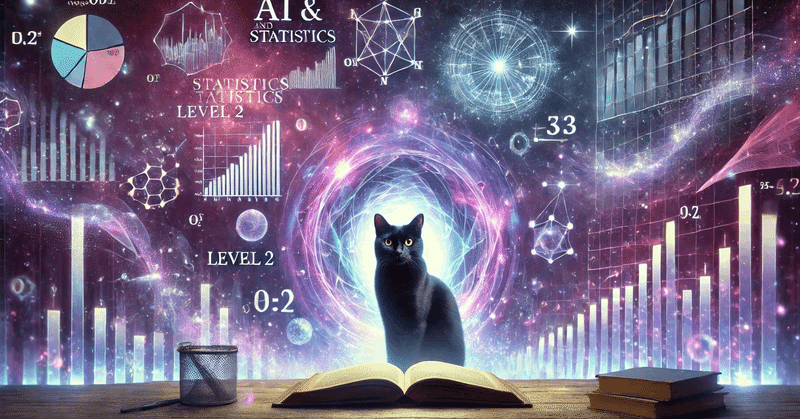
AI×統計:検定2級合格への意外な学習法
近頃、生成AIを活用して統計学や機械工学の勉強を進めています。その中で、統計検定2級を取得したいという思いが次第に強くなり、統計学へのコミットメントを増やしながら学習を進めることにしました。
とはいえ、前回の記事で触れたように、機械工学と英語の学習も引き続き取り組みたいと考えています。そこで今回は、前回の記事の方法をさらに発展させ、統計検定2級に焦点を当てた学習法について考えていきます。
まずは統計検定2級の試験範囲についてのPDFをAIに読み込ませ、学習内容を整理しました。
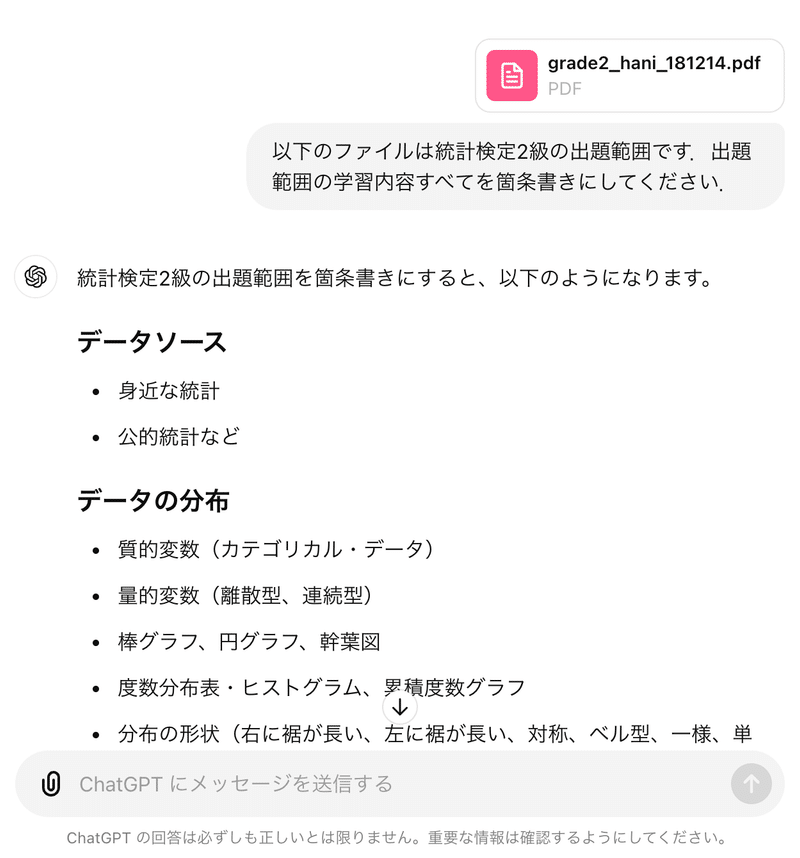
その後、これらの要素を含む自動車に関連する英語の問題をAIに作成してもらいました。
以下の要素をすべて含んだ究極の1問を作成してください.
