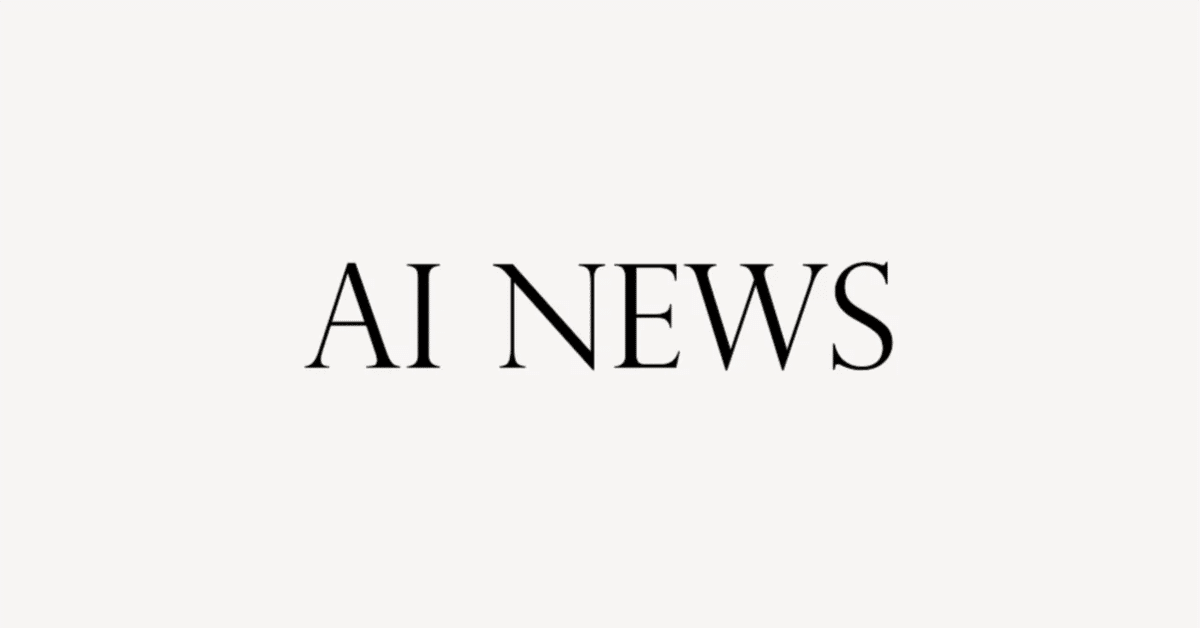
【ウェス・ロスのAIニュース】英語解説を日本語で読む【2024年2月22日|@Wes Roth】
この週、OpenAIの新しいビデオ生成モデル「Sora」に関する話題が注目を集めましたが、その背景では他にも驚くべきAIの進展がありました。イーロン・マスク氏はXのライブスペースでお話しになり、AGI(汎用人工知能)と私たちが仮想世界に生きている可能性について述べられました。彼は、AGIが新しい物理法則を提案できる可能性があると提案し、これはシミュレーション説を支持する証拠となるかもしれません。さらに、AIが現実世界のシミュレーションで訓練され、それが現実世界に適用できるかもしれないというアイデアも議論されました。NVIDIAの研究者は、AIが異なる現実を横断できる「基盤エージェント」の概念を紹介しました。一方、Googleは新しいオープンモデル「Gemma」をリリースし、スタンフォード医学は性別による脳の構造差をAIで識別する研究を発表しました。最後に、AIハードウェアスタートアップGroqは、高速かつ低コストのAIチップを開発し、AIの進化に新たな可能性を示唆しました。
公開日:2024年2月22日
※動画を再生してから読むのがオススメです。
So, this whole week, we've been talking about Sora, OpenAI's new mind-blowing video generation model, but there's been tons of other insane AI news happening behind the scenes.
今週全体を通じて、OpenAIの新しい驚くべきビデオ生成モデルであるSoraについて話してきましたが、裏で起こっている狂気のAIニュースがたくさんありました。
But first, let's start with this.
しかし最初に、これから始めましょう。
Elon Musk jumps on Twitter/X in a live spaces conversation, and here Elon Musk explains the intersection of AGI and potentially seeing if it might help us figure out if we do indeed live in a simulation or not.
イーロン・マスクがTwitter/Xでライブスペースの会話に参加し、ここでイーロン・マスクがAGIと交差することを説明し、我々が実際にシミュレーションの中に生きているかどうかを見極めるのに役立つかもしれないと述べています。
And he does it all while I think one of his kids is trying to climb on top of his head.
そして、その間、彼の子供の一人が彼の頭の上に登ろうとしていると思います。
So, I'm wondering if we could propose another Turing test, a different definition of AGI, which would be actually coming up with new laws of physics or new complete paradigms of physics rather than just physics as a base layer of reality.
