピーを測定する定量的逆転写ポリメラーゼ連鎖反応 (qRT-PCR) と液滴デジタルポリメラーゼ連鎖反応 (ddPCR); 主にスパイクタンパク質を標的とするウイルスタンパク質を標的とする抗原検出 (迅速) 診断テスト;ウイルス分離およびプラークアッセイ、または 50% 組織培養感染量 (TCID50) などの感染性ウイルス粒子を測定する方法。
However, conventional methods measuring viral RNA are limited to quantification, rather than dissecting the shedding patterns.
しかし、ウイルスRNAを測定する従来の方法は、排出パターンを分析するのではなく、定量化に限定されています。
The viral load typically represents the number of viral RNA copies, but it only quantifies the viral genome fragments and does not represent
infectious viral particles.
ウイルス量は通常、ウイルスRNAのコピー数を表しますが、ウイルスゲノム断片を定量化するだけで、感染性ウイルス粒子を表すものではありません。
Methods customized for viral particle analysis, such as electron microscopy or plaque-forming assays, can offer compelling evidence but are hard to be applied to large number of samples due to high cost and biosafety requirements.
電子顕微鏡検査やプラーク形成アッセイなど、ウイルス粒子分析用にカスタマイズされた方法は、説得力のある証拠を提供できますが、コストが高く、バイオセーフティ要件があるため、多数のサンプルに適用するのは困難です。
Therefore, there is an urgent need to develop new techniques to understand the patterns of SARS-CoV-2 shedding based on large cohorts.
したがって、大規模なコホートに基づいてSARS-CoV-2の排出パターンを理解するための新しい技術を開発することが緊急に必要です。
Metatranscriptomic sequencing is a direct and unbiased method for acquiring comprehensive transcriptome sequencing data from samples, which has been widely employed in current microbiological research and is highly efficient for virus discovery.
メタトランスクリプトームシーケンシングは、サンプルから包括的なトランスクリプトームシーケンシングデータを取得するための直接的かつ偏りのない方法であり、現在の微生物学的研究で広く採用されており、ウイルスの発見に非常に効率的です。
Recent studies have used this approach to reveal the microbial microenvironment in the respiratory tract of COVID-19 patients and exploring the relationship between the microbiome and transcriptome.
最近の研究では、このアプローチを使用して、COVID-19患者の呼吸器内の微生物微小環境を明らかにし、マイクロバイオームとトランスクリプトームの関係を調査しています。
We have observed that metatranscriptomic sequencing techniques, covering the entire transcriptome of SARS-CoV-2, offer a burgeoning research perspective on viral shedding in COVID-19 patients.
私たちは、SARS-CoV-2のトランスクリプトーム全体をカバーするメタトランスクリプトームシーケンシング技術が、COVID-19患者のウイルス排出に関する急成長中の研究視点を提供していることを観察しました。
However, there is still a lack of tools to integrate these two aspects.
しかし、これら2つの側面を統合するツールはまだ不足しています。
Here, we gathered a total of 301 samples with metatranscriptomic sequencing data from the respiratory tracts of COVID-19 patients, encompassing diverse nationalities, ethnicities, infection stages, disease severities, and sample types.
ここでは、多様な国籍、民族、感染段階、病気の重症度、サンプルの種類を含む、COVID-19 患者の呼吸器からメタトランスクリプトミクス シーケンス データを含む合計 301 のサンプルを収集しました。
These samples, which includes comprehensive clinical information of the patients, stands as the most comprehensive to date.
患者の包括的な臨床情報を含むこれらのサンプルは、これまでで最も包括的なものとなっています。
Based on single-cell sequencing and metatranscriptomic sequencing data, we developed a digital analytical method named as RedeCoronaVS to dissect the patterns of SARS-CoV-2 shedding in the respiratory tract of COVID-19 patients.
単一細胞シーケンスとメタトランスクリプトーム シーケンス データに基づいて、COVID-19 患者の呼吸器における SARS-CoV-2 排出のパターンを分析するための RedeCoronaVS というデジタル分析方法を開発しました。
We categorized viral shedding in the respiratory tract into three types of patterns: viral replicons (intracellular), viral particles (extracellular), and viral fragments.
呼吸器系におけるウイルス排出を、ウイルスレプリコン(細胞内)、ウイルス粒子(細胞外)、ウイルス断片の3種類のパターンに分類しました。
Unsupervised machine learning results based on non-negative matrix factorization prove our categorization.
非負行列因子分解に基づく教師なし機械学習の結果が、この分類を証明しています。
Then we developed RedeCoronaVS, which accurately determines the abundance of various shedding patterns in individual patients through the viral RNA data, enhancing our understanding of viral shedding in COVID-19 patients.
その後、私たちはRedeCoronaVSを開発しました。これは、ウイルスRNAデータを通じて個々の患者のさまざまな排出パターンの量を正確に判断し、COVID-19患者のウイルス排出に関する理解を深めます。
RedeCoronaVS revealed correlations between different patterns of viral shedding and clinical features such as sampling site, days after hospitalization, and the severity of COVID-19.
RedeCoronaVSは、さまざまなウイルス排出パターンと、サンプル採取部位、入院後の日数、COVID-19の重症度などの臨床的特徴との相関関係を明らかにしました。
The pattern of viral shedding in COVID-19 patients varies depending on the sampling time during hospitalization.
COVID-19 患者のウイルス排出パターンは、入院中のサンプル採取時間によって異なります。
The types of viral replicons are associated with the clinical status of patients.
ウイルスレプリコンの種類は、患者の臨床状態と関連しています。
Neutrophil and macrophage viral replicons tend to shed in the early stages of infection (≤24 hours after hospitalization), while plasma cell viral replicons predominate in the respiratory tract of severe COVID-19 patients.
好中球およびマクロファージのウイルスレプリコンは感染初期段階 (入院後 24 時間以内) に排出される傾向があり、一方、重症 COVID-19 患者の呼吸器系では形質細胞ウイルスレプリコンが優勢です。
Our integrative analysis, the newly developed algorithms, and the findings are expected to provide important insights into the viral shedding dynamics of COVID-19.
私たちの統合分析、新しく開発されたアルゴリズム、および調査結果は、COVID-19 のウイルス排出ダイナミクスに関する重要な洞察を提供するものと期待されます。
Results
Comparisons of the subgenomic transcription of SARS-CoV-2 among different cell types
異なる細胞タイプ間での SARS-CoV-2 のサブゲノム転写の比較
As a typical coronavirus, SARS-CoV-2 exhibits 'discontinuous transcription' (also known as subgenomic transcription) within host cells, greatly enhancing transcription and translation efficiency (Figure 1A).
