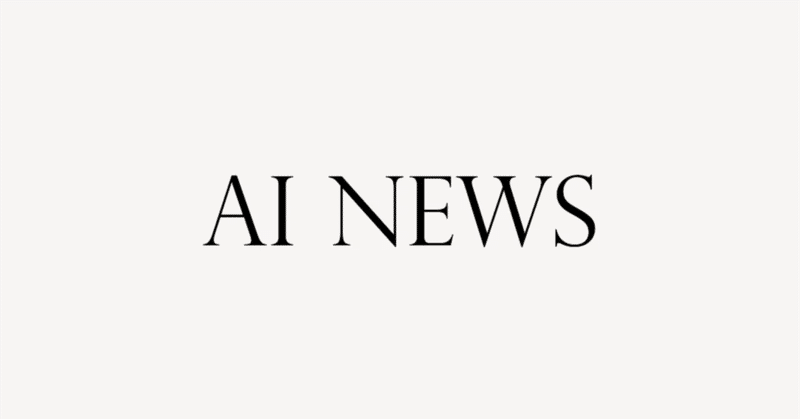
【自学習と高速シミュレーション:マインクラフトを舞台にしたAIの未来】英語解説を日本語で読む【2024年1月23日|@Wes Roth】
この動画では、マインクラフトとVoyagerプロジェクトについて説明されています。マインクラフトは創造性を重視した人気ゲームで、14億人以上のプレイヤーがいます。Voyagerはマインクラフト内で自動プレイし、新しいスキルを自己学習できます。また、Metamorphアルゴリズムは数千のロボットを制御でき、Isaac Simは高速の物理シミュレーションを可能にします。将来的にはAIエージェントが自律的になり、仮想と現実の世界を超えて活動することが予想されています。
公開日:2024年1月23日
※動画を再生してから読むのがオススメです。
Earlier this year, I led the Voyager Project, and there's no game better than Minecraft for the infinite creative things it supports.
今年の初め、私はVoyagerプロジェクトを率いていましたが、無限の創造的なことをサポートするマインクラフトほど素晴らしいゲームはありません。
Minecraft has 140 million active players, and Minecraft is so insanely popular because it's open-ended.
マインクラフトには1億4000万人のアクティブプレイヤーがおり、オープンエンドなためとてつもなく人気があります。
It does not have a fixed storyline for you to follow, and you can do whatever your heart desires in the game.
固定されたストーリーラインがなく、ゲーム内で心が望むことを何でもできます。
And when we set Voyager free in Minecraft, we see that it's able to play the game for hours on without any human intervention.
そして、Voyagerをマインクラフトで自由にしたとき、人間の介入なしに何時間もゲームをプレイできることが分かります。
The video here shows snippets from a single episode of Voyager where it just keeps going.
こちらのビデオは、Voyagerの単一エピソードからの断片を示しており、ただひたすらに続きます。
It can explore the terrains, mine all kinds of materials, fight monsters, craft hundreds of recipes, and unlock an ever-expanding tree of skills.
さまざまな地形を探索し、あらゆる種類の素材を採掘し、モンスターと戦い、何百ものレシピを作成し、絶えず拡大するスキルツリーを解除することができます。
Voyager is able to not only master but also discover new skills along the way, and we did not pre-program any of this.
Voyagerは、新しいスキルを発見するだけでなく、マスターすることもでき、これらは事前にプログラムされていませんでした。
It's all Voyager's idea, and this is what we call lifelong learning, where an agent is forever curious and forever pursuing new adventures.
これはすべてVoyagerのアイデアであり、これが我々が「生涯学習」と呼ぶものです。ここでは、エージェントが常に好奇心旺盛で、新しい冒険を追い求めています。
Compared to Arago, Voyager scales up massively on a number of things he can do but still controls only one body in Minecraft.
Aragoと比較して、Voyagerはできることの数を大幅に拡大していますが、マインクラフトではまだ一つの体だけを制御しています。
So the question is, can we have an algorithm that works across many different bodies?
そこで、質問です。多くの異なる体で機能するアルゴリズムは可能でしょうか?
Enter Metamorph.
Metamorphが登場します。
It is an initiative I co-developed at Stanford.
これは私がスタンフォードで共同開発したイニシアティブです。
We created a foundation model that can control not just one but thousands of robots with very different arm and leg configurations.
我々は、一つではなく何千ものロボットを制御できる基礎モデルを作成しました。これらのロボットは非常に異なる腕と脚の構成を持っています。
We show that Metamorph is able to control thousands of robots to go upstairs, cross difficult terrains, and avoid obstacles.
Metamorphは、階段を上がり、難しい地形を渡り、障害物を避けるために何千ものロボットを制御できることを示しました。
Compared to Voyager, Metamorph takes a big stride towards multi-body control.
