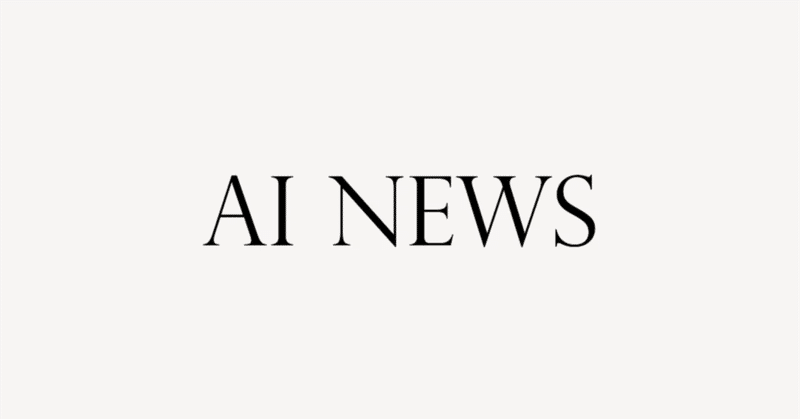
【Gemini 1.5:Googleの驚異の進化】英語解説を日本語で読む【2024年2月16日|@TheAIGRID】
Googleは、Geminiモデルファミリーの最新バージョンとして、予想外にGemini 1.5を発表しました。このモデルは、最大3時間のビデオや22時間のオーディオ、700万語(1000万トークン)のテキストを処理でき、約99~100%の驚異的な精度を誇ります。Gemini 1.5は、テキスト、ビジョン、オーディオの処理能力が全面的に向上しており、Gemini Pro 1.0やUltraモデルと比較しても、特にテキストとビジョンで優れた性能を示します。Gemini 1.5 Proは、長文理解、マルチモーダルプロンプト、コーディングタスクなど、複数の実験的機能を備え、Apollo 11の432ページにわたるトランスクリプトやThree.jsのコード例、バスター・キートンの映画など、さまざまなデモを通じてその能力を示しました。また、ビデオやテキストの大量のデータから特定の情報を正確に抽出する「ヘイスタック」能力を持ち、これまでにないレベルのコンテキストウィンドウと精度でAIの可能性を拡張しています。
公開日:2024年2月16日
※動画を再生してから読むのがオススメです。
So Google actually did just surprise everyone by releasing Gemini 1.5.
Googleは実際にGemini 1.5をリリースすることで、みんなを驚かせました。
And this is their latest iteration of their family of Gemini models.
これは、Geminiモデルファミリーの最新のイテレーションです。
And this is a rather surprising model in the fact that it is able to do something incredible.
そして、これは信じられないことができるモデルであるという点でかなり驚くべきものです。
Gemini 1.5 is the behemoth that is able to take up to three hours of video in a single context length.
Gemini 1.5は、1つのコンテキストの長さで最大3時間のビデオを撮影できる巨大なものです。
It's also able to take 22 hours of audio at once.
また、一度に22時間のオーディオを録音することもできます。
It's also able to take up to seven million words or 10 million tokens with remarkable accuracy as well, because lots of the time when we see new models appear, many of the times what happens is is that their accuracy rates are very, very underwhelming.
また、新しいモデルが登場するたびに、その精度が非常に低いことが多い中で、最大700万語または1000万トークンを驚くほどの精度で扱うこともできます。
And Gemini is just outstanding because on these capabilities, their accuracy rate is around 99 to 100 percent.
そして、Geminiはこれらの能力において、その精度率が約99〜100%であるため、卓越しています。
So that is absolutely incredible.
それは本当に信じられないことです。
This multimodal model is going to change everything.
このマルチモーダルモデルはすべてを変えるでしょう。
And let's take a look at some of the things you do need to know, because once you see a few videos, you're going to be truly surprised by how good this AI really is.
そして、知っておく必要があるいくつかのことを見てみましょう。数本のビデオを見ると、このAIが本当にどれほど優れているかに本当に驚くでしょう。
So where is Gemini 1.5?
Gemini 1.5はどこにありますか?
Before we dive into some of the examples of how good this AI system is, where does it fit?
このAIシステムがどれほど優れているかのいくつかの例について掘り下げる前に、それはどこに適合するのでしょうか?