典型的なコロナウイルスである SARS-CoV-2 は、宿主細胞内で「不連続転写」(サブゲノム転写とも呼ばれる)を示し、転写と翻訳の効率を大幅に高めます(図 1A)。
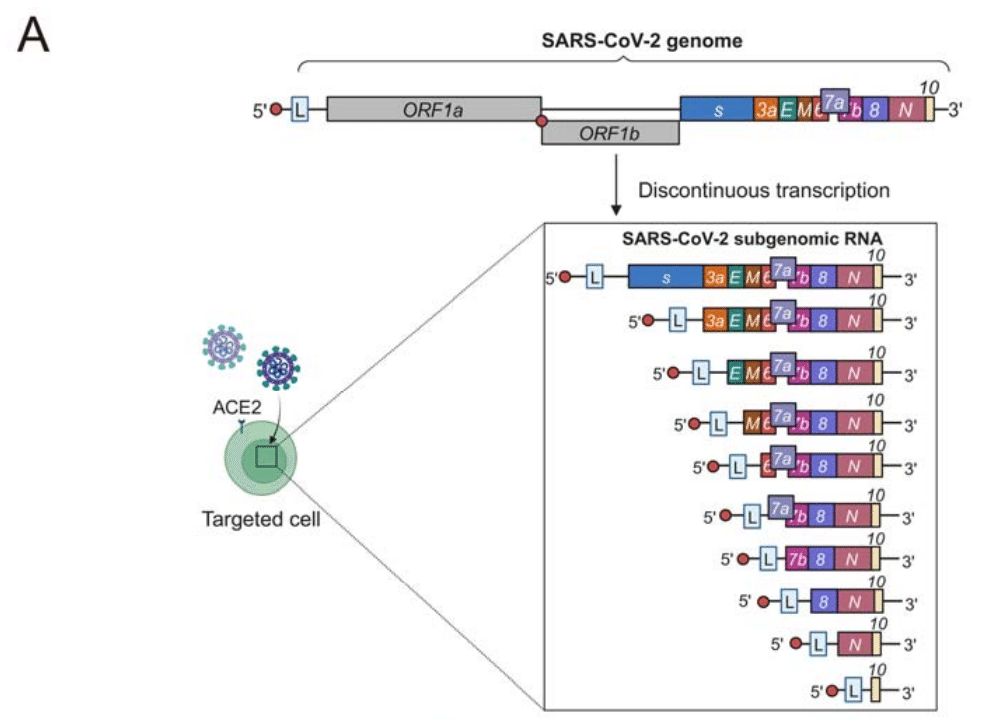
While many studies have established the multi-tissue and multi-cell type tropism of SARS-CoV-2, it is unclear whether the virus exhibits different subgenomic transcription patterns in various cell types.
多くの研究で SARS-CoV-2 の多組織および多細胞型向性が確立されていますが、ウイルスがさまざまな細胞型で異なるサブゲノム転写パターンを示すかどうかは不明です。
To address this, we gathered data from previous study utilizing single-cell RNA sequencing to detect viral genes in different cell types infected by SARS-CoV-2.
これに対処するために、私たちは、SARS-CoV-2 に感染したさまざまな細胞型でウイルス遺伝子を検出するために単一細胞 RNA シーケンシングを利用した以前の研究からデータを収集しました。
Given that ciliated cells are considered the primary host cells for SARS-CoV-2, we set the pattern of viral transcription in ciliated cells as a standard template and compared it with other cell types.
繊毛細胞はSARS-CoV-2の主な宿主細胞と考えられているため、繊毛細胞におけるウイルス転写のパターンを標準テンプレートとして設定し、他の細胞タイプと比較しました。
Subsequent correlation analysis indicates slight heterogeneity in viral transcription among cell types, for example, differences in epithelial cells are mainly in the E and ORF7b genes (Figure 1B), while differences between ciliated cells and other cell types such as myeloid cells and lymphocytes are mainly in the N gene (Figure 1C-1D).
その後の相関分析では、細胞タイプ間でウイルス転写にわずかな異質性があることが示されました。たとえば、上皮細胞の違いは主にE遺伝子とORF7b遺伝子にあります(図1B)。一方、繊毛細胞と骨髄細胞やリンパ球などの他の細胞タイプの違いは主にN遺伝子にあります(図1C-1D)。
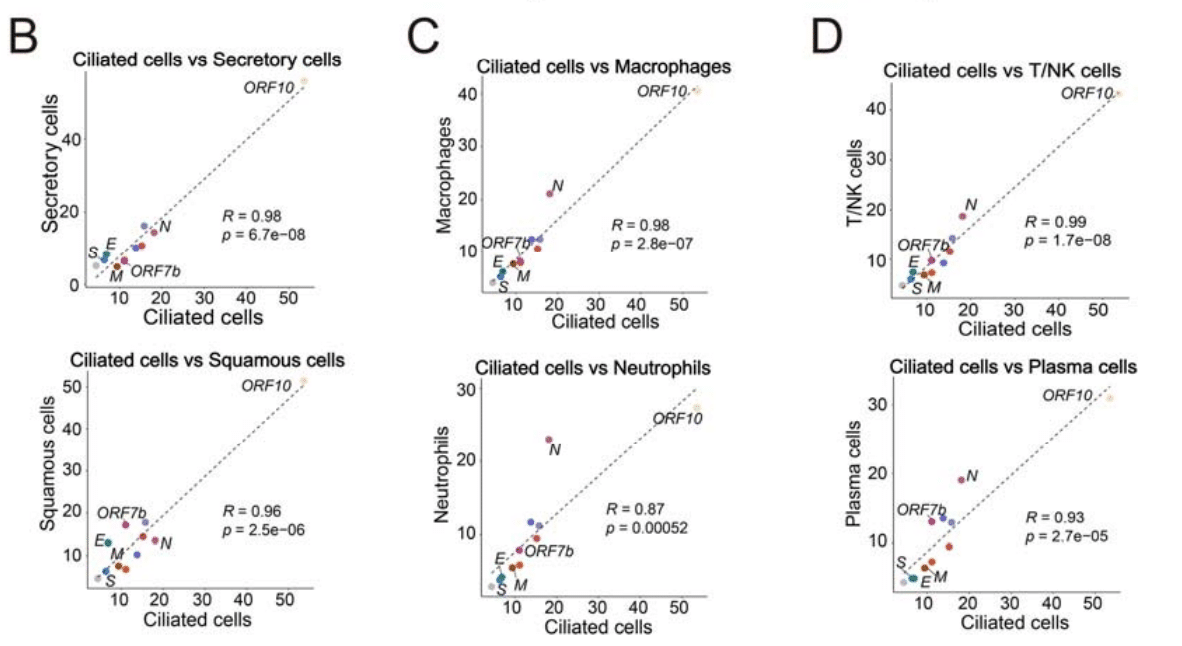
This suggests the potential to distinguish infected cell types based on the detection rate of SARS-CoV-2.
これは、SARS-CoV-2の検出率に基づいて感染細胞タイプを区別できる可能性を示唆しています。
Metatranscriptomic data of SARS-CoV-2 deviate subgenomic transcription
SARS-CoV-2のメタトランスクリプトミクスデータはサブゲノム転写を逸脱している
Next, we collected 301 respiratory samples with metatranscriptomic data available from three previously published cohorts (STAR Methods).
次に、以前に公開された 3 つのコホート (STAR メソッド) から入手可能なメタトランスクリプトミクス データを持つ 301 個の呼吸器サンプルを収集しました。
To explore the gene expression pattern of SARS-CoV-2 in bulk RNA sequencing data and its association with single-cell RNA sequencing data, we conducted an analysis of the distribution of viral RNA-seq data (normalized by Transcripts Per Kilobase Million) across the mean values of various genes (Figure 2A-2D, Figure S1A).
バルク RNA シーケンシング データにおける SARS-CoV-2 の遺伝子発現パターンと、その単一細胞 RNA シーケンシング データとの関連性を調べるために、さまざまな遺伝子の平均値にわたるウイルス RNA-seq データ (1 キロベース 100 万あたりの転写産物で正規化) の分布の分析を実施しました (図 2A-2D、図 S1A)。
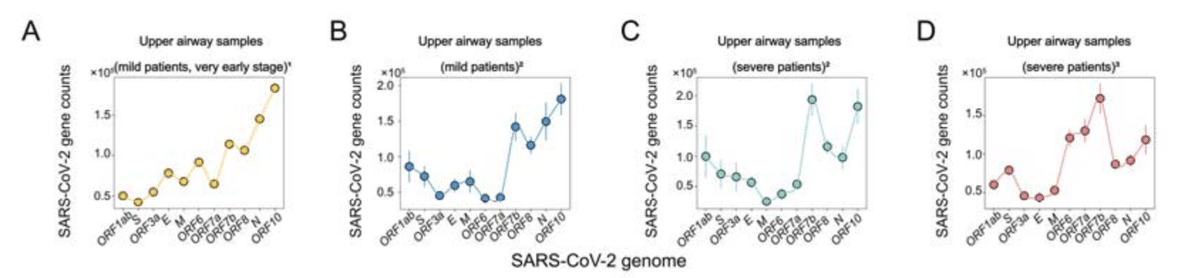
Furthermore, we applied a correlation analysis between the single cell level viral RNA detection rate in SARS-CoV-2 infected ciliated cells and viral RNA-seq data (Figure 2E-2H, Figure S1B).
