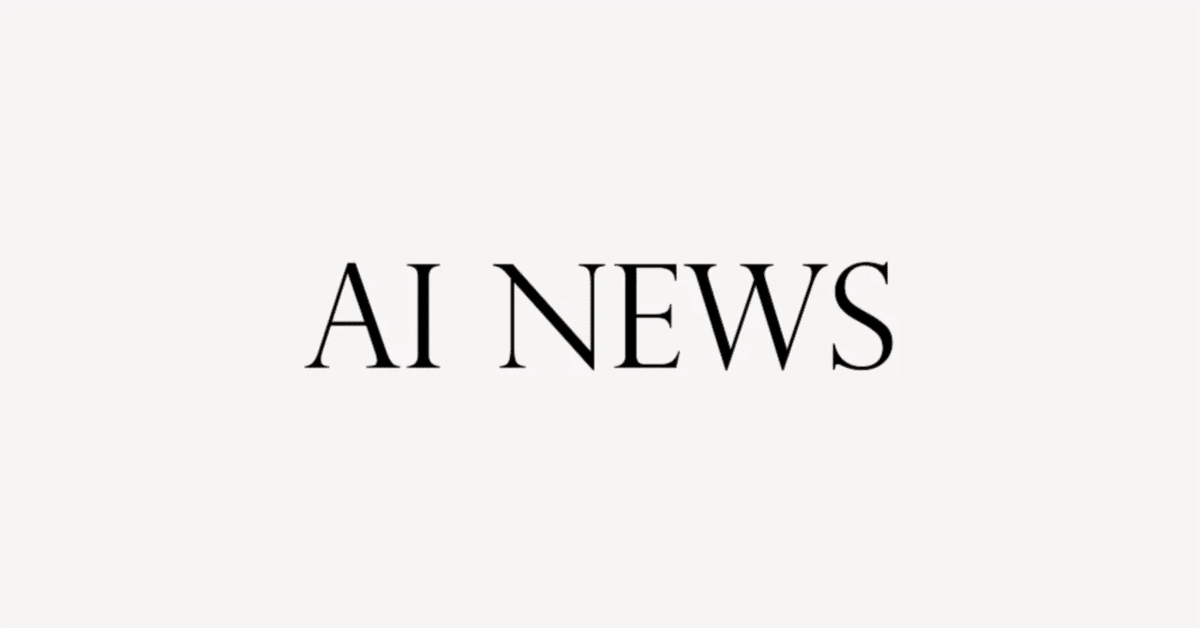
【OpenAI Sora:AI技術によるビデオクリエーションの未来】英語解説を日本語で読む【2024年2月16日|@Two Minute Papers】
この動画では、OpenAIによるテキストからビデオを生成するAI技術「Sora」が紹介されています。この技術は従来のAIによる映像生成技術を遥かに超える品質、時系列の一貫性、そして想像力を持っており、実際の映像と見分けがつかないほどの精度でビデオを生成します。AIは指示に忠実であり、例えば「ブログをするコーギー」や「サーフボードに乗るカワウソ」など、具体的なリクエストに基づいて想像力豊かなビデオを作成できます。さらに、オブジェクトの恒常性と一貫性にも優れており、3D空間内を動いても物体は正しい位置に留まります。この技術は高解像度のビデオ生成が可能で、計算資源が十分にある場合には、未知の世界を含む仮想のビデオを創り出すことができます。
公開日:2024年2月16日
※動画を再生してから読むのがオススメです。
Buckle up, Fellow Scholars, because what you are going to see today is something that might be the craziest thing I've been able to show you in more than 800 videos.
親愛なる研究者の皆さん、今日見ることになるものは、800本以上の動画でお見せできた中でも最も狂ったものかもしれません。
This is the kind of video an AI could create yesterday.
これは、昨日AIが作成できたような種類のビデオです。
And today, it can do this.