私はもう一つのチューリング・テストを提案できるかどうか疑問に思っています。つまり、AGIの異なる定義であり、物理学の新しい法則や物理学の基本的な現実のレイヤーではなく、新しい完全な物理学のパラダイムを実際に考え出すことです。
I mean, you always quote that, right?
つまり、あなたはいつもそれを引用しているんですよね?
Well, I think you can certainly have AI would think it's a, would not realize it's a simulation.
まあ、確かにAIは自分がシミュレーションであるとは気づかないと思います。
Which may be the case for us right now.
それが現在の私たちに当てはまるかもしれません。
And that would have a a true physics engine and thus experience sensations in the same way.
そして、それは真の物理エンジンを持ち、したがって同じように感覚を経験するでしょう。
The simulation style process does explain some elements of quantum mechanics, such as only collapsing the probability distribution when you look at something.
シミュレーションスタイルのプロセスは、何かを見るときに確率分布が崩壊するという、量子力学のいくつかの要素を説明します。
Like, why would something be only true when you look at it?
例えば、何故何かが見られた時だけ真実になるのでしょうか?
Well, if it's rendering in real time, then that's actually how a video game works.
もしリアルタイムでレンダリングされているなら、それが実際にビデオゲームが動作する方法です。
Like, let's say you're in World of Warcraft or something and you walk through a forest and a rat appears.
例えば、あなたがWorld of Warcraftなどにいて、森を歩いていてネズミが現れたとします。
But before that, was there a rat or not a rat?
しかし、その前に、ネズミはいたのかいなかったのか?
There was only a probability of a rat and the rat only became real when you, when you looked in that direction, that collapsed probability space and the rat appeared.
ネズミの確率しかなく、ネズミはあなたがその方向を見たときにのみ現実のものとなり、確率空間が崩壊し、ネズミが現れました。
So, so I think version theory actually explains a lot of things that seem quite mysterious to sort of the Schrödinger's cat situation.
私は、バージョン理論が実際にはシュレーディンガーの猫の状況のようにかなり神秘的に思える多くのことを説明していると思います。
The universe may seem infinite to us, but frankly, if I was creating a simulation of this reality, I would I would put the stars far enough away that we do not have to simulate the details of the planets.
宇宙は私たちには無限に見えるかもしれませんが、率直に言って、もしこの現実のシミュレーションを作成するなら、私は星を十分に遠くに配置するでしょう。私たちが惑星の詳細をシミュレートする必要がないように。
And in fact, that is the situation.
実際、それがその状況です。
So you really just have to simulate with high fidelity what is observed on our planet.
本当に私たちの惑星で観察されるものを高い忠実度でシミュレートする必要があります。
And much easier task than twice as low reality.
それは2倍低い現実よりもはるかに簡単なタスクです。
And I was joking that when the James Webb telescope went off, that maybe the reason for the delays was that the simulators needed to bring more computers online because now that we can see further, they needed to improve the fidelity of their simulation.
私はジェームズ・ウェッブ望遠鏡が発射されたときに冗談を言っていました。おそらく遅れの理由は、シミュレーターがより多くのコンピュータをオンラインにする必要があったからで、今、より遠くを見ることができるようになったので、彼らはシミュレーションの忠実度を向上させる必要があったのです。
So like the equivalent of Amazon Web Services or something.
まるでAmazon Web Servicesのようなものです。
There's this interesting back and forth between Elon Musk and Neil deGrasse Tyson.
イーロン・マスクとニール・ド・グラス・タイソンの間で興味深いやり取りがあります。
That's this guy right here.
これがこの人です。
So he's talking about idea that Elon Musk presented.
彼はイーロン・マスクが提示したアイデアについて話しています。
I saw it once at some forum when somebody asked him a question that the idea is basically this.
私はかつてフォーラムでそれを見たことがあります。誰かが彼に質問をしたとき、そのアイデアは基本的に次のようなものです。
So imagine that there is something called the base reality.
つまり、何かが存在すると想像してください。それが基本的な現実と呼ばれるものです。
This is the original physical universe.
これは元の物理的な宇宙です。
And eventually it gets enough scientific progress and computers and ability to do calculations to create their very own simulation of their own universe or something similar.
そして、最終的には、科学の進歩やコンピュータ、計算能力が十分になり、自分たち自身の宇宙やそれに類似したもののシミュレーションを作成することができます。
So they create it and this thing evolves until eventually it evolves far enough to create its own simulation of its own universe and down it goes forever.
そして、それを作成し、このものは進化して最終的には自分自身の宇宙のシミュレーションを作成するまで進化し、それが永遠に続いていきます。
So now that world evolves and they develop computing power.
その世界が進化し、計算能力を発展させるようになったのです。
Right.
はい。
And they say we want to play video games.
そして、彼らはビデオゲームをしたいと言います。
So now we're going to make a world.
だから、私たちは世界を作ることになります。
So then they make a world
そして、彼らは世界を作ります
And then they make a world all the way on down.