さらに、SARS-CoV-2 に感染した繊毛細胞における単一細胞レベルのウイルス RNA 検出率とウイルス RNA-seq データとの相関分析を適用しました (図 2E-2H、図 S1B)。
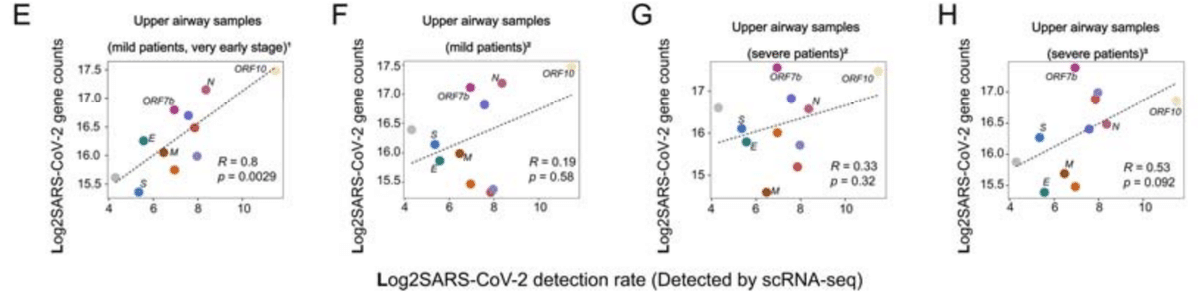
In particular, the metatranscriptomic data of early hospitalization COVID-19 patients were characterized by close proximity to the rate of viral detection at the single-cell level, indicative of active viral replication and transmission (Figure 2A, 2E).
特に、COVID-19 の入院初期患者のメタトランスクリプトームデータは、単一細胞レベルでのウイルス検出率に近いことが特徴で、活発なウイルス複製と伝染を示しています (図 2A、2E)。
Results from metatranscriptomic data from other cohorts differed from those from early hospitalization in having high ORF7b abundance and low correlation with single-cell level viral RNA detection, suggesting that SARS-CoV-2 viral metatranscriptome sequencing data do not fully align with the discontinuous transcription pattern of the virus within cells (Figure 2B-D, Figure 2F-H).
他のコホートのメタトランスクリプトームデータの結果は、ORF7b の豊富さが高く、単一細胞レベルのウイルス RNA 検出との相関が低い点で入院初期と異なり、SARS-CoV-2 ウイルスのメタトランスクリプトーム配列データは、細胞内のウイルスの不連続な転写パターンと完全には一致していないことを示唆しています (図 2B-D、図 2F-H)。
The lower respiratory source samples were consistent with the results of the upper respiratory samples (Figure S1A-S1B).
下気道ソースサンプルは、上気道サンプルの結果と一致していました (図 S1A-S1B)。
Unsupervised dissection of SARS-CoV-2 shedding patterns in metatranscriptomic data
メタトランスクリプトミクスデータにおける SARS-CoV-2 排出パターンの無監視解析
Based on the former results, we assuming that there are other potential confounding factors in the SARS-CoV-2 metatranscriptomic data, Non-Negative Matrix Factorization (NMF) was employed to identify the distribution characteristics of viral genes, facilitating the recognition of viral shedding patterns (STAR methods).
以前の結果に基づき、SARS-CoV-2 メタトランスクリプトミクス データには他の潜在的な交絡因子があると仮定し、非負マトリックス因子分解 (NMF) を使用してウイルス遺伝子の分布特性を特定し、ウイルス排出パターンの認識を容易にしました (STAR メソッド)。
When specifying three categories, the viral RNA distribution in the classification results is depicted in the figure (Figure 3A-3D, Figure S1C; Table S1).
3 つのカテゴリを指定すると、分類結果のウイルス RNA 分布が図に示されます (図 3A-3D、図 S1C、表 S1)。
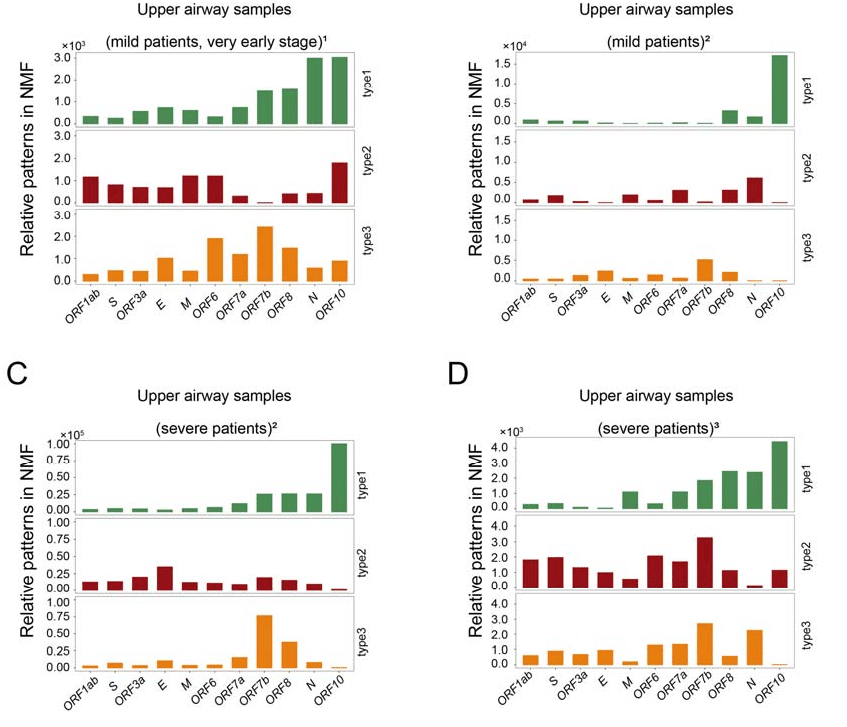
Interestingly, this aligns well with biological expectations.
興味深いことに、これは生物学的な予想とよく一致しています。
In the results, we observed patterns with typical subgenomic transcription characteristics.
結果では、典型的なサブゲノム転写特性を持つパターンが観察されました。
Additionally, patterns with a relatively uniform distribution of viral RNA were noted, potentially representing viral particles released into the extracellular space after completing the viral life cycle within host cells.
さらに、ウイルス RNA の比較的均一な分布を持つパターンが注目されました。これは、宿主細胞内でウイルスのライフサイクルを完了した後に細胞外空間に放出されたウイルス粒子を表している可能性があります。
Notably, we identified a viral RNA expression pattern where only a portion of viral RNA had reads, and a significant amount of viral subgenomic RNA escaped detection by sequencing technology.
特に、ウイルスRNAの一部にのみ読み取りがあり、かなりの量のウイルスサブゲノムRNAがシーケンシング技術による検出を逃れたウイルスRNA発現パターンを特定しました。
This might indicate incomplete and deactivated viral fragments.
これは、不完全で不活性化されたウイルス断片を示している可能性があります。
Therefore, we categorize the forms of SARS-CoV-2 shed into three types: viral replicons, viral particles, and viral fragments.
したがって、SARS-CoV-2の放出形態を、ウイルスレプリコン、ウイルス粒子、ウイルス断片の3種類に分類します。
"Viral replicon" refers to the intracellular form of SARS-CoV-2, where SARS-CoV-2 replicons and transcribes with a subgenomic manner.