So on the left hand side, you can see Gemini Ultra, our most capable and largest model for highly complex tasks.
左側には、高度な複雑なタスク向けの最も能力が高く最大のモデルであるGemini Ultraがあります。
And then in the middle, you can see Gemini Pro and you've got two iterations.
そして、中央には、Gemini Proがあり、2つのイテレーションがあります。
Gemini 1.0 and Gemini 1.5.
Gemini 1.0とGemini 1.5。
And Gemini 1.5 is the model that was released today, which is essentially for larger, more tedious tasks that require a longer context length.
Gemini 1.5は、本日リリースされたモデルであり、より大きな、より煩雑なタスクに適しており、より長いコンテキスト長が必要です。
So how much better is Gemini 1.5?
Gemini 1.5はどれだけ優れているのですか?
So you can see that in text, in vision and in audio, Gemini 1.5 is better across the board.
テキスト、ビジョン、オーディオのいずれにおいても、Gemini 1.5は全般的に優れていることがわかります。
However, compared to the Ultra benchmarks, you can see that only on vision and audio on the right hand side, there are some areas where Ultra is slightly better.
ただし、Ultraのベンチマークと比較すると、右側のビジョンとオーディオにおいて、Ultraの方がわずかに優れている部分があることがわかります。
So overall, this model is substantially better than Gemini Pro 1.0, which is currently available.
全体的に、このモデルは現在利用可能なGemini Pro 1.0よりもかなり優れています。
And in terms of Gemini Ultra, largely, it's better than text and in vision.
Gemini Ultraに関しては、大部分ではテキストとビジョンにおいて優れています。
So across the board, this is a model that is most certainly more capable.
全般的に、このモデルは間違いなくより能力があるものです。
Now I'm going to be showing you guys one of these examples of Gemini Pro 1.5 reasoning across a 432 page transcript.
Gemini Pro 1.5の推論の例を、432ページのトランスクリプトでお見せします。
This is a demo of long context understanding, an experimental feature in our newest model, Gemini 1.5 Pro.
これは、最新モデルGemini 1.5 Proの実験的な機能である長いコンテキスト理解のデモです。
We'll walk through a screen recording of example prompts using a 402 page PDF of the Apollo 11 transcript, which comes out to almost 330,000 tokens.
私たちは、ほぼ33万のトークンになるアポロ11号の記録の402ページのPDFを使用して、例のプロンプトのスクリーンレコーディングを行います。
We started by uploading the Apollo PDF into Google AI Studio and asked, find three comedic moments, list quotes from this transcript and emoji.
まず、アポロのPDFをGoogle AI Studioにアップロードし、この記録から3つのコミカルな瞬間を見つけ、引用と絵文字のリストを作成するように依頼しました。
This screen capture is sped up.
このスクリーンキャプチャはスピードアップされています。
This timer shows exactly how long it took to process each prompt.
このタイマーは、各プロンプトの処理にかかった正確な時間を示しています。
And keep in mind that processing times will vary.
そして、処理時間は異なることを覚えておいてください。
The model responded with three quotes, like this one from Michael Collins.
モデルは、マイケル・コリンズからのこのような引用を含む3つの引用で応答しました。
I'll bet you a cup of coffee on it.
それについてはコーヒーを賭けてもいいですよ。
If we go back to the transcript, we can see the model found this exact quote and extracted the comedic moment accurately.
もしトランスクリプトに戻れば、モデルがこの正確な引用を見つけ、コミカルな瞬間を正確に抽出したことがわかります。
Then we tested a multimodal prompt.
その後、マルチモーダルなプロンプトをテストしました。
We gave it this drawing of a scene we were thinking of and asked, what moment is this?
私たちはこのシーンの描画を与え、これはどんな瞬間か尋ねました。
The model correctly identified it as Neil's first steps on the moon.
モデルはそれを正しくニールの月面での最初の一歩として特定しました。
Notice how we didn't explain what was happening in the drawing.
描画の中で何が起こっているかを説明しなかったことに注目してください。
Simple drawings like this are a good way to test if the model can find something based on just a few abstract details.
このようなシンプルな描画は、モデルがわずかな抽象的な詳細だけで何かを見つけられるかどうかをテストする良い方法です。
And for the last prompt, we asked the model to cite the time code of this moment in the transcript.