Voyagerと比較して、Metamorphはマルチボディ制御に向けて大きな一歩を踏み出しています。
And now, let's take everything one level further and transfer the skills and embodiments across realities.
そして今、すべてをさらに一つのレベル上に持っていき、スキルと身体性を現実間で転送しましょう。
Enter Isaac Sim, NVIDIA's simulation effort.
Isaac Simの登場です。これはNVIDIAのシミュレーション努力です。
The biggest strength of Isaac Sim is to accelerate physics simulation to a thousand X faster than real-time.
Isaac Simの最大の強みは、リアルタイムよりも1000倍速い物理シミュレーションを加速することです。
For example, this character here learns some impressive martial arts by going through 10 years of intense training in only 3 days of simulation time.
例えば、こちらのキャラクターは、シミュレーション時間のたった3日間で10年間の激しいトレーニングを経て、印象的な武術を学びます。
So it's very much like the virtual sparring dojo in the movie Matrix.
これは、映画「マトリックス」の仮想スパーリング道場のようなものです。
And what's more, Isaac Sim can procedurally generate worlds with infinite variations so that no two look the same.
さらに、Isaac Simは無限のバリエーションを持つ世界を手続き的に生成できるため、2つの世界が同じに見えることはありません。
If an agent is able to master 10,000 simulations, then it may very well just generalize to our real physical world, which is simply the 10,000 and 1st reality.
エージェントが1万のシミュレーションをマスターできれば、私たちの実際の物理世界、つまり1万1番目の現実にも一般化する可能性が非常に高いです。
As we progress through this map, we will eventually get to the upper right corner, which is a single agent that generalizes across all three axes, and that is the foundation agent.
このマップを進むにつれて、最終的には右上の角に到達します。それは3つの軸すべてにまたがって一般化する単一のエージェント、基礎エージェントです。
And we train it by simply scaling it up massively across lots and lots of realities.
そして、我々はそれを、たくさんの現実で大規模にスケールアップすることで訓練します。
I believe in a future where everything that moves will eventually be autonomous, and one day we will realize that all the AI agents across Wally, Star Wars, Ready Player One, no matter if they are in the physical or virtual spaces, will all just be different prompts to the same foundation agent.
私は、動くすべてのものが最終的に自律的になり、ウォーリー、スターウォーズ、レディプレーヤー1に登場するすべてのAIエージェントが、物理的空間であれ仮想空間であれ、すべて同じ基礎エージェントへの異なるプロンプトにすぎないことに気づく日が来ると信じています。
And that, my friends, will be the next Grand Challenge our request for AI.
そして、それが私たちのAIに対する次の大きな挑戦、私たちの要求になるでしょう。
So this is Dr. Jim Fan, and outside of OpenAI, he's probably one of my favorite AI researchers.
これはジム・ファン博士です。OpenAIの外では、彼が私のお気に入りのAI研究者の一人です。
Recently, he posted this announcement that his TED Talk is finally live.
最近、彼のTEDトークがついに公開されたというアナウンスメントを投稿しました。
He proposed the recipe for the foundation agent, one model to rule the mod, if you will, a single model that learns how to act in different worlds.
彼は基礎エージェントのレシピを提案しています。もしあなたが意志を持っていれば、異なる世界で行動する方法を学ぶ単一のモデルです。
Now, LLMs scale across lots and lots of texts.
今、LLMは多くのテキストにわたってスケールします。
Foundation agent scales across lots and lots of realities.
基礎エージェントは、多くの現実にわたってスケールします。
It is able to master 10,000 diverse simulated realities.
それは1万の多様なシミュレーションされた現実をマスターすることができます。
It may very well generalize to our physical world, which you can think of as simply the 10,000 and 1st reality.
それは非常にうまく私たちの物理的世界に一般化する可能性があります。それはあなたが1万1番目の現実として単純に考えることができるものです。
I did not know this, but TED Talks do not have teleprompters.
私はこれを知りませんでしたが、TEDトークにはテレプロンプターがありません。
All he had is a confidence monitor at his feet showing the current slide and timer.
彼が持っていたのは、現在のスライドとタイマーを表示する足元のコンフィデンスモニターだけです。
I got to say, he did a phenomenal job.
彼は素晴らしい仕事をしたと言わざるを得ません。
Congratulations to him, and I'm very excited about seeing more.
おめでとうございます。そして、もっと見ることに非常に興奮しています。
I recommend everyone checks out the full talk at ted.com.
ted.comでのフルトークを皆さんに是非チェックしていただきたいとお勧めします。
I'll link it in the show notes below because he goes into a lot more depth about what he's proposing.
彼が提案していることについてもっと詳しく語っていますので、下のショーノートにリンクを貼っておきます。
Now, Dr. Jim Fan was one of the people behind Voyager, the open-ended embodied agent with Large Language Models.
