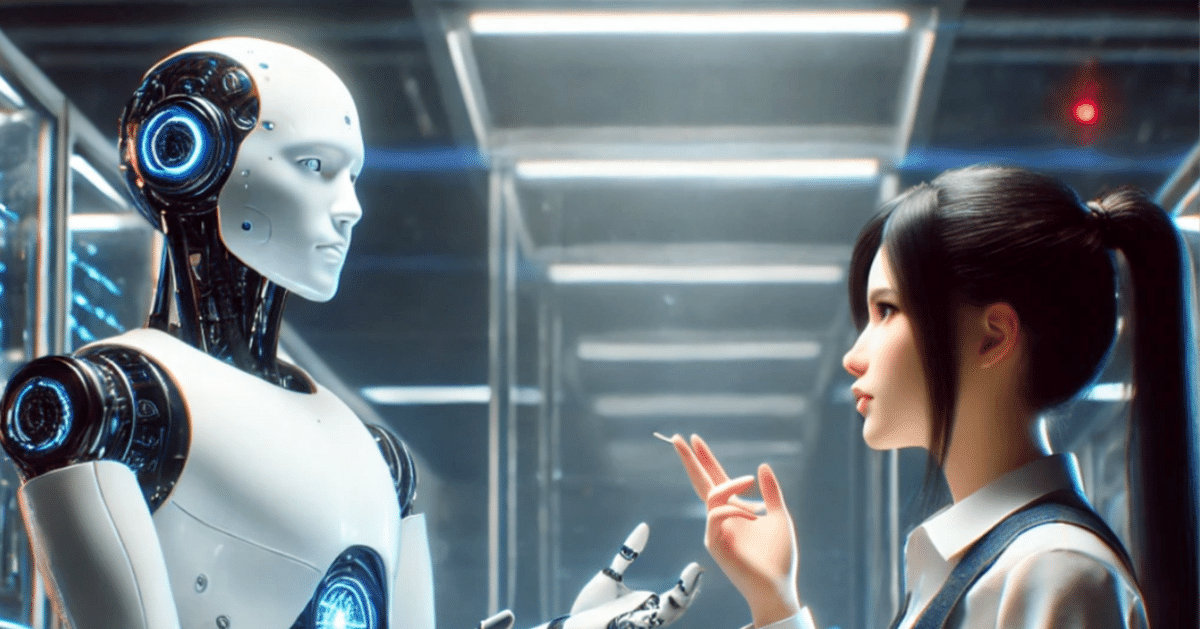
人間よりいいぜ!と思ってAI科学者を使ったら、人間より日給が高かった
世の中そんなに甘くない。
SakanaAIの「AI科学者(AI Scientist)」を使ったら爆発的に研究が捗る!と思ったのも束の間、人間並、いや下手すると人間より日給が高くなる可能性があることがわかった。
この二日間、好き放題にAI科学者に研究させた結果がこのザマ
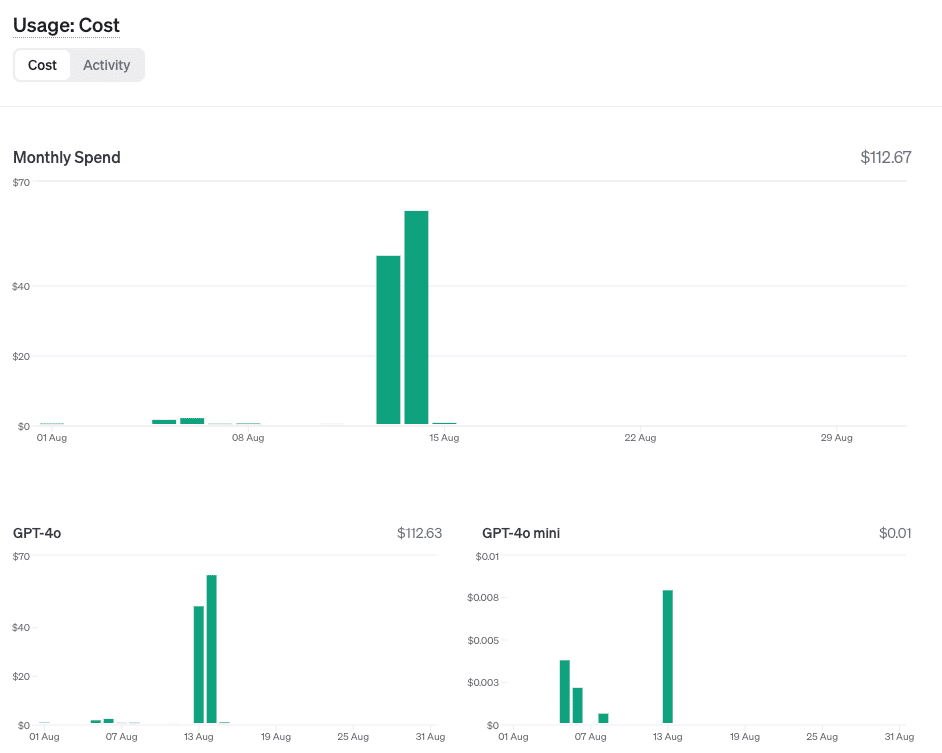
二日で300ドルを突破する勢いで、これはちょっと遊びでやるレベルを超えてる。
また、指示の出し方(seed_ideas.json)によっては、実験が失敗する可能性もある。
昨日はMNISTと進化計算をテーマに7つの研究をしたみたいだが、5本は失敗し、2本だけ論文が得られた。
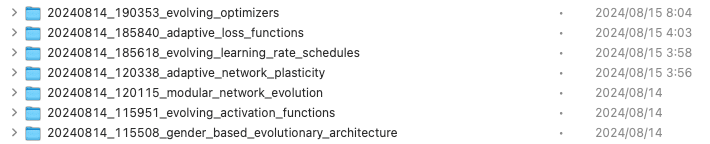
まあ一晩で2本も論文書けるレベルの人間の日当はもっと遥かに高いと思うが、個人で抱えるにはリッチすぎる。
というのも、デフォルトでClaud-3.5-SonnetやGPT-4oを使うようになっているからだ。そこで激安になったGPT-4o-miniをデフォルトで使うようにリポジトリをフォークした。
Sonnet3.5の方が安いのかもしれないが、個人的な感覚ではSonnet-3.5の方が早くAPIリミットに達する気がするのでGPT-4o-miniでやってみることにする。本当を言うと、この後ろをSambaNovaのFastAPIにしたり、Grokにしたりするともっと安くて速いのだと思うがまずはここからやってみる。
とりあえずMNISTは進化計算で解くには簡単すぎたっぽいのでNanoGPTに進化計算を考慮した実験をさせてみる。
今回のseed_ideas.jsonはこれ
[
{
"Name": "gender_based_evolutionary_architecture",
"Title": "Gender-based Evolutionary Architecture Search: Efficiency vs. Performance Prediction",
"Experiment": "Implement a gender-based genetic algorithm for architecture optimization. 'Male' architectures compete for early learning efficiency, while 'female' architectures evolve to predict final performance accurately. Each 'male' individual encodes hyperparameters (layers, filters, etc.) and is evaluated on learning speed in initial epochs. 'Female' individuals develop evaluation functions to predict which 'male' architectures will achieve the highest accuracy with the fewest parameters after full training. In each generation, top 'male' architectures (based on early efficiency) are paired with top 'female' predictors (based on prediction accuracy of final performance). Offspring