そして最後のプロンプトでは、モデルにこの瞬間のトランスクリプト内のタイムコードを引用するように求めました。
Like all generative models, responses like this won't always be perfect.
すべての生成モデルのように、このような回答は常に完璧ではありません。
They can sometimes be a digit or two off.
時々、1つまたは2つの数字がずれることがあります。
But let's look at the model's response here.
しかし、ここでモデルの応答を見てみましょう。
And when we find this moment in the transcript, we can see that this time code is correct.
そして、トランスクリプトでこの瞬間を見つけると、このタイムコードが正しいことがわかります。
These are just a few examples of what's possible with a context window of up to one million multimodal tokens in Gemini 1.5 Pro.
これらはGemini 1.5 Proの最大100万のマルチモーダルトークンのコンテキストウィンドウで可能なことのいくつかの例にすぎません。
That demo right there was rather impressive.
そのデモはかなり印象的でした。
And there are a lot more examples in the paper.
そして論文にはさらに多くの例があります。
But let's take a look at another example of Gemini's massive capabilities on doing this with coding tasks.
これをコーディングタスクで行うためのGeminiの大規模な能力の別の例を見てみましょう。
This is a demo of long context understanding, an experimental feature in our newest model, Gemini 1.5 Pro.
これは、当社の最新モデルであるGemini 1.5 Proの実験的な機能である長い文脈理解のデモです。
We'll walk through some example prompts using the Three.js example code, which comes out to over 800,000 tokens.
800,000以上のトークンになるThree.jsの例コードを使用したいくつかの例のプロンプトを見ていきます。
We extracted the code for all of the Three.js examples and put it together into this text file, which we brought into Google AI Studio over here.
私たちは、すべてのThree.jsの例のコードを抽出し、それらをこのテキストファイルにまとめ、ここにGoogle AI Studioに持ち込みました。
We asked the model to find three examples for learning about character animation.
モデルに、キャラクターアニメーションについて学ぶための3つの例を見つけるように求めました。
The model looked across hundreds of examples and picked out these three, one about blending skeletal animations, one about poses, and one about morph targets for facial animations.
モデルは何百もの例を調べ、スケルタルアニメーションのブレンディングに関する1つ、ポーズに関する1つ、そして顔のアニメーションのモーフターゲットに関する1つを選び出しました。
All good choices based on our prompt.
私たちのプロンプトに基づいてすべて良い選択です。
In this test, the model took around 60 seconds to respond to each of these prompts.
このテストでは、モデルはこれらのプロンプトに対して約60秒かかりました。
But keep in mind that latency times might be higher or lower, as this is an experimental feature we're optimizing.
ただし、これは最適化中の実験的な機能であるため、遅延時間は高くなるか低くなる可能性があることを念頭に置いてください。
Next, we asked, what controls the animations on the littlest Tokyo demo?
次に、最小の東京デモのアニメーションを制御するのは何かを尋ねました。
As you can see here, the model was able to find that demo, and it explained that the animations are embedded within the GLTF model.
ここで見るように、モデルはそのデモを見つけ、アニメーションがGLTFモデル内に埋め込まれていると説明しました。
Next, we wanted to see if it could customize this code for us, so we asked, show me some code to add a slider to control the speed of the animation.
次に、このコードをカスタマイズできるかどうかを見たかったので、スライダーを追加してアニメーションの速度を制御するコードを示してください、と尋ねました。
Use that kind of GUI the other demos have.
他のデモにあるようなGUIを使用してください。
This is what it looked like before on the original Three.js site.
これが元のThree.jsサイトでの見た目です。
And here's the modified version.
そしてこちらが修正されたバージョンです。
It's the same scene, but it added this little slider to speed up, slow down, or even stop the animation on the fly.
それは同じシーンですが、アニメーションをスピードアップ、スローにしたり、途中で停止させるためのスライダーが追加されました。
It used this GUI library the other demos have, set a parameter called animation speed, and wired it up to the mixer in the scene.
他のデモにあるGUIライブラリを使用し、アニメーションスピードというパラメータを設定し、シーン内のミキサーに接続しました。
Like all generative models, responses aren't always perfect.