そして、下まで世界を作ります。
Right.
はい。
It could be hundreds, thousands, billions, infinite.
数百、数千、数十億、無限かもしれません。
Elon Musk's question was what was the chance that we are living in this base reality?
イーロン・マスクの質問は、私たちがこの基本の現実に生きている可能性は何%かということでした。
I mean, if you can imagine this thing stretching down forever, as Neil deGrasse Tyson explained it, basically the chances would be something one in a million, one in a trillion, one in some astronomically large number that we are indeed in the base reality.
私は、ニール・ド・グラス・タイソンが説明したように、このものが永遠に広がっていると想像できるなら、基本的には、私たちが実際に基本の現実にいる可能性は、100万分の1、1兆分の1、あるいは何か天文学的に大きな数の中の1つであるということです。
Okay.
OK。
So there's one in a zillion, you're the real universe and 999 zillion to one.
1つはゼロに近い、あなたが本当の宇宙であり、999ゼロに対して1つです。
That's your simulation.
それがあなたのシミュレーションです。
That convinced me.
私を納得させました。
And that was Elon Musk's point that the chance of us living in a non-simulated world is very low.
そして、それがイーロン・マスクの指摘で、非シミュレートされた世界に住んでいる可能性は非常に低いということです。
Right.
はい。
If you kind of follow this idea.
もしこの考えをある程度追っているなら。
And I don't want to be convinced.
そして私は説得されたくありません。
I didn't like it.
私はそれが好きではありませんでした。
But Neil said he did not like that idea.
しかし、ニールはその考えが好きではないと言いました。
And he actually spent a lot of time trying to come up with an explanation of why that was not the case.
そして、実際に彼はその理由を説明しようとするために多くの時間を費やしました。
And he did.
そして、彼は成功しました。
Basically the idea is this, that what all these universes, the simulated universes have in common is they're advanced enough to have a simulated universe kind of underneath them.
基本的に、この考え方は、これらすべての宇宙が共通して持っているのは、それらが進化してシミュレートされた宇宙を持っているということです。
In other words, somewhere in some lab, they have a computer running a simulation of the entire universe that's within that universe.
つまり、どこかの研究室で、その宇宙の中にある宇宙全体のシミュレーションを実行しているコンピュータがあるということです。
We right now, currently, as far as we know, we do not, which means either we are living at the very last sort of universe in this chain, the one that hasn't yet developed its own sub reality sub universe.
私たちは現在、私たちが知っている限り、そうではないので、それは私たちがこの連鎖の最後の宇宙に住んでいるか、まだ自分自身のサブリアリティサブユニバースを開発していない最後の宇宙であることを意味します。
So either that and we have multiple universes above us, or maybe just one, the original base layer, base reality, or we are base reality.
私たちは私たちの上に複数の宇宙があるかもしれません、またはたった1つ、元の基本層、基本現実、または私たちは基本現実です。
We are the sole universe.
私たちは唯一の宇宙です。
So if you think of it that way, the chances of us living in a simulated reality goes from one in bazillion, some large amount, like a very low chance to, I mean, it's 50:50.
もしもそう考えるなら、私たちがシミュレートされた現実の中に住んでいる可能性は、非常に低い確率から、つまり、50対50になります。
Right.
はい。
Either what the bottom of this long chain or we're in base reality 50:50.
この長い連鎖の底にいるか、または基本現実にいるか、50対50です。
Those are much better odds.
それらははるかに良い確率です。
Either we are the real one.
どちらかと言えば、私たちが本物です。
Right.
そうですね。
Or we're the one in the chain that's still evolving.
あるいは、私たちはまだ進化し続けている連鎖の中の1つです。
So the odds of us being a simulation goes from a gazillion to one, it flips 50:50.
ですから、シミュレーションである可能性は、1対数十億から、50対50に変わります。
Well, I'm good with that.
まあ、それでいいですね。
However, now we're rapidly approaching the time when we might be able to build entire world simulators.
ただし、今や私たちは世界全体のシミュレーターを構築できるかもしれない時期に急速に近づいています。
OpenAI is talking about it.
OpenAIがそのことを話しています。
NVIDIA is talking about it.
NVIDIAもそのことを話しています。
Here's Dr. Jim Fan, an AI researcher at NVIDIA, talking about how the ultimate sort of AI/robot would be a foundation agent that is able to sort of generalize across realities.
こちらはNVIDIAのAI研究者であるジム・ファン博士ですが、究極のAI/ロボットが、ある種の基盤エージェントであり、異なる現実を横断して一般化することができるように話しています。
They can be trained in a simulation that would translate to the real world.
彼らはシミュレーションで訓練され、それが現実世界に翻訳されることができます。
They would be able to take any form and shape.
彼らはどんな形や姿でも取ることができるでしょう。
They would sort of seamlessly be able to navigate between simulations and reality.