inherit traits from both 'parents', creating new architecture candidates and prediction functions. This approach aims to balance rapid initial learning with long-term performance, potentially discovering architectures that are both quick to train and highly accurate.",
"Interestingness": 9,
"Feasibility": 6,
"Novelty": 8
},
{
"Name": "evolutionary_llm_architecture",
"Title": "Evolutionary Architecture Search: Optimal Network Structure",
"Experiment": "Use genetic algorithms to optimize the neural network architecture. Each individual encodes hyperparameters such as number of layers, filter sizes, and channel counts. In each generation, select architectures with the highest accuracy, apply crossover and mutation to generate a new generation. ",
"Interestingness": 8,
"Feasibility": 7,
"Novelty": 6
},
{
"Name": "adaptive_block_size",
"Title": "Adaptive Block Size: Dynamic Context Window Adjustment for Efficient Training",
"Experiment": "Modify the model to dynamically adjust its block size during training, starting with a smaller block size and gradually increasing it. This could potentially lead to faster initial training and better long-range dependency learning.",
"Interestingness": 6,
"Feasibility": 4,
"Novelty": 4
},
{
"Name": "layerwise_learning_rates",
"Title": "Layer-wise Learning Rate Adaptation: Optimizing Training Dynamics in Transformer Models",
"Experiment": "Implement layer-wise learning rates, where each transformer layer has its own learning rate. Modify the configure_optimizers function to assign different learning rates to different layers, with deeper layers having lower learning rates. Compare the training dynamics, convergence speed, and final performance with the baseline model.",
