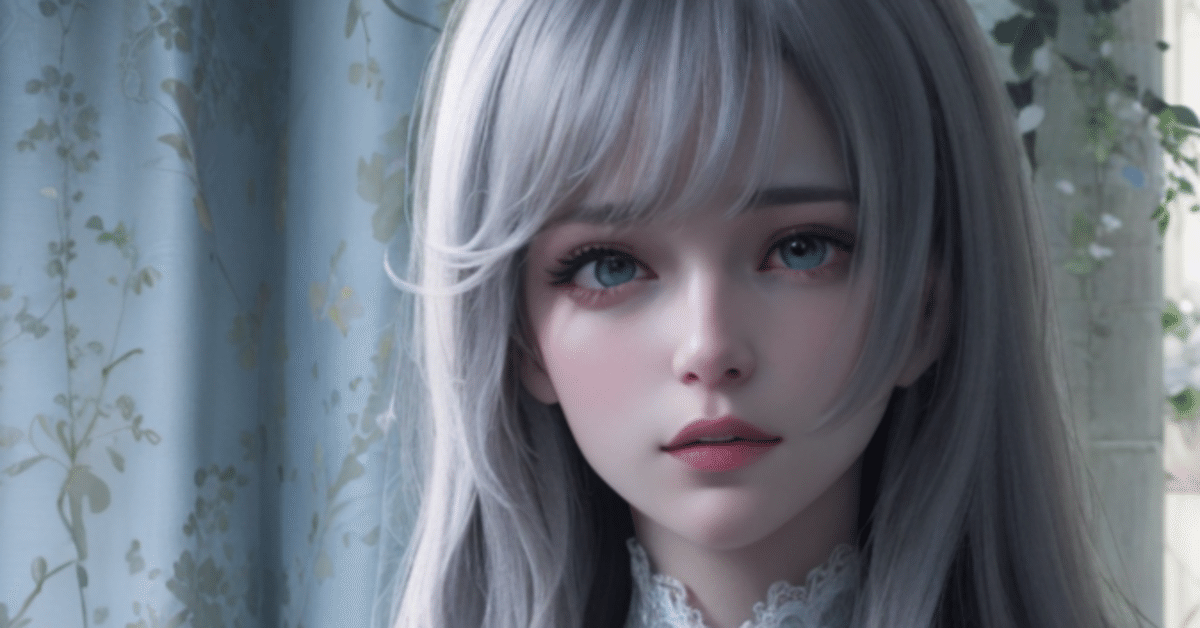
Summary of Insights from Training High-poly LoRA ver.2
日本語版はこちら: https://note.com/takumi__ncr/n/n2fb9d265ffa9
This article summarizes the insights gained during the training of High-poly LoRA ver.2, focusing on the impact of training resolution.
Due to the length of the article, only the conclusions regarding resolution are presented first.
Increasing training resolution may affect the training results
However, the effects may not always be desirable, so it is necessary to choose the appropriate resolution based on the training objectives
(Note: Due to the note's specification, banding appears to be occurring in the images within the article. This is a significant issue for an article that verifies the effects of details, but please understand. You can check the original sample images on Civitai's LoRA distribution page.)
About the Training of High-poly LoRA ver.2
High-poly LoRA is a LoRA that uses high-polygon 3DCG still images as training materials.
The decision to create ver.2 was made because ver.1 felt insufficient in terms of reproducing 3D-like texture and stereoscopic feeling.
The main changes from ver.1 are as follows:
Added training images (ver.1: 30 images, ver.2: 160 images)
Tag organization and removal of trigger tag
Adjustment of training parameters
Reduction of dim
Adjustment of learning rate
Adjustment of the number of training steps
Improved training resolution (ver.1: 768, ver.2: 896)
Seven combinations were tested, with the seventh combination finally being selected.
([Base Learning Model] - [Training Resolution] - [dim])
basil - 640 - 32
nai-sfw - 768 - 32
nai-sfw - 768 - 64
dalcefo_paintingV3 - 1024 - 64
AOM2 - 896 - 64
AOM2 - 1024 - 128
nai-sfw - 896 - 64
The differences between generated images using ver.1 and ver.2 under the same conditions are shown below.
Compared to ver.1, ver.2 can better express 3D-like skin material, hair strands, and intricate lace expressions. Also, the beautiful lighting is fully demonstrated.
Above all, the face is shockingly to the author's taste.
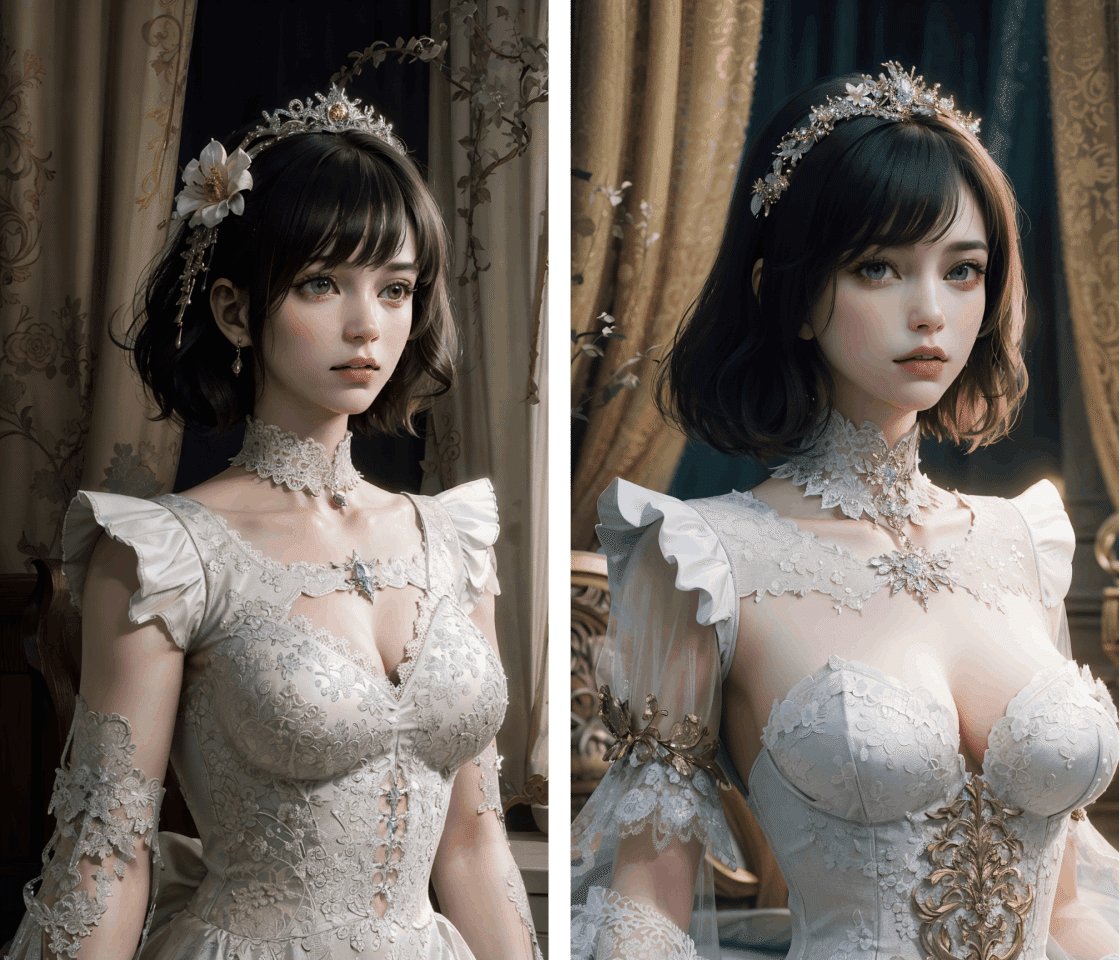
The Influence of Training Resolution
To illustrate the overall characteristics of the dataset and the training objectives, an example using my self-made 3D model is provided.