すべての生成モデルと同様に、応答は常に完璧ではありません。
There's actually not an init function in this demo like there is in most of the others.
実際、このデモには、ほとんどの他のデモにあるようなinit関数はありません。
However, the code it gave us did exactly what we wanted.
ただし、与えられたコードはまさに私たちが望んでいたことをしました。
Next, we tried a multimodal input by giving it a screenshot of one of the demos.
次に、デモのスクリーンショットを与えることで、マルチモーダル入力を試してみました。
We didn't tell it anything about this screenshot and just asked where we could find the code for this demo, seen over here.
このスクリーンショットについて何も伝えず、ただこのデモのコードをどこで見つけられるか尋ねました。
As you can see, the model was able to look through the hundreds of demos and find the one that matched the image.
見ての通り、モデルは数百のデモを見て、画像に一致するものを見つけることができました。
Next, we asked the model to make a change to the scene, asking, how can I modify the code to make the terrain flatter?
次に、モデルにシーンを変更するように尋ね、どのようにしてコードを変更して地形を平らにできるか尋ねました。
The model was able to zero in on one particular function called generateHeight and showed us the exact line to tweak.
モデルはgenerateHeightという特定の関数に絞り込み、調整すべき正確な行を示しました。
Below the code, it clearly explained how the change works.
コードの下に、変更がどのように機能するかが明確に説明されています。
Over here in the updated version, you can see that the terrain is indeed flatter, just like we asked.
更新されたバージョンでは、地形が実際に私たちが求めたように平らになっているのが見て取れます。
We tried one more code modification task using this 3D text demo over here.
こちらの3Dテキストデモを使用して、もう1つのコード修正タスクを試しました。
We asked, I'm looking at the text geometry demo and I want to make a few tweaks. How can I change the text to say goldfish and make the mesh materials look really shiny and metallic?
お尋ねしたのですが、テキストジオメトリーデモを見ていて、少し調整したいと思っています。テキストを金魚というように変更し、メッシュ素材を本当に光沢のある金属質に見せるにはどうすればいいですか?
You can see the model identified the correct demo and showed the precise lines in it that need to be tweaked.
モデルが正しいデモを特定し、調整する必要のある正確なラインを示してくれました。
Further down, it explained these material properties, metalness and roughness, and how to change them to get a shiny effect.
さらに下には、これらの素材の特性、金属度と粗さについて説明され、それらを変更して光沢のある効果を得る方法が説明されています。
You can see that it definitely pulled off the task and the text looks a lot shinier now.
確かに、そのタスクを遂行し、テキストがかなり光沢のある見た目になっているのがわかります。
These are just a couple examples of what's possible with a context window of up to one million multimodal tokens in Gemini 1.5 Pro.
これらは、Gemini 1.5 Proの最大100万のマルチモーダルトークンを持つコンテキストウィンドウで可能な例のほんの一部です。
Now, you just saw Google's Gemini 1.5 Pro problem solving across 100,000 lines of code.
今、GoogleのGemini 1.5 Proが10万行のコードを横断的に解決するのを見ました。
And my oh my, this is something that is truly impressive.
そして、まあまあ、これは本当に印象的なものです。
There is no other AI system out there that can do this with the accuracy level of Google's Gemini.
GoogleのGeminiの精度レベルでこれを行う他のAIシステムは存在しません。
But now let's take a look at some of the multimodal prompting which is going to be used by a lot of standard users.
しかし、今度は多くの一般ユーザーが使用するマルチモーダルプロンプティングのいくつかを見てみましょう。
This is a demo of long context understanding, an experimental feature in our newest model, Gemini 1.5 Pro.
これは、当社の最新モデルGemini 1.5 Proの実験的な機能である長いコンテキスト理解のデモです。
We'll walk through a screen recording of example prompts using a 44 minute Buster Keaton film, which comes out to over 600,000 tokens.
私たちは、44分間のバスター・キートンの映画を使用した例のプロンプトの画面録画を見ていきます。これは60万以上のトークンに相当します。
In Google AI Studio, we uploaded the video and asked, find the moment when a piece of paper is removed from the person's pocket and tell me some key information on it with the time code.
