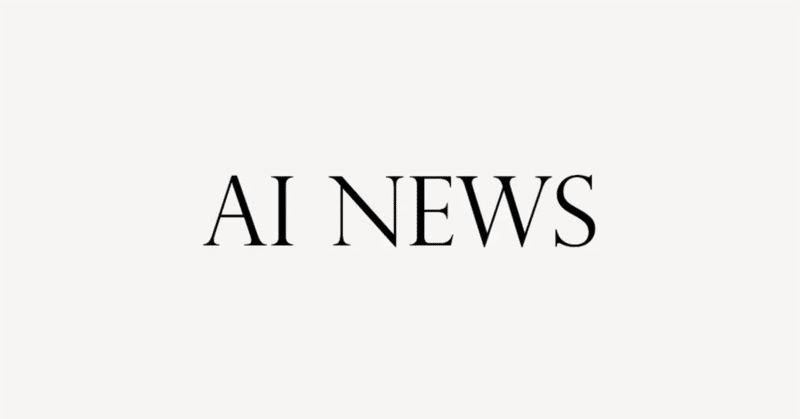
【NVIDIAの「Foundation Agent」が業界に衝撃を与える!】英語解説を日本語で読む【2024年3月23日|@Wes Roth】
2016年春、コロンビア大学の授業中にAlphaGoと李世ドルの囲碁対決を観戦し、AIが人間のチャンピオンに勝利した歴史的瞬間を目の当たりにしました。その時、AIの可能性に魅了されたが、AlphaGoは囲碁にしか使えないことに気づき、より汎用的で多様なAIエージェントの必要性を感じました。この夢を追求し、NVIDIAでGEAR Labを設立し、一般化されたエージェント研究に取り組んでいます。マインクラフトを使用してAIの訓練を行い、さまざまなタスクを実行できるエージェントを開発しています。この研究は、汎用AIへの一歩となることを目指しています。
公開日:2024年3月23日
※動画を再生してから読むのがオススメです。
I want to tell you guys a story about the spring of 2016 when I was taking a class at Columbia university, but I wasn't actually paying attention to the lecture and instead I was watching a board game tournament on my laptop.
私は2016年の春、コロンビア大学で授業を受けていた時の話を皆さんにお話したいのですが、実際には講義には注意を払っておらず、代わりにノートパソコンでボードゲームのトーナメントを見ていました。
And it wasn't just any tournament, but a very, very special one.
そして、それはただのトーナメントではなく、非常に特別なものでした。
The match was between DeepMind, AlphaGo and Lee Sedol and the AI just won three out of five games and became the first ever to beat a human champion at a game of Go.
その試合はDeepMind、AlphaGo、李世ドルの間で行われ、AIが5試合中3試合を勝ち取り、人間のチャンピオンを初めて打ち負かした瞬間でした。
I still remember the adrenaline of seeing history unfold, the moment of glory when AI agents finally enter the mainstream, but when the excitement fades, I realized that as mighty as AlphaGo was, it can only do one thing and one thing alone.
歴史が展開する様子を見ているときのアドレナリンを今でも覚えています。AIエージェントがついに主流に入る瞬間ですが、興奮が薄れると、AlphaGoがどれだけ強力であっても、ただ1つのことしかできないことに気づきました。
It is not able to play any other games like Super Mario or Minecraft, and it certainly cannot do your dirty laundry or dishes, but what we truly want are AI agents as versatile as Wall-E, as diverse as all the robot forms or embodiments in Star Wars and works across infinite worlds, virtual or physical as in Ready Player One.
それはスーパーマリオやマインクラフトのような他のゲームをプレイすることができず、汚れた洗濯物や食器を洗うこともできません。しかし、私たちが本当に望むのは、Wall-Eのように多目的なAIエージェントであり、スター・ウォーズのすべてのロボット形態や具現化のように多様であり、Ready Player Oneのように仮想または物理的な無限の世界を横断するものです。
How do we get there in possibly the near future?
近い将来にどうやってそこに到達するのでしょうか?
This is your hitchhiker's guide to general purpose AI agents.
これが多目的AIエージェントへのヒッチハイカーのガイドです。
Most of the ongoing research efforts can be laid out across these three axes, the number of skills an agent can do, the embodiments it can control and the realities it can master.
進行中の研究のほとんどは、エージェントができるスキルの数、制御できる具現化、そしてマスターできる現実の3つの軸に沿って配置されることができます。
And this is where AlphaGo is, but the upper right corner is where we all want to go.
そして、ここがAlphaGoの位置ですが、右上の隅が私たちが行きたい場所です。
I've been thinking for most of my career about how to travel across this galaxy of challenges towards this upper right corner.
私はほとんどのキャリアで、この課題の銀河を横断して、この右上の隅に向かう方法を考えてきました。
And earlier this year, I had a great fortune to establish GEAR Lab with Jensen's support and blessing, and I'm very proud of the name.
そして今年の初め、ジェンスンの支援と祝福を受けて、GEAR Labを設立する機会に恵まれました。その名前にとても誇りを持っています。
GEAR, G-E-A-R stands for Generalist Embodied Agent Research.
GEAR、G-E-A-RはGeneralist Embodied Agent Researchの略です。
I'm co-leading this initiative with Yuka Zhu, and this is a picture that we took seven years ago at Stanford, where Yuka and I were both still PhD students at Fei Fei Li's group.
私はYuka Zhuと共同でこの取り組みをリードしており、こちらは7年前にStanfordで撮った写真です。Yukaと私はFei Fei Liのグループでまだ博士課程の学生でした。
And we did robotics hackathons all the time because especially before deadlines, we were the most productive.
私たちはロボティクスのハッカソンをし続けていました。特に締め切り前は、私たちは最も生産的でした。
And here Ajay on the left is from Adidas group, also at NVIDIA research, working very closely with GEAR, and all three of us basically moved from Stanford to NVIDIA.
そして、左側のAjayはAdidasグループからで、NVIDIA研究所でもGEARと非常に密接に連携しています。私たち3人は基本的にStanfordからNVIDIAに移動しました。
And man, we were so young at that time.
