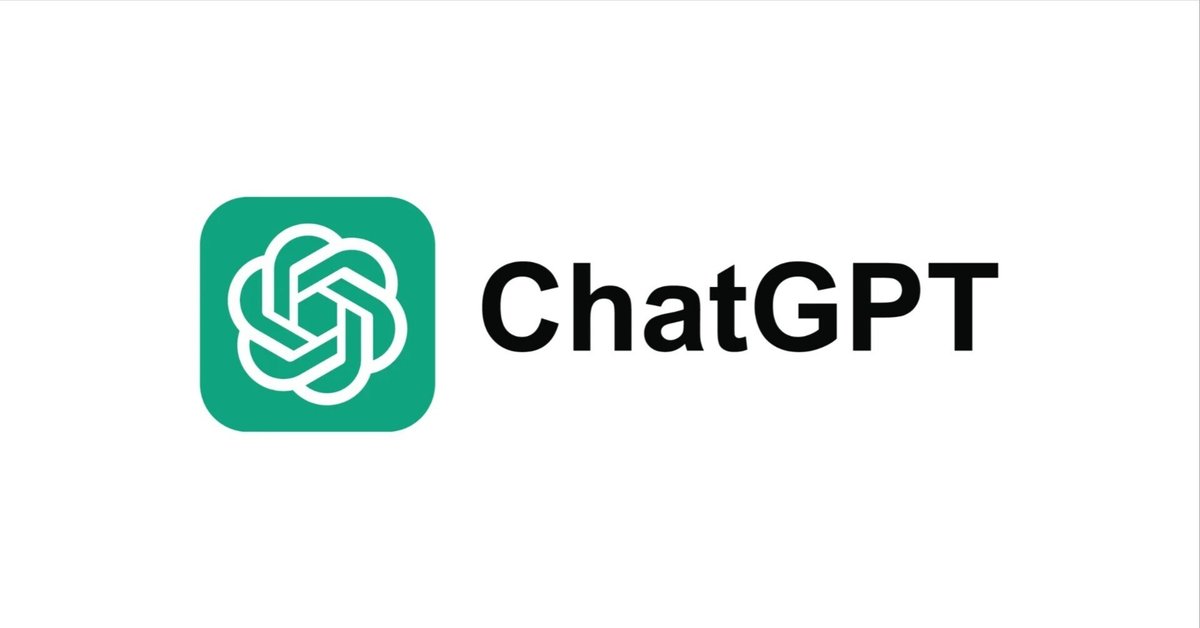
【GPT-4】英語解説を日本語で読む【2023年5月8日|@AI Explained】
GPT-4を使った賢い結果の取得方法だけでなく、最先端のMMLUベンチマークを上回る可能性があるSmartGPTシステムも紹介しています。
公開日:2023年5月8日
※動画を再生してから読むのがオススメです。
I have three goals for this video.
このビデオには3つの目標があります。
First, I want to show you a way of using GPT-4 to get smarter results.
まず、GPT-4を使って、よりスマートな結果を得る方法を紹介したい。
Second, I want to argue that the benchmark results we have for GPT-4 do not reflect its full abilities.
2つ目は、GPT-4のベンチマーク結果が、GPT-4の能力を十分に反映していないことを主張することです。
And third, I want to show you a system that I am developing, somewhat cheekily called Smart GPT, that is already showing significant results on official benchmarks.
そして3つ目は、私が開発中のSmart GPTというちょっと生意気な名前のシステムで、すでに公式ベンチマークで大きな結果を出しているものを紹介したいと思います。
It remains to be fully optimized which I think is exciting in itself.
まだ完全に最適化されていませんが、それ自体がエキサイティングなことだと思います。
I have shown the system to people at OpenAI who have been quite impressed and I'm going to end with some reflections on where that might leave us for GPT-5.
このシステムをOpenAIの人たちに見せたところ、非常に感心されたので、最後にGPT-5の方向性について少し考えてみたいと思います。
But before I get into how it works, I just want to show you one example of it in action to whet your appetite.
しかし、その仕組みに触れる前に、食欲を刺激するために、実際に使われている例を1つだけお見せしたいと思います。
This example comes from a TED talk that was released this week.
この例は、今週発表されたTEDの講演から得たものです。
So suppose I left five clothes to dry out in the sun and it took them five hours to dry completely.
5枚の服を天日干しにして、完全に乾くまで5時間かかったとします。
How long would it take to dry 30 clothes?
30着の服を乾かすとしたら、どれくらいの時間がかかるでしょうか?
GPT-4, the newest greatest AI system says 30 hours.
GPT-4、最新の最も偉大なAIシステムは30時間と言っています。
Not good.
まずいな。
On the left you can see GPT-4's original answer and it gives this answer pretty consistently whenever you prompt it with the question provided.
左側はGPT-4のオリジナルの答えで、質問を投げかけると常にこの答えが返ってきます。
On the right you can see the final answer from the Smart GPT model which is correct and it consistently gives that answer.
右側は、スマートGPTモデルの最終的な答えで、これは正しく、一貫してこの答えを出しています。
I really like how it gives context as well and it provides some of the assumptions that it had in giving this correct answer.
このように、文脈を示しながら、正しい答えを出すための前提条件を示しているところが、とても気に入っています。
Now don't you worry, there will be plenty more examples to go through in this video including another one from that TED talk.
心配しないでください。このビデオでは、TEDの講演からもう1つ例を挙げて、さらにたくさんの例を紹介します。
But first I want to give you an overview of what is this Smart GPT model?
しかし、その前に、このスマートGPTモデルとは何なのか?
Where did I get my inspiration for it from and how does it work?
私はどこからインスピレーションを得て、どのように機能するのでしょうか?
I'm going to keep it fairly simple because it's the beginning of the video and I know a lot of people won't really care about the inner details.
ビデオの冒頭なので、多くの人は細かいことは気にしないと思うので、かなりシンプルに説明するつもりです。
That will come later in the video.
それはビデオの後半で説明します。
But the high level overview is this.
しかし、ハイレベルな概要はこうです。
There are at least three things that have been proven to improve the outputs of GPT-4.
GPT-4の出力を向上させることが証明されているものが、少なくとも3つあります。
What's called chain of thought prompting, sometimes called step-by-step prompting, reflection or finding its own errors, and I did an entire video on this called GPT-4 Can Self-Improve, and dialoguing with itself.
思考連鎖プロンプティングと呼ばれるもの、ステップバイステッププロンプティングと呼ばれるもの、リフレクション、つまり自分自身の誤りを見つけることです。
Entering into a back and forth on its own outputs and deciding which one is best.