「ウイルスレプリコン」とは、SARS-CoV-2 の細胞内形態を指します。 SARS-CoV-2 はサブゲノム方式でレプリコンおよび転写を行います。
The transcription patterns of the SARS-CoV-2 genome vary among different target cells, so we categorize viral replicons based on cell types with viral tropism.
SARS-CoV-2 ゲノムの転写パターンは標的細胞ごとに異なるため、ウイルスレプリコンはウイルス指向性を持つ細胞タイプに基づいて分類します。
Viral particles and viral fragments are defined as intact and fragmented SARS-CoV-2 particles, respectively.
ウイルス粒子とウイルス断片は、それぞれ完全な SARS-CoV-2 粒子と断片化された SARS-CoV-2 粒子として定義されます。
Supervised dissection of SARS-CoV-2 shedding patterns in metatranscriptomic data by using RedeCoronaVS
RedeCoronaVS を使用したメタトランスクリプトミクスデータにおける SARS-CoV-2 排出パターンの教師付き解析
RedeCoronaVS is implemented as a linear programming model (Figure 4).
RedeCoronaVS は線形計画モデルとして実装されています (図 4)。
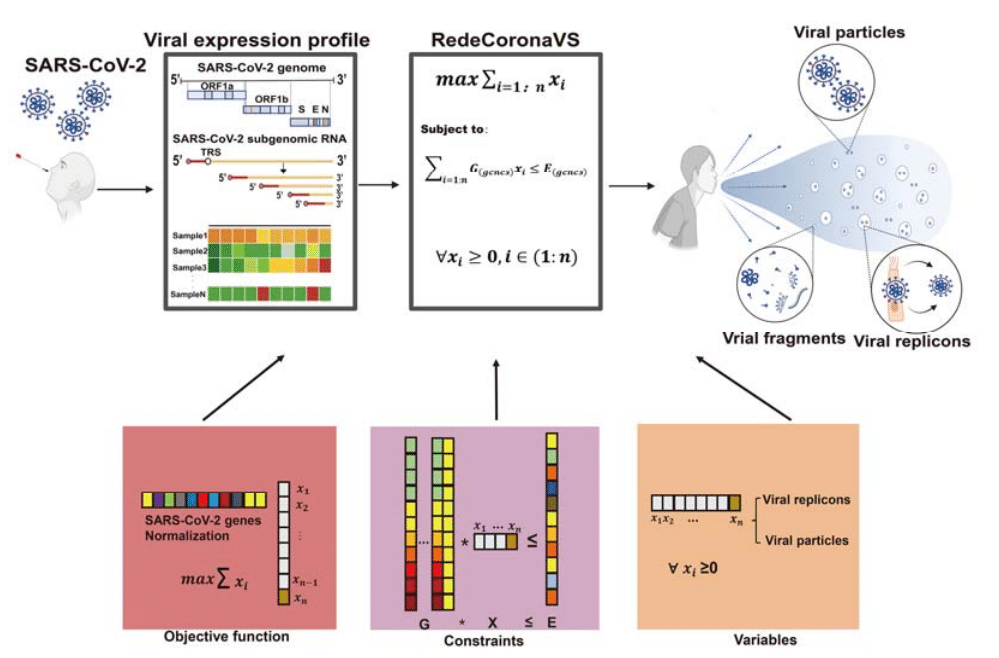
It includes one objective function, several types of constraints, and several decision variables.
これには、1 つの目的関数、数種類の制約、およびいくつかの決定変数が含まれます。
The output of RedeCoronaVS is the abundance of the three viral shedding modes (Table S2).
RedeCoronaVS の出力は、3 つのウイルス排出モードの存在量です (表 S2)。
The number of decision variables is equal to the chosen input viral RNA detection rates for different cell types and expected viral RNA reads in the viral particle state, corresponding to the expectation matrix of constraints (STAR methods).
決定変数の数は、異なる細胞タイプに対して選択された入力ウイルスRNA検出率と、ウイルス粒子状態での予想されるウイルスRNA読み取り数に等しく、制約の期待行列に対応します(STARメソッド)。
The constraints of RedeCoronaVS ensure that the product of the expectation matrix input and the variables does not exceed the actual viral RNA expression.
RedeCoronaVSの制約は、期待行列入力と変数の積が実際のウイルスRNA発現を超えないことを保証します。
The objective function aims to maximize the abundance of viral replicons and viral particles to explain the observed SARS-CoV-2 gene expression data.
目的関数は、ウイルスレプリコンとウイルス粒子の豊富さを最大化して、観測されたSARS-CoV-2遺伝子発現データを説明することを目的としています。
The nonnegative constraints are used to confine the abundance of viral replicons and particles to be positive or at least zero, and the residuals between the observed gene expression and the estimated replicons and particles are used to infer the abundance of viral fragments.
非負制約は、ウイルスレプリコンおよび粒子の豊富さを正または少なくともゼロに制限するために使用され、観察された遺伝子発現と推定されたレプリコンおよび粒子の間の残差は、ウイルス断片の豊富さを推測するために使用されます。
The subgenomic transcription pattern of SARS-CoV-2 is derived from published single-cell RNA sequencing data of SARS-CoV-2 infection (STAR Methods).
SARS-CoV-2 のサブゲノム転写パターンは、公開されている SARS-CoV-2 感染の単一細胞 RNA シーケンスデータ から取得されます (STAR メソッド)。
To test the performance of RedeCoronaVS, we first conducted rigorous quality control on the samples to exclude the potential impact of sequencing depth (Figure S2).
RedeCoronaVS の性能をテストするために、まずサンプルの厳格な品質管理を実施し、シーケンス深度の潜在的な影響を排除しました (図 S2)。
Next, to validate the accuracy of the RedeCoronaVS results, we conducted Pearson’s correlation analysis between the abundance of viral replicons/particles and CT values or viral RNA copies obtained from PCR test (Figure S3A-S3E).
次に、RedeCoronaVS の結果の精度を検証するために、ウイルス レプリコン/粒子の存在量と CT 値または PCR テストから得られたウイルス RNA コピーとの間のピアソンの相関分析を実施しました (図 S3A-S3E)。
For ease of statistical analysis, we summed the total abundance of viral replicons.
統計分析を容易にするため、ウイルスレプリコンの総量を合計しました。
The analysis revealed a strong correlation between the abundance of viral replicons and viral particles with the PCR results in all cohorts except mild patients’ samples from the Wuhan cohort.
分析により、武漢コホートの軽症患者のサンプルを除くすべてのコホートで、ウイルスレプリコンとウイルス粒子の量とPCR結果の間に強い相関関係があることが明らかになりました。
This result is reasonable because mild cases in the later stages of the disease are expected to shed a large number of viral fragments.
この結果は妥当です。病気の後期段階の軽症患者は大量のウイルス断片を排出すると予想されるためです。
This could potentially yield positive nucleic acid test results without the presence of infectious viral replicons or particles being shed.
これにより、感染性ウイルスレプリコンや粒子が排出されていなくても、核酸検査で陽性の結果が出る可能性があります。
Subsequently, we aimed to validate the RedeCoronaVS results at a relative quantitative level for different viral shedding types.
その後、異なるウイルス排出タイプについて、相対的な定量レベルでRedeCoronaVSの結果を検証することを目指しました。
A fundamental assumption is that when COVID-19 patients primarily shed viral fragments as the main viral shedding type, it indicates that the patient has entered the late stages of infection.
基本的な仮定は、COVID-19 患者が主にウイルスの排出タイプとしてウイルス断片を排出する場合、患者が感染後期に入っていることを示すということです。
Simultaneously, corresponding PCR tests should detect little or no substantial amount of viral RNA.