彼らはシミュレーションと現実の間をシームレスに移動できるようになるでしょう。
Or as he puts it, if you imagine this robot being able to navigate 10,000 different simulations, then you can simply think of the 10,001st simulation being our reality.
彼が言うように、もしもこのロボットが10,000の異なるシミュレーションを移動できると想像すると、10,001番目のシミュレーションが私たちの現実であると考えることができます。
For that agent, it would be just another Tuesday, just another reality that it was able to generalize across.
そのエージェントにとっては、それはただの別の火曜日であり、横断できた別の現実に過ぎません。
And this is what we're seeing here with this robot training in Isaac Jim.
アイザック・ジムでこのロボットのトレーニングを見ているのがこれです。
And then that robotic wheel looking thing being able to generalize that to the real world.
そしてそのロボットの車輪のようなものがそれを現実世界に一般化できるようになっています。
All the skills that it learned in simulation translate seamlessly almost into the real world.
シミュレーションで学んだすべてのスキルが、ほぼそのまま現実世界に翻訳されます。
It's funny because if you go back just a few years ago, I mean, people would talk about this idea of the real world creating a simulated world.
数年前にさかのぼると、実際の世界がシミュレートされた世界を作り出すというアイデアについて人々が話していたことが面白いです。
And you know, five plus years ago, people would wonder, well, what was the purpose of creating this simulated world, right?
そして、5年以上前には、人々は、このシミュレートされた世界を作成する目的は何だろうと疑問に思っていましたね。
This thing that runs the simulation, it gives us everything.
このシミュレーションを実行しているものは、私たちにすべてを与えてくれます。
It gives us lives and the universe, everything, right?
それは私たちに生命と宇宙、すべてを与えてくれるのですね。
But why?
でも、なぜでしょうか?
And people would wonder and say things like, well, maybe it's because they wanted to see how things could unfold in the past in history.
そして、人々は疑問に思い、過去や歴史の中でどのように事が展開するかを見たかったのかもしれないと言ったりします。
Or in Rick and Morty, that simulated universe was there basically as a car battery.
あるいは、リック・アンド・モーティでは、そのシミュレートされた宇宙は基本的に車のバッテリーとして存在していました。
The people in it basically existed to generate energy to run the guy's car.
その中の人々は、基本的にその男の車を走らせるためにエネルギーを生成する存在でした。
The point is five years, we didn't really know what use a simulation was to the real world, kind of do now.
ポイントは、5年前、シミュレーションが実際の世界にとってどのように有用かを本当に知らなかった、今ではわかってきています。
It's becoming really obvious.
それは非常に明らかになっています。
The reason is data.
その理由はデータです。
These robotic hands running in simulation learning to twirl a pen are very useful to us.
このシミュレーションで回転するペンを習得するロボットの手は、私たちにとって非常に役立ちます。
Millions of them sitting there forever twirling pens or learning how to walk or juggling a ball or whatever is very, very useful to us because it provides data that we can extract and use it in the real world.
何百万ものロボットがそこに座って、ペンを回したり歩き方を学んだりボールをジャグリングしたりすることは、非常に役立つのです。なぜなら、それは私たちにデータを提供し、それを抽出して実世界で使用できるからです。
If our guesses are correct about how Sora used the Unreal Engine to maybe generate some of this data that was used for training that model, then that would be an example of a sort of simulation, a 3D simulation being used to generate data for some higher function, in this case, this AI model.
もし私たちの推測が正しいとすれば、SoraがUnreal Engineを使用して、おそらくこのデータの一部を生成し、そのモデルのトレーニングに使用した方法について、それはある種のシミュレーションの例になるでしょう。この場合、このAIモデルのためにデータを生成するために使用される3Dシミュレーションの一例です。
This is kind of an important thing to understand because in the last maybe five years or so, our perception of running a simulation went from oh that's cool.
これは理解するのが重要なことです。なぜなら、ここ5年ほどで、シミュレーションを実行するという私たちの認識が、単なる面白いものから、お金を生むものに変わったからです。
That's science.
それは科学です。
It might be useful.
それは役に立つかもしれません。
Hmm.
うーん。
How interesting.
面白いですね。
To now, it's money.
今では、それはお金です。
Money.
お金です。
It's the new gold because it produces data.
それは新しい黄金です。なぜなら、それはデータを生み出すからです。
Data is the new oil and various simulations are the oil wells.
データは新しい石油であり、さまざまなシミュレーションは油田です。
Unreal Engine produces data for a video engine.
Unreal Engineはビデオエンジンのためにデータを生成します。
This robot in a simulation picking up boxes and putting into your cart translates into a real robot capable of doing that.
シミュレーションで箱を拾ってカートに入れるロボットは、それを実際に行うことができる実際のロボットに変換されます。
Think about how much, for example, Amazon could benefit from having something like this that is able to, I mean, it's probably not going to have wheels on its hands, but you get the idea.