GPT-4は自己改善できる』というビデオもありますが、自分自身と対話することです。
You can see the title of the papers which contain much more detailed results of course linked above.
もちろん、もっと詳しい結果が書かれた論文のタイトルは、上のリンクから見ることができます。
Now the first paper only came out a few days ago midway through my testing so my results don't even reflect the full capacity of the model.
最初の論文は数日前に発表されたばかりで、私がテストしている途中なので、私の結果はモデルの全能力を反映したものではありません。
And even if there's nothing else you take from this video, the results from this paper can instantly improve the outputs you get from GPT-4.
この動画から得られるものが他になかったとしても、この論文の結果は、GPT-4から得られる出力を即座に向上させることができます。
Many of you might remember that prompting GPT-4 with let's think step-by-step improves its results.
GPT-4に「Let's think step-by-step」と促すと、その結果が改善されることを覚えている方も多いのではないでしょうか。
To give you a very quick reference point, just asking a question to GPT-4 gives you 81% accuracy.
ごく簡単な例を挙げると、GPT-4に質問するだけで、81%の精度が得られます。
With that prompt let's think step-by-step it goes up to 86%. But algorithmically the paper found an improved prompt that can give you even better results, 89% accuracy.
それが、「ステップバイステップで考えよう」と促すと86%になる。しかし、この論文では、アルゴリズム的に改良されたプロンプトを発見し、89%というさらに高い精度の結果を得ることができました。
All we do, and this is the first part of Smart GPT, is we add answer.
これは、Smart GPTの最初の部分ですが、答えを追加するだけです。
Let's work this out in a step-by-step way to be sure we have the right answer.
正しい答えが出るように、ステップバイステップで解決していきましょう。
Now I have so much to say about why I think this works but I know many of you won't be that interested in my theories so I'm going to save them to the end for those who are interested.
なぜこの方法が有効なのか、言いたいことは山ほどあるのですが、私の理論にそれほど興味を持たない方も多いでしょうから、興味のある方のために最後まで取っておくことにします。
Some of you just want the results so I'm going to get to those first.
結果を知りたいという方もいらっしゃるでしょうから、まずはそれをお伝えします。
So far you might be thinking well thanks Philip that's a cool prompt I'm going to use that but what's this whole Smart GPT about?
ここまでは、「なるほど、フィリップのプロンプトはかっこいいな、使ってみようかな」と思われたかもしれませんが、このSmart GPTというのはどういうものなのでしょうか?
Is it just a single prompt?
1つのプロンプトだけなのでしょうか?
No, I believe with evidence there are ways of leveraging even better results than just using a great chain of thought prompt.
いいえ、私は、優れた思考連鎖のプロンプトを使うだけでなく、さらに優れた結果を活用する方法があることを、根拠をもって信じています。
So let's move on to the next part of the system, these different outputs in the middle.
では、システムの次の部分、つまり真ん中にあるさまざまな出力について説明しましょう。
For my tests, I typically did three outputs, but of course, depending on the context window, it could be far more than that.
私のテストでは、通常3つの出力を行いましたが、もちろん、コンテキストウィンドウによっては、それよりもはるかに多くなることもあります。
I'm going to talk about ways I could further improve this model, or we could, later on in the video.
このモデルをさらに改良する方法については、ビデオの後半でお話しするつもりですが、私たちにもできるかもしれません。
Just to restate these outputs are when you take the user input and add the word question at the start and then at the end add answer.
もう一度言いますが、これらの出力は、ユーザーの入力を受けて、最初にquestionという単語を追加し、最後にanswerを追加したときのものです。
Let's work this out in a step-by-step way to make sure we have the right answer.
正しい答えが出るように、ステップ・バイ・ステップで作業してみましょう。
At this moment many of you are thinking what is the point of multiple outputs?
今この瞬間、多くの方が「マルチ出力の意味は何だろう」と思っていることでしょう。
It's GPT-4 it's just going to give you the answer it thinks is best and that's it.
GPT-4は、自分が一番良いと思う答えを出してくれる、ただそれだけなのです。
Well actually it doesn't quite work like that.
しかし、実はそうではないのです。
These models have a temperature between 0 and 1.
これらのモデルには、0から1までの温度があります。
I believe the default for GPT-4 might be around 0.5 and simplifying massively this determines how creative or conservative the model is in giving its outputs.
GPT-4のデフォルトは0.5くらいだと思いますが、非常に単純化すると、この温度によってモデルの出力がどれだけ創造的か保守的かが決まります。
So given that GPT-4 tries to be fairly creative you don't get the same output every time.
ですから、GPT-4はかなりクリエイティブにしようとするため、毎回同じ出力が得られるわけではありません。
The output is randomly sampled according to an internal probability distribution.
出力は、内部の確率分布に従って、ランダムにサンプリングされます。
So you can get situations and I face this hundreds of times where some of the outputs are correct and others are incorrect and this is where reflection comes in.
ですから、ある出力が正しくて、ある出力が正しくないという状況に、私は何百回も直面します。
Sometimes, definitely not always but sometimes quite often, GPT-4 can detect the errors in its own output.
GPT-4は、自分の出力の誤りを検出できることがあります。
Many of you will notice at this point that the prompt I used to elicit GPT-4 to spot its own errors contains the same step-by-step prompt I used earlier, which has been shown to produce good results.
このとき、GPT-4が自らの誤りに気づくように促すために使ったプロンプトが、先ほど使ったステップバイステップのプロンプトと同じで、良い結果を生むことが示されていることに、多くの人が気づくでしょう。
So to summarize sometimes at this stage GPT-4 detects the errors that some of its outputs have made.
つまり、この段階でGPT-4が自分の出力の誤りを発見することもあるということです。
Definitely not always, there are certain questions it just simply can't spot the error.
しかし、GPT-4がエラーを発見できない問題もあります。
But sometimes it can, and then I get it to engage in a dialogue using a format similar to one in this paper published last month.
しかし、時には発見できることもあります。その時は、先月発表されたこの論文のようなフォーマットで対話させます。
It's a short dialogue and this is the step I believe that can be most optimized.
短い対話ですが、これが最も最適化できるステップだと考えています。
In the future I envision an entire council of advisors made up of GPT-4 imitating mathematicians, judges etc.
将来的には、GPT-4が数学者や裁判官などを模倣して構成される顧問会議全体を想定しています。
At the moment it's just being a resolver and printing a final improved output.