Google AI Studioでは、ビデオをアップロードし、人物のポケットから紙が取り出される瞬間を見つけ、その時刻コードとともにその重要な情報を教えてくださいと尋ねました。
This screen capture is sped up and this timer shows exactly how long it took to process each prompt.
この画面キャプチャはスピードアップされており、各プロンプトの処理にかかった時間を正確に示しています。
And keep in mind that processing times will vary.
処理時間は異なることを念頭に置いておいてください。
The model gave us this response, explaining that the piece of paper is a pawn ticket from Goldman and company pawnbrokers with the date and cost.
モデルは、ゴールドマン&カンパニー質屋の質屋券であり、日付と費用が記載されていることを説明するこの回答を提供しました。
And it gave us this time code 1201.
そして、それは私たちにこのタイムコード1201を与えました。
When we pulled up that time code, we found it was correct.
そのタイムコードを調べると、正しいことがわかりました。
The model had found the exact moment the piece of paper is removed from the person's pocket and it extracted text accurately.
モデルは、紙片が人のポケットから取り出される正確な瞬間を見つけ、テキストを正確に抽出しました。
Next, we gave it this drawing of a scene we were thinking of and asked, what is the time code when this happens?
次に、私たちはこのシーンの描画を与え、これが起こるときのタイムコードは何か尋ねました。
This is an example of a multimodal prompt where we combine text and image in our input.
これは、テキストと画像を組み合わせたマルチモーダルプロンプトの例です。
The model returned this time code, 1534.
モデルはこのタイムコード1534を返しました。
We pulled that up and found that it was the correct scene.
それを調べると、正しいシーンであることがわかりました。
Like all generative models, responses vary and won't always be perfect.
すべての生成モデルと同様に、応答は異なり、常に完璧であるとは限りません。
But notice how we didn't have to explain what was happening in the drawing.
しかし、この描画で何が起こっているか説明する必要がなかったことに注意してください。
Simple drawings like this are a good way to test if the model can find something based on just a few abstract details like it did here.
このようなシンプルな描画は、モデルがここで行ったように、わずかな抽象的な詳細に基づいて何かを見つけることができるかどうかをテストする良い方法です。
These are just a couple examples of what's possible with a context window of up to 1 million multimodal tokens in Gemini 1.5 Pro.
これらは、Gemini 1.5 Proの最大100万のマルチモーダルトークンのコンテキストウィンドウで可能なことのいくつかの例にすぎません。
That right there goes to show us how crazy this is.
それはこの状況がどれだけクレイジーなものかを示しています。
I think the only caveat to this is that it does take a little bit of time for it to go ahead and get the footage.
これには唯一の注意点があると思いますが、それは映像を取得するのに少し時間がかかるということです。
But looking through a 44 minute video is absolutely incredible.
しかし、44分のビデオを見ることは本当に信じられないことです。
And doing the reasoning across that is not to be understated because think about how long it would take a human to watch through an entire movie and find something from one frame.
そして、その理論を通して行うことは過小評価されるべきではありません。なぜなら、人間が映画全体を見て1フレームから何かを見つけるのにどれだけ時間がかかるか考えてみてください。
And whilst these demos are impressive, what's even more impressive is the paper that they attached to this, which I read, that shows a whole host of other incredible capabilities.
これらのデモは印象的ですが、さらに印象的なのは、私が読んだこの論文に添付されている内容で、他の信じられないほどの能力を示しています。
So let's take a look at some of the stunning examples from the paper, which is going to show you all exactly how accurate this AI system really is and why Google are really leading the entire AI industry with Gemini 1.5 Pro.
では、論文からいくつかの驚くべき例を見てみましょう。これにより、このAIシステムが実際にどれだけ正確であり、GoogleがGemini 1.5 ProでAI業界全体をリードしている理由が明らかになります。
So there was this example and it stated, given a reference grammar book and bilingual word list, a dictionary, Gemini 1.5 is able to translate from English to Kalamang with similar quality to a human who learned from the same materials.
この例があり、参照文法書とバイリンガル単語リスト、辞書が与えられた場合、Gemini 1.5は、同じ教材から学んだ人間と同様の品質で英語からカラマン語に翻訳できると述べています。
This is incredibly substantial because it means that not only is it able to get the entirety of this context length and a dictionary, it's able to reason and do translation based on new data, just like a human would.