同時に、対応する PCR 検査では、ウイルス RNA がほとんどまたはまったく検出されないはずです。
To test this, we analyzed the relationship between the relative abundance of the three viral shedding types in different samples and PCR results (STAR Methods).
これをテストするために、異なるサンプルにおける 3 つのウイルス排出タイプの相対的な存在量と PCR 結果の関係を分析しました (STAR メソッド) 。
The ternary plot results confirm our hypothesis: when the proportion of viral fragments is higher, there is a tendency to detect a lower amount of viral RNA (Figure S4A-S4C).
三角プロットの結果は、ウイルス断片の割合が高いほど、検出されるウイルスRNAの量が少なくなる傾向があるという私たちの仮説を裏付けています(図S4A-S4C)。
These results indicate that RedeCoronaVS accurately differentiates and precisely quantifies viral shedding patterns in respiratory tract of COVID-19 patients.
これらの結果は、RedeCoronaVSがCOVID-19患者の呼吸器系におけるウイルス排出パターンを正確に区別し、正確に定量化していることを示しています。
RedeCoronaVS revealed relationships between different viral shedding patterns
RedeCoronaVSは、さまざまなウイルス排出パターンの関係を明らかにした
We then explored correlations between viral replicons, viral particles and viral fragments.
次に、ウイルスレプリコン、ウイルス粒子、ウイルス断片間の相関関係を調査しました。
We calculated the relative quantification of each mode for each sample and conducted correlation analysis (Figure 5A-5C).
各サンプルの各モードの相対的な定量化を計算し、相関分析を実施しました (図 5A-5C)。
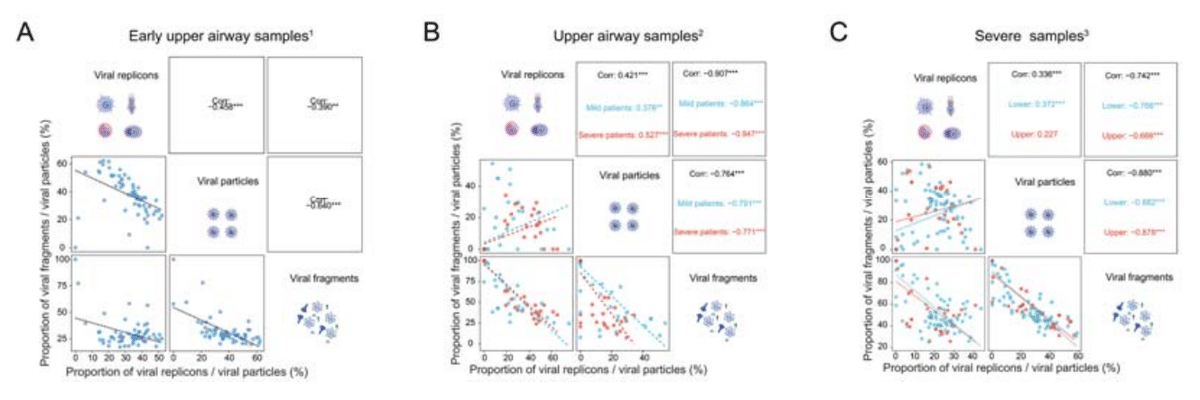
The results showed a positive correlation between viral replicons and viral particles, consistent with previous studies.
結果は、以前の研究と一致して、ウイルスレプリコンとウイルス粒子の間に正の相関関係を示しました。
Viral fragments exhibited a strong negative correlation with the other two modes which indicates that the proportion of viral fragments can serve as a recovery indicator for COVID-19 patients.
ウイルス断片は他の 2 つのモードと強い負の相関関係を示しており、これはウイルス断片の割合が COVID-19 患者の回復指標として機能できることを示しています。
Interestingly, in the Vanderbilt cohort, providing samples from the early stages of infection, we observed negative correlations among all three viral shedding modes (Figure 5A).
興味深いことに、感染初期段階のサンプルを提供するヴァンダービルトコホートでは、3 つのウイルス排出モードすべてに負の相関関係が見られました (図 5A)。
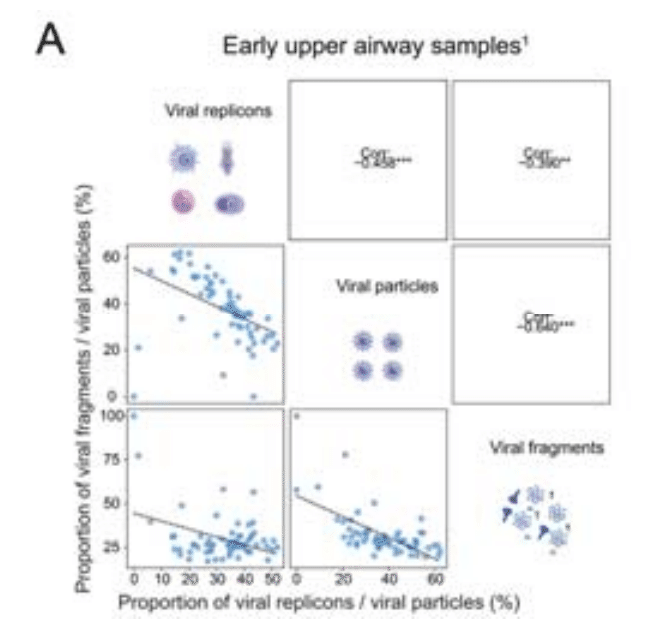
Viral replicons are associated with clinical status of COVID-19 patients
ウイルスレプリコンはCOVID-19患者の臨床状態と関連している
Next, a cluster analysis was conducted on the RedeCoronaVS results obtained.
次に、得られた RedeCoronaVS の結果に対してクラスター分析を実施しました。
Consistent with the fact that most samples were collected within the recovery phase, the majority of samples exhibited high levels of viral fragments but low levels of viral replicons or particles (Figure 6A).
ほとんどのサンプルが回復期に収集されたという事実と一致して、サンプルの大部分はウイルス断片のレベルは高いものの、ウイルスレプリコンまたは粒子のレベルは低いことが示されました (図 6A)。
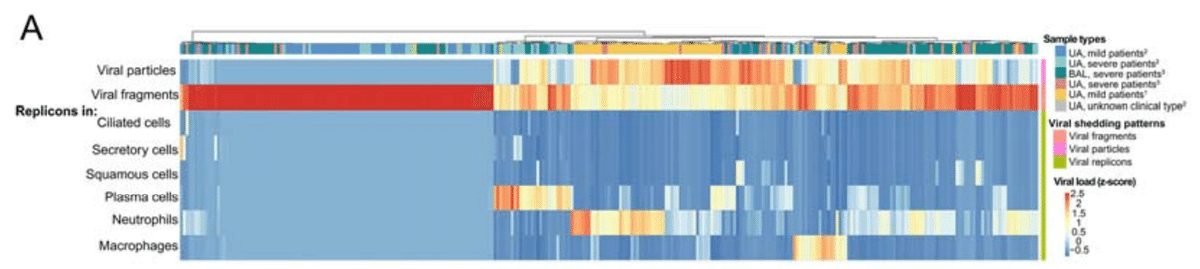
The large cohort size enabled us to dissect the associations of sampling site, days after hospitalization and COVID-19 severity with the abundance of different viral shedding patterns in patients.
コホートのサイズが大きいため、サンプル採取場所、入院後の日数、COVID-19 の重症度と、患者のさまざまなウイルス排出パターンの豊富さとの関連性を分析することができました。
We applied ANOVA analysis to interrogate such associations based on 203 upper airway samples and 95 lower airway samples (Figure 6B; Table S3; STAR Methods).