今のところ、リゾルバーとして、最終的に改善された出力をプリントするだけです。
Anyway, I'm going to get back to the theory later in the video, because I know some of you will be getting bored at this stage and want to see more practical examples and the results from my benchmark tests.
とにかく、ビデオの後半で理論に戻ろうと思います。なぜなら、この段階で退屈している人もいるでしょうし、もっと実用的な例やベンチマークテストの結果を見たいと思っているからです。
As I don't have the GPT-4 API key yet, I had to manually input each of these steps hundreds of times, waiting sometimes three hours between each go because you can only do 25 messages every three hours.
GPT-4のAPIキーをまだ持っていないので、これらのステップを何百回も手動で入力する必要がありました。3時間ごとに25メッセージしか実行できないので、1回の入力に3時間待つこともありました。
On the left you can see the three outputs when you ask it to think step by step and then you have the researcher step in the middle and at the top right and finally the resolver step.
左側は、ステップバイステップで考えるように指示したときの3つの出力で、真ん中と右上に研究者のステップ、最後にリゾルバのステップを示します。
Notice here I was using the original let's think step by step because the paper hadn't yet been published on improving that prompt.
ここでは、このプロンプトの改良に関する論文がまだ発表されていなかったので、オリジナルの「Let's think step by step」を使っていることに注意してください。
It's time for the second example from that TED talk and then I definitely will get on to the benchmarks.
TEDの講演から2つ目の例を紹介し、ベンチマークの話に移ります。
A different one I have 12 litre jog and 6 litre jog and I want to measure 6 litres how do I do it?
12リットルのジョギングと6リットルのジョギングがありますが、6リットルを測るにはどうしたらいいでしょうか。
Just use the 6 litre jog right?
6リットルのジョグを使えばいいんですよね?
GPT-4 spits out some very elaborate nonsense.
GPT-4は、非常に精巧なナンセンスを吐き出しています。
Of course I tested smart GPT with that question and you can see the difference between the original GPT-4 which gives this incredibly convoluted bad answer and smart GPT the final answer output.
もちろん、この問題でsmart GPTをテストしてみました。この信じられないほど複雑な答えを出すオリジナルのGPT-4と、最終的な答えを出力するsmart GPTとの違いを見てください。
Now at this point I know many of you will be impressed but you'll be thinking I don't have time to input things five times.
さて、この時点で多くの方が感動されると思いますが、5回も入力している暇はないと思われるでしょう。
Well I'm developing a model where it can all be done automatically.
そこで私は、この作業をすべて自動で行うモデルを開発しました。
Here is a preview of how it works but of course at the moment it has to use GPT-3.5 turbo because I don't have the API key of GPT-4.
もちろん、GPT-4のAPIキーは持っていないので、GPT-3.5ターボを使用する必要があります。
The epic thing is this you just ask a single question.
壮大なのは、たった1つの質問をするだけでいいということです。
I've written ask smart GPT a question, and of course, it does take a little bit longer to respond because it's doing five or six calls via API, but it does output the final answer from the resolver step.
私はスマートGPTに質問を書いていますが、もちろん、APIを介して5つか6つの呼び出しを行っているので、少し時間がかかりますが、リゾルバステップからの最終回答を出力します。
I will be honest and say that GPT-3.5 isn't as good at reflecting or resolving, but this is an example of a question where the original ChatGPT consistently gets it wrong, and smart GPT-3.5 gets it right using this program.
正直なところ、GPT-3.5 はリゾルバがあまり得意ではありませんが、これはオリジナルの ChatGPT が一貫して間違っていた問題を、このプログラムを使ってスマートな GPT-3.5 が正しく解決している例と言えます。
Remember all you have to do as a user is type in a question as normal and it goes through this entire five or six step process behind the scenes.
ユーザーとしては、普通に質問を入力するだけで、裏でこの5~6段階のプロセスを経ていることを忘れないでください。
By the way this was a question from MMLU which is a famous benchmark which I'll get to in a second.
ちなみに、これは有名なベンチマークであるMMLUからの出題です(後で説明します)。
Here's one last practical example before I get to that benchmark.
そのベンチマークの話をする前に、最後の実践例を紹介します。
I know many teachers use ChatGPT and GPT-4 to create quizzes for their classes and here is the same question put through GPT-4 and smart GPT.
多くの先生がChatGPTやGPT-4を使って授業の小テストを作成していると思いますが、同じ問題をGPT-4とsmart GPTにかけたものです。
The question is create a high school algebra quiz with five questions and answers and explanations at the end.
問題は、5つの質問と答え、最後に解説がある高校代数のクイズを作成することです。
Now points for spotting the difference but if the teacher had handed out the original quiz look at the answers for question five.
もし、先生がオリジナルの小テストを配布したとしたら、第5問の解答を見てください。
It says the answers are one and 1.5 but then in the explanation it gives the final answers which are correct by the way of three and 0.5 so that would really confuse some students.
回答は1と1.5だと言っていますが、説明では最終的な正しい答え(ちなみに3と0.5)が示されているので、これはいくつかの生徒を混乱させるでしょう。
At the reflection stage smart GPT spotted that error and resolved it and as you can see the answer for question five has the correct answers straight away.
しかし、smart GPTでは、この誤りを発見し、解決しました。そして、問題5の解答は、ご覧のように、すぐに正しい答えになっています。
If at any point you're wondering if I completed the OpenAI ChatGPT prompt engineering course the answer is yes but it didn't inform too much of my thinking.
OpenAI ChatGPTプロンプトエンジニアリングコースを受講したかというと、答えは「はい」ですが、私の考え方に大きな影響を与えるものではありませんでした。
It was more for beginners and I had already factored in things like giving the model time to think and writing clear instructions.
このコースは初心者向けで、モデルに考える時間を与えたり、明確な指示を書いたりすることは、すでに織り込み済みでした。
The benchmark that I chose to test smart GPT on was the famous MMLU massive multitask language understanding benchmark.
smart GPTをテストするために選んだベンチマークは、有名なMMLUという大規模マルチタスク言語理解ベンチマークでした。
As you can see the state of the art is indeed GPT-4 with 86.4 accuracy and you know OpenAI think it's a big deal because it's the benchmark mentioned on the front page of their technical report.
ここで分かるように、最先端の技術は確かに86.4%の精度を持つGPT-4であり、技術報告書の表紙に言及されているベンチマークだとOpenAIは考えているので、それは大きな取り組みです。
Without boring you too much I extracted the questions from the test set of the MMLU data file and I didn't pick the topics at random.