#制約
・自動車に関連する問題にすること
・英語で出題すること
・実際の数値を用いた問題にすること
#入力
*
* ### データソース
* - 身近な統計
* - 公的統計など
*
* ### データの分布
* - 質的変数(カテゴリカル・データ)
* - 量的変数(離散型、連続型)
* - 棒グラフ、円グラフ、幹葉図
* - 度数分布表・ヒストグラム、累積度数グラフ
* - 分布の形状(右に裾が長い、左に裾が長い、対称、ベル型、一様、単峰、多峰)
*
* ### 中心傾向の指標
* - 平均値、中央値、最頻値(モード)
*
* ### 散らばりなどの指標
* - 分散(n-1で割る)
* - 標準偏差
* - 範囲(最小値、最大値)
* - 四分位範囲、箱ひげ図
* - ローレンツ曲線、ジニ係数
* - 2つのグラフの視覚的比較
* - カイ二乗値(一様な頻度からのずれ)
* - 歪度、尖度
*
* ### 中心と散らばりの活用
* - 偏差、標準化(z得点)、変動係数、指数化
*
* ### 散布図と相関
* - 散布図、相関係数、共分散
* - 層別した散布図、相関行列
* - みかけの相関(擬相関)、偏相関係数
*
* ### カテゴリカルデータ
* - 度数表、2元クロス表
*
* ### 単回帰と予測
* - 最小二乗法、変動の分解、決定係数、回帰係数
* - 分散分析表、観測値と予測値、残差プロット、標準誤差、変数変換
*
* ### 時系列データの処理
* - 成長率、指数化、幾何平均、系列相関・コレログラム、トレンド、平滑化(移動平均)
*
* ### 観察研究と実験研究
* - 観察研究、実験研究、調査の設計
* - 母集団、標本、全数調査、標本調査、ランダムネス、無作為抽出
*
* ### 標本調査と無作為抽出
* - 標本サイズ(標本の大きさ)、標本誤差、偏りの源
* - 標本抽出法(系統抽出法、層化抽出法、クラスター抽出法、多段抽出法)
*
* ### 実験
* - 実験のデザイン(実験計画)、フィッシャーの3原則
*
* ### 確率
* - 事象と確率、加法定理、条件付き確率、乗法定理、ベイズの定理
*
* ### 確率変数
* - 離散型確率変数、連続型確率変数
* - 確率変数の期待値・分散・標準偏差
* - 確率変数の和と差(同時分布、和の期待値・分散)
* - 2変数の共分散・相関
*
* ### 確率分布
* - ベルヌーイ試行、二項分布、ポアソン分布、幾何分布
* - 一様分布、指数分布、正規分布、2変量正規分布、超幾何分布、負の二項分布
*
* ### 推測統計の基礎
* - 標本分布、独立試行
* - 標本平均の期待値・分散
* - チェビシェフの不等式、大数の法則、中心極限定理
* - 二項分布の正規近似、連続修正、母集団、母数(母平均、母分散)
*
* ### 正規母集団に関する分布
* - 標準正規分布、標準正規分布表の利用
* - t分布、カイ二乗分布、F分布、分布表の活用、上側確率点(パーセント点)
*
* ### 点推定と区間推定
* - 点推定、推定量と推定値
* - 有限母集団、一致性、不偏性、信頼区間、信頼係数
*
* ### 1つの母集団の母数の区間推定
* - 正規母集団の母平均・母分散の区間推定
* - 母比率の区間推定、相関係数の区間推定
*
* ### 2つの母集団の母数の区間推定
* - 正規母集団の母平均の差・母分散の比の区間推定
* - 母比率の差の区間推定
*
* ### 統計的検定
* - 仮説検定の理論、p値、帰無仮説(H0)と対立仮説(H1)
* - 両側検定と片側検定、第1種の過誤と第2種の過誤、検出力
*
* ### 1つの母集団の母数に関する仮説検定
* - 母平均の検定、母分散の検定、母比率の検定
*
* ### 2つの母集団の母数に関する仮説検定
* - 母平均の差の検定(分散既知、分散未知であるが等分散、分散未知で等しいとは限らない場合)
* - 母分散の比の検定、母比率の差の検定
*
* ### 適合度検定と独立性の検定
* - 適合度検定、独立性の検定
*
* ### 回帰分析
* - 回帰直線の傾きの推定と検定、重回帰モデル
* - 偏回帰係数、回帰係数の検定、多重共線性
* - ダミー変数を用いた回帰、自由度調整(修正)済み決定係数
*
* ### 実験計画の概念の理解
* - 実験、処理群と対照群、反復、ブロック化、一元配置実験
* - 3群以上の平均値の差(分散分析)、F比
*
* ### 統計ソフトウェアの活用
* - 計算出力を活用できるか、問題解決に活用できるか
試験範囲の順番に学習するのが基本ですが、実際の試験では順番に出題されるわけではありませんし、現実の問題でも必要な知識を選んで使うことが求められます。そこで、問題にはランダム性や網羅性を持たせる必要があると考えました。
以下がAIが生成した問題です↓
Ultimate Question: Automotive Engineering Statistical Analysis
You are an automotive engineer tasked with analyzing the performance and reliability of different steel types used in manufacturing car chassis. Your goal is to determine the most suitable steel type based on various statistical analyses.
Data Sources
You have collected data from the following sources:
Public Statistics: Industry standards and material databases such as MatWeb and ASM International.
Field Studies: Real-world data collected from car chassis tested under various conditions.
Data Collected
The data includes tensile strength, yield strength, and ductility for three types of steel (Steel A, Steel B, and Steel C). You also have frequency distributions for the number of defects found in cars using each steel type after one year of use.
Data Summary
Steel Type Tensile Strength (MPa) Yield Strength (MPa) Ductility (%) Number of Defects (per 100 cars)
Steel A 800 600 15 10
Steel B 1000 850 10 5
Steel C 600 400 20 15
Problem Statement
Data Distribution:
Create a bar graph showing the tensile strength, yield strength, and ductility for each steel type.
Draw a histogram of the number of defects for each steel type.
Measures of Central Tendency:
Calculate the mean, median, and mode for the tensile strength of the three steel types.
Determine the range, variance, and standard deviation for the yield strength of the steel types.
Scatterplot and Correlation:
Create a scatterplot showing the relationship between tensile strength and ductility for the three steel types.
Calculate the correlation coefficient between tensile strength and ductility.
Regression Analysis:
Perform a simple linear regression to predict the number of defects based on tensile strength. Provide the regression equation and interpret the slope.
Hypothesis Testing:
Conduct a hypothesis test to determine if there is a significant difference in the mean number of defects between Steel A and Steel B. Use a 5% significance level and state the null and alternative hypotheses, test statistic, p-value, and conclusion.
Confidence Intervals:
Calculate the 95% confidence interval for the mean tensile strength of Steel B.
Decision Making:
Based on your analyses, recommend the best steel type for manufacturing car chassis, considering both performance (tensile strength, yield strength, ductility) and reliability (number of defects).
実際に問題を解くのは次回以降にしたいと思います。この方法でしばらく学習を続け、より効果的な勉強法を見つけていきたいです。
この記事を通じて、私の学習方法や統計検定2級への挑戦が、皆さんの学び方の参考になれば幸いです。
この記事が気に入ったらサポートをしてみませんか?