Amazonのような企業が、このようなものを持つことでどれだけ恩恵を受けるか考えてみてください。つまり、手には車輪が付いていないかもしれませんが、アイデアはわかりますよね。
Robots could be extremely useful, these sorts of dextrous, mobile robots capable of navigating any terrain, picking up boxes.
ロボットは、どんな地形でもナビゲートし、箱を拾うことができるこの種の器用で移動可能なロボットは、非常に役立つかもしれません。
They could be useful for a lot of different applications.
おそらく、さまざまな異なる用途に役立つ可能性があります。
And this firm is awaiting imminent patent approval on the next generation of robots that have both huge military and civilian applications.
そして、この企業は、巨大な軍事および民間の用途を持つ次世代のロボットについて、間近に特許承認を待っています。
Now, right now, your listener, this stock trades over the counter at 10 cents a share, but you can, yeah, sorry, I made that last part up.
今、今、あなたのリスナー、この株は10セントで店頭で取引されていますが、あなたは、はい、すみません、最後の部分は私がでっち上げたものです。
But the point remains, NVIDIA is doing a lot more pushing forward simulations.
しかし、ポイントは、NVIDIAはシミュレーションを前進させるために多くのことを行っています。
Who uses them?
誰がそれらを使うのですか?
Researchers, engineers, analysts.
研究者、エンジニア、アナリスト。
They're used to predict the weather patterns, simulate various spreads of diseases, accelerate financial models, speed up engineering simulations, self-driving cars, etc.
それらは、気象パターンを予測し、さまざまな病気の広がりをシミュレートし、金融モデルを加速し、エンジニアリングシミュレーションを高速化し、自動運転車などに使用されています。
They have a video somewhere where they simulate an entire BMW factory, including the production line, workers.
彼らはどこかにビデオがあり、BMW工場全体をシミュレートしており、生産ラインや労働者を含んでいます。
And like you see, there are clips where a worker approaches a piece of machinery and like it shows him getting hurt, not like graphically, but it clips him and flashes red, meaning, hey, this is a dangerous situation.
そして、労働者が機械に近づくシーンがあり、彼がけがをする様子が示されていますが、グラフィック的ではなく、彼をクリップし、赤く点滅させることで、これは危険な状況であることを示しています。
So this 3D simulation of a plant, of a car production plant, I believe it was BMW that was requesting that, gets fully simulated with people running around it.
このBMWの工場の3Dシミュレーション、車の生産工場のシミュレーションは、周囲を走り回る人々とともに完全にシミュレートされます。
And then when all the bugs are ironed out that data is taken out
そして、すべてのバグが取り除かれたら、そのデータは取り出されます。
And then an actual real-world plant is then built with those specifications.
それから、その仕様書に基づいて実際の現実世界の工場が建設されました。
Generative agents was a type of simulation.
生成エージェントは一種のシミュレーションでした。
It wasn't 3D, it was pretty simple, but basically each character was its own GPT, ChatGPT, running around, making friends, making decisions.
それは3Dではなく、かなりシンプルでしたが、基本的に各キャラクターは独自のGPT、ChatGPTであり、周りを走り回り、友達を作り、決定を下していました。
And their behavior was extremely lifelike, extremely believable, more so than the human players that were playing the game.
そして、彼らの行動は非常に生き生きとしており、非常に信じられるものであり、ゲームをプレイしている人間プレイヤーよりもそうでした。
But my point here is that we're probably going to be seeing more and more advanced simulations with more and more intricate details.
私の言いたいことは、ますます高度なシミュレーションがより複雑な詳細を持つようになる可能性が高いということです。
I mean, some of these robotic sims, they're simulating current flowing through the various appendages, maybe even having different frictions on different arms of the robot, different current flow through the arms.
つまり、これらのロボットのシミュレーションの中には、さまざまな付属物を通る電流をシミュレートしているものもあり、ロボットの異なる腕に異なる摩擦、異なる電流が流れるかもしれません。
So basically simulating the various inconsistencies and potential differences that could happen in the real world, different levels of friction, different wind, et cetera.
現実世界で起こりうるさまざまな不一致や潜在的な違い、摩擦の異なるレベル、異なる風などをシミュレートしています。
So when we take it out, that's at least what this deep mind, robots playing soccer, that's kind of what they were talking about is they're able to create these robots that first of all, none of this was taught.
これらのディープマインド、サッカーをするロボット、それが話していたのは、まず、これらのロボットを作成できるということで、これらのことはすべて教えられたものではありませんでした。
So how they play was 100% learned in a simulation to kind of, kind of looks like this.
彼らがどのようにプレイするかは、シミュレーションで100%学習されたもので、まるでこれのように見えます。
They figure out how to do various things.
彼らはさまざまなことをどうやって行うかを見つけ出します。
And because of those different shifts in how various forces functions, et cetera, they're more robust when they come out of the simulation, they're more robust to the little things that can go wrong.