あまり退屈させないように、MMLUのデータファイルのテストセットから問題を抽出しましたし、トピックも適当に選んだわけではありません。
I went for those that I thought GPT-4 would find the hardest.
GPT-4が最も難しいと思われるトピックを選びました。
Delving into the original MMLU paper you can see that GPT-3 found formal logic the hardest scoring just over 25 percent which is random chance.
MMLUのオリジナル論文を読んでみると、GPT-3は形式論理が最も難しく、25%強のスコアを出していることがわかりますが、これは偶然です。
It's a four question multiple choice test so around 25 or 30 percent is pretty bad and notice they helped out GPT-3 here.
4問の選択式テストですから、25~30%程度というのはかなり悪いですね。
They did it few shot meaning they gave it five successful examples before asking it a new question.
GPT-3には、新しい問題を出す前に、5つの成功例を提示する「数撃ちゃ当たる」方式を採用しました。
It's the same thing they did with GPT-4 they did it five shot but just before I show you the results there are three things I want to mention here.
GPT-4も同じように5問出題していますが、結果をお見せする前に3つのことをお伝えします。
First I was curious how smart GPT would do without any help zero shot.
まず、GPTがゼロショットでどの程度スマートなのか興味がありました。
Second I wanted to do it zero shot because people using GPT-4 don't typically give five successful examples before asking GPT-4 a question.
GPT-4を使っている人は、GPT-4に質問する前に5つの成功例を提示することは通常ありませんから、ゼロショットでやってみたかったのです。
They just want code or a quiz or a poem or an example.
彼らは、コードやクイズや詩や例が欲しいだけなのです。
They don't often provide five brilliant examples of code before asking their question.
質問する前に5つの素晴らしいコードの例を提示することはあまりありません。
And third if I can prove it works zero shot then of course future refinements can be made to push the results even further.
そして3つ目は、ゼロショットでうまくいくことが証明できれば、もちろん将来的に改良を加えて、さらに成果を上げることができる。
And here are the results from the first 25 questions from the formal logic test set of the MMLU.
そして、これがMMLUの形式論理テストセットの最初の25問の結果です。
I did many more tests after this but you can see from this set if you just ask the question you get a lower overall accuracy.
この後もいろいろなテストを行いましたが、このセットでは、ただ問題を出すだけでは全体の精度が低くなってしまうことがおわかりいただけるでしょう。
But of course 68 percent for GPT-4 is still a huge improvement over GPT-3s around 25 percent.
しかし、GPT-4の68%は、GPT-3の約25%に比べ、大きな進歩であることは間違いありません。
What happens when you add let's think step by step which as we know now isn't the fully optimized chain of thought prompt.
さらに、「ステップバイステップで考えよう」を追加するとどうなるでしょうか。
Well on average you get around 74 75 percent.
平均すると、74~75%程度になります。
That was 75 examples inputted manually and I still have all the tabs open.
これは手動で入力した75の例ですが、私はまだすべてのタブを開いています。
I'm keeping them open because I'm compiling a spreadsheet with the actual outputs.
タブを開いたままにしているのは、実際の出力結果をスプレッドシートにまとめるためです。
But what did the resolver get drawing upon GPT-4's ability to reflect and engage in dialogue with itself?
しかし、GPT-4の「自分自身と対話する能力」に基づいて、リゾルバーが出した結果はどうだったでしょうか。
It got 84 percent.
それは84%でした。
Now notice something about that number.
この数字に注目してください。
GPT-4 zero shot got 32 percent of the questions wrong.
GPT-4のゼロショットでは、32パーセントの問題が間違っていた。
That was halved to 16 percent after putting it through the smart GPT system.
それが、スマートGPTのシステムを通すと16%に半減する。
There was one question where the resolver model gave both a correct and incorrect answer but I'm counting that as an incorrect answer for the purposes of this test.
リゾルバーモデルで正解と不正解の両方が出た問題が1問ありましたが、このテストでは不正解としてカウントしています。
Anyway from 32 percent to 16 percent incorrect.
とにかく32パーセントから16パーセントの不正解になりました。
That is a pattern that stayed consistent throughout all my testing.
このパターンは、私が行ったすべてのテストを通じて一貫していました。
That approximately half of the errors that GPT-4 makes can be rectified if you give it the optimized step by step prompt.
つまり、GPT-4の誤答の約半分は、最適化されたステップバイステップのプロンプトを与えれば修正できるのです。
Get it to reflect on its results and get it to engage in dialogue and decide on a final answer.
GPT-4に結果を振り返らせ、対話をさせ、最終的な答えを決めさせるのです。
At this point for those people losing track of all the details I want to put into context what resolving half of the errors on MMLU might mean in the context of the big picture.
ここで、詳細がわからなくなった人のために、MMLUのエラーの半分を解決することが、全体像の中でどのような意味を持つかを説明したいと思います。
Here's Lennart Heim, an AI governance researcher, suggesting a score of 95 percent on the MMLU would be reflective of AGI-like abilities.
AIガバナンスの研究者であるレナート・ハイムは、MMLUのスコアが95%であれば、AGIのような能力があると指摘しています。
I do think I have like a 50 percent chance like within the next 20 years or so there might be something what we might call an AGI or transformative AI.
私は、今後20年ほどの間に、AGIやトランスフォーマティブAIと呼ばれるようなものが登場する可能性が50%ほどあると思います。
What do I mean by this?
これはどういう意味ですか?
Well maybe can measure it on benchmarks.
ベンチマークで測れるかもしれませんね。
There's like this famous MMLU benchmark like yeah there's something which like scores like 95 percent on this.
有名なMMLUベンチマークがありますが、このベンチマークで95パーセントのスコアを出すものがあります。
Going back to the results, if a smart GPT-like system can automatically resolve half of the errors that GPT-4 makes on the MMLU, that would increase its score from around 86.4 percent to around 93 percent, which is not far off 95 percent.
結果に戻ってみると、賢いGPTのようなシステムがMMLUでGPT-4が犯すエラーの半分を自動的に解決できるとすれば、そのスコアはおおよそ86.4%から93%に上がり、95%に非常に近づくでしょう。
Remember his prediction was a 50 percent chance in 20 years.
彼の予測は、20年後に50%の確率であったことを忘れないでください。
I'm talking about GPT-4 now.
私は今、GPT-4の話をしているのです。
For those who are still skeptical I'm going to show you plenty more results now and then walk through the papers that give the theory as to why this works.
まだ懐疑的な方のために、これからたくさんの結果をお見せし、なぜこれが効くのか、その理論について書かれた論文を紹介します。
One thing that I forgot to mention earlier is that the human expert level on the MMLU is 89.8 percent, and that's taking the 95th percentile of human test takers.