これは非常に重要です。なぜなら、これは文脈の全体と辞書を取得できるだけでなく、新しいデータに基づいて推論し、翻訳することができるということを意味しています。
There was also this example right here that was another stunning example from the paper.
また、ここにも、その論文からのもう1つの見事な例がありました。
And essentially it states that with the entire text of this really, really long novel, it's able to understand exactly what's happening just through a very simple drawing.
本当に長い小説の全文が与えられた場合、非常にシンプルな図面だけで何が起こっているかを正確に理解できると述べています。
And I'm no artist, but I'm sure you can all appreciate the fact that this drawing here isn't a very, very artistic one and it's really, really simple.
私は芸術家ではありませんが、この図面が非常に芸術的ではないこと、非常にシンプルであることを皆さんが認めてくれると思います。
So the genius here is of this system to be able to understand the nuance of what's happening in the image, then extrapolate that data out and of course, reason to figure out exactly where that is.
このシステムの天才的な点は、画像で何が起こっているかを理解し、そのデータを推測し、もちろん、それがどこにあるかを正確に理解することができるということです。
That is something that is unheard of in our current AI systems.
これは現在のAIシステムでは考えられないことです。
And that's why I stated this is truly game-changing stuff.
これが私が述べた理由で、これは本当にゲームを変えるものです。
There was another example in the paper and I'm pretty sure you've already seen this one based in the video, but it just goes to show how crazy this is.
論文には別の例もあり、おそらくすでにこのビデオで見たことがあると思いますが、これはどれだけクレイジーなものかを示しています。
Now in the paper, some of the stuff I was looking at was really cool because there was this thing called video haystack, okay?
今、論文で見ていた中には、ビデオヘイスタックというものがあって、本当にクールなものがありました。
And I'm gonna break this down for you guys because it's truly fascinating on how accurate this really is and how they tested it goes to show how accurate this is.
これを皆さんに分かりやすく説明しますが、これがどれだけ正確であり、それをテストした方法がどれだけ正確であるかを示しています。
Now on the image, you can see Gemini 1.5 Pro compared to GPT-4 with Vision.
画像では、Gemini 1.5 ProがGPT-4 with Visionと比較されています。
And unfortunately, GPT-4 with Vision can only take in three minutes in their API, whereas Gemini 1.5 can do one minute of content all up to the way of three hours.
残念ながら、GPT-4 with VisionはAPIで3分間のコンテンツしか処理できませんが、Gemini 1.5は1分間のコンテンツから最大3時間まで対応できます。
So essentially, they set up a game.
基本的に、彼らはゲームを設定しました。
The computer, which is Gemini 1.5, has to find a secret message and the secret word is needle.
コンピューターであるGemini 1.5は、秘密のメッセージを見つけなければならず、秘密の単語はneedleです。
But this message was sneakily hidden in one tiny part of a very long movie.
しかし、このメッセージは非常に長い映画の一部にひそかに隠されていました。
And this movie isn't just any movie, it was a three long hour movie made by sticking two copies of a documentary about the game of Go together.
そして、この映画はただの映画ではなく、囲碁のゲームについてのドキュメンタリーの2つのコピーをくっつけて作られた3時間の長い映画でした。
And this makes the video really long with lots of places that could have hidden the message.
そして、これにより、メッセージが隠されている可能性のある場所がたくさんある非常に長いビデオになりました。
Now in this demo, what they did was they put the secret message only in one single frame of the video.
今回のデモでは、彼らは秘密のメッセージをビデオの1つのフレームにだけ入れました。
That's just one picture out of a thousands and thousands that make up the entire movie.
それは映画全体を構成する何千もの画像のうちの1枚に過ぎません。
And of course, there's a picture every single second.
そしてもちろん、1秒ごとに1枚の写真があります。
Now Gemini 1.5 Pro's job was to watch this entire super long movie and find that one frame with the secret message.
Gemini 1.5 Proの仕事は、この非常に長い映画を見て、その秘密のメッセージが入った1つのフレームを見つけることでした。
And all they did was they asked Gemini 1.5 what was this secret word, which is essentially like finding a needle in a haystack and can you guess what?
そして彼らがしたことは、Gemini 1.5にこの秘密の単語が何か尋ねるだけでした。これは本質的には草の中の針を見つけるようなもので、あなたは推測できますか?