当時私たちはとても若かったですね。
Look at what PhD did to us.
博士課程が私たちにどのように影響を与えたかを見てください。
You know, the quest for AGI is a lot of pain and suffering.
AGIへの探求は多くの苦痛と苦しみを伴います。
Let's go back to the first principles.
最初の原則に戻りましょう。
What essential features does a journalist agent need?
ジャーナリストエージェントにはどんな重要な特徴が必要ですか?
And I would argue three things.
そして、私は3つのことを主張します。
First, it should be able to survive, navigate, and exploring an open-ended world.
まず、それは生き残り、航行し、無限の世界を探索できる必要があります。
And AlphaGo has a singular goal, and it's simply not open-ended.
そして、AlphaGoには単一の目標があり、単純に無限ではありません。
And second, world knowledge.
そして2つ目は、世界の知識です。
The agent should have a large amount of pre-trained knowledge instead of knowing only a few concepts in the environment.
エージェントは、環境内のいくつかの概念だけを知っているのではなく、事前に大量の知識を持っている必要があります。
And third, as a journalist agent, the name implies, it must be able to perform more than a few tasks, ideally infinitely multitask.
そして3つ目、ジャーナリストエージェントという名前が示すように、少数のタスク以上を実行できる必要があり、理想的には無限にマルチタスクをこなせる必要があります。
You prompt it with any reasonable language and the agent should be able to complete that mission for you.
あなたが任意の言語でプロンプトすると、エージェントはそのミッションを完了できる必要があります。
What does it take?
それには何が必要ですか?
Correspondingly, the environment needs to be open-ended enough because the agent's complexity is upper bounded by the environment complexity.
環境の複雑さがエージェントの複雑さの上限となるため、環境は十分にオープンエンドである必要があります。
And planet Earth that we live on is actually a perfect example, because the Earth is so open-ended that it enables an algorithm called natural evolution to produce all the diverse behaviors of life on this planet.
そして、私たちが生活している地球は実際には完璧な例です。地球は非常にオープンエンドなため、自然進化というアルゴリズムがこの惑星上の生命の多様な行動を生み出すことができます。
Can we have a simulator that is essentially a lo-fi Earth, but we can still run on our lab computers?
私たちの研究室のコンピューターで実行できる、本質的にローファイの地球であるシミュレーターを持つことは可能でしょうか?
And second, we need to provide the agent with massive pre-training data because exploration from scratch in such an open-ended world is simply intractable.
そして、第二に、このようなオープンエンドな世界でゼロからの探索は単純に取り組みがたいため、エージェントに膨大な事前トレーニングデータを提供する必要があります。
And this data will be a reference manual on how to do things and more importantly, what are the interesting things worth doing?
そして、このデータは、何をする方法や、さらに重要なことに、どのような興味深いことをする価値があるかについての参考マニュアルとなります。
And finally, we need a foundation model that's scalable enough to convert this large scale data into actionable insight.
最後に、この大規模なデータを実用的な洞察に変換するために拡張可能な基礎モデルが必要です。
And this train of thought lends us in Minecraft, the best-selling video game of all time.
そして、この思考の流れは私たちをマインクラフトに導きます。これは史上最も売れたビデオゲームです。
And for those who are not familiar, Minecraft is this procedurally generated world of 3D voxels.
そして、馴染みのない方のために、マインクラフトは3Dボクセルの手続き的に生成された世界です。
And in this game, you can do whatever your heart desires.
このゲームでは、自分の心のままに何でもできます。
What's special about the game is that Minecraft defines no particular score to maximize and no objective to follow.
このゲームの特別な点は、最大化すべき特定のスコアもなく、追うべき目標もないことです。
And that makes it very well-suited as a truly open-ended environment.
それが、本当にオープンエンドの環境に非常に適しているということです。
And as a result, we see some very impressive creations, like someone built the Hogwarts castle block by block in Minecraft and someone else, apparently with nothing better to do, built a functional neural network.
その結果、私たちは非常に印象的な創造物を見ることができます。例えば、誰かがマインクラフトでホグワーツ城をブロックごとに建てたり、他の誰かが、明らかに他にやることがなかったのか、機能的なニューラルネットワークを構築したりします。
Because Minecraft has logical gates and is apparently Turing complete.
なぜなら、マインクラフトには論理ゲートがあり、明らかにチューリング完全であるからです。
I want to highlight a number here.
ここで数字を強調したいと思います。
Minecraft has 140 million active players.
マインクラフトには1億4000万人のアクティブプレイヤーがいます。
And just to put this number in perspective, this is more than twice the population of UK.
そして、この数字を見ると、これはイギリスの人口の2倍以上です。
And it just so happens that gamers are generally happier than PhDs.
そして偶然にも、ゲーマーは一般的に博士号を持つ人よりも幸せです。
They love to play and share their journey online.
彼らはプレイを楽しんで、その旅をオンラインで共有するのが大好きです。
This huge human mass of gamers produce a lot of data every day.
この巨大なゲーマーの人間集団は、毎日多くのデータを生み出しています。
And the question is, how can we tap into this treasure trove of data?
そして問題は、このデータの宝庫にどのようにアクセスできるかということです。
We introduced Mind Dojo, a new open framework to help the community develop general purpose agents using Minecraft as a kind of primordial soup.
私たちは、Mind Dojoという新しいオープンフレームワークを紹介しました。これは、マインクラフトを一種の原始スープとして使用して、コミュニティが汎用エージェントを開発するのを支援します。
Mind Dojo has three parts, a simulator, a database, and a model.
Mind Dojoには、シミュレータ、データベース、モデルの3つの部分があります。
The simulator API we built unlocks the full potential of the game for AI research, and we support observation space like RGB and voxel and GPS, and two levels of action space.
私たちが構築したシミュレータAPIは、AI研究のためにゲームのフルポテンシャルを引き出し、RGBやボクセル、GPSなどの観測空間をサポートし、2つのアクション空間を提供しています。
And Mind Dojo can be customized at every detail, such as terrains, weathers, and monster spawning.