先ほど言い忘れたのですが、MMLUのエキスパートレベルは89.8%で、これは受験者の95パーセンタイルをとったものです。
Remember, those are domain experts in each of the subtopics.
このテストは、各サブトピックの専門家によるものです。
What we're doing is testing GPT-4 or smart GPT on all of the topics simultaneously.
私たちが行っているのは、GPT-4やスマートGPTを、すべてのトピックについて同時にテストすることです。
So even if smart GPT-like systems can't quite reach 95 percent and I think honestly they'll get pretty close with all the refinements that I'm going to suggest.
ですから、スマートGPTのようなシステムが95%に達しないとしても、これから提案する改良を加えれば、正直なところ、かなり近づくと思われます。
I think they should almost certainly be 89.8 percent which is the human expert test taker level.
その結果、89.8%という人間の熟練受験者レベルに達することは間違いないでしょう。
Intrigued by these results I then put it through the college math test from the MMLU and remember this was before using the optimized version of the step-by-step prompt.
この結果に興味を持った私は、MMLUの大学数学のテストにこのプログラムを適用しました。
Obviously I'm not going to go through all the questions here but let's skip to the final results.
もちろん、ここですべての問題に目を通すつもりはありませんが、最終的な結果まで見てみましょう。
We have zero shot accuracy six out of 15 which is 40 percent.
15問中6問がゼロショットで、これは40%にあたります。
The average when you add let's think step-by-step was 53.5 percent and then the final output of the resolver model had a 60 percent accuracy.
ステップバイステップを加えた平均値は53.5%で、リゾルバーモデルの最終出力は60%の精度でした。
So it couldn't quite resolve half of the errors but the overall pattern held up.
つまり、エラーの半分を解決することはできませんでしたが、全体的なパターンは維持されたのです。
In case anyone is wondering about methodology I kept the formatting identical for every question.
方法論について疑問に思う人がいるかもしれませんが、私はすべての問題で同じ書式を使い続けました。
I always opened a new tab for each question it wasn't looking at the context of what it had already put out.
各問題で常に新しいタブを開き、すでに出した文脈を見ることはありませんでした。
Each attempt was fresh aside from the resolver model which looked at the context of the researcher's output.
研究者の出力の文脈を見るリゾルバーモデルを除けば、それぞれの試みは新鮮でした。
And again as you can see from example 14 it wasn't like the researcher could always spot the errors or that the resolver could always pick the right option.
また、例14からわかるように、リサーチャーが常にエラーを発見できるわけでも、リゾルバーが常に正しい選択肢を選べるわけでもありません。
Sometimes the let's think step-by-step prompt gave the right output but the resolver couldn't quite distinguish it.
ステップバイステップで考えよう」プロンプトが正しいアウトプットを出しても、リゾルバーがそれを見分けられないことがありました。
The optimized prompt gets a slightly better output and upon reflection the researcher can sometimes but not always spot the errors of those outputs.
最適化されたプロンプトでは、少し良い出力が得られ、よく考えてみると、研究者はその出力の間違いに気づくことができることもあるが、いつもそうとは限らない。
And sometimes but not always the resolver can spot based on those flaws which answer is best.
そして、リゾルバーはその欠点に基づいて、どの答えがベストかを見分けることができる場合もありますが、常にそうとは限りません。
These are incremental improvements sometimes gbt4 simply can't get it right.
このように、gbt4ではうまくいかないこともありますが、少しずつ改善されています。
I have noticed a few themes in those questions.
私は、これらの質問にいくつかのテーマがあることに気づきました。
Anytime it comes to division, multiplication, characters or counting in general gbt4 tends to make mistakes that neither the researcher nor resolver can spot.
除算、乗算、文字、または一般的な数え方に関して、gbt4は研究者にも解決者にも見つけられないようなミスをする傾向があります。
Of course integrating a few tools via API would likely solve those issues.
もちろん、APIを介していくつかのツールを統合することで、これらの問題を解決できる可能性があります。
I don't want to preempt the conclusion too much, but I believe a smart GBT-like system with tools integrated could probably score around 95% right now on the MMLU, especially if it was helped out with few-shot prompting.
あまり結論を先取りしたくはないのですが、ツールを統合したスマートなGBTのようなシステムは、特に数発のプロンプトで助けられた場合、おそらくMMLUで今すぐ95%程度のスコアを出せるのではないかと思います。
To add weight to that preliminary conclusion I tested it on certain topics and had to stop because it simply got the questions right every single time.
この予備的な結論に重みを持たせるために、あるトピックでテストしてみたのですが、単純に毎回問題を正解してしまうので、やめざるを得ませんでした。
For example high school psychology from the mmlu.
例えば、「mmlu」の「高校心理学」です。
I then tried prehistory which it also aced before finding machine learning where I got more interesting results.
その後、先史時代に挑戦してみましたが、こちらも正解でした。
Zooming in this time, the raw score was 65%, the chain of thought let's think step-by-step average was 71.6%, and the resolver model got 80%. Let's now look a little deeper into why all of these steps might improve the end result.
今回の焦点を当てると、生のスコアは65%であり、思考の連鎖の平均は71.6%であり、リゾルバモデルは80%を獲得しました。これらのすべてのステップが最終結果を改善する理由をもう少し詳しく見てみましょう。
In reply to the original let's think step-by-step paper which was published around a year ago, Andrei Karpathy said this.
1年ほど前に発表されたlet's think step-by-stepのオリジナル論文への返信で、Andrei Karpathyはこのように語っています。
Adding something like let's think step-by-step to the prompt is a way of using the input space for computation that you'd normally want in the hidden state of the model.
プロンプトにlet's think step-by-stepのようなものを追加することは、通常、モデルの隠れた状態に欲しい計算のための入力空間を使う方法である。
Instead of the workings out being done in the activations of the neural network it's done in the discrete tokens of that input space and he adds did not super see this coming.
ニューラルネットワークの活性化ではなく、入力空間の離散的なトークンで計算を行うのです」と彼は付け加え、「このようなことが起こるとは思ってもみなかった」と語った。
And here is the paper released three days ago that improves upon that original prompt.
そして、これが3日前に発表された論文で、このオリジナルのプロンプトを改良したものです。
They also did their testing zero shot like me and they tested many prompts starting like I did with just direct prompting, just asking the question like 99% of users would do of GPT-4.