Gemini 1.5 was able to do it 100% of the time.
Gemini 1.5は100%の確率でそれを行うことができました。
So that is why the video capabilities, the video haystack capabilities are absolutely incredible.
そのため、ビデオの能力、ビデオの草の中の針の能力は本当に信じられないほどです。
In addition, they did the same kind of game with the Gemini 1.5 Pro system and they did it with 22 hours of footage.
さらに、彼らはGemini 1.5 Proシステムで同じ種類のゲームを行い、22時間の映像でそれを行うことができました。
And you can see here that it was able to do it up to 100%. And they compared it to Whisper and GPT-4 Turbo with 12 minutes all the way up to 11 hours.
そしてここで、それが100%までできたことがわかります。そして、WhisperとGPT-4 Turboと12分から11時間まで比較しました。
And you can see the boxes in red.
そして、赤い箱が見えます。
They're essentially areas where it completely failed.
それらは完全に失敗した領域です。
In addition, they also did this with text haystack.
さらに、彼らはテキストのヘイスタックでも同じことをしました。
And this is where things start to get crazy because this was something that people didn't really think was possible.
そしてここから事態は狂っていく始まりです、なぜならこれは人々が本当に可能だとは思っていなかったことだったからです。
There were certain research papers that were stating that using Mambo was essentially going to be possible with this kind of output that we really wanted.
私たちが本当に望んでいたこの種の出力でMamboを使用することが本質的に可能になると述べている特定の研究論文がありました。
If we really wanted to be able to get the retrieval that we wanted, we're gonna have to use different architectures.
私たちが本当に望んでいる検索結果を得たいのであれば、異なるアーキテクチャを使用しなければならないでしょう。
But it seems like Google managed to figure out how to do that.
しかし、Googleはそれをどうやって行うかを見つけたようです。
And you can see right here that up to 10 million tokens, they were able to get the accuracy up to around, I think it was 99%, a ridiculous level of accuracy.
そしてここで見ることができるように、最大で1000万トークンまで、彼らは精度を約99%まで上げることができました、信じられないほどの精度です。
And that is something that is unheard of.
そしてそれは聞いたことのないものです。
A 1 million context length window is incredible.
100万のコンテキスト長のウィンドウは信じられないことです。
And of course, compared to GPT-4 Turbo, it's only a 128,000 context length.
もちろん、GPT-4 Turboと比較すると、コンテキスト長は128,000にすぎません。
So this is a truly game-changing thing because imagine having 1 million tokens.
これは本当にゲームを変えるものです、100万のトークンを持っていることを想像してみてください。
and then getting an AI system to be able to reason about the entirety of that or find certain things and then reason on that.
そしてAIシステムをその全体について論理的に考えさせたり、特定のことを見つけてそれについて論理的に考えさせたりすることができるようにする。
That is gonna be a hugely different thing.
それは非常に異なることになるでしょう。
Now there were additionally some benchmarks.
さらにいくつかのベンチマークがありました。
So we can see here the comparison between GPT-4 Vision and Gemini 1.5 Pro on a one hour video QA.
ここではGPT-4 VisionとGemini 1.5 Proの1時間のビデオQAの比較が見られます。
And experiments are run by sampling one video frame per second and linearly subsampling 16 or 150 frames.
実験は、1秒ごとに1つのビデオフレームをサンプリングし、16フレームまたは150フレームを線形にサブサンプリングして実行されます。
And you can see here that Gemini 1.5 Pro outperforms GPT-4 with Vision substantially because not only does it outperform the 16 frames and the 150 frames, it does actually support full video, whereas GPT-4 with Vision currently doesn't.
ここで、Gemini 1.5 ProがGPT-4 with Visionを大幅に上回っていることがわかります。なぜなら、16フレームと150フレームを上回るだけでなく、実際にはフルビデオをサポートしているからです。一方、GPT-4 with Visionは現時点ではサポートしていません。
Now in addition, we can take a look at some of the benchmarks to see exactly what is going on.
さらに、いくつかのベンチマークを見て、具体的に何が起こっているのかを確認することができます。
You can see right here that the core capabilities like math, science, and reasoning, and coding, and instruction following are up across the board in this model.