そして今日、これができるようになりました。
Holy mother of papers, yes, OpenAI just released their own text-to-video AI, Sora, and it is so far beyond anything else I have ever seen, it is hard to put into words.
まさか、OpenAIが自分たちのテキストからビデオに変換するAI、Soraをリリースしたのですが、これはこれまで見たものの中で最も進化しており、言葉に表すのが難しいほどです。
Dear Fellow Scholars, this is Two Minute Papers with Dr. Károly Zsolnai-Fehér.
親愛なる研究者の皆さん、ドクター・カーロイ・ゾルナイ=フェヘールとのTwo Minute Papersです。
When I first saw these results, I thought this was some April Fool's joke.
最初にこれらの結果を見たとき、これはエイプリルフールの冗談だと思いました。
No, this is not a video coming from a real camera.
いいえ、これは実際のカメラからのビデオではありません。
This is a video that was synthesized pixel by pixel, by a new AI.
これは、新しいAIによってピクセル単位で合成されたビデオです。
So let's give this one a spin.
これを試してみましょう。
These AI videos we will evaluate by three criteria.
これらのAIビデオは、3つの基準で評価します。
One, quality.
1つ目は、品質です。
This is shocking.
これは驚くべきことです。
The quality of these works is out of this world.
これらの作品の品質は非常に高いです。
If we are not actively seeking for errors in the footage, in many cases, we may not even know that they are made by an AI.
映像の中で積極的にエラーを探していない限り、多くの場合、それがAIによって作成されたものだと気づかないかもしれません。
And it gets better, their DALL·E 3 system, that is, an expert at creating images, and I just stop these videos here and there, and the still images are often as good or even better than what DALL·E 3 can make.
さらに、彼らのDALL·E 3システムは、画像を作成するエキスパートであり、私はこれらのビデオを途中で止めて、静止画はDALL·E 3が作成できるものと同等かそれ以上のものが多いです。
This is beating the king in its own game.
これは王様の得意分野で王様を打ち負かすことです。
Unbelievable.
信じられない。
Two, temporal coherence.
2つ目は、時間的な一貫性です。
This means that the AI understands exactly how each image in the video should follow each other.
これは、AIがビデオ内の各画像がどのように続くべきかを正確に理解していることを意味します。
This is what it looks like if you don't have temporal coherence.
これが時間的な一貫性がない場合の見た目です。
A paper from just a few years ago.
数年前の論文。
And now we have this.
そして今、これがあります。
Once again, temporal coherence second to none.
再び、他に類を見ない時間的な一貫性。
Wow.
わぁ。
And three, wait, this may still not be a great technique.
そして3つ目は、待ってください、これはまだ素晴らしいテクニックとは限らないかもしれません。
Now I hear you asking, Károly, why is that?
さて、あなたが尋ねているのが聞こえますが、カーロイ、なぜそうなのですか?
Well, it has to follow our prompt correctly.
まあ、それは私たちの指示に正しく従わなければならないからです。
It has to be true to what we asked for.
私たちが求めたものに忠実でなければなりません。
You see, there are techniques out there that give you really good quality, coherent videos, however, they don't care too much about the prompts that we write.
実際、非常に良質で一貫したビデオを提供する技術がありますが、私たちが書いた指示にあまり気を配っていません。
And what about this technique?
このテクニックについてはどうですか?
Goodness, that is exactly what the prompt is asking for.
おやおや、まさにその通りですね。
I am out of words.
言葉を失います。
But it gets better.
でも、さらに良くなります。
It even has a hint of imagination.
それに、想像力のヒントさえあります。
For instance, we can ask for a corgi, that's also a vlogger, an otter on a surfboard, an Italian pup, you name it, just ask and it will do it.
例えば、コーギーを頼んで、それがブロガーでもいいし、サーフボードに乗ったカワウソやイタリアの子犬など、何でも名前を挙げて頼むと、それをやってくれます。
Imagination in a machine.
機械の中の想像力。
What a time to be alive!