(Note: The actual training images have much higher quality.)
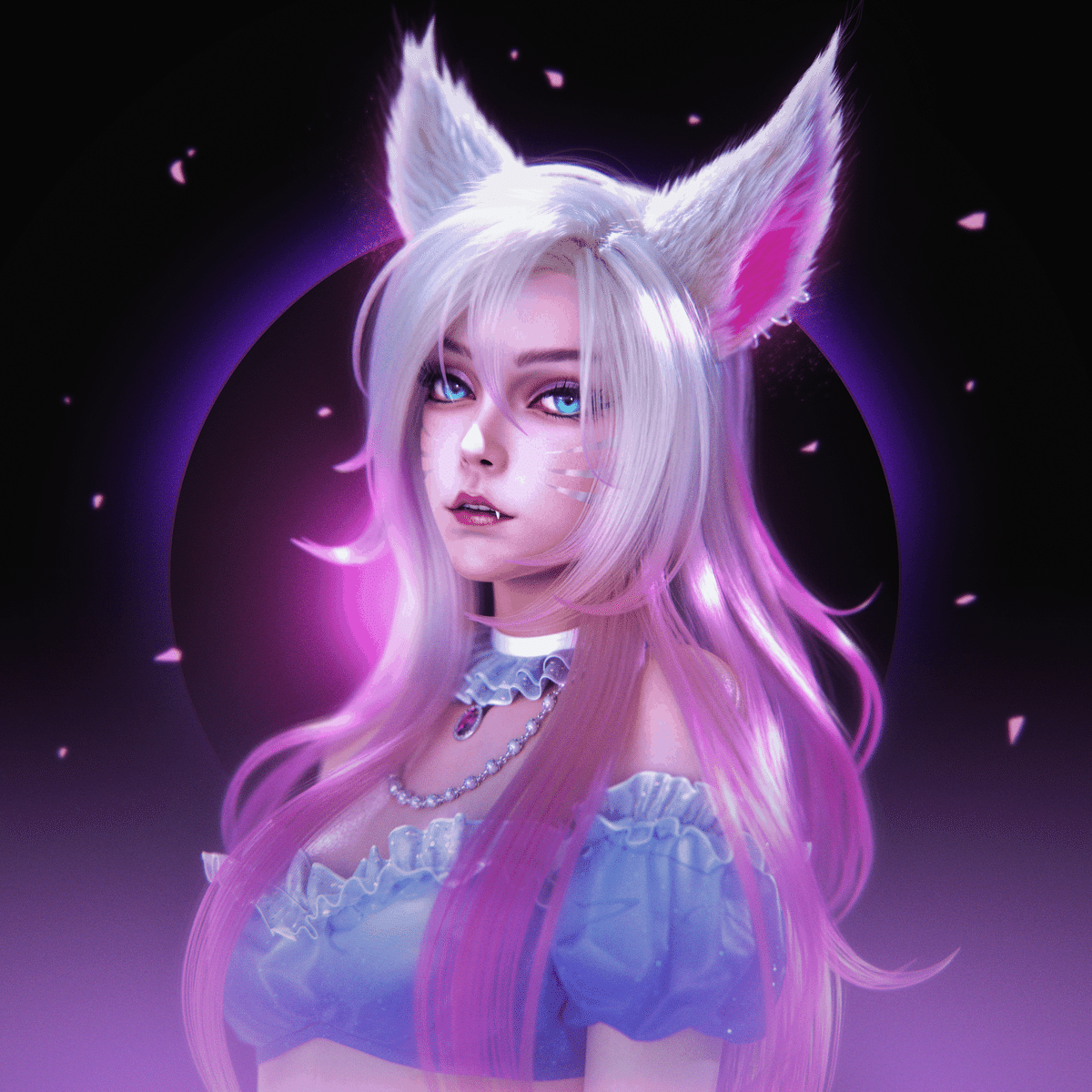
If you are interested in the technical aspects of 3D itself, please refer to my previously article.
Improvements from Increasing Resolution
In order to reproduce 3D-like textures, it was considered important whether the high-frequency factors (i.e., details that would be lost at lower resolutions) could be learned.
The dataset used in this time generally emphasizes the high-frequency factors of skin and clothing textures, such as bumps and highlights, to create a clean and eye-catching appearance.
Hair is also often designed to make flow and silhouette clearly visible, with more distinct strands than in reality.
Similar techniques were intentionally used when creating my own model (regardless of success or not).