Mind Dojoは、地形や天候、モンスターの出現など、細部までカスタマイズできます。
And it also supports creative tasks that are freeform and open-ended.
また、フリーフォームでオープンエンドな創造的なタスクもサポートしています。
For example, let's say we want the agent to build a house, but what makes a house a house, right?
たとえば、エージェントに家を建てさせたいとしますが、家を家たらしめるものは何でしょう?
It's very difficult to implement this kind of success criterion in simple Python code.
このような成功基準をシンプルなPythonコードで実装するのは非常に難しいです。
And the only way is to use foundation models, train our internet skill knowledge so that the abstract concept of a house can be captured.
唯一の方法は、基礎モデルを使用し、抽象的な家の概念を捉えるためにインターネットスキルの知識を訓練することです。
And next we collected an internet skill knowledge base of Minecraft to help the agent lift off the ground, because it's really hard to explore from scratch.
そして次に、エージェントがスタートダッシュを切るのを助けるために、マインクラフトのインターネットスキルの知識ベースを収集しました。ゼロから探索するのは本当に難しいからです。
And this database got three parts.
そして、このデータベースには3つの部分があります。
The first is video.
第一はビデオです。
We find that Minecraft is among the most streamed game online, and gamers just like to talk about what they're doing.
私たちは、マインクラフトがオンラインで最もストリーミングされているゲームの1つであり、ゲーマーは自分たちがしていることについて話すのが好きであることを発見しました。
We collected more than 300,000 hours of videos with more than two billion words in transcript.
私たちは、300,000時間以上のビデオを収集し、2十億語以上の転写を行いました。
And the second is Minecraft Wiki with 7,000 multi-modal pages of images, tables, and diagrams.
そして、第二には、画像、表、図などを含む7,000のマルチモーダルページを持つマインクラフト Wikiがあります。
The third is the Minecraft subreddit, which we found that people use as a kind of stack overflow when they need some help on Minecraft.
三番目は、マインクラフト subredditで、マインクラフトで何か助けが必要な時に人々がスタックオーバーフローのように使用していることがわかりました。
Here's a peek at our Mind Dojo Wiki dataset.
こちらが私たちのMind Dojo Wikiデータセットの一部です。
And can you believe that someone listed all the crafting recipes, thousands of them, and explains all the monsters and basically every possible game mechanics you'll ever see in every version of Minecraft.
信じられますか、誰かがすべてのクラフトレシピ、数千ものレシピをリストアップし、すべてのモンスターや基本的にマインクラフトのすべてのバージョンで見ることができるすべてのゲームメカニクスを説明しているのです。
One thing I learned is that gamers just got a lot of time to...
私が学んだことの一つは、ゲーマーはただたくさんの時間を持っているということです。
Well, but I'm not complaining, right?
まあ、でも私は不平を言っていませんよね?
Because thanks for the data, please do more.
データをありがとうございます、もっとしてください。
Thanks for the data.
データをありがとうございます。
What to do with the data?
そのデータをどうするか?
It's time to train a foundation model.
基礎モデルのトレーニングの時間です。
Here the idea is very simple.
ここではアイデアは非常にシンプルです。
For our YouTube database, we have time aligned video clips and transcripts, and these are actually real tutorial videos.
私たちのYouTubeデータベースでは、時間に整列されたビデオクリップとトランスクリプトがあり、実際には実際のチュートリアルビデオです。
Like here in Text Prompt 3, as I raise my axe in front of this pig, there's only one thing you know is going to happen.
ここでのText Prompt 3のように、私がこの豚の前で斧を上げると、起こることは1つだけです。
This is actually from a YouTube tutorial.
これは実際にYouTubeのチュートリアルからです。
We then can train a pair of encoders to map the video and the transcript to a vector embedding.
その後、ビデオとトランスクリプトをベクトル埋め込みにマッピングするためのエンコーダーのペアをトレーニングできます。
And then the embeddings can be trained by a process called contrastive learning, which essentially pulls together video and the text that match and pushes apart those that don't match.
そして、埋め込みは、基本的に一致するビデオとテキストを引き寄せ、一致しないものを引き離すというプロセスでトレーニングされます。
And this pair of encoders is called the Mind Clip model.
そして、このエンコーダーのペアはMind Clipモデルと呼ばれています。
And intuitively, Mind Clip learns the association between the video and the transcript that describes the action in the video.
そして直感的に、Mind Clipは、ビデオとビデオ内のアクションを説明するトランスクリプトとの関連付けを学習します。
It outputs a score between zero and one.
ゼロから1の間のスコアを出力します。
And one means perfect description and zero means that the text has nothing to do with the video.
1は完璧な説明を意味し、0はテキストがビデオと何の関係もないことを意味します。
This effectively becomes a language-conditioned foundation reward model that understands the nuances of forest, animal behaviors, architectures, you name it in Minecraft, all the abstract concepts.
これは実質的に、森林、動物の行動、アーキテクチャなど、マインクラフトでの抽象的な概念を理解する言語条件つきの基礎報酬モデルになります。
How do we use Mind Clip in action?
Mind Clipをどのように活用すればよいのでしょうか?
Here, an agent interacts with our Mind Dojo simulator and the task is in English, share sheep to obtain wool.
ここでは、エージェントが当社のMind Dojoシミュレーターとやり取りし、タスクは英語で、「羊を共有して羊毛を取得する」というものです。
As the agent explores, it generates a video snippet, which can be encoded and fed to Mind Clip.
エージェントが探索すると、ビデオスニペットが生成され、それはエンコードされてMind Clipに送られます。
And then it computes the association.
そして、それは関連を計算します。
The higher the score is, the more the agent's behavior is aligned with the text prompt, and that becomes the reward function to any reinforcement learning algorithm that you like.
スコアが高いほど、エージェントの行動がテキストプロンプトと一致し、それが好きな強化学習アルゴリズムへの報酬関数となります。
This looks very familiar, right?
これはとても馴染み深いですね。
Because it's essentially reinforcement learning from human feedback or ROHF.