生きている今が何と素晴らしい時なのでしょう!
Hmm, wait, I just noticed that we need to take a look at a fourth thing from now on, and that is object permanence and consistency.
えーと、待ってください。今後、4つ目のことを見ていかなければならないことに気づきました。それは、物体の恒久性と一貫性です。
With previous techniques, when something got occluded and is now visible again, the AI might not remember, and it might look completely different.
以前の技術では、何かが隠れて再び見えるようになったとき、AIはそれを覚えていないかもしれず、見た目が全く異なるかもしれません。
But here, let's see.
でも、ここでは、見てみましょう。
This has a consistent world model, so much so, that even when we move around in 3D space, everything remains where it should be.
これは一貫した世界モデルを持っていて、3D空間で移動しても、すべてが本来の位置に留まっています。
And this can do so much more.
そして、これはさらに多くのことができます。
We can even transform an existing video into a completely new one by just writing one text prompt.
既存のビデオを完全に新しいものに変換することさえ、たった一つのテキストプロンプトを書くだけでできます。
And now, hold on to your paper's fellow scholars, because it can also synthesize virtual worlds, whether that will be something that already exists, like Minecraft, or a completely new game made from scratch, up to you.
そして今、諸君の論文を持っている者たち、しっかりしてください。それは仮想世界さえ合成できるのです。それが既存のものであるか、マインクラフトのようなものであるか、あるいはゼロから作られた完全に新しいゲームであるかは、あなた次第です。
Just one more paper down the line, and it might be that you don't even need to develop your own games, you can maybe just hook up a controller, write a text prompt, and OpenAI Sora will give that game to you immediately.
もう1つの論文があれば、自分でゲームを開発する必要すらなくなるかもしれません。コントローラーを接続し、テキストプロンプトを書くだけで、OpenAI Soraがそのゲームをすぐに提供してくれるかもしれません。
So, how does all this magic work?
では、この魔法のような仕組みはどのように機能するのでしょうか?
Well, one of the key ideas is that the synthesis takes place in a latent space.
まあ、その鍵となるアイデアの1つは、合成が潜在空間で行われるということです。
What is that?
それは何ですか?
It looks something like this.
それはこのようなものです。
This is one of my papers where you can walk around in this 2D latent space, and each point in this space represents a material for a virtual world.
これは私の論文の1つで、この2Dの潜在空間を歩き回ることができ、この空間の各点が仮想世界の素材を表しています。
And here comes the key.
そして、ここが鍵です。
The latent space works well if you can guarantee that when exploring a nearby point, you get similar material models.
潜在空間は、近くの点を探索するときに似たような素材モデルを得られることが保証されている場合にうまく機能します。
The link to the paper is available in the video description.
論文へのリンクは動画の説明にあります。
And this concept also works for creating new fonts, and now, to create new videos too.
そして、このコンセプトは新しいフォントを作成するためにも機能し、今は新しいビデオを作成するためにも使われています。
And they come in full HD resolution.
そして、それらはフルHD解像度で提供されます。
So, is this concept any good so far?
それでは、これまでのところこのコンセプトはどうでしょうか?
Well, let's have a look.
まあ、見てみましょう。
Wait a second, that is not even close to what we've seen.
ちょっと待って、それは今まで見てきたものとはまったく違います。
What happened?
何が起こったのですか?
Well, one word.
まあ、一言で言うと。
Compute happened.
計算が起こったのです。
You see, if you don't have enough computational power, this is what you get.
計算能力が足りないと、こうなります。
If you have 4 times more, you get this.
4倍の計算能力があれば、こうなります。
And if you have 16 times more, you get this.
そして、16倍の計算能力があれば、こうなります。
Oh yes, so the concept comes alive, only with a sufficient amount of compute.
ああ、そうですね、概念は、十分な計算量がある場合にのみ実現します。
The virtual brain, if you will, has to be developed enough to imagine all of these videos in high quality.