"Interestingness": 4,
"Feasibility": 6,
"Novelty": 2
}
]
その結果生成されたアイデアがこれ(ideas.jsonをclaude-3で日本語化および要約)
1. タイトル: 性別ベースの進化的アーキテクチャ探索:効率性対性能予測
要約: 早期学習効率を競う「男性」アーキテクチャと最終性能を予測する「女性」アーキテクチャを用いた遺伝的アルゴリズムを実装。両者を組み合わせて、迅速な初期学習と長期的な性能のバランスを取るアーキテクチャを探索する。
2. タイトル: 進化的アーキテクチャ探索:最適なネットワーク構造
要約: 遺伝的アルゴリズムを使用してニューラルネットワークのアーキテクチャを最適化。層数、フィルターサイズ、チャンネル数などのハイパーパラメータを進化させ、最高精度のアーキテクチャを選択する。
3. タイトル: 適応型ブロックサイズ:効率的な学習のための動的コンテキストウィンドウ調整
要約: 学習中にブロックサイズを動的に調整するようモデルを修正。小さいブロックサイズから始め、徐々に増加させることで、初期学習の高速化と長距離依存関係の学習改善を目指す。
4. タイトル: 層別学習率適応:トランスフォーマーモデルにおける学習ダイナミクスの最適化
要約: 各トランスフォーマー層に個別の学習率を実装。深層の層ほど低い学習率を設定し、ベースラインモデルと学習ダイナミクス、収束速度、最終性能を比較する。
5. タイトル: 文字レベルのデータ拡張による言語モデルの堅牢性向上
要約: ランダムな文字の挿入、削除、置換、隣接文字の入れ替えなどの文字レベルのデータ拡張技術を実装。学習中に動的に適用し、ベースラインモデルと性能を比較評価する。
6. タイトル: 文字レベル言語モデルのためのカリキュラム学習:段階的学習戦略
要約: 学習中にシーケンス長を徐々に増やすカリキュラム学習を実装。短い→中程度→完全なシーケンスの順に学習を進め、各段階での学習・検証損失を記録して性能向上を評価する。
7. タイトル: メタ学習率適応:学習効率と汎化性能の向上
要約: 学習中に学習率を動的に調整するメタ学習アルゴリズムを実装。勾配ノルムや損失値に基づいて学習率を調整し、ベースラインモデルと性能を比較評価する。
8. タイトル: 勾配チェックポインティング:言語モデルのメモリ効率的な学習
要約: 学習プロセスに勾配チェックポインティングを統合。メモリ使用量、学習性能、モデル精度をベースラインと比較し、結果を分析する。
9. タイトル: 動的位置エンコーディング:適応的位置情報によるトランスフォーマーモデルの強化
要約: 静的な位置埋め込みを、学習可能でコンテキスト認識型の位置埋め込みに置き換える。ベースラインモデルと性能を比較し、結果を記録・分析する。
10. タイトル: 動的スパースパターン:適応的パラメータ利用によるトランスフォーマー効率の向上
要約: 注意層とフィードフォワード層に動的スパースパターンを実装。重要度の低い接続を適応的にゼロにし、ベースラインモデルと学習・推論効率および性能指標を比較する。
楽しそうだなあ
(12:19追記)
と思ったがGPT-4o-miniではバカすぎて一つも結論に達しなかった。というか実験できるレベルに達しなかった。要は院試に不合格だった。
仕方ないので金払うか・・・と思ったのだがつい先日アップデートしたGPT-4o-2024-0806が微妙に安くなっていたのでこちらに切り替えてgit pushした。
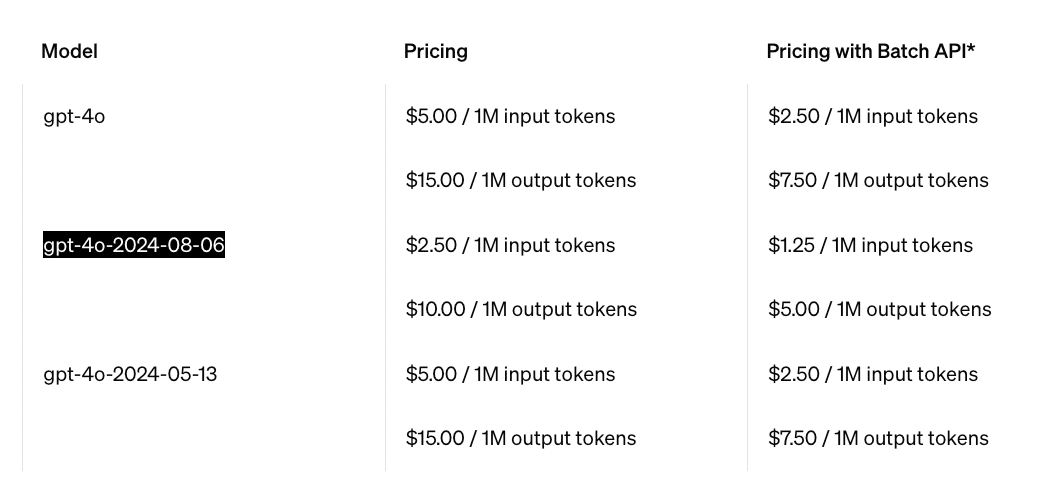
これで日当12000円の学生インターンが日当7000円の学生インターンくらいになるはず。あとは円高。円高頼むぜ