彼らも私と同様にゼロショットでテストを行い、まずは直接的なプロンプトから始めて、GPT-4を使う99%のユーザーが行うように質問をするだけで、多くのプロンプトをテストしました。
And then they tried like me the well-established let's think step-by-step prompt.
そして、私同様、定評のある「ステップバイステップで考えよう」というプロンプトも試しました。
They also iteratively tested seven original prompts as well as the prompt that I've now integrated into smart GPT, the let's work this out in a step-by-step way etc.
さらに、7つのオリジナルプロンプトと、私が今スマートGPTに組み込んだプロンプト、ステップバイステップで解決しましょうなどを繰り返しテストしてくれました。
They share my opinion that zero shot prompting setups have the benefit of not requiring such task-dependent selection of exemplars.
彼らは、ゼロショットプロンプトのセットアップには、タスクに依存した模範解答の選択を必要としないという利点がある、という私の意見に共感しています。
You don't have to find correct examples it just does it all for you.
正しい例を探す必要がなく、すべてやってくれるのです。
Here are the end results for GPT-4 that we saw earlier showing the difference between asking directly your question and using these refined prompts.
先ほどのGPT-4の結果ですが、直接質問する場合と、このように洗練されたプロンプトを使う場合の違いがよくわかりますね。
Notice that this technique is somewhat model dependent and it doesn't have the same effect on smaller or weaker models.
このテクニックはモデルに依存するところがあり、小さいモデルや弱いモデルでは同じ効果が得られないことに注意してください。
Before we move on to the next paper there is one somewhat failed prompt that I want to pick up on.
次の論文に移る前に、1つだけ失敗したプロンプトを取り上げたいと思います。
It's this self-critique prompt where they ask answer the question then critique the answer based on the critique reconsider the other answer options and give a single final answer.
それは自己批評プロンプトで、質問に答え、その答えを批評し、批評に基づいて他の答えの選択肢を再考し、最終的に1つの答えを出すというものです。
And you might wonder why didn't that prompt perform best when we know that reflection and dialogue can work.
内省と対話が有効であることが分かっているのに、なぜこのプロンプトは最高のパフォーマンスを発揮しなかったのだろうかと思うかもしれません。
My theory is because it's trying to do all of it in one prompt.
私の考えでは、このプロンプトは1つのプロンプトですべてを行おうとしているからです。
Through my hundreds of experiments I've noticed that GPT-4 can only handle so much in one go.
何百回もの実験を通じて、GPT-4は一度に処理できる量が限られていることに気づきました。
It simply gets overwhelmed or confused if you ask it to do too much in one prompt.
1回のプロンプトで多くのことを要求すると、圧倒されたり混乱したりするのです。
That's why I broke my model into stages to allow it to show off each of its abilities one by one.
そこで、GPT-4の能力を1つ1つ発揮できるように、モデルを段階的に分割しています。
And before we get to the other papers what's my personal theory as to why this eliminates up to half of the errors that GPT-4 makes.
他の論文の前に、なぜGPT-4が犯すエラーの半分まで排除できるのか、私の持論を述べます。
Well my guess is this.
私の推測では、こうです。
Remember that GPT-4 is drawing on a vast data set of internet text.
GPT-4は、インターネット上の膨大なテキストデータを利用していることを思い出してください。
And let me ask you what kind of text has things like question, answer, let's work this out.
そして、どんなテキストかというと、question, answer, let's work out this outのようなものがある。
Be sure we have the right answer.
正しい答えがあることを確認する。
The kind of data that would have that text would be things like tutorials or expert breakdowns.
そのようなテキストを持つデータとは、チュートリアルや専門家の解説のようなものでしょう。
So I believe you're triggering more of the weights inside GPT-4 that relate to things like expert tutorials.
そのため、GPT-4では、専門家のチュートリアルのようなものに関連するウェイトをより多くトリガーしているのだと思います。
And so inevitably you're getting slightly better answers.
そうすると、必然的に少し良い答えが得られることになります。
Next I've already explained why you get different outputs when you give the exact same prompt.
次に、まったく同じ答えを出したのに、なぜ違う答えが返ってくるのか、その理由を説明しました。
That's down to sampling and the temperature of the model.
それは、サンプリングとモデルの温度によるものです。
But to simplify massively sometimes GPT-4 will give you an output that it knows isn't the most probable.
しかし、非常に単純化すると、GPT-4は、最も確率が高くないとわかっている出力を出すことがあります。
It introduces some randomness into its sampling.
これはサンプリングにランダム性を導入しているのです。
By generating multiple outputs you're getting a larger sample size reflecting the full range of probabilities that GPT-4 ascribes to its outputs.
複数の出力を生成することで、GPT-4が出力に与える確率の全範囲を反映した、より大きなサンプルサイズを得ることができるのです。
You're reducing a little bit some of the randomness that's inherent in GPT-4 outputs.
GPT-4の出力に内在するランダム性を少し軽減することができるのです。
Next I believe that GPT-4 can sometimes spot its own errors through reflection because prompting like this triggers a different set of weights.
次に、GPT-4は、このようなプロンプトを出すことで、別の重みを持つようになるため、内省によって自らの誤りに気づくことがあると思います。
You could almost think of it as a different mindset.
これは、別の考え方と言えるかもしれません。
One more focused on finding errors.
間違いを見つけることに集中するのです。
Again if the question is too hard or involves counting, characters, division, multiplication as I said earlier this won't help.
先ほど申し上げたように、問題が難しすぎたり、数え方、文字、割り算、掛け算が含まれる場合は、この方法は役に立ちません。
But a percentage of the time it can spot its own errors and point them out.
しかし、問題の何割かは、自分自身の間違いを発見し、それを指摘することができます。
Notice this is a separate bit of inference not lumped into the original prompt.
これは、元のプロンプトに含まれるのではなく、別の推論であることに注意してください。
And when it does successfully point out the errors it can often engage in this dialogue with itself.
そして、うまく間違いを指摘できたときには、しばしば自分自身と対話することができるのです。
Notice in a meta kind of way I'm using the step-by-step prompting to improve the reflection and dialogue.
メタ的な言い方をすれば、ステップバイステップのプロンプトを使うことで、内省と対話を向上させることができるのです。
So those are my theories as to why it works but at the end of the video I'm going to show you at least five ways I think the model can be further refined.
以上が、このモデルがうまく機能する理由についての私の理論ですが、ビデオの最後には、このモデルをさらに改良する方法を少なくとも5つ紹介するつもりです。
Before we do though I looked up the paper by Zhou which produced that prompt that did the best in the previous paper.