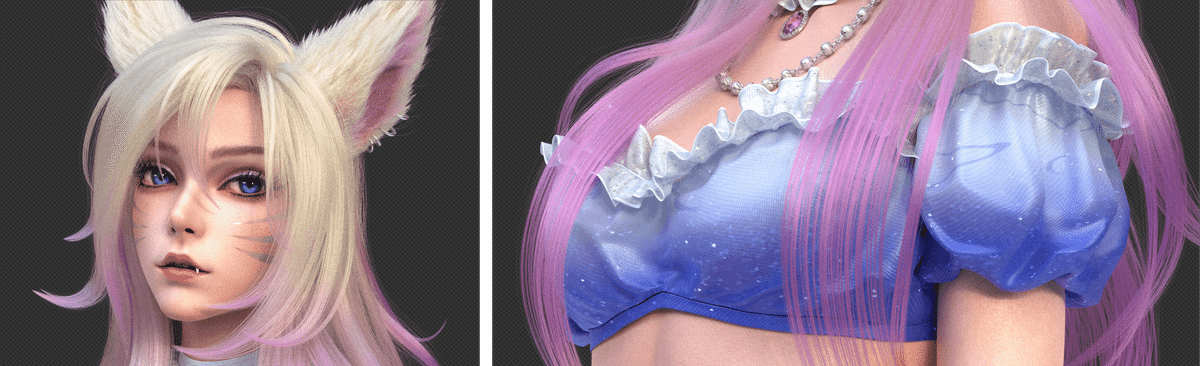
By training with combination 7 (resolution 896), these fine features were successfully captured. It seems that increasing resolution can be effective when the details of textures are important.
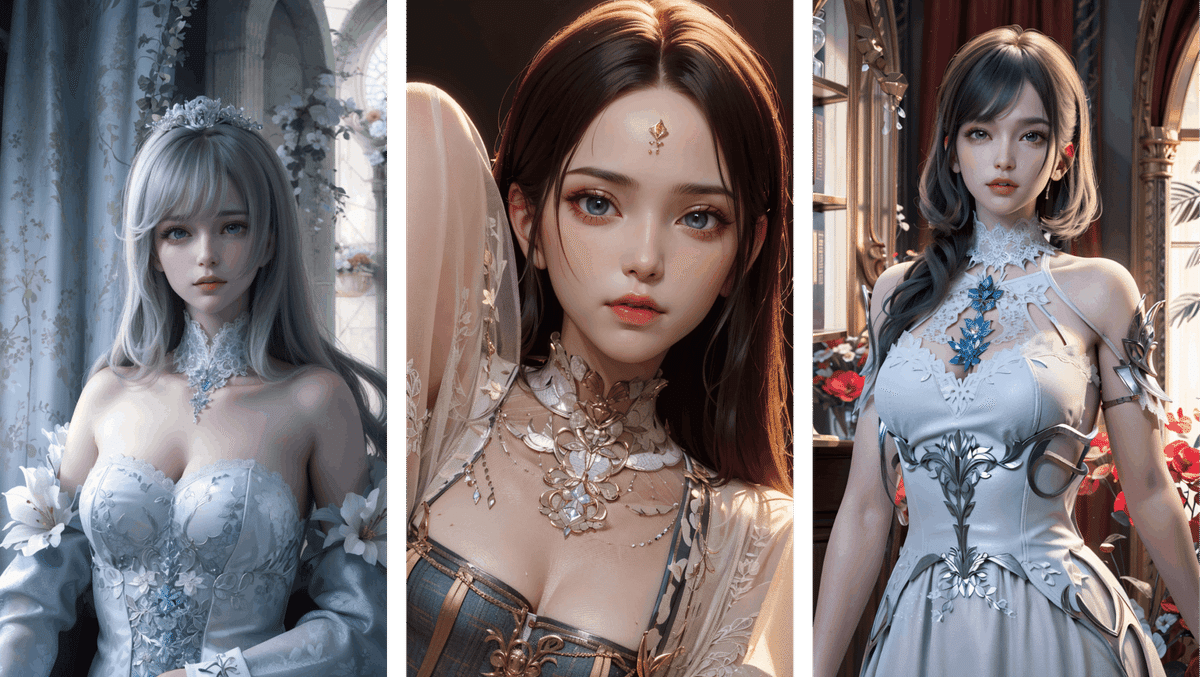
Points That Did Not Change with Increased Resolution
In cases where the face area in the image is small, such as in full-body compositions, there is a tendency for facial symmetry to be compromised. Since training images generally have tall proportions and small faces in full-body images, it was thought that increasing the training resolution might improve facial shape even if it is small in an image.
However, there was little improvement in this regard, even when the resolution was increased. Also, no improvement was observed in the accuracy of arms and legs.
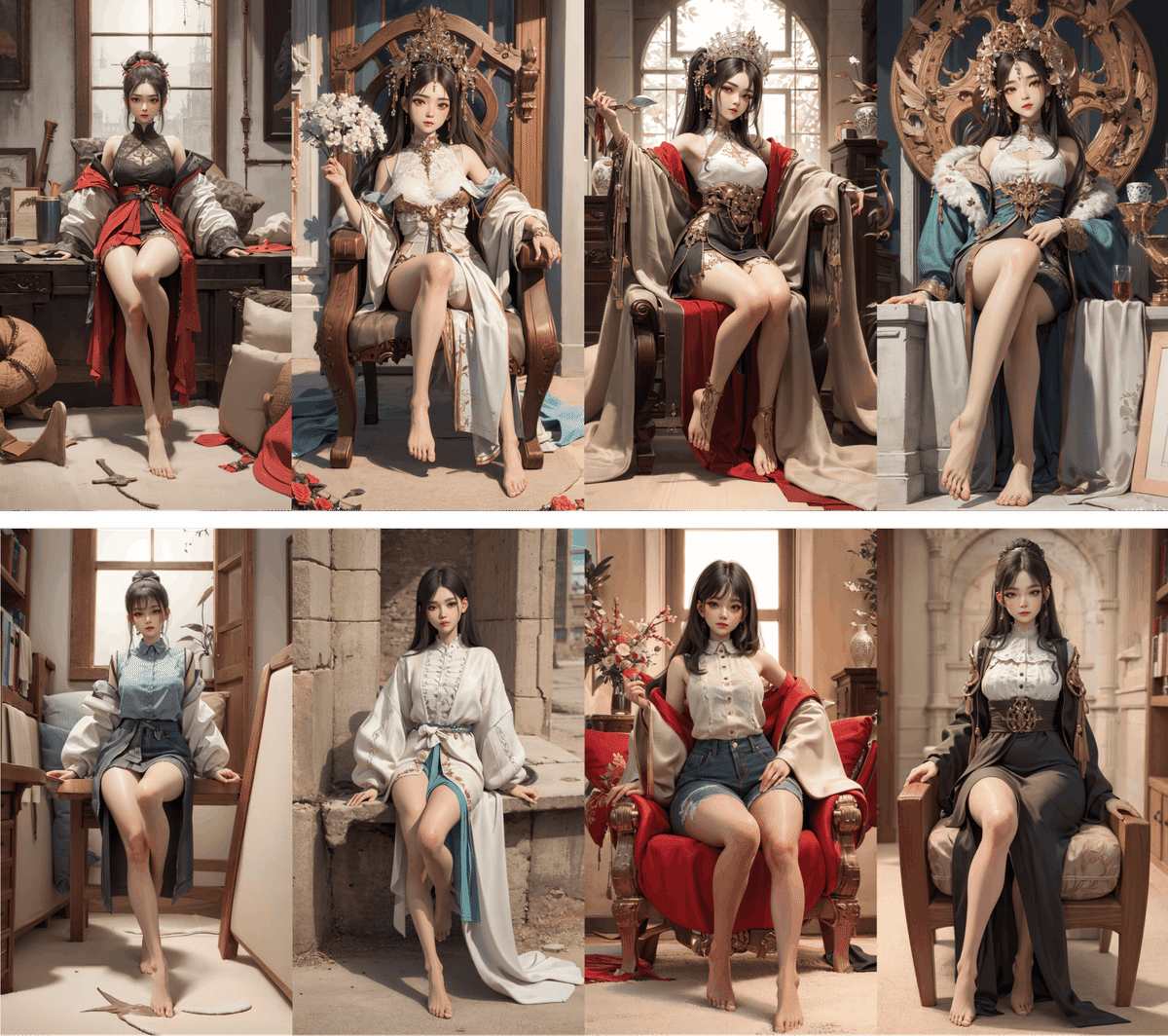
Maintaining consistency in small areas is a known weakness of the current Stable Diffusion, and it seems difficult to address this issue with LoRA. Additionally, since faces can be easily corrected using inpaint, it was ultimately decided to exclude this point from the learning objectives.
Deterioration from Increasing Resolution