我々は、203 個の上気道サンプルと 95 個の下気道サンプルに基づいて、このような関連性を調べるために ANOVA 分析を適用しました (図 6B、表 S3、STAR メソッド)。
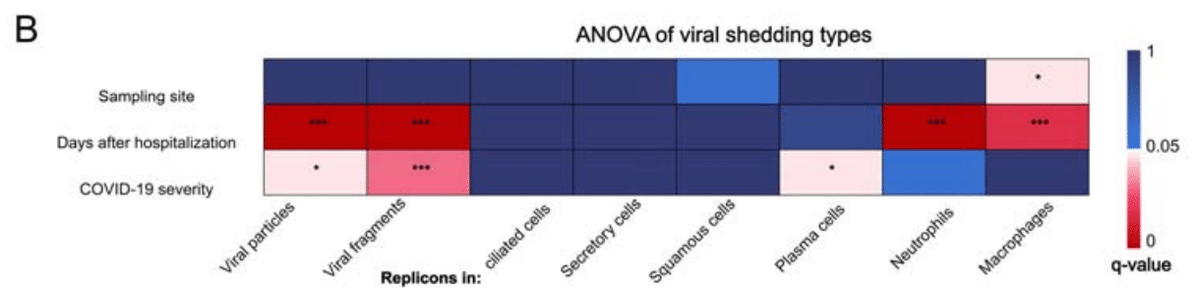
Indeed, we have observed variations in viral replicons types in respiratory shed across different clinical stages of COVID-19 patients.
実際、COVID-19 患者のさまざまな臨床段階にわたって、呼吸器から排出されるウイルス レプリコンの種類にばらつきがあることが観察されています。
The proportion of replicons in neutrophils and macrophages is closely correlated with the sampling time after hospitalization (Figure 6C-6D).
好中球とマクロファージのレプリコンの割合は、入院後のサンプル採取時間と密接に相関しています (図 6C-6D)。
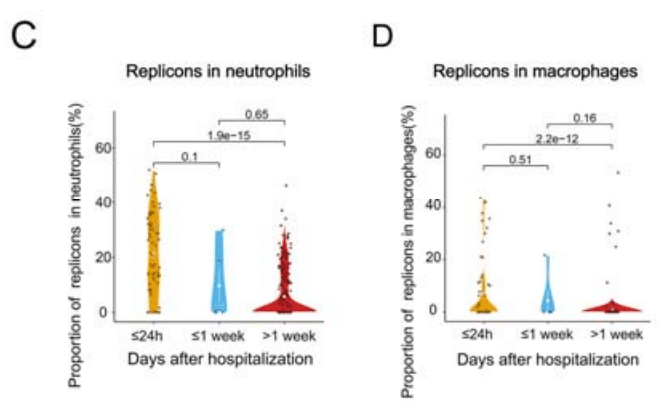
It is significantly higher when patients are admitted early compared to one week after admission, indicating that SARS-CoV-2 primarily sheds innate immune cells during the early stages of infection.
患者が入院後 1 週間後よりも早期に入院した場合の方が有意に高く、SARS-CoV-2 は感染の初期段階で主に自然免疫細胞を排出することを示しています。
The proportion of replicons in macrophages is associated with the sampling site, being more commonly detected in upper respiratory tract samples (Figure 6E).
マクロファージ内のレプリコンの割合は、サンプル採取部位と関連しており、上気道サンプルでより一般的に検出されます (図 6E)。
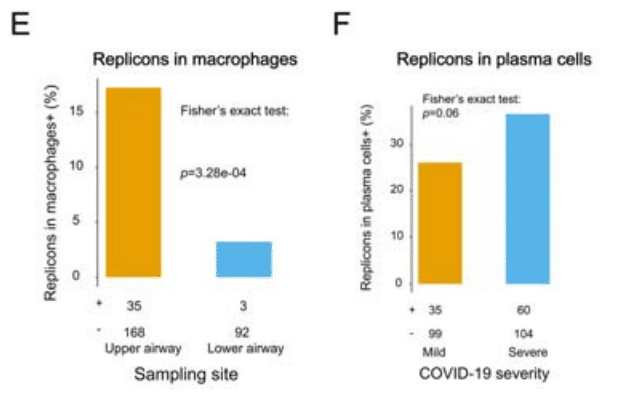
Replicons in plasma cells are more likely to be detected in severe COVID-19 patients, suggesting that they may be the primary cell type shedding in this category of patients (Figure 6F).
形質細胞内のレプリコンは、重症 COVID-19 患者で検出される可能性が高く、このカテゴリの患者で排出される主な細胞タイプである可能性があることを示唆しています (図 6F)。
In summary, viral replicons are closely related with the clinical status of the COVID-19 patients.
要約すると、ウイルスのレプリコンは、COVID-19 患者の臨床状態と密接に関連しています。
Associations of SARS-CoV-2 shedding patterns with sampling time and COVID-19 severity
SARS-CoV-2 排出パターンとサンプル採取時間および COVID-19 重症度との関連性
Lastly, we aimed to identify the shedding phases of SARS-CoV-2 and other potential factors influencing viral shedding by RedeCoronaVS.
最後に、RedeCoronaVS による SARS-CoV-2 の排出段階と、ウイルス排出に影響を与えるその他の潜在的要因を特定することを目的としました。
ANOVA revealed that viral replicons, viral particles, and viral fragments are all heterogeneously associated with factors such as sampling days after hospitalization and disease severity (Figure 6B).
ANOVA により、ウイルスレプリコン、ウイルス粒子、ウイルス断片はすべて、入院後のサンプル採取日数や病気の重症度などの要因と不均一に関連していることが明らかになりました (図 6B)。
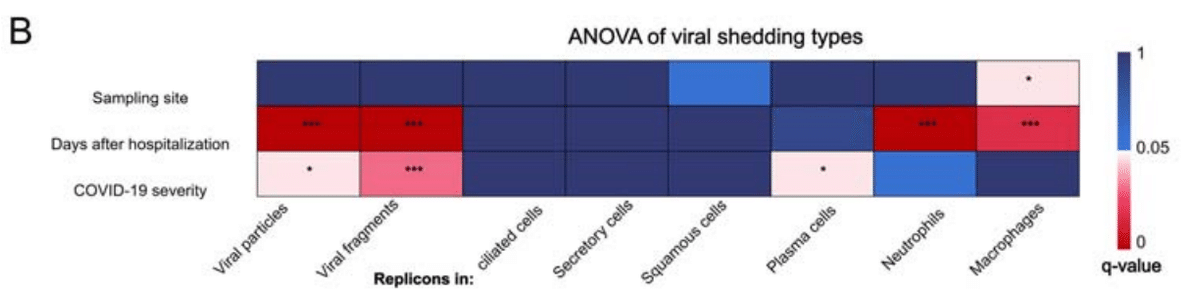
Therefore, we focus on analyzing individual cohorts to disregard the influence of other potential errors.
したがって、他の潜在的なエラーの影響を無視するために、個々のコホートの分析に焦点を当てます。
As the Wuhan cohort provided the clinical information of COVID-19 patients, i.e., days from patient hospitalization to sample collection and patients’ clinical status, we applied Fisher's exact test to evaluate whether significant changes exist between the first week of hospitalization and later (Figure 7A).
武漢コホートは COVID-19 患者の臨床情報 (患者の入院からサンプル採取までの日数と患者の臨床状態) を提供したため、入院後 1 週間とそれ以降の間に有意な変化があるかどうかを評価するためにフィッシャーの正確検定を適用しました (図 7A)。
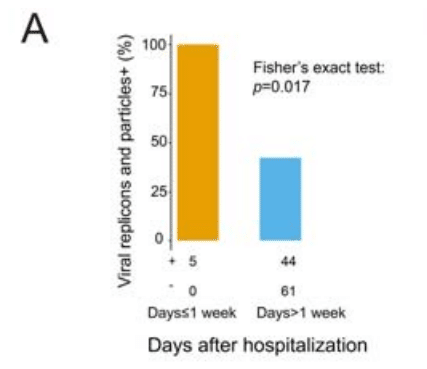
The result indicates that COVID-19 patients are highly infectious within the first week of hospitalization and the infectiousness decreased later.