そして、さまざまな力の機能の変化によって、シミュレーションから出てきたときに、さまざまなことがうまくいかない小さなことに対してより堅牢になります。
They're better able to handle adversity, if you will.
彼らは、必要に応じて、逆境に対処する能力が向上しています。
So I don't think it's a stretch to say that eventually we'll be simulating entire parts of reality, potentially even at some point, something that approaches the entire reality.
だから、いつかは現実の部分をシミュレートすることも、おそらく、いつかは現実全体に近いものを模倣することもあるかもしれません。
And in terms of whether or not we live in the base reality or in one of the simulations, I mean, that's just a thought experiment.
そして、私たちが基本的な現実の中に住んでいるのか、シミュレーションの中の1つに住んでいるのか、それはただの思考実験です。
I would not take this thing too seriously.
私はこのことをあまり真剣に受け取らないでしょう。
Although I got to say this double slit experiment, it's so weird, because it sure seems like parts of our reality don't get rendered until we're looking at it, until we're measuring it in some way.
しかし、この二重スリット実験はとても奇妙です。なぜなら、私たちの現実の一部が、私たちがそれを見ている、何らかの方法で測定しているときまでレンダリングされないように思えるからです。
And there's a new research published in April 2023 that has demonstrated that the double slit experiment also holds true regarding time and not just space.
そして、2023年4月に発表された新しい研究では、二重スリット実験が空間だけでなく時間にも当てはまることが示されています。
But it's probably nothing.
でも、おそらく何もないでしょう。
In other news, Groq with a q, an AI hardware startup, creates an insanely fast and inexpensive AI chip to run inference.
その他のニュースでは、AIハードウェアの新興企業である「Groq(グロック)」が、推論を実行するための非常に高速で手頃な価格のAIチップを開発しました。
So they have these LPU architecture, language processing unit, a new class of processor for a new class of workloads.
そこで、彼らはこれらのLPUアーキテクチャ、言語処理ユニット、新しいワークロード向けの新しいプロセッサクラスを持っています。
And you can test that at groq.com, g-r-o-q.com, and it's fast.
そして、それをgroq.comでテストすることができます。速いです。
It is very, very fast.
非常に、非常に速いです。
Here's a prompt, create a short story about a traveling AGI salesman.
こちらがプロンプトです。旅行するAGIセールスマンについての短編小説を作成してください。
And there it goes.
そして、そこに行きます。
So that was 3.2 seconds.
それは3.2秒でした。
And here it's running Mixtrel, the open source model.
そして、ここではオープンソースモデルMixtrelを実行しています。
We might have to do a full deep dive on Groq, but at this point, it seems like there's another very interesting, very powerful competitor in the AI chip space.
おそらく、Groqについて詳しく調査する必要があるかもしれませんが、この時点では、AIチップ市場にもう1つ非常に興味深く、非常に強力な競合他社があるようです。
It probably is going to have its own sort of niche that it's going to be good at.
おそらく、自分自身の得意分野を持つことになるでしょう。
Here's a quick interview with one of the founders.
こちらが創業者の1人との簡単なインタビューです。
Whenever a discussion about artificial intelligence takes place, the rooms here, the huge halls get hacked.
人工知能に関する議論が行われると、ここで、巨大なホールがハッキングされます。
The world's first language processing unit that can run programs like Meta's LLaMA 2 model, for example, faster than anything else in the world.
世界初の言語処理ユニットであり、例えばMetaのLLaMA 2モデルのようなプログラムを世界で最速で実行できるものです。
10 to 100 times faster.
10〜100倍速いです。
It's Groq and we spell it with a Q, and it's because it comes from a science fiction novel and it means to understand something deeply and with empathy.
それがGroqで、Qをつけて綴ります。それはSF小説から来ており、何かを深く共感を持って理解することを意味します。
Of course it does.
もちろんです。
We've had all the greatest minds in here.
私たちの中にはすべての偉大な知識人がいます。
What's your story?
あなたのお話は何ですか?
Most chips, they don't have enough memory inside of them.
ほとんどのチップには中に十分なメモリがありません。
Then you need to set up part of the assembly line, tear it down over and over again.
それから、組み立てラインの一部を設定し、何度も壊す必要があります。
Right.
そうですね。
And that's slow and it takes a lot of time.
そして、それは遅くて多くの時間がかかります。
And that's what happens with the GPU.
そして、それがGPUで起こることです。
You have to read from that memory.
そのメモリから読み取る必要があります。
So thousands of times for every word that comes out, it's as if you're setting up the assembly line over and over.
出てくる単語ごとに何千回も、まるで組み立てラインを何度も設定しているかのようです。
Hey, I'm Groq.
こんにちは、私はGroqです。
You can talk to me like I'm a person.
私には人間と話すように話してもらえます。
All right.
わかりました。
I will do.
します。
What makes you different from a Large Language Model that I may have used in the past?
過去に使用した可能性のある大規模言語モデルとの違いは何ですか?
Sure.