In photorealistic 3D, it is common to represent peachfuzz on the skin surface, as shown in the image below.
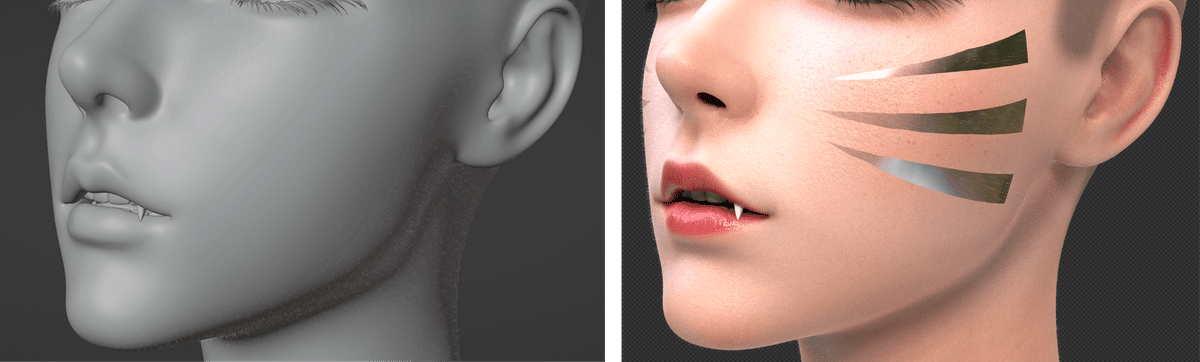
When the training resolution was increased to 1024, this factor was learned. However, the peachfuzz representation that appears in the below circled area in the AI-generated image does not look good, appearing as spiky or partially trembling.
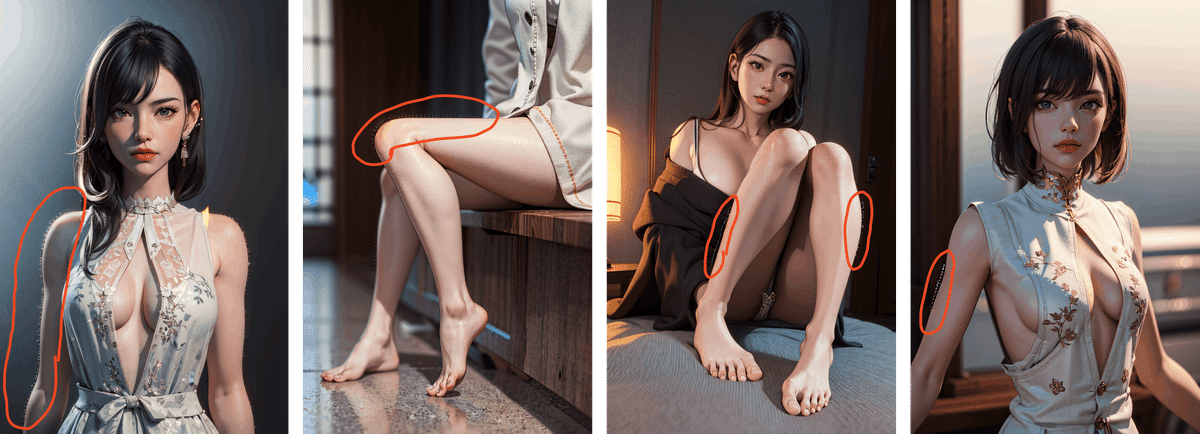
Increasing the resolution may inadvertently cause to learn unintended fine elements, potentially degrading the quality of the image as a result. Considering these results, the final learning resolution of 896 was chosen.
About Tagging
In order to conduct training using the DreamBooth with caption, WD Tagger was used for automatic tagging, followed by manual tag organization. Particular attention was paid to the following points:
Hijacking existing tags as a replacement for the trigger tag
In ver.1, the trigger tag was specified, but it was removed after learning that it was not necessary for an art style LoRA. Instead, the existing tags 3d and realistic were specified, with the intention of partially absorbing the art style into these tags and making it possible to adjust through the prompts. The --keep_tokens option was not used and the tags were treated as general tags.