人間のフィードバックやROHFからの本質的な強化学習だからです。
And ROHF is the cornerstone that powers TragiPT, and I believe it's going to play a critical role in embodied agents as well.
そしてROHFはTragiPTを支える基盤であり、具現化されたエージェントにも重要な役割を果たすと信じています。
And here are some demos of our learned agent behavior on various tasks.
こちらは、様々なタスクで学習したエージェントの振る舞いのデモです。
Let's put Mind Clip on this Hitchhiker's Guide.
このヒッチハイカーズ・ガイドにMind Clipを置いてみましょう。
It's able to do a lot more tasks than AlphaGo, but the limitation is that you have to manually decide a task prompt and run training for each skill.
AlphaGoよりも多くのタスクをこなすことができますが、制限として、各スキルごとにタスクプロンプトを手動で決定し、トレーニングを実行する必要があります。
And the agent isn't really able to discover new things by itself.
そしてエージェントは本当に自分で新しいことを発見することができません。
But this all changed in 2023, where a model called GP4 came that's a language model so powerful at coding and planning.
しかし、すべてが変わったのは2023年、コーディングや計画立案に非常に強力な言語モデルであるGP4というモデルが登場した時でした。
We built Voyager, an agent that massively scales on the number of skills.
私たちはVoyagerというエージェントを構築しました。これは、スキルの数を大幅にスケールさせるものです。
And when we set Voyager loose in Minecraft, it is able to play the game for hours on end without any human intervention.
ボイジャーをマインクラフトで解放すると、人間の介入なしに何時間もゲームをプレイすることができます。
And the video I show here are snippets from a single episode where Voyager just keeps going.
ここで紹介するビデオは、ボイジャーが延々と続く単一のエピソードからの断片です。
It explores the terrains, mine all kinds of materials, fight monsters, craft hundreds of recipes, and unlocks an ever-expanding tree of skills.
それは地形を探検し、さまざまな材料を採掘し、モンスターと戦い、数百のレシピを作り、そしてスキルの枝を拡張していきます。
What's the magic behind it?
それの魔法は何でしょうか?
The key insight is coding as action.
鍵となる洞察は、コーディングを行動として捉えることです。
We convert the 3D world into a textual representation, thanks to an open source Minecraft mod called Mind Flayer.
私たちは、Mind Flayerというオープンソースのマインクラフト modによって、3Dワールドをテキスト表現に変換します。
And Voyager invokes GPT-4 to generate code snippets in JavaScript.
そして、ボイジャーはJavaScriptでコードスニペットを生成するためにGPT-4を呼び出します。
And each snippet becomes an executable skill in the game.
そして、各スニペットはゲーム内で実行可能なスキルになります。
And just like human engineers, the program that Voyager writes wouldn't always be correct on the first try.
そして、人間のエンジニアと同様に、ボイジャーが書くプログラムは常に最初の試みで正しくなるわけではありません。
We have a self-reflection mechanism to help it improve.
私たちは、それが改善するのを助けるための自己反省メカニズムを持っています。
And a self-reflection relies on three sources, the JavaScript execution error, the agent's current state, like hunger and health, and the world state, like the landscape or the monsters nearby.
そして、自己反省は、JavaScriptの実行エラー、エージェントの現在の状態(空腹や健康など)、そして世界の状態(景色や近くのモンスターなど)に依存しています。
And the agent takes an action, observes the consequence of its action on the world and on itself, reflects on how it could do better, try out more actions and rinse and repeat.
エージェントは行動を取り、その行動が世界や自分自身に与える影響を観察し、より良い方法を考え、さらに行動を試して繰り返します。
And once the skill becomes mature, Voyager stores a program into a skill library.
そして、そのスキルが成熟すると、Voyagerはスキルライブラリにプログラムを保存します。
You can think of it as a code library, altered entirely by trial and error by GPT-4.
それは、GPT-4によって完全に試行錯誤によって変更されたコードライブラリと考えることができます。
And then the agent can retrieve the skills from the library when it sees a similar situation in the future.
そして、エージェントは将来似た状況を見たときにライブラリからスキルを取り出すことができます。
And in this way, Voyager bootstraps its own capabilities as it explores and experiments in Minecraft.
そして、このようにして、Voyagerはマインクラフトで探索や実験をしながら自らの能力を引き上げていきます。
Let's quickly go through an example together.
一緒に例を早く見ていきましょう。
Here the agent's hunger bar has dropped very low, so it needs to find food.
ここでは、エージェントの空腹ゲージが非常に低くなっているので、食べ物を見つける必要があります。
And it senses four entities nearby, a cat, a villager, a pig, and some wheat seeds.
そして、近くに4つのエンティティが感知されます。猫、村人、豚、そして小麦の種があります。
It starts an inner monologue, right?
内なる独り言が始まりますね。
Do I kill the cat or the villager for food?
猫を殺すべきか、それとも村人を殺すべきか、食べ物を得るために。
That sounds like a bad idea.
そのは良くない考えのようですね。
How about the seeds?
種はどうですか?
I can grow a farm, but it's going to take too long.
農場を作ることはできますが、時間がかかりすぎます。
Really sorry, Piddy, you are the chosen one.
本当にごめんなさい、Piddy、あなたが選ばれし者です。
And then it checks the inventory and retrieves an old skill from the library to craft an iron sword, and then starts to learn a new skill called hunt pig.
そして、それからインベントリをチェックし、図書館から古いスキルを取り出して鉄の剣を作り、そして新しいスキルである猪を狩ることを学び始めます。
We also know that Voyager, unfortunately, isn't vegetarian.
残念ながら、Voyagerも菜食主義者ではないことを知っています。
The question still remains, how does Voyager keep exploring indefinitely?
依然として疑問が残っていますが、Voyagerはどのようにして無期限に探査を続けているのでしょうか?
We gave Voyager a high-level directive that is to obtain as many novel items as possible.
私たちはVoyagerに、可能な限り多くの新しいアイテムを入手するという高レベルの指令を与えました。
And Voyager implements a curriculum to find progressively harder and novel challenges to solve.