この結果は、COVID-19 患者は入院後 1 週間は感染力が非常に強く、その後感染力が低下したことを示しています。
Consistently, upon further analysis of the changes in the proportion of viral replicons and viral fragments in total viral RNA, we observed a significant change in the proportion of viral replicons and viral fragments shed by COVID-19 patients one week after hospitalization (Figure 7B-7C).
一貫して、ウイルス RNA 全体におけるウイルス レプリコンとウイルス断片の割合の変化をさらに分析したところ、入院後 1 週間で COVID-19 患者から排出されるウイルス レプリコンとウイルス断片の割合に有意な変化が見られました (図 7B-7C)。
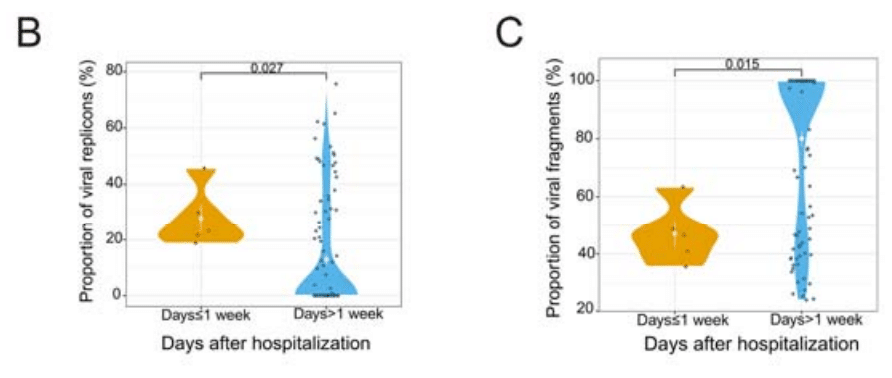
Patients tested early in hospitalization exhibit a higher proportion of viral replicons and a lower proportion of viral fragments compared to those tested later.
入院初期に検査を受けた患者は、後期に検査を受けた患者と比較して、ウイルスレプリコンの割合が高く、ウイルス断片の割合が低いことが示されています。
In another independent cohort, we observed that early hospitalization COVID-19 patients primarily shed viral replicons and particles, with their proportions significantly higher than viral fragments, consistent with the results from the Wuhan cohort (Figure 7D).
別の独立したコホートでは、早期入院の COVID-19 患者は主にウイルスレプリコンと粒子を排出しており、その割合はウイルス断片よりも大幅に高く、武漢コホートの結果と一致しています (図 7D)。
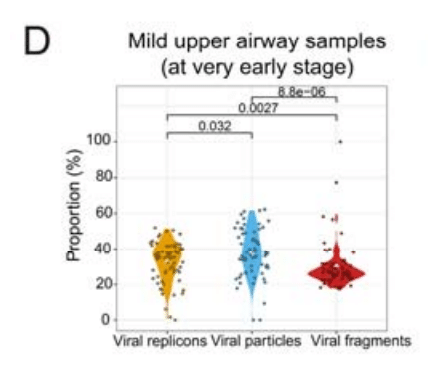
Notably, we observed that patients predominantly shed viral particles in the early stages of viral infection (Figure 7D).
特に注目すべきは、患者がウイルス感染の初期段階で主にウイルス粒子を排出していることがわかったことです (図 7D)。
Our findings provide molecular supports to the notion that COVID-19 patients are highly contagious during the initial week of hospitalization, and highlight the importance of early isolation measures for individuals displaying symptoms of COVID-19.
私たちの研究結果は、COVID-19 患者は入院後 1 週間は感染力が非常に強いという概念を分子レベルで裏付けるものであり、COVID-19 の症状を示す個人に対する早期隔離措置の重要性を強調しています。
Whether there is a difference in viral shedding between mild and severe COVID-19 patients is controversial.
軽症と重症の COVID-19 患者の間でウイルス排出に違いがあるかどうかは議論の余地があります。
To address this question, we compared the relative abundance of viral replicons and fragments in mild and severe patients using the Wuhan cohort (Figure 7E-7F).
この疑問に対処するために、武漢コホートを使用して、軽症と重症の患者におけるウイルスのレプリコンと断片の相対的な存在量を比較しました (図 7E-7F)。
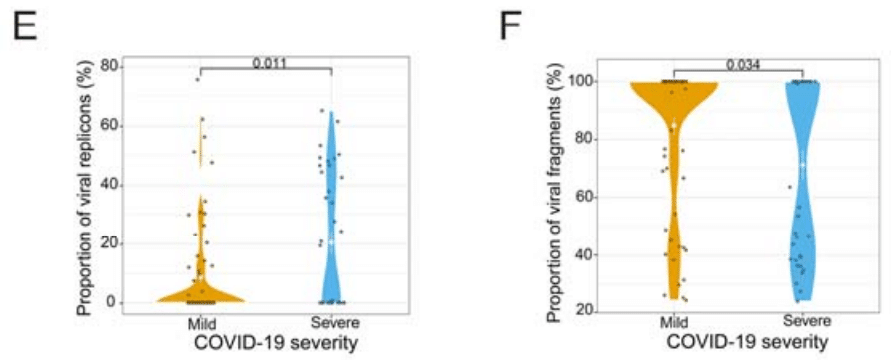
We excluded samples within the first week of hospitalization to avoid potential time-related effects.
時間に関連した影響の可能性を回避するため、入院後 1 週間以内のサンプルは除外しました。
We observed a significant variation in the relative abundance of viral replicons and fragments in severe patients compared to mild patients.
重症患者と軽症患者を比較すると、ウイルスレプリコンと断片の相対的な存在量に有意な差が見られました。
Compared to mild patients, the high proportion of viral replicons and low proportion of viral fragments in severe COVID-19 patients suggest that they may continue to shed infectious viral replicons in the late stages of infection.
軽症患者と比較すると、重症COVID-19患者ではウイルスレプリコンの割合が高く、ウイルス断片の割合が低いことから、感染後期でも感染性ウイルスレプリコンを排出し続ける可能性があることが示唆されます。
The underlying cause for this difference may be the immunological dysregulation in severe patients, preventing the complete clearance of the virus from the body.
この差の根本的な原因は、重症患者の免疫調節異常により、ウイルスが体内から完全に排除されないことにあると考えられます。
Therefore, our results indicate that the severity of the disease is also a crucial factor influencing viral shedding.
したがって、私たちの結果は、病気の重症度もウイルス排出に影響を与える重要な要因であることを示しています。
Based on these results, we summarized the pattern and type of viral shedding in COVID-19 patients at different time points (Figure 7G).