さて、ジム・ファン博士は、大規模言語モデルを搭載したオープンエンドのエンボディエージェント「Voyager」の立役者の一人でした。
That's him right there, Dr. Linki Jim Fan.
それが彼、リンキ・ジム・ファン博士です。
He's one of the senior AI researchers over there at NVIDIA.
彼はNVIDIAでの上級AI研究者の一人です。
The really impressive thing about Voyager was that it was able to learn continuously.
Voyagerの本当に印象的な点は、連続して学習できることでした。
As you can see here, a lot of these other ones, including AutoGPT, eventually they plateau.
ここで見ることができるように、AutoGPTを含む他の多くのものは、最終的に頭打ちになります。
They stop learning.
学習を止めます。
They don't progress.
進歩しません。
In fact, even Voyager without its skill library plateaus at some point.
実際、スキルライブラリーがなければVoyagerもある時点で頭打ちになります。
It stops improving.
改善が止まります。
The full architecture Voyager keeps going and going and going and going.
しかし、フルアーキテクチャのVoyagerは途切れることなく続けます。
To get it, it's a lifelong learner.
それを得るためには、一生涯学び続けるものです。
It has an automatic curriculum where it learns skills.
自動的なカリキュラムがあり、そこでスキルを学びます。
It writes code that executes those skills.
それらのスキルを実行するコードを書きます。
The code is basically the skill.
コードは基本的にスキルそのものです。
It tests it out in the environment to see if it works, the self-verification, and adds that skill to the skill library.
それは環境でテストして、動作するかどうかを確認し、自己検証を行い、そのスキルをスキルライブラリに追加します。
Now, if you want to see this in detail, I did a video.
この詳細を見たい場合は、私が動画を作成しました。
I'll link that in the show notes.
それもショーノートにリンクします。
This was one of the first big AI research studies that kind of blew my mind and opened me up to what was possible.
これは私の心を打ち震わせ、可能性を広げてくれた最初の大規模なAI研究の一つでした。
I had no idea that GPT-4 just out of the box could do all of this, much less without vision.
GPT-4が箱から出してすぐにこれだけのことができるとは、目も当てられませんでした。それも視覚なしで。
Since then, the same team dropped another massive bombshell.
それ以来、同じチームが別の大きな驚きを発表しました。
By the way, a lot of them are at NVIDIA.
ちなみに、彼らの多くはNVIDIAにいます。
This was sort of NVIDIA's research arm.
これはNVIDIAの研究部門の一環でした。
They taught a robot how to spin a pencil in its fingers like this, something that was before considered near impossible.
彼らはロボットが指でこのように鉛筆を回転させる方法を教えました。これは以前はほぼ不可能と考えられていました。
But how they did it was even more interesting.
しかし、それをどのように行ったかはさらに興味深いです。
Here's that paper, Eureka: Human-Level Reward Design via Coding Large Language Models.
その論文はこちら、「Eureka: Human-Level Reward Design via Coding Large Language Models」です。
Again, they use GPT-4 here.
再び、ここではGPT-4を使用しています。
GPT-4 codes what's called reward models for various robots that are simulated in Isaac Sim, NVIDIA's simulation for robots.
GPT-4は、NVIDIAのロボットシミュレーションであるIsaac Simでシミュレートされるさまざまなロボットのための報酬モデルをコーディングします。
The code is tested out.
コードはテストされます。
It's ran through in the Isaac Sim.
それはIsaac Simで実行されます。
And then, the results are given back to the GPT-4 with feedback.
そして、その結果はフィードバックと共にGPT-4に戻されます。
It looks at it, tries again, and this keeps going in circles as GPT-4 tries to improve on its ability to write code that gets these simulated robots to do various functions.
GPT-4はそれを見て、再度試みます。これはGPT-4がこれらのシミュレートされたロボットにさまざまな機能を実行させるコードを書く能力を向上させるために繰り返し行われます。
Again, you can see the full video that goes into details about how it did, but the main point, I think, is it did very, very well.
再び、詳細については全動画を見ることができますが、主なポイントは、それが非常にうまく行ったということです。
A++++.
A++++です。
It was better at writing reward code for robots than human experts were.
それは人間の専門家よりもロボットのための報酬コードを書くのが上手でした。
It came up with novel solutions, new never-before-seen solutions that humans didn't even think of.
それは人間が考えもしなかった新しい解決策、前例のない解決策を生み出しました。
And finally, Dr. Jim Fan, towards the end of the lecture, was talking about how that can translate to Foundation agents, agents that are basically able to do anything in any world, regardless of physics or complexity or friction or whether it's a digital world or the real world, our world.