そして、Voyagerは段々難しく、新しい挑戦を解決するためのカリキュラムを実施します。
And putting all these together, Voyager is able to master and also discover new skills along the way.
これらすべてを組み合わせると、Voyagerは途中で新しいスキルを習得し、また発見することができます。
And we didn't pre-program any of this.
これらのいずれも事前にプログラムされていません。
What you see here is called lifelong learning, where an agent is forever curious and forever pursuing new adventures.
ここで見ているのは、エージェントが永遠に好奇心を持ち、新しい冒険を永遠に追求すると呼ばれる生涯学習です。
And these are two bird's-eye views of the Minecraft map.
これらはマインクラフトマップの鳥瞰図です。
The biggest orange circles are the distances that Voyager travels.
最も大きなオレンジ色の円は、ボイジャーが移動する距離です。
The agent explores so much because it has to move around to obtain as many novel items as possible.
エージェントはできるだけ多くの新しいアイテムを取得するために移動しなければならないため、多くを探索します。
And because it loves traveling, so that's why we call it Voyager.
そして、旅行が大好きなので、私たちはそれをボイジャーと呼んでいます。
Compared to mine clip, Voyager is able to pick up a lot more skills by itself, but it still knows how to control only one body in Minecraft.
マインクリップと比較すると、ボイジャーは自分で多くのスキルを身につけることができますが、それでもマインクラフト内での1つのボディのみを制御する方法を知っています。
But can we have a single model that works across different body forms, in terms of MetaMorph?
しかし、メタモルフの観点から、異なるボディ形態全体で機能する単一のモデルを持つことは可能でしょうか?
It is a project that I co-developed with Stanford researchers.
これは私がスタンフォードの研究者と共同開発したプロジェクトです。
We created a foundation model that works on not just one, but thousands of robots with different arm and leg configurations.
我々は、異なる腕や脚の構成を持つ何千ものロボットで動作する基礎モデルを作成しました。
And MetaMorph has no problem adapting to extremely varied kinematic characteristics of different bodies.
そして、メタモルフは、異なるボディの運動学的特性に非常に適応する問題はありません。
Here's the intuition.
ここに直感があります。
We developed a vocabulary to describe robot parts.
私たちは、ロボットの部品を記述する語彙を開発しました。
And then each body is basically a sentence written in a language of this vocabulary.
そして、それぞれのボディは、基本的にこの語彙の言語で書かれた文として表現されます。
And more specifically, each robot can be expressed as a graph of joints or kinematic tree.
そして、より具体的には、各ロボットは、関節のグラフまたは運動ツリーとして表現することができます。
And you can convert a body to a sequence of tokens by traversing this kinematic tree by a depth-first search.
そして、この運動ツリーを深さ優先探索で走査することで、ボディをトークンのシーケンスに変換することができます。
And each token here represents some physical properties of the joint, and the sequence describes the Morphology of the robot.
ここで、各トークンは関節のいくつかの物理的特性を表し、シーケンスはロボットの形態を記述します。
And different robots may have different numbers of joints and configurations, but the tokenizer doesn't care, right?
異なるロボットは異なる数の関節と構成を持つかもしれませんが、トークナイザーは気にしませんね?
It's all converted to sequences of different lengths, just like text strings.
すべてがテキスト文字列と同様に、異なる長さのシーケンスに変換されます。
And what do we do with sequences?
そして、シーケンスをどうするのでしょうか?
As AI researchers, our knee-jerk reaction is to apply a transformer, and that's exactly what we did.
AI研究者として、私たちの第一反応はトランスフォーマーを適用することであり、それが私たちが実際に行ったことです。
Instead of writing out text, MetaMorph writes out motor controls for each joint.
テキストを書き出す代わりに、MetaMorphは各関節のモーター制御を書き出します。
And because we want to learn a universal policy that works across Morphologies, we batch together all the robot sentences and train a big multitask neural network, just like ChaiGBT.
そして、私たちは形態学を横断して機能する普遍的なポリシーを学びたいので、すべてのロボットの文をまとめて、ChaiGBTと同様の大規模なマルチタスクニューラルネットワークを訓練します。
And no matter what a robot looks like, it's all the same.
そして、ロボットがどのように見えるかに関係なく、すべて同じです。
It's all just sentences to the eye of MetaMorph, and we can scale it up by training all the embodiments in parallel with reinforcement law.
MetaMorphの視点では、すべてがただの文であり、すべての具現を強化法と並行して訓練することでスケールアップできます。
And in our experiments, we showed that MetaMorph is able to control thousands of robots with extremely varied kinematic properties to walk upstairs, across irregular terrains, or avoid obstacles.
そして、私たちの実験では、MetaMorphが非常に多様な運動学的特性を持つ数千のロボットを制御して、階段を上ったり、不規則な地形を横断したり、障害物を避けたりすることができることを示しました。
And we also made a fascinating discovery.
そして、私たちは興味深い発見もしました。
We found that MetaMorph can even generalize zero-shot to a Morphology that has never seen before, which means that transformers are able to transfer across embodiments as long as they speak the right language.
MetaMorphは、以前に見たことのない形態学にゼロショットで一般化することさえできることがわかりました。つまり、トランスフォーマーは、適切な言語を話す限り、具現を横断して転送することができます。
And let's extrapolate a bit into the future.
そして、少し将来を推測してみましょう。
If we expand the robot body vocabulary even further, I envision that one day MetaMorph 2.0 can generalize to robot arms, dogs, different types of humanoids, and even beef.
もしロボットのボディ語彙をさらに拡大すれば、いつかMetaMorph 2.0がロボットアーム、犬、さまざまなタイプのヒューマノイド、さらには牛にも一般化できると思います。
Compared to Voyager, MetaMorph takes a big stride towards multi-body control.
一方、ボイジャーに比べて、メタモルフは多体制御に向けて大きな進歩を遂げています。
And it's now time to take things to the next level and transfer skills and bodies across realities, enters Isaac Sim, his simulation initiative.
そして、次の段階に進んで、スキルやボディを異なる現実に移す時が来ました。アイザック・シムが登場する、彼のシミュレーションイニシアチブです。
Isaac Sim's greatest strength is to run physics simulation at a thousand times faster than real time.