このモデルでは、数学、科学、推論、コーディング、指示の従うなど、核となる能力が全体的に向上していることがわかります。
And what's crazy is that in terms of the actual families of model, like if we take a look at where Gemini Pro 1.5 sits, we know that Pro 1.5 sits in the middle in terms of what the model is going to be able to do.
そして驚くべきことは、Gemini Pro 1.5がどのようなモデルファミリーに位置しているかを見ると、Pro 1.5がモデルができることの中間に位置していることがわかります。
So that leads me to believe that potentially we could be getting an Ultra 2.0 or an Ultra 1.5.
それが私に、おそらくUltra 2.0やUltra 1.5を手に入れる可能性があると考えさせます。
But with these benchmarks, we can see that Gemini 1.5 is literally better across the board and it has a hugely increased contact length that's going to allow a lot more things.
これらの基準を使うと、Gemini 1.5は実際に全般的に優れており、接触長が大幅に増加して、さらに多くのことが可能になります。
Now, if you want to take a look at some of the individual detailed benchmarks, you can see the math ones right here.
個々の詳細なベンチマークのいくつかを見たい場合は、こちらで数学のものを見ることができます。
You can see that 1.5 Pro outperforms it on the Hellaswag, doesn't on the MMLU, does on the GSM8K, does on the math, doesn't on the rest of these, and does on the BigBench.
1.5 ProがHellaswagでそれを上回っているのが見えますが、MMLUではそうではなく、GSM8Kではそうであり、数学ではそうであり、残りの基準ではそうではなく、BigBenchではそうです。
So across the board, you can see that Gemini 1.5 Pro is really taking the cake here in terms of what is possible with an AI system.
全般的に見て、Gemini 1.5 ProがAIシステムで可能なことに関して、本当に優れていることがわかります。
And of course, in addition, the detailed benchmarks encoding, we can see that it's off and half in terms of these capabilities, but it is 77.7% on the natural two code benchmarks.
そしてもちろん、詳細な基準エンコーディングにおいて、これらの能力に関しては半分オフであり、自然な2つのコードの基準では77.7%です。
And one thing that I did want to find out was of course how they train this model.
そして私が知りたかったことの1つは、もちろんこのモデルをどのようにトレーニングしたのかです。
And like Gemini 1.0 Ultra and Gemini 1.0 Pro, Gemini 1.5 Pro was actually trained on multiple 496 chip pods of Google's TPU or VU accelerators distributed across multiple data centers and on a variety of multimodal and multilingual data.
Gemini 1.0 UltraやGemini 1.0 Proと同様に、Gemini 1.5 Proは実際にGoogleのTPUまたはVUアクセラレータの複数の496チップポッドでトレーニングされ、複数のデータセンターとさまざまな多モードおよび多言語データで分散されました。
Now, with that being said, are you excited for Google's family of models that are absolutely incredible?
それでは、Googleの信じられないほど素晴らしいモデル群に興奮していますか?
And are you going to be taking a look and using this model in Google's AI?
このモデルをGoogleのAIで見て、使用する予定ですか?
And of course, with things like the video capabilities that haven't been done by any other AI system before, are you excited to potentially use these to reason and figure out things in certain videos?
もちろん、他のどのAIシステムでも行われていないビデオ機能など、これらを使って特定のビデオで理由をつけたり、物事を理解することに興奮していますか?
Either way, I'm excited for Google to finally beef up the competition and make a more competitive AI space, but it will be interesting to see how other AI companies do respond, because right now it seems that Google is well in the lead.
とにかく、Googleが競争を強化し、より競争力のあるAI空間を作ることに興奮していますが、他のAI企業がどのように反応するか興味深いです。現時点では、Googleがリードしているように見えます。
Benchmarks are here and the benchmarks are clear, and some of the AI systems right now don't even have some of these capabilities.
ベンチマークはここにあり、ベンチマークは明確ですが、現在の一部のAIシステムにはこれらの機能すら備わっていないものもあります。
So with that being said, if you did enjoy this, don't forget to leave your comment below on where you think Google is going to go next.
もしこれを楽しんだなら、Googleが次にどこに進むと思うかについてのコメントをお忘れなく。
Thank you.
ありがとうございました。
この記事が気に入ったらサポートをしてみませんか?