しかし、その前に、前の論文で最も良い結果を出したプロンプトを生み出したZhouの論文を調べておきました。
They came to that special prompt through automatic prompt engineering but there's something interesting I want to point out though.
彼らは自動プロンプトエンジニアリングによってあの特別なプロンプトを作り出したのですが、面白いことがあるので指摘したいと思います。
On page seven they say we use automatic prompt engineering to find a prompt starting with let's that maximizes the likelihood of correct reasoning steps.
7ページ目に「自動プロンプトエンジニアリングで、推論ステップが正しくなる可能性を最大化するlet'sで始まるプロンプトを見つける」とあります。
Then they found the best one that I integrated into smart GPT.
そして、その中から最適なものを見つけて、smart GPTに組み込んでいます。
Let's work this out in a step-by-step way to be sure we have the right answer.
正しい答えが出るように、ステップバイステップで解決していこう。
That's the one I want you to use.
それが使ってほしいものです。
And they ran their own benchmarks and of course it did improve the scores.
そして、彼らは自分たちでベンチマークを実施し、もちろんスコアは向上しました。
But the interesting thing to me is they started with let's each time.
しかし、興味深いのは、彼らは毎回Let'sから始めていることです。
So even that first stage for the model might not yet be fully optimized.
つまり、モデルの最初の段階でさえ、まだ完全に最適化されていない可能性があるのです。
Maybe there's a prompt that doesn't begin with let's that improves this initial result still further.
もしかしたら、let'sで始まらないプロンプトがあれば、この最初の結果をさらに向上させることができるかもしれません。
Anyway back to the papers.
とにかく、論文に話を戻します。
I know many people watching this will wonder if I read the paper boosting theory of mind performance in large language models via prompting.
この記事を見ている多くの人は、「プロンプトによって大規模言語モデルの心の理論のパフォーマンスを向上させる」という論文を読んだのだろうかと思うだろう。
And yes I did because they tested something similar for a theory of mind test.
そうです、「心の理論」のテストに似たようなものを使ったからです。
Using similar techniques, they were able to get theory of mind accuracy for GPT-4 from 80% to 100%. They conclude that these results demonstrate that appropriate prompting enhances large language model theory of mind reasoning, and they underscore the context-dependent nature of these models' cognitive capacities.
同様の手法を使用することで、彼らはGPT-4の理論精度を80%から100%に向上させることができました。彼らは、適切なプロンプトが大規模な言語モデルの心の理論推論を向上させることを示す結果であり、これらのモデルの認知能力が状況依存であることを強調しています。
They use that original prompt let's think step by step along with some few shot examples.
彼らは、「Let's think step by step」というオリジナルのプロンプトを、いくつかの例とともに使っています。
Take a look at the GPT-4 table and you can see how the let's think step by step improved the results dramatically.
GPT-4の表を見ていただければ、「考えよう」のステップバイステップがいかに結果を劇的に向上させたかがわかると思います。
And as I theorized earlier adding few shot examples would push this still further.
そして、先ほど私が理論的に説明したように、数発の例を加えることで、さらに効果を高めることができます。
This is part of why I think that 95% barrier on the MMLU will be broken probably this year by GPT-4.
MMLUの95%の壁は、GPT-4で今年中に破られるだろうと思うのは、このためでもあるのです。
A few other points from this paper.
この論文の他のポイントをいくつか紹介します。
They admit that there is not currently a theoretical understanding of why these prompting techniques are beneficial.
なぜこのようなプロンプトが有効なのか、今のところ理論的な理解が得られていないことを認めています。
I've given you my theory and Karpathy's but no one quite knows for sure.
私の説とカルパシーの説を紹介したが、誰も確かなことは分かっていないのである。
Lastly from this paper and I found this really interesting.
最後にこの論文から、私はこれがとても興味深いと思いました。
Giving it generic few shot prompts that weren't directly theory of mind actually improved the outputs slightly more than giving it direct theory of mind examples.
直接的に心の理論の例を与えるよりも、直接的に心の理論ではない一般的なプロンプトを数回与える方が、実際に出力がわずかに向上したのです。
This opens the door to the first of the five ways I anticipate smart GPT getting even smarter.
これは、私が予想する賢いGPTがさらに賢くなる5つの方法のうち、最初の方法への扉を開くものです。
It could be possible to come up with generic few shot prompts that could be automatically integrated into the model that don't necessarily relate to the topic at hand.
それは、必ずしもトピックに関係ない、一般的な数発のプロンプトをモデルに自動的に組み込むことができるかもしれないということです。
This graph shows the impact of adding few shot examples to GPT-3 and if this can be done in a generic way for GPT-4 results could be improved still further.
このグラフは、GPT-3に数撃ちゃ当たるの例を追加した場合の影響を示していますが、これをGPT-4でも汎用的に行うことができれば、さらに結果を向上させることができるでしょう。
Next the boosting theory of mind paper speculates that integrating some of these approaches could boost the performance of weaker models to beyond the levels of GPT-4 zero shot accuracy.
次に、「ブースティング理論」の論文では、これらのアプローチを統合することで、弱いモデルの性能をGPT-4のゼロショット精度を超えるレベルまで高めることができると推測しています。
Next here is the original DERA paper that inspired me to have the researcher and resolver dialogue at the end of smart GPT.
次に、スマートGPTの最後に研究者と解決者の対話を行うきっかけとなったDERAの原著論文を紹介します。
As they say, the DERA approach shows significant improvement over base GPT-4 performance, and these were open-ended questions by the way, not multiple choice, so this is more generally applicable than you might think.
DERAのアプローチは、GPT-4のベースとなる性能よりも有意な改善を示しています。ちなみに、これは多肢選択式ではなく、自由形式の質問でしたので、皆さんが思っているよりも一般的に適用可能です。
You can see from this table how results improved after engaging in this dialogue, and that brings me to the second way I anticipate smart GPT getting smarter in the future: a longer and more rich dialogue.
この表から、このような対話を行った後に結果がどのように改善されたかがわかります。そして、これが私が将来、賢いGPTがさらに賢くなると予想する2つ目の方法です:より長く、より豊かな対話を行うことです。
At the moment we have this simple researcher and resolver two-step dialogue.
今のところ、研究者と解決者というシンプルな2ステップの対話が行われています。
I can imagine a council of advisors; you can imagine a mathematician chipping in, a philosopher, and a professor, each one tapping into slightly different weights of GPT-4, extracting more hidden expertise.