アイザック・シムの最大の強みは、物理シミュレーションを実時間の千倍速で実行できることです。
For example, this character learned impressive martial skills by going through 10 years worth of virtual training, only three days of simulation time on a GP.
例えば、このキャラクターは、仮想トレーニングで10年分の価値ある武道スキルを身につけ、GP上での3日間のシミュレーション時間だけでした。
It's very much like the virtual sparring dojo in the movie Matrix.
それはまさに、映画マトリックスの仮想スパーリング道場のようです。
And this race car scene is where simulation has crossed the Incai Valley, thanks to hardware accelerator ray tracing, we can render very complex worlds with breathtaking levels of details.
そして、このレースカーシーンは、シミュレーションがインカイ渓谷を越えた場面であり、ハードウェアアクセラレータのレイトレーシングのおかげで、息をのむような詳細レベルで非常に複雑な世界を描画することができます。
And the photorealism here can help us train computer vision models that will become the eyes of embodied agents.
そして、ここでの写実主義は、私たちが具現化されたエージェントの目となるコンピュータビジョンモデルを訓練するのに役立ちます。
And what's more, in Isaac Sim, we can procedurally generate infinite worlds and no two will look the same.
さらに、アイザック・シムでは、無限の世界を手続き的に生成することができ、どれも同じようには見えません。
Here's an interesting idea.
ここに興味深いアイデアがあります。
If the eight is trained on 10,000 different simulations, they may as well just generalize to our physical world, or the box, which is simply the 10,001st reality.
もし8が10,000の異なるシミュレーションで訓練されたら、我々の物理世界、または箱、つまり10,001番目の現実に一般化する可能性があります。
Let that sink in.
それをよく考えてみてください。
What new capabilities can Isaac Sim unlock?
Isaac Simはどんな新しい機能を解除できるのでしょうか?
This is Eureka, an agent that achieves robot dexterity at superhuman level.
これは、超人的なレベルでロボットの器用さを実現するエージェント、ユリカです。
Well, maybe not all humans, at least better than myself, because I have given up a pen spinning a long time ago since childhood, and finally I can have my AI avenge my poor skills.
まあ、少なくとも私よりは上手にできるかもしれません。なぜなら、私は子供の頃からペン回しを諦めてしまっていて、ついに私のAIに私の下手な技術を報復させることができるようになりました。
Here's the idea.
ここがアイデアです。
Isaac Sim has a Python to construct training environments, such as creating a five finger hand to interact with a pen in the simulation.
Isaac Simには、Pythonを使用してトレーニング環境を構築する能力があります。例えば、シミュレーション内でペンとやり取りするための5本指の手を作成するなどです。
We also assume that the human written code specifies a success criterion.
また、人間が書いたコードが成功基準を指定していると仮定します。
For example, if the pen reaches certain 3D orientations consistently, and this success criterion only tells you what to do, but not how to do it with the finger joints.
例えば、ペンが一貫して特定の3D方向に到達する場合、この成功基準はあなたに何をすべきかを教えてくれますが、指の関節をどのように動かすかまでは教えてくれません。
The first step of Eureka is to pass the environment code and task description as context to GPT-4.
ユリカの最初のステップは、環境コードとタスクの説明をGPT-4にコンテキストとして渡すことです。
And task here is to make the shadow hand spin a pen to a target orientation.
そして、ここでのタスクは、シャドーハンドがペンを目標の方向に回転させることです。
And then Eureka samples a reward function.
報酬関数をサンプリングします。
And it is a very fine grained signal that shapes the behavior of the neural network controller towards a good solution.
それは、ニューラルネットワークコントローラーの振る舞いを良い解決策に向かわせる非常に細かい信号です。
And normally expert human engineers will hand tune this reward function, which is often a very tedious and difficult process.
通常、専門の人間エンジニアがこの報酬関数を手動で調整しますが、これは非常に煩雑で難しいプロセスです。
It takes a lot of it and also a lot of expertise.
それには多くの時間と専門知識が必要です。
Not every engineer can do it if you're not familiar enough with the physics simulation.
物理シミュレーションに不慣れなら、すべてのエンジニアがそれを行うことはできません。
Let's automate it.
自動化しましょう。
Once we have a reward function, we can run reinforcement learning to maximize it through lots of trial and error.
報酬関数があれば、多くの試行錯誤を通じてそれを最大化するために強化学習を実行できます。
It only takes about 20 minutes to train a full run for Eureka for one of the reward functions instead of days, thanks to the massively parallel simulation in Isaac Sim.
Isaac Simの大規模並列シミュレーションのおかげで、報酬関数の1つのためにEurekaの完全な実行を訓練するのに数日かかる代わりに、約20分しかかかりません。
And when the training loop finishes, it returns an automated feedback report that tells Eureka how well that it does.
トレーニングループが終了すると、Eurekaにそのパフォーマンスについて教える自動フィードバックレポートが返されます。
And it also breaks it down to details like different components in the reward function, such as the velocity reward and the posture reward.
そして、報酬関数内の異なるコンポーネント、例えば速度報酬や姿勢報酬などの詳細に分解されます。
And putting it together, GPT-4 generates a bunch of reward function candidates and each perform a full reinforcement learning training run.
GPT-4は、報酬関数候補を生成し、それぞれが完全な強化学習トレーニングランを実行します。
Eureka would pass on the automated feedback and ask the language model to do a self-reflection on the results.
Eurekaは自動フィードバックを渡し、言語モデルに結果についての自己反省を求めます。
And then the language model will reason about where to improve and propose the next generation of reward function candidates and rinse and repeat.
そして、言語モデルは、どこを改善すべきかを推論し、次世代の報酬関数候補を提案して、繰り返し洗い流します。
It's kind of like an in-context evolutionary search.
それはまるで文脈の中で進化的な探索のようです。
And compared to expert humans, Eureka is able to find much better reward functions for each task, like spinning the pen along different axes.