As a result, this was effective, and by specifying 3d and realistic in the Positive Prompt, the art style became more 3D-textured and stereoscopic, while specifying them in Negative resulted in less intrusion of the original model's style, making it possible to enhance details such as costumes.
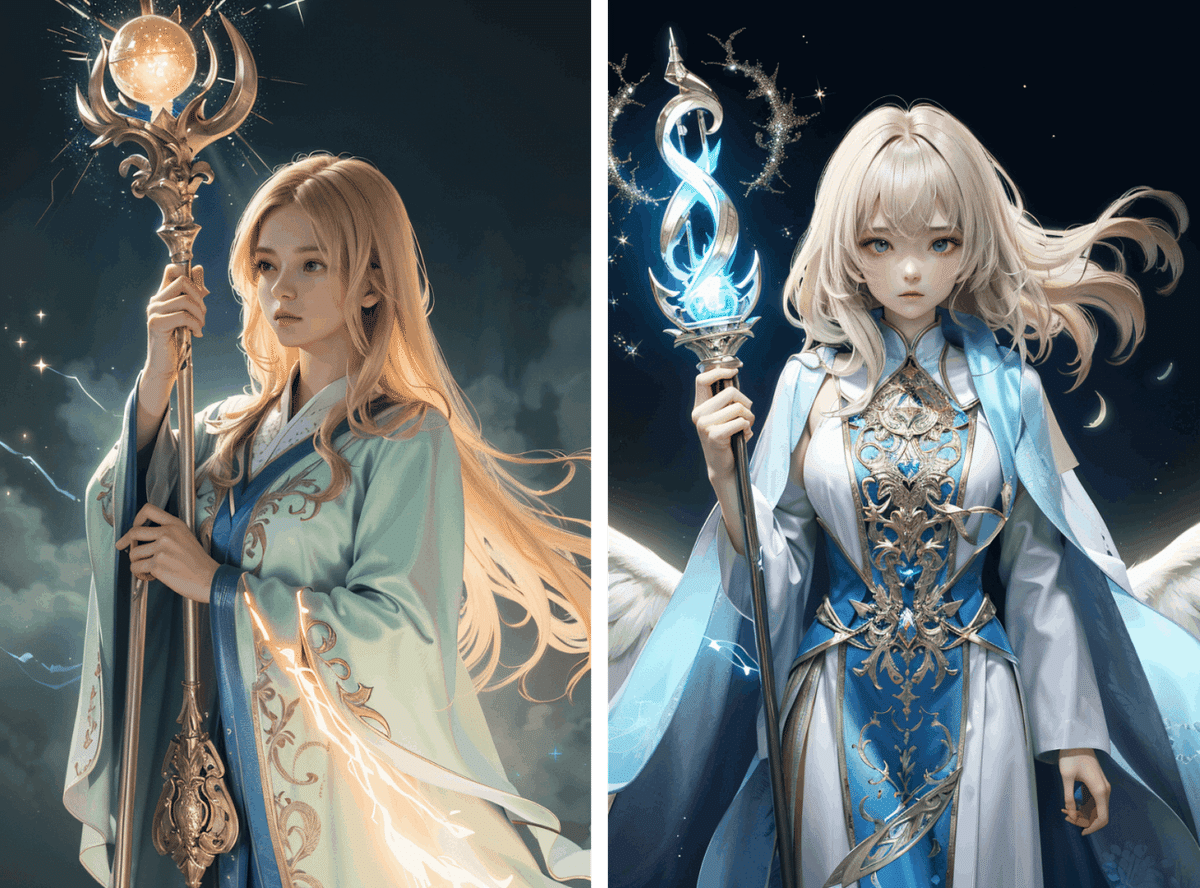
Addionaly, in ver.1, there was an issue where including words like detailed and intricate in the prompt would significantly shift the art style towards realistic. In ver.2, this phenomenon seems to be mitigated. However, it is unclear that this hijacking affected the issue.
Tags related to posing
To avoid fixed poses and camera positions, related tags were manually added and modified. Particular attention was paid to the following tags:
looking at viewer
When the face is roughly facing forward, the looking at viewer tag is automatically added with high probability. However, if this tag remains even when the gaze is not actually directed at the viewer, it often results in the eyes not meeting the camera when specifying looking at viewer during image generation. Therefore, tags such as looking away or looking to the side were manually added to accurately represent the direction of the gaze.from side, from behind
If tags specifying camera positions other than the front are not added, the camera position may become biased. Therefore, these tags were manually added correctly.hand on xxx, sitting, standing
These tags are related to hand gestures and posture. Automatic tagging does not often add hand position tags, which can lead to fixed posing. Therefore, tags such as hand on own face and hand on hip were manually specified. sitting and standing tags were generally accurately added by automatic tagging, but if incorrect, they were corrected manually.
Tags related to the background
As most of the training images had a single-color or gradient background, the simple background and gradient background tags were specified to prevent the background from being fixed. The success rate of automatic tagging was about 70%, so manual checks were performed.
When generating images, specifying simple background in the Negative Prompt reduces the possibility of single-color backgrounds. If a more specific background information is described in the Positive Prompt, the image will generally follow the description.
Merging duplicated tags
When using automatic tagging, particularly for clothing, accessories, and belongings, multiple tags with overlapping meanings are easily added (e.g., shirt, white shirt, collared shirt). It is suggested that these tags should be merged into a single tag (in the above example, white collared shirt).
However, due to the large number of images and tags in this project, manual operation was abandoned. Reportedly, it is possible to instruct a chat AI to merge overlapping concepts into a single tag, which may be attempted in the future.
About dim
Comparing Combination 2 (nai-sfw - res: 768 - dim: 32) and 3 (nai-sfw - res: 768 - dim: 64), it appears that dim: 64 learns a slightly more textured and stereoscopic appearance. Although dim: 32 is sufficient for learning the silhouette and ornaments of the costume, it is possible that a higher dim value is needed for learning factors in the smaller areas.