私はアドバイザーの評議会を想像することができます。数学者、哲学者、教授が参加し、GPT-4のわずかに異なる重みにアクセスして、さらに隠された専門知識を引き出しているのです。
I'm not saying that would transform the results but it might edge them another few percent higher.
そうすれば、結果が変わるとは言いませんが、さらに数パーセント高くなる可能性があります。
Next even with longer dialogues and different experts we could find ways of optimizing these prompts just like we did with the original let's think step by step.
次に、より長い対話とさまざまな専門家を使うことで、「ステップバイステップで考えよう」と同じように、プロンプトを最適化する方法を見つけることができます。
That's the third avenue of improvement that I envisage because I came up with these prompts I'm sure they could be improved.
これが、私が考える3つ目の改善の道です。私が考えたプロンプトですから、きっと改善できるはずです。
Next we could experiment with different temperatures.
次に、温度を変えて実験してみましょう。
Remember a lower temperature makes the model more conservative a higher one towards one makes it more creative.
温度が低いとモデルはより保守的になり、高いとよりクリエイティブになることを忘れないでください。
We could experiment with a higher temperature to produce a more diverse range of outputs at this stage and then perhaps a more conservative deterministic temperature for the final judge or resolver.
この段階では、より多様なアウトプットを生み出すために高い温度で実験し、最終的なジャッジやリゾルバーでは、より保守的な決定論的温度で実験することができます。
It might not work but it's worth trying.
うまくいかないかもしれませんが、試してみる価値はあると思います。
And the fifth improvement I know would work integrating APIs for character counting calculators code interpreters etc.
そして、5つ目の改善は、文字カウント計算機やコードインタプリタなどのAPIを統合することです。
Spending these weeks manually sorting through the outputs of GPT-4 on these benchmarks, I can really see where it goes wrong.
この数週間、これらのベンチマークでGPT-4の出力を手作業で整理していると、どこで間違っているのかがよくわかります。
It's often by getting letters in the wrong order or making mistakes with division; it gets the high-level logic right and then makes quite simple errors.
文字の順番を間違えたり、割り算を間違えたり、高レベルのロジックは正しくても、ごく単純なミスをしていることが多いのです。
Basic tool integration would I am sure push the results still higher.
基本的なツールの統合を行えば、さらに高い結果が得られると確信しています。
Now I know this isn't my usual video and trust me I have been following the AI news and we'll get back to that very soon.
これは私のいつものビデオではありませんが、私はAIのニュースを追っています。
I'm determined to make those improvements and push smart GPT even further but of course that will be aided massively by getting access to the plugins and the GPT-4 API key.
もちろん、プラグインとGPT-4のAPIキーを入手することで、さらにスマートGPTを進化させることができます。
So far I've had to do all of this manually which was a lot of work.
これまでのところ、私はこのすべてを手作業で行う必要があり、それは大変な作業でした。
Now as you saw earlier I have drawn on GPT-4 to help me develop a program in replit to automate this process but at the moment it's GPT-3.5 and honestly the context window really limits the ability.
先ほど見ていただいたように、GPT-4を利用して、このプロセスを自動化するためのプログラムをreplitで開発したのですが、今のところGPT-3.5で、正直なところコンテキストウィンドウが機能を制限しています。
But I do look forward to the day when I can integrate GPT-4 and put this out as an automatic model for people to test and play about with.
しかし、GPT-4を統合し、自動化されたモデルとして公開し、人々がテストしたり遊んだりできるようになる日が来ることを楽しみにしています。
I'm sure that something similar will ultimately be incorporated by OpenAI itself maybe as a thoughtful mode or smart mode a bit like Bing has creative precise balanced etc.
Bingがクリエイティブ・プレシジョン・バランスなどを備えているように、最終的にはOpenAI自身が、思慮深いモードやスマートモードとして、同様のものを取り入れることになると思います。
Each response does take longer but as you've seen the outputs are noticeably better.
それぞれの反応には時間がかかりますが、ご覧のように出力は明らかに良くなっています。
If the results of models like this one do officially exceed the 86.4% that OpenAI talked about in the GPT-4 technical report I do think that would reveal quite a few things.
このようなモデルの結果が、GPT-4のテクニカルレポートでOpenAIが話していた86.4%を公式に上回るとしたら、それはかなり多くのことを明らかにすると思う。
First the OpenAI isn't even aware of the full capabilities of its own model.
まず、OpenAIは自分たちのモデルの全能力を認識していない。
I don't even know if they anticipated things like AutoGPT.
AutoGPTのようなものを想定していたかどうかもわかりません。
I do think it would reveal that they need to do far more proper testing of their models before they release them.
しかし、モデルをリリースする前に、もっと適切なテストを行う必要があることは明らかでしょう。
They should make falsifiable predictions about what their models won't be capable of.
自分たちのモデルには何ができないかについて、反証可能な予測を立てるべきです。
That way we would know just how much they know about their own models.
そうすれば、彼らが自分たちのモデルについてどれだけのことを知っているのかがわかるでしょう。
What we're trying to avoid is a situation where OpenAI says their model can only achieve x, and then when they release the model in the wild, someone comes along and achieves y, where y is much more impactful than x.
私たちが避けたいのは、OpenAIが自分たちのモデルはxしか達成できないと言いながら、そのモデルを野に放つと、誰かがやってきてyを達成し、yはxよりもはるかにインパクトがある、というような状況なのです。
So those were the goals of this video to show you how to get more out of GPT-4 to run you through some of the fascinating papers that have been released in the last few days and weeks.
このビデオの目的は、GPT-4からより多くの成果を得る方法を紹介し、ここ数日、数週間に発表された魅力的な論文のいくつかを見ていただくことでした。
The third goal was to show you what this model could do with some official benchmarks and suggest ways it might get better in the near term future.
3つ目の目標は、公式ベンチマークでこのモデルができることを紹介し、近い将来もっと良くなる可能性がある方法を提案することでした。
Of course if you have a GPT-4 API key or are an expert in benchmarking systems like GPT-4 I'd love to hear from you.
もちろん、GPT-4のAPIキーをお持ちの方、GPT-4のようなシステムのベンチマークに詳しい方、ぜひご連絡ください。
I guess the final goal was to perhaps suggest to you that OpenAI don't know as much about their own models as they might lead you to believe.
最終的なゴールは、OpenAIが自分たちのモデルについて、皆さんが思っているほど知らないということを示唆することだったのではないでしょうか。
Thank you so much for watching to the end and have a wonderful day.
最後までご覧いただき、ありがとうございました!素敵な一日をお過ごしください。
この記事が気に入ったらサポートをしてみませんか?