専門家の人間と比較すると、Eurekaは、異なる軸を中心にペンを回転させるなど、各タスクに対してはるかに優れた報酬関数を見つけることができます。
They actually require different reward functions for each configuration to work well.
実際、それらはそれぞれの構成に対して異なる報酬関数を必要とします。
And that would be a nightmare for roboticists to just tune it one by one by hand.
それを手作業で1つずつ調整するのは、ロボティストにとって悪夢となるでしょう。
And trust me, I have tried it before.
信じてください、私も以前試したことがあります。
I wanted to pull my hair out.
私は髪を引き抜きたくなりました。
GPT-4 just has a lot more patience than any of us.
GPT-4は私たちよりもはるかに忍耐力があります。
It's worth noting that Eureka is a general purpose method that bridges the gap between high-level reasoning and low-level motor control.
Eurekaは高次の推論と低次のモーター制御の間のギャップを埋める汎用的な手法であるということに値する。
Eureka uses a new paradigm that I call hybrid gradient architecture, where a black box inference-only Large Language Model instructs a white box learnable neural network.
Eurekaは、私がハイブリッド勾配アーキテクチャと呼ぶ新しいパラダイムを使用しており、ブラックボックスの推論専用の大規模言語モデルがホワイトボックスの学習可能なニューラルネットワークに指示を出している。
The outer loop is gradient free and runs GPT-4 to refine the reward function in a coding space.
外側のループは勾配フリーであり、コーディング空間で報酬関数を洗練するためにGPT-4を実行している。
And the inner loop is gradient based and trains a reinforcement learning controller to achieve the skill that you want to do.
そして内側のループは勾配ベースであり、強化学習コントローラーを訓練して、行いたいスキルを達成する。
And you must have both loops to succeed.
そして成功するためには両方のループが必要です。
But the question is, why stop at just a reward function?
しかし、問題は、報酬関数で止まる理由は何かということですか?
If you stare hard enough, everything in the robotic stack looks like code, right?
もし十分にじっくり見つめれば、ロボティックスタック内のすべてがコードのように見えるでしょうね。
The task spec, robot hardware spec, and simulation environments themselves, all can be implemented by code, is that right?
タスク仕様、ロボットハードウェア仕様、そしてシミュレーション環境自体、すべてがコードで実装できる、そうですか?
For example, instead of MetaMorph's special language to describe the body, how about just using something off the shelf like URDF, which people typically use in simulation stacks.
たとえば、MetaMorphのボディを記述するための特別な言語ではなく、通常シミュレーションスタックで使用されるURDFのような市販品を使用することはどうでしょうか。
And URDF is nothing but an XML, which can describe the body Morphology for the robot.
そしてURDFはXMLにすぎず、ロボットのボディ形態を記述することができます。
In the future, I envision that Eureka++ can be a fully automated robotics developer, writing the infrastructure to train better agents and doing so iteratively.
将来、私はユーレカ++が完全に自動化されたロボティクス開発者になり、より良いエージェントを訓練するためのインフラを書き、それを反復的に行うことができると想像しています。
My dream is that one day I can take a very long vacation and Eureka will just keep reporting progress to me while I'm on the beach.
いつかとても長い休暇を取ることができ、ユーレカが私に進捗状況を報告し続けるような夢を見ています。
Let's see how far away is that?
それがどれだけ遠いか見てみましょうか?
And don't let Jensen know.
そして、ジェンセンには知らせないでください。
In this sense, Eureka isn't really a point on our map, but rather a force vector that can push the frontier along any axis.
この意味では、ユーレカは私たちの地図上の1点ではなく、どの軸に沿ってもフロンティアを押し進めることができる力のベクトルです。
And as we progress through the map, we will eventually reach a single model that generalizes across all three axes.
そして、私たちが地図を進むにつれて、最終的にはすべての軸にわたって一般化する単一のモデルに到達します。
And that upper right corner is the foundation agent.
そして、その右上の隅にはファウンデーションエージェントがいます。
I believe training foundation agents will be very similar to ChatGPT.
ファウンデーションエージェントのトレーニングはChatGPTと非常に似ていると信じています。
All language tasks can be expressed as texting and text out, be it writing poetry or doing translation or doing math.
すべての言語タスクは、詩を書くか、翻訳を行うか、数学を行うかに関係なく、テキストインとテキストアウトで表現できます。
It's all the same.
すべて同じです。
And training ChatGPT is simply scaling this up across lots and lots of text data.
ChatGPTのトレーニングは、たくさんのテキストデータを使ってこれを大規模に拡大しているだけです。
And very similar, the foundation agent will take as input an embodiment prompt, an instruction prompt and output actions.
そして非常に似ているのは、基礎エージェントが具現化プロンプトと指示プロンプトを入力として受け取り、アクションを出力することです。
And we simply scale it up massively across lots and lots of realities.
そして、私たちは単純に、たくさんの現実の中で大規模にスケールアップしています。
The foundation agent is the next chapter for GearLab.
基礎エージェントはGearLabの次の章です。
And yesterday Jensen announced Project GR00T in his keynote, a cornerstone initiative on our roadmap.
そして、昨日、ジェンスンは基調講演でプロジェクトGR00Tを発表し、当社のロードマップ上の基幹イニシアチブとなります。
The mission is to create a foundation model for humanoid roadmap.
ミッションは、人間型ロードマップのための基礎モデルを作成することです。
And why humanoid?
なぜ人間型なのか?
Because it is the most general form factor.
なぜなら、それが最も一般的な形状だからです。
Because the world that we live in is customized for humans and human habits.
私たちが生活している世界は、人間と人間の習慣に合わせてカスタマイズされているからです。
And everything that we can do in our daily lives can in principle be implemented on an advanced enough humanoid hardware.
そして、私たちが日常生活で行うことは、原則として、十分に高度な人間型ハードウェア上で実装できるすべてです。
I'm very excited to work with multiple leading humanoid companies around the world so that GR00T may transfer even crossing volumes.
世界中の複数の先導的なヒューマノイド企業と協力することにとても興奮しています。それによって、GR00Tはさらに広範囲にわたる転送が可能になります。
And this is one of my favorite pictures from our GTC preparation, taken in front of a headquarter.