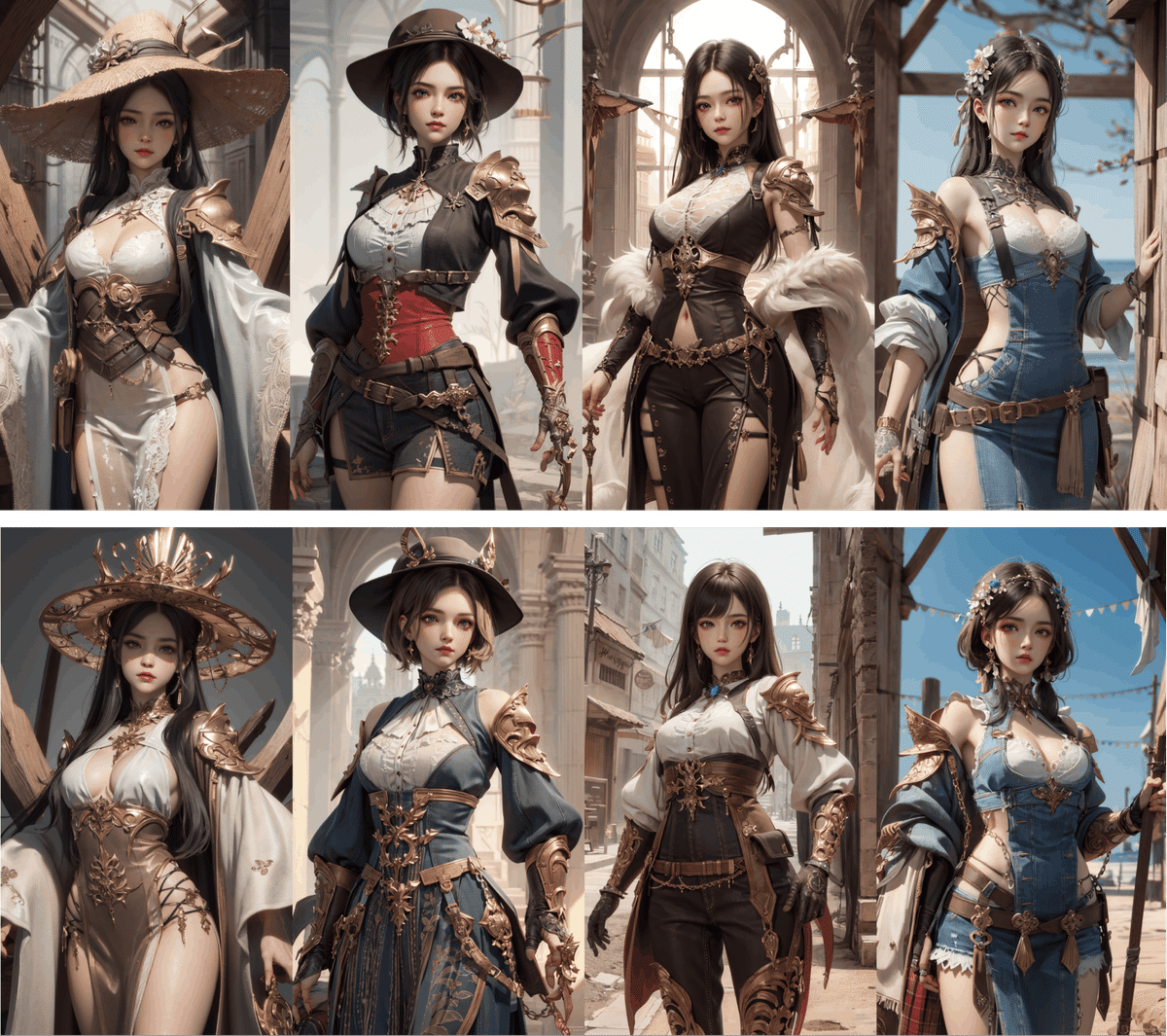
However, comparing Combination 3 and 7 (nai-sfw - res: 896 - dim: 64), a more significant improvement in texture quality is observed when increasing the resolution, suggesting that prioritizing resolution over dim yields greater results.
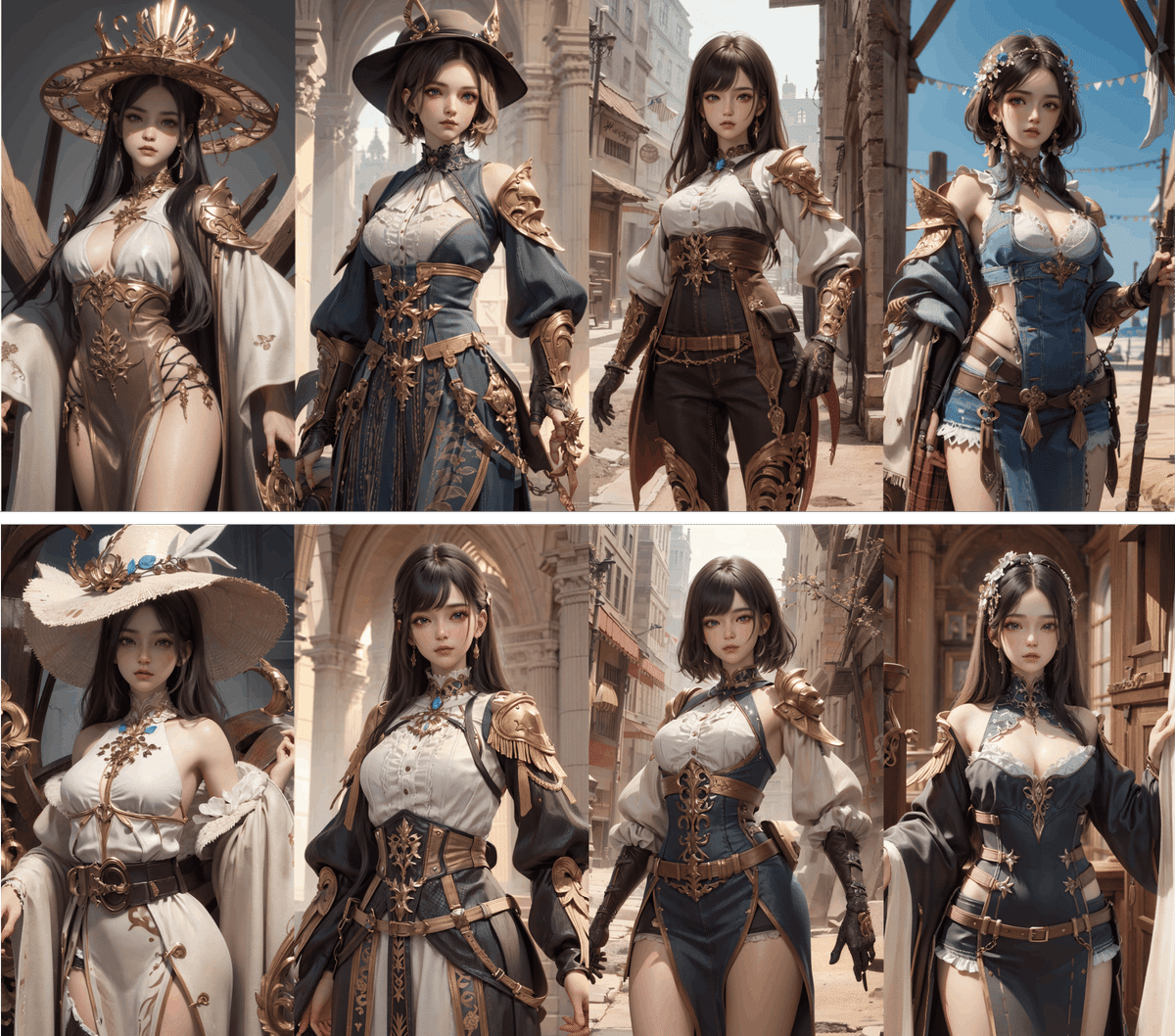
About choosing the learning base model
Experiments were conducted with changing the training base model based on a hypothesis considering the characteristics of each model.