仮想脳、と言えば、これらのビデオをすべて高品質で想像するためには、十分に発展させる必要があります。
And my goodness, this is perhaps the biggest jump in quality between two research works that I have ever seen, and this video series has been around for more than 800 episodes now.
そして、まあ、これはおそらく私が今まで見た研究作業の中で最も品質が向上したジャンプかもしれません。このビデオシリーズはすでに800エピソード以上続いています。
And now, it's time.
そして今、その時が来ました。
Time for what, you ask?
何のための時間か、と尋ねるでしょうか?
Of course, it is time to invoke the first law of papers.
もちろん、論文の第一法則を発動する時です。
The first law of papers says that research is a process.
論文の第一法則とは、研究はプロセスであるということです。
Do not look at where we are, look at where we will be, two more papers down the line.
私たちがどこにいるかではなく、さらに2つの論文を進めたときにどこにいるかを見てください。
And here is the one more paper down the line.
そして、これがさらに1つの論文を進めたときの結果です。
Now, exercise.
今、運動をしてください。
Leave a comment about what you think we will be able to do, just two more papers down the line.
次に2つの論文が出ると、私たちが何ができるようになると思うかについてコメントを残してください。
I'd love to know what you Fellow Scholars think, especially now, because once again, we share one of those sweet moments where we witness history in the making.
フェロー・スカラーの皆さんがどう思っているか知りたいですが、特に今は、再び歴史が作られる瞬間を共有しているからです。
In his excellent video, which I highly recommend, MKBHD says that since this is trained on videos made by humans, it likely cannot go beyond what it had seen from humans.
彼の優れたビデオでは、私が強くお勧めするように、MKBHDは、これが人間によって作成されたビデオに訓練されているため、それが人間から見たものを超えることはできない可能性が高いと述べています。
I would like to note that in some cases, we see AI papers that have proper zero-shot performance.
私は、いくつかの場合には、適切なゼロショットのパフォーマンスを持つAI論文を見ることができることを指摘したいと思います。
What is that?
それは何ですか?
This means that leaning on all this knowledge like a human, it can try to create new things it hasn't seen before.
これは、人間のようにすべてのこの知識に頼って、それが以前に見たことのない新しいものを作ろうとすることができることを意味します。
For instance, you could ask for a new kind of vehicle for T-Rexes.
例えば、T-Rex用の新しい種類の乗り物を要求することができます。
And it could infer that T-Rexes have these tiny little hands, so it would have to have a wheel that is suitable for their little hands.
そして、それはT-Rexがこれらの小さな手を持っていると推論するかもしれませんので、それは彼らの小さな手に適したホイールを持っている必要があります。
We will be able to test that, and so much more, as soon as it is out there.
それが出てくるとすぐに、それをテストすることができるようになります。
And we will soon be back with a video on a different AI video system that is not as good as this, but it is more controllable, and it is something that you will be able to try out for free right away.
そして、私たちはすぐに、このよりコントロール可能で、無料で試すことができるこのより良くない別のAIビデオシステムに関するビデオで戻ってきます。
We will also have a more in-depth video about the capabilities of this new technique soon too.
また、この新しい技術の能力についてより詳細なビデオも近日中に公開します。
So make sure to subscribe and hit the bell icon to not miss out.
お見逃しなく、購読してベルアイコンをクリックしてください。
Experiment tracking, model evaluation, and production monitoring for your deep learning projects and LLM apps.
あなたの深層学習プロジェクトと大規模言語モデルアプリのための実験追跡、モデル評価、および製品監視。
This is what Weights & Biases does, and it is the best!
これがWeights&Biasesが行うことであり、それが最高のものです!
Everyone is using it!
皆がそれを使っています!
Try it out now at wandb.me/papers or click the link in the description below!
今すぐwandb.me/papersで試してみてください。または、下記の説明文中のリンクをクリックしてください!
この記事が気に入ったらサポートをしてみませんか?