そして最後に、ジム・ファン博士は講演の最後に、それがどのようにファウンデーションエージェントに翻訳できるかについて話しました。ファウンデーションエージェントは、物理法則や複雑さ、摩擦、デジタル世界であれ現実世界であれ、どんな世界でも何でもできるエージェントです。
They go into your simulation, they learn how to do that.
それらはあなたのシミュレーションに入り、それを学びます。
The time runs very, very fast.
時間は非常に速く進みます。
That simulation, years pass very quickly, and millions of these robots work very, very hard in the simulation to figure out how to do stuff.
そのシミュレーションでは、年が非常に速く過ぎます。そして、何百万ものロボットが非常に一生懸命そのシミュレーションで何かをする方法を見つけるために働きます。
When they do it right, they get rewards, plus one little robot.
正しく行うと、ロボットには報酬が与えられます、プラスワンリトルロボット。
And when that sort of neural network, the AI brain, is then taken out of the simulation and put into an actual physical robot, well, it retains all those skills.
そして、そのようなニューラルネットワーク、AIの脳がシミュレーションから取り出されて実際の物理的なロボットに入れられると、それはすべてのスキルを保持します。
It's still really, really good at doing the things that it was supposed to do.
それはまだそれがするべきことをするのが本当に上手です。
So the simulation learning translates really well into real-life physical scenarios.
したがって、シミュレーション学習は実際の物理的なシナリオに非常にうまく翻訳されます。
Now, NVIDIA isn't the only one that is seeing these results.
NVIDIAだけがこれらの結果を見ているわけではありません。
Google DeepMind, of course, has seen a lot of similar results.
もちろん、Google DeepMindも多くの類似した結果を見ています。
We're seeing the same thing from OpenAI and some of their earlier research.
OpenAIとその初期の研究からも同じことが見られます。
So this is the next generation of how robotics will be trained in these time compression chambers where time runs very, very fast, and all they do is train.
これは、ロボティクスがこれらの時間圧縮チャンバーで訓練される次世代の方法です。そこでは時間が非常に速く進み、彼らは訓練することしかしません。
Which does that remind you of something?
それはあなたに何かを思い出させますか?
Feel like we've heard this idea somewhere before?
以前どこかでこのアイデアを聞いたような気がしませんか?
Ah, yes, the hyperbolic Time Chamber from Dragon Ball Z, a cartoon out of the90s.
ああ、そうですね、ドラゴンボールZの精神と時の部屋、90年代のアニメです。
The characters would go into this hyperbolic Time Chamber, spend a very long time there, and come out fully trained and ready to rock and roll.
登場人物たちはこの精神と時の部屋に入り、非常に長い時間を過ごし、訓練を積んで元気に出てきます。
One reason why this is important is that it's important to understand that NVIDIA isn't just this chip company.
これが重要な理由の一つは、NVIDIAが単なるチップ会社ではないということを理解することが重要だからです。
It doesn't just make graphic chips for computers so you can play games really, really fast at eye-watering resolutions.
NVIDIAは、ただコンピューター用のグラフィックチップを作っているだけではありません。それによってゲームを非常に速く、目がくらむほどの解像度でプレイできます。
It is also a world leader in AI research.
NVIDIAはまた、AI研究の世界的リーダーでもあります。
It's getting really good at simulating factories, robots, physics, constructing those robots in those simulated realities, and testing them out.
NVIDIAは、工場やロボット、物理学のシミュレーションが非常に上手になっており、それらのシミュレートされた現実においてロボットを構築し、テストを行っています。
And those realities function very, very similarly to how our base reality functions, the reality in which we live in.
そして、それらの現実は、私たちが生活する基本的な現実と非常に似た方法で機能しています。
But the more and more simulations we build, the deeper and deeper it goes.
しかし、より多くのシミュレーションを構築するほど、それはより深くなっていきます。
The more and more you have to ask yourself the question, is this indeed the base reality?
より多くのシミュレーションがあるほど、これは本当に基本的な現実なのかと自分自身に問いかけなければなりません。
Or are we just little automatons running in here, learning new skills, figuring out how to do stuff for the benefits of the people above us in the real base reality who are probably wondering if their reality is the base reality?
それとも、私たちはここで小さな自動機械として動いており、実際の基本的な現実にいる上位の人々のために新しいスキルを学び、物事をどうやって行うかを理解しているのでしょうか?彼らはおそらく自分たちの現実が基本的な現実かどうか疑問に思っているでしょう。
All right, I'll just leave it right there.
では、この辺で終わりにしましょう。
I hope you enjoyed that.
楽しんでいただけたら幸いです。
My name is Wes Roth, and thank you for watching.
私の名前はウェス・ロスです。ご覧いただきありがとうございました。
この記事が気に入ったらサポートをしてみませんか?