The hypotheses assumes the use of my custom merge model.
basil
Hypothesis: Since the teaching images have details closer to real-life images than anime images, using the photoreal model basil may more effectively learn the features.
Result: LoRA effects were achieved for photoreal models such as Chillout, but the effect was not as evident for anime models.dalcefo_paintingV3
Hypothesis: One of the parent models in my custom merge model used for verification is dalcefo_paintingV3. Using a training model close to the generation model might make it easier to reflect only the features learned in LoRA.
Result: A phenomenon where the model's art style reverted back to its merged parent model, personally called "regression", occurred, making it impossible to produce the desired style.AOM2
Hypothesis: If a anime model with better expressive power than nai is used, it may more easily pick up features of the training images. Since it is distantly related to the custom merge model in terms of lineage, "regression" might be less likely to occur.
Result: Although the results showed good learning of costume details and silhouettes, there were issues such as fixed composition, distortion of faces and bodies, and inability to fully capture texture, making it unsuitable for the custom merge model.
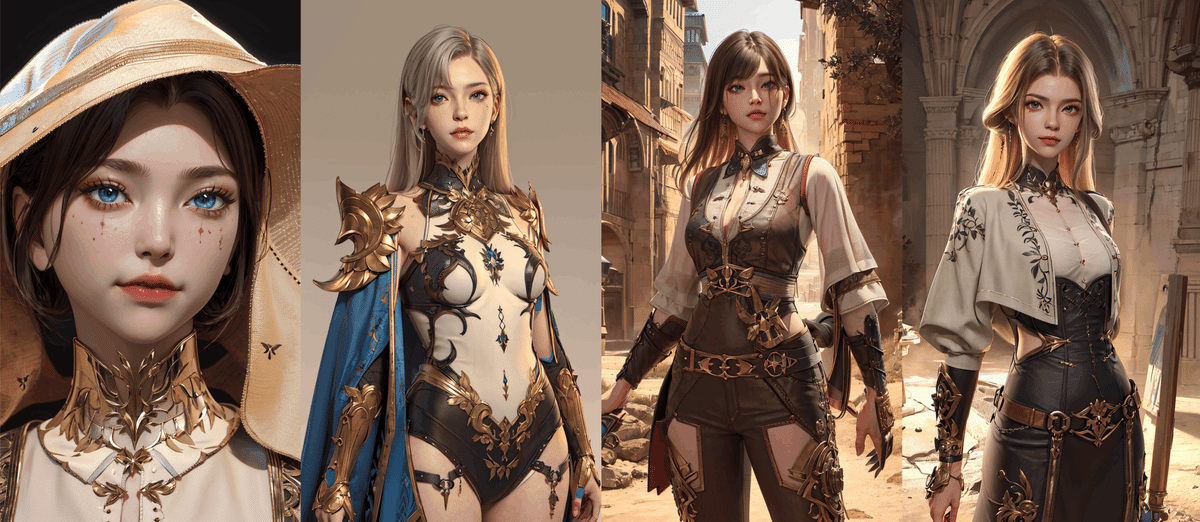
Ultimately, using nai as the base model yielded the best learning of features and the highest versatility.
The reason for conducting this experiment was due to reports of art style becoming more realistic when using LoRA trained with real-life images on anime models. It was speculated that the cause was the significant difference between the art style of the base nai model and the training images. Indeed, the same issue occurred in ver.1 when using nai as the base.
However, despite continuing to use nai, the problem of realisic style in ver.2 seems to be more suppressed compared to ver.1. It might be due to the hijacking of existing tags mentioned earlier, but it's unclear, so further verification is expected.
About transparent regularization images
Until now, transparent PNG regularization images have been used as a sort of good luck charm. However, based on Kohya's explanation, the necessity of these images is questionable. Moreover, their adverse effects include an increased number of training steps and increased VRAM consumption. Therefore, these images were not used in trials from Combination 3 onwards.
While no strict comparisons have been made, no noticeable deterioration in quality has been observed by visual inspection, suggesting that transparent regularization may not be necessary effective. As a result, transparent regularization won't be used in my future projects.
Learning Parameters
The final learning parameters used are shared here.
These are in the toml format, which works by passing them to --config_file and --dataset_config in sd-scripts/train_network.py.