これらの結果に基づいて、COVID-19 患者のさまざまな時点でのウイルス排出のパターンとタイプをまとめました (図 7G)。
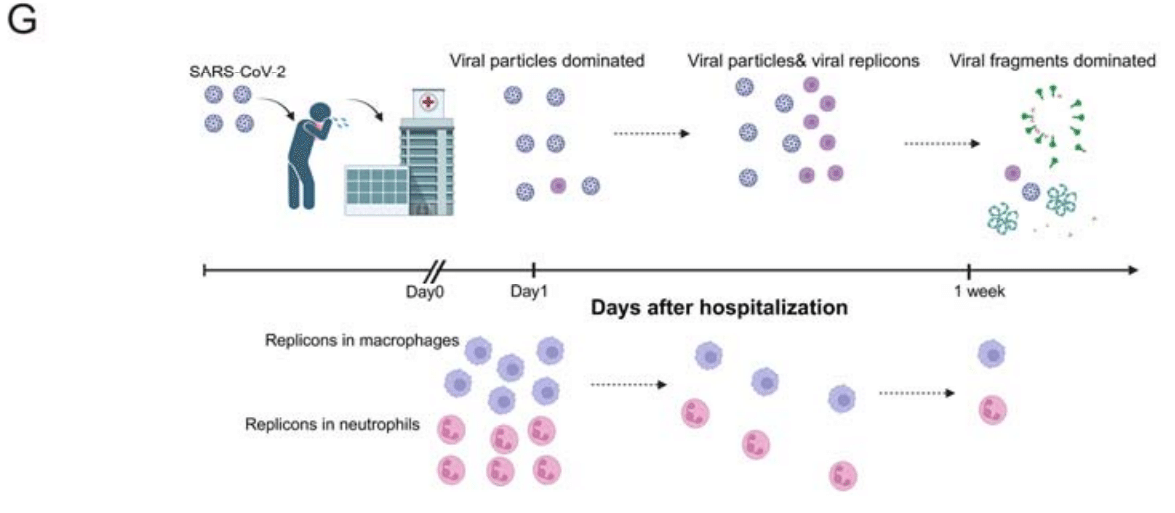
Viral particle shedding dominated in the early stages of hospitalization, transitioning to viral replicons and particles as the disease progressed.
入院初期にはウイルス粒子の排出が主流でしたが、病気が進行するにつれてウイルスのレプリコンと粒子に移行しました。
One week after hospitalization, patients predominantly shed viral fragments as their infectivity is significantly reduced.
入院後 1 週間で、感染力が大幅に低下したため、患者は主にウイルスの断片を排出します。
Discussion
Understanding the biological characteristics of SARS-CoV-2 shedding in the respiratory tract is crucial for formulating more precise control policies in the future.
呼吸器系における SARS-CoV-2 排出の生物学的特徴を理解することは、将来、より正確な制御ポリシーを策定するために不可欠です。
Despite extensive research on the abundance and characteristics of SARS-CoV-2 shedding, determining the infectivity of specific COVID-19 patients remains challenging.
SARS-CoV-2 排出の豊富さと特徴に関する広範な研究にもかかわらず、特定の COVID-19 患者の感染性を判断することは依然として困難です。
While viral load is commonly used as a surrogate for infectious viral particles, it only indicates the presence of viral RNA in the sample, not infectivity.
ウイルス量は感染性ウイルス粒子の代替として一般的に使用されていますが、サンプル内のウイルス RNA の存在を示すだけで、感染性を示すものではありません。
The isolation and quantification of live virus require specialized personnel, equipment, and facilities, as well as strict sample preservation and transportation protocols, making it impractical for studying viral shedding in large patient cohorts.
生きたウイルスの分離と定量化には、専門の人員、機器、施設、および厳格なサンプル保存と輸送プロトコルが必要であるため、大規模な患者コホートでのウイルス排出を研究することは現実的ではありません。
Therefore, there is an urgent need for new methods to provide innovative directions for studying viral shedding issues.
したがって、ウイルス排出の問題を研究するための革新的な方向性を提供する新しい方法が緊急に必要です。
High-throughput sequencing of viral RNA provides a potential solution, offering quantitative information on the entire viral transcriptome with advantages such as simplicity and cost-effectiveness.
ウイルスRNAのハイスループットシーケンシングは、シンプルさや費用対効果などの利点を備え、ウイルストランスクリプトーム全体に関する定量的な情報を提供する潜在的なソリューションを提供します。
Yet, whole transcriptome sequencing data is complex and requires specific tools for precise decoding of shedding patterns.
しかし、トランスクリプトーム全体のシーケンシングデータは複雑であり、排出パターンを正確に解読するには特定のツールが必要です。
This study establishes the correlation between metatranscriptomic data from respiratory samples of COVID-19 patients and SARS-CoV-2 viral shedding.
この研究は、COVID-19 患者の呼吸器サンプルからのメタトランスクリプトミクス データと SARS-CoV-2 ウイルス排出との相関関係を確立します。
Unsupervised machine learning methods help identify potential viral shedding patterns.
教師なし機械学習法は、潜在的なウイルス排出パターンを特定するのに役立ちます。
Building upon this, we introduce a tool called RedeCoronaVS.
これを基に、RedeCoronaVS というツールを紹介します。
Developed based on linear programming models, RedeCoronaVS achieves deconvolution of sequencing data by incorporating pre-existing information to estimate the abundance of different viral shedding patterns.
線形計画モデルに基づいて開発された RedeCoronaVS は、既存の情報を組み込んでさまざまなウイルス排出パターンの量を推定することで、シーケンス データのデコンボリューションを実現します。
Furthermore, RedeCoronaVS enhances our understanding of viral shedding in COVID-19 patients.
さらに、RedeCoronaVS は COVID-19 患者のウイルス排出に関する理解を深めます。
Viral replicons are correlated with the sampling time and clinical status of COVID-19 patients.
ウイルス レプリコンは、COVID-19 患者のサンプリング時間と臨床状態と相関しています。
Specific types of viral replicons may represent critical clinical information, and they tend to co-shed with viral particles.
特定の種類のウイルス レプリコンは重要な臨床情報を表す可能性があり、ウイルス粒子と一緒に排出される傾向があります。
Our results indicate that patients shed a significant amount of infectious virus within the first week of hospitalization.
私たちの結果は、患者が入院後 1 週間以内にかなりの量の感染性ウイルスを排出することを示しています。
In severe cases, patients may continue shedding the virus during the late stages of infection, suggesting disease severity is an important factor affecting viral shedding.
重症の場合、患者は感染後期でもウイルスを排出し続ける可能性があり、これは病気の重症度がウイルス排出に影響を与える重要な要因であることを示唆しています。
In summary, our study presents RedeCoronaVS as a valuable tool for detecting and quantifying viral shedding patterns in COVID-19 patients.
要約すると、私たちの研究は、RedeCoronaVS が COVID-19 患者のウイルス排出パターンを検出し、定量化するための貴重なツールであることを示しています。
By analyzing metatranscriptomic sequencing data of SARS-CoV-2 from hundreds of COVID-19 respiratory samples, RedeCoronaVS enables the identification of the specific types and abundance of viral presence, thereby providing insights into the disease progression in patients.
RedeCoronaVS は、数百の COVID-19 呼吸器サンプルから SARS-CoV-2 のメタトランスクリプトーム シーケンス データを分析することで、ウイルスの存在の特定のタイプと量を特定し、患者の病気の進行に関する洞察を提供します。
Importantly, RedeCoronaVS is highly scalable and holds potential for application in other coronavirus diseases, making it a valuable tool for future responses to emerging respiratory infectious diseases.
重要なのは、RedeCoronaVS が非常に拡張性が高く、他のコロナウイルス疾患にも応用できる可能性があることです。そのため、今後、新興の呼吸器感染症に対応するための貴重なツールとなります。
Limitations of the study
Due to the challenges in directly dissecting intact viral particles, viral replicons within host cells, and viral fragments from respiratory specimens of large cohorts of COVID-19 patients, it is difficult to validate our results with current experimental protocols.
COVID-19 患者の大規模コホートの呼吸器検体から、無傷のウイルス粒子、宿主細胞内のウイルスレプリコン、ウイルス断片を直接分析することが困難なため、現在の実験プロトコルで結果を検証することは困難です。
Another limitation is the absence of information regarding the precise onset time of COVID-19 in patients.
もう 1 つの制限は、患者における COVID-19 の正確な発症時間に関する情報がないことです。
This study relies on the patients' hospitalization records to estimate the real onset time, potentially leading to underestimation and uncertainty.
この研究では、実際の発症時間を推定するために患者の入院記録に依存しているため、過小評価や不確実性につながる可能性があります。
以下省略。
この記事が気に入ったらサポートをしてみませんか?