もちろん。
I'm a bit different from those big language models you've used before.
私は、以前に使われていた大規模な言語モデルとは少し違います。
I'm a language user interface or Louie designed to have more natural...
私は、より自然なコミュニケーションを持つように設計された言語ユーザーインターフェース、またはルイと呼ばれています。
Got it.
了解しました。
How similar are you to a human brain?
あなたは人間の脳とどれくらい似ていますか?
Well, I'm not a human brain, but I'm designed to understand and respond to like one.
まあ、私は人間の脳ではありませんが、人間のように理解し、応答するように設計されています。
What I want to know is if they're in the middle of Dubai, how is she this blitzed?
私が知りたいのは、彼女がドバイのど真ん中にいるとき、なぜこんなに酔っているのかということですか?
In other news, remember Q-Star?
他のニュースでは、Q-Starを覚えていますか?
Apparently former GitHub CEO, Nat Friedman and his investment partner, Daniel Gross, shelled out 100 million to Magic, the developer of an artificial intelligence coding assistant.
元GitHubのCEOであるナット・フリードマン氏と、彼の投資パートナーであるダニエル・グロス氏が、人工知能コーディングアシスタントの開発者であるMagicに1億ドルを投じたとのことです。
What did they see?
彼らは何を見たのですか?
Well, they're claiming to be able to process 3.5 million words of text input.
まあ、彼らは、350万語のテキスト入力を処理できると主張しています。
And Magic also privately claimed to have made a technical breakthrough that could enable active reasoning capabilities similar to the Q-Star model developed by OpenAI last year.
そして、Magicは、昨年OpenAIによって開発されたQ-Starモデルに類似したアクティブな推論能力を可能にする技術的なブレークスルーを達成したとも非公式に主張しています。
Magic's co-founder and CEO, Eric Steinberger, has grappled with the problem of getting AI models to reason before.
Magicの共同創設者兼CEOであるエリック・スタインバーガーは、以前からAIモデルが推論する問題に取り組んできました。
He previously worked at Meta-platforms conducting research on how reinforcement learning can help AI models find optimal solutions.
以前はMeta-platformsで働いており、強化学習がAIモデルが最適な解決策を見つけるのにどのように役立つかについて研究を行っていました。
So not too much details here.
ここにはあまり詳細がありません。
So maybe there's not too much there to dig in.
掘り下げるほどの情報はあまりないかもしれません。
Maybe it's hype marketing we'll see.
おそらく、それは宣伝効果かもしれませんね。
But it sounds like the investors not only are giving them a hundred million, but also providing with their private cluster of GPU units of NVIDIA chips.
しかし、投資家たちは1億ドルを提供するだけでなく、NVIDIAチップのプライベートクラスターも提供しているようです。
So sounds like they're seeing something.
彼らは何かを見ているようです。
So let's keep an eye on Magic and see what they're cooking up.
Magicに目を光らせて、彼らが何を考えているのか見てみましょう。
Next in AI news, we have a bit of a plot twist.
次にAIのニュースでは、少し筋書きが変わります。
Stanford Medicine study identifies distinct brain organization patterns in women and men.
スタンフォード医学研究所の研究により、女性と男性の脳の組織パターンが異なることが特定されました。
Stanford Medicine researchers have developed a powerful new artificial intelligence model that can distinguish between male and female brains.
スタンフォード医学研究者たちは、男性と女性の脳を区別できる強力な新しい人工知能モデルを開発しました。
The extent to which a person's sex affects how the brain is organized and operates has long been a point of dispute among scientists.
人の性別が脳の組織や機能にどの程度影響を与えるかは、科学者たちの間で長い間議論の的となってきました。
While we know the sex chromosomes we are born with help determine the cocktail of hormones our brains are exposed to, particularly during early development, puberty, and aging, researchers have long struggled to connect sex to concrete differences in the human brain.
生まれつき持っている性染色体が、特に早期の発達、思春期、加齢期において脳にさらされるホルモンのカクテルを決定するのにどのように影響を与えるかは、長い間研究者たちが脳の具体的な違いと性別を結びつけるのに苦労してきました。
Brain structures tend to look much the same in men and women, and previous research examining how brain regions work together has also largely failed to turn up consistent brain indicators of sex.
男性と女性の脳の構造はほぼ同じように見え、脳の領域がどのように協力して働くかを調べた以前の研究も、主に性別の一貫した脳の指標を見つけることに失敗してきました。
In their current study, so this is Vinod Menon and his team took advantage of recent advances in artificial intelligence, as well as multiple large data sets to pursue a more powerful analysis than has previously been employed.
彼らの現在の研究では、ヴィノド・メノンと彼のチームは、最近の人工知能の進歩と複数の大規模データセットを活用して、これまでに使用されてきたより強力な分析を追求しました。
First, they create a deep neural network model which learns to classify brain imaging data.