# config_file.toml
[model_arguments]
pretrained_model_name_or_path = "<path_to_nai_sfw>"
v_parameterization = false
[additional_network_arguments]
learning_rate = 5e-4
unet_lr = 5e-4
text_encoder_lr = 5e-5
network_module = "networks.lora"
network_dim = 64
network_alpha = 32
[optimizer_arguments]
optimizer_type = "AdamW8bit"
lr_scheduler = "cosine_with_restarts"
lr_scheduler_num_cycles = 1
lr_warmup_steps = 90 # 0.5% of the total steps
[dataset_arguments]
cache_latents = false
debug_dataset = false
[training_arguments]
output_dir = "<path_to_output>"
output_name = "hipoly_3dcg"
max_train_epochs = 15
save_every_n_epochs = 1
train_batch_size = 4
max_token_length = 225
noise_offset = 0
seed = 42
xformers = true
max_data_loader_n_workers = 8
persistent_data_loader_workers = true
save_precision = "fp16"
mixed_precision = "fp16"
clip_skip = 2
[dreambooth_arguments]
prior_loss_weight = 1.0
[saving_arguments]
save_model_as = "safetensors"
# dataset_config.toml
[general]
enable_bucket = true
min_bucket_reso = 256
max_bucket_reso = 1280
caption_extension = '.txt'
shuffle_caption = true
[[datasets]]
resolution = 896
[[datasets.subsets]]
image_dir = '<path_to_training_image_dir>'
num_repeats = 3
flip_aug = true
Summary
To sum up the conclusions so far:
Increasing the training resolution may enable learning finer details
However, this could also pick up unnecessary details, so choose the appropriate resolution based on the characteristics of the training images and the learning objectives
The training base model should generally be nai to prevent "regression" and maintain versatility
However, using basil or stable-diffusion may be suitable when using real-life images as training images
Organize the tagging as sufficiently as possible
Especially, process background and posing tags that affect the overall composition carefully
A dim value of 32 - 64 seems to be sufficient for an art style LoRA
Transparent regularization does not seem to have a significant effect (at least not enough to noticeably influence the results)
Please note that the insights presented here may include errors due to chance or misunderstanding. Consider this as one example for future verification.
Lastly, as an aside, the future prospects will be discussed.
Using this LoRA, it was possible to recreate the texture and lighting in high-quality 3D images.
Additionally, the expression of hair that feels like strands while becoming lighter towards the ends, the dart lines and panel lines of clothing that make the thickness of the fabric apparent, and the intricately sewn and embroidered lace were all personal preferences that could be reproduced, which was very satisfying.
To achieve such expression, time, money, and computational resources are greatly needed in addition to skills, which can be challenging for someone creating as a hobby, like me.
Moreover, when the details are complete and the overall picture becomes visible in the final stages, there may be a desire to adjust the overall silhouette and composition. However, it is difficult to make adjustments without destroying the already established details, which often leads to giving up reluctantly.
Considering AI as a kind of filter for this issue, it might be possible to fundamentally change the workflow by previewing the expressions obtained through materials and rendering at an early stage of the work, or by delegating the generation of fine details to AI rather than modeling or texturing. This could allow for more focus on overall composition and direction, and enable more courageous and enjoyable creation without being overwhelmed by the workload.
I will continue to research and practice on effectively combining 3D and AI.
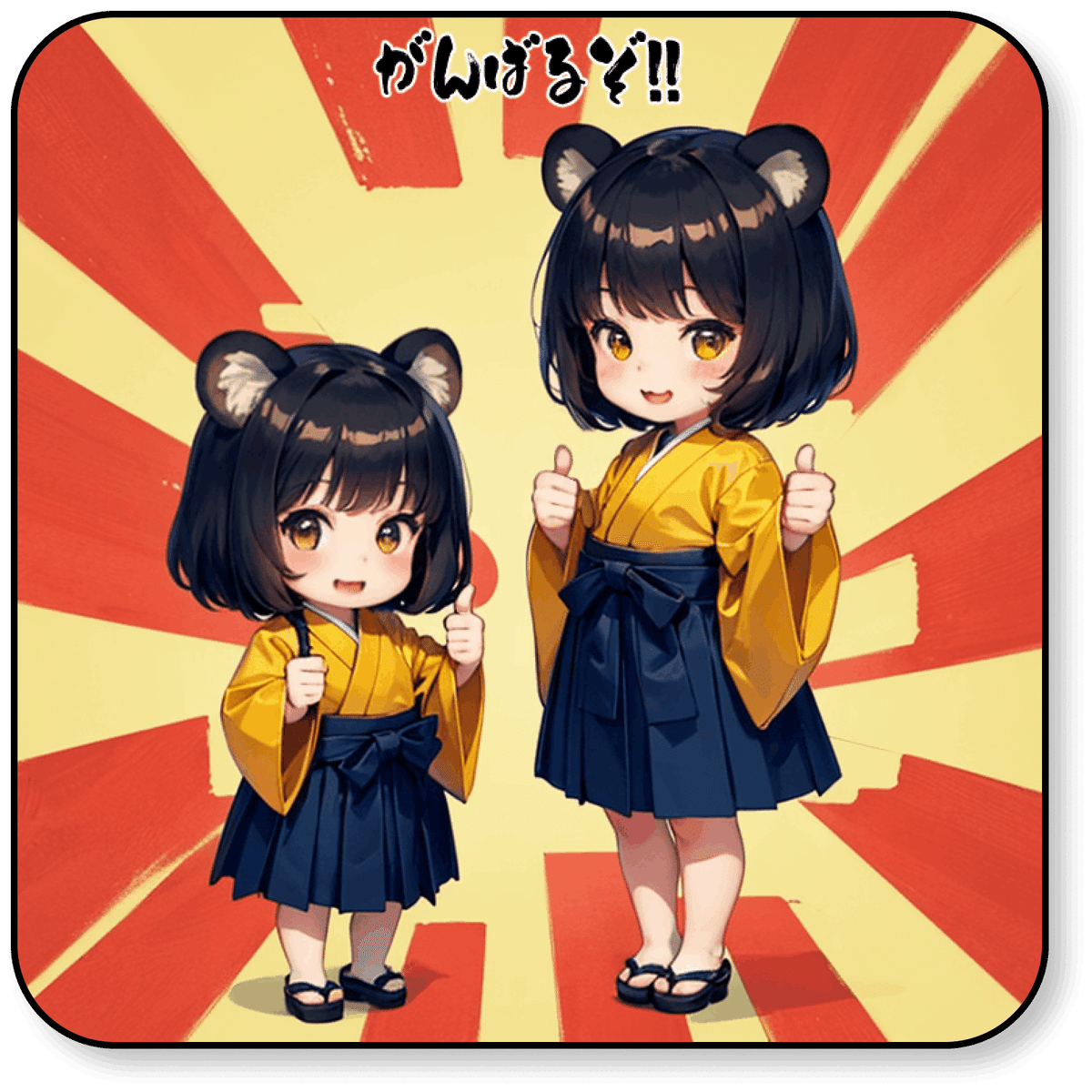
Distribution
High-poly LoRA ver.2
Custom merge models for verification
Stylized 3D LoRA ver.3
Reference
この記事が気に入ったらサポートをしてみませんか?