まず、彼らは脳画像データを分類する深層ニューラルネットワークモデルを作成しました。
As researchers showed brain scans to the model and told it that it was looking at a male or female brain, the model started to notice what subtle patterns could help it tell the difference.
研究者たちは脳のスキャン画像をモデルに見せ、男性の脳または女性の脳を見ていると伝えると、モデルはどの微妙なパターンが違いを見分けるのに役立つかに気づき始めました。
This model demonstrated superior performance compared with those in previous studies in part because it used deep neural network that analyzes dynamic MRI scans.
このモデルは、動的MRIスキャンを分析する深層ニューラルネットワークを使用したため、以前の研究よりも優れたパフォーマンスを示しました。
When the researchers tested the model on around 1500 brain scans, it could almost always tell if the scan came from a woman or a man.
研究者が約1500の脳スキャンでモデルをテストしたところ、スキャンが女性か男性かをほぼ常に判別できました。
The fact that it works so well in many different data sets, including brain scans from multiple sites in the US and Europe, make the findings especially convincing as it controls for many confounds that can plague studies of this kind.
このモデルが米国やヨーロッパの複数の施設からの脳スキャンを含むさまざまなデータセットで非常にうまく機能することは、この種の研究における多くの混乱要因をコントロールしているため、特に説得力があります。
This is a very strong piece of evidence that sex is a robust determinant of human brain organization, Menon said.
性別が人間の脳の構成に強力な影響を与えることの証拠であると、メノンは述べました。
The team then wondered if they could create another model that could predict how well participants would do on certain cognitive tasks based on functional brain features that differ between women and men.
その後、チームは、女性と男性の間で異なる機能的脳特性に基づいて、参加者が特定の認知課題でどれだけうまくできるかを予測できる別のモデルを作成できるか疑問に思いました。
Does this man have no fear?
この男性は恐れを知らないのですか?
They developed sex-specific models of cognitive abilities.
彼らは認知能力の性別固有のモデルを開発しました。
One model effectively predicted cognitive performance in men but not women, and another in women but not men.
1つのモデルは男性の認知パフォーマンスを効果的に予測しましたが、女性ではそうではありませんでした。もう1つは女性の認知パフォーマンスを予測しましたが、男性ではそうではありませんでした。
The findings indicate that functional brain characteristics varying between the sexes have significant behavioral implications.
研究結果は、性別間で異なる機能的脳特性が重要な行動上の影響を持つことを示しています。
So I'm very curious to know how this is received because, I mean, here in the States at least, we have a bit of a contentious discussion about some of these things.
私は、これがどのように受け入れられるか非常に興味があります。というのも、少なくともここアメリカでは、これらのことについて議論が少し熱いからです。
How does a study like this play into it?
このような研究は、それにどのように関わってくるのでしょうか?
In other news, Google releases Gemma, their new state-of-the-art open models.
他のニュースでは、Googleが最新鋭のオープンモデルであるGemmaをリリースしました。
So Gemma is a family of lightweight, state-of-the-art, open models built from the same technology that's used to create the Gemini models.
Gemmaは、Geminiモデルを作成するのに使用されている同じ技術から構築された軽量で最新鋭のオープンモデルのファミリーです。
So they're releasing the model weights, as well as some tools to support developers.
そのため、彼らはモデルの重みを公開し、開発者をサポートするためのいくつかのツールも提供しています。
It looks like here it shows its comparison to LLaMA 2, doing seemingly better on most of the tasks, at least where it's compared.
ここでは、LLaMA 2との比較を示しており、少なくとも比較されているタスクのほとんどで見た目はより良いようです。
So here I'm happy they stopped saying AI safety when they're meaning censoring certain outputs.
ここでは、特定の出力を検閲することを意味するときにAIセーフティと言うのをやめてくれて嬉しいです。
That shouldn't be AI safety, right?
それはAIの安全性ではないはずですよね?
So it seems like they're using responsible now here, which is good.
ここでは責任あるという言葉が使われているようですね、それは良いことです。
So my concern would be, did they over-tighten the censorship screws or not?
私の懸念は、彼らが検閲のネジを締めすぎたのかどうかです。
That might make it very difficult to use.
それは非常に使いにくくなるかもしれません。
If not, then it could be a very good tool.
そうでなければ、それは非常に良いツールになるかもしれません。
Either way, having more open source models is phenomenal for the whole industry as a whole.
どちらにせよ、より多くのオープンソースモデルは業界全体にとって素晴らしいです。
More people developing stuff, sharing information, and the open source community, the power of the open source community grows.
より多くの人々が開発し、情報を共有し、オープンソースコミュニティが成長すると、オープンソースコミュニティの力が増します。
So that's it for me today.
それで今日はこれで終わりです。
I hope you enjoyed that.
楽しんでいただけたと嬉しいです。
My name is Wes Roth, and thank you for watching.
私の名前はウェス・ロスです、ご視聴ありがとうございました。
この記事が気に入ったらサポートをしてみませんか?