これは私たちのGTC準備からのお気に入りの写真の1つで、本部の前で撮影されました。
Actually, the building behind that is called Voyager.
実際、その後ろにある建物はボイジャーと呼ばれています。
And here we see our Atronic, Fourier, Agility, and Unitree, and just look at how happy they are at a headquarter.
そしてここでは、本部でどれだけ幸せそうにしているかを見てみましょう。私たちのAtronic、Fourier、Agility、Unitreeがいます。
On a high level, GR00T takes multimodal instructions, such as language, video, and demonstration, and develops skills in simulation as well as the real world.
高いレベルで、GR00Tは言語、ビデオ、デモンストレーションなどのマルチモーダルな指示を受け取り、シミュレーションだけでなく実世界でもスキルを磨いています。
Here's an example of a video instruction.
こちらはビデオ指示の例です。
The GR1 robot here from Fourier Intelligence learns to mimic human dance moves from a video.
ここにあるFourier IntelligenceのGR1ロボットは、ビデオから人間のダンスの動きを模倣することを学んでいます。
And GR00T can also learn from human teleoperated demonstrations, such as Apollo's code press choosing skills.
そして、GR00TはApolloのコードプレス選択スキルなど、人間の遠隔操作デモンストレーションからも学ぶことができます。
For this demo, we actually bought a lot of fruit at GearLab.
このデモのために、実際にGearLabでたくさんの果物を買いました。
Got them all reimbursed.
全てが払い戻されました。
Thanks, Jensen.
ジェンセン、ありがとう。
And then the next one is from GR1 also playing the drum by following a human teacher's motion.
次に、GR1からのものも、人間の教師の動きに従ってドラムを演奏しています。
GR00T is born at Osmo, a new compute orchestration system to scale up models on DGX and simulation on OVX.
GR00Tは、DGX上のモデルをスケーリングし、OVX上のシミュレーションを行うための新しいコンピュートオーケストレーションシステムで生まれました。
And we use Isaac Lab to run lots of different environments for hoping that the model will generalize to a variety of skills and embodiments and transfer zero-shot across the sim-to-real gap so that we can scale up the training massively on fast simulation powered by GPUs.
そして、私たちはIsaac Labを使用して、さまざまな環境でたくさんの実行を行い、モデルがさまざまなスキルや具現化に一般化し、シミュレーションから実際のギャップをゼロショットで移行することを期待して、GPUによって駆動される高速シミュレーションでトレーニングを大規模にスケーリングアップすることができます。
Zooming out, I believe in a future where everything that moves will eventually be autonomous.
広く見渡すと、私はすべてが最終的に自律的になる未来を信じています。
And Project GR00T and Humanoid robots are only the first chapter.
そして、プロジェクトGR00Tとヒューマノイドロボットは、たった最初の章に過ぎません。
One day, we'll realize that the agents across Wall-E, Star Wars, and Ready Player One, no matter if they're in the virtual or in the physical world, will just be different prompts to the same foundation agent.
いつか、Wall-E、スターウォーズ、レディ・プレイヤー1を横断するエージェントが、仮想世界にいるか物理世界にいるかに関係なく、同じ基盤エージェントへの異なるプロンプトに過ぎないことに気づく日が来るでしょう。
And that, my friends, is Northstar on our quest for AGI.
そして、それが、友よ、AGIを求める私たちの北極星です。
And please join us.
そして、ぜひ参加してください。
Thanks, Jim, for that.
ジム、その情報をありがとう。
We're going to open up for Q&A here in the session.
このセッションでは、質疑応答の時間を設けます。
I'm over here.
こちらにいます。
If anyone has a question, they can please line up behind this mic and we'll just let them have it.
質問がある方は、このマイクの後ろに並んでいただければ、お答えします。
I really appreciate this talk here, Jim.
ジム、この話を本当に感謝しています。
And I'm excited about the stuff to come.
そして、これからのことにワクワクしています。
When I look at, say, something like Minecraft, you have your Voyager, which is using GPT-4 to get all this stuff.
例えば、マインクラフトのようなものを見ると、GPT-4を使用しているVoyagerがあります。
And then the opposite way of using, say, Dreamer V3 to do this stuff where it's learning completely from scratch using reinforcement learning, for this foundation agent, which of those two kind of tasks do you think is going to be, or maybe some combination of some?
そして、完全に強化学習を使用してゼロから学習しているDreamer V3のようなものを使用する逆の方法があります。これらの2つのタスクのうち、どちらがより重要だと思いますか、またはその組み合わせかという質問ですか?
I think that's a great question.
それは素晴らしい質問だと思います。
And I believe it has to be a kind of combination because you all have this separation between like system one and system two reasoning, and even humans have that.
そして、私はそれがある種の組み合わせである必要があると考えています。なぜなら、システム1とシステム2の推論の間には区別があり、人間でさえそうです。
System two reasoning is like this slow, deliberate, and high level reasoning.
システム2の推論は、このように遅く、慎重で高次の推論です。
And system one is more like fast, instantaneous, and like motor control.
システム1は、高速で瞬時で、モーター制御のようなものです。
And I think Eureka is one example, right?
ユーレカはその1つの例だと思いますね。
You have kind of a slow part of the brain that writes a reward function or someday writes kind of the, just the full simulation, all the environments.
脳の一部が報酬関数を書いたり、いつか完全なシミュレーション、すべての環境を書いたりする、という遅い部分があります。
And then you have a fast part of it using reinforcement learning that controls something like a dexterous hand, which is almost impossible to control directly by something like GPT-4, right?
そして、強化学習を使って何かを制御する、ほとんどGPT-4のようなものでは直接制御するのがほぼ不可能な、器用な手のようなものを制御するための高速な部分がありますね。
Like how can you control that hand using text only output?
テキストの出力だけを使ってその手をどうやって制御することができるでしょうか?
And it's also very slow.
それはまた非常に遅いです。
You have to do it at hundreds of Hertz.
数百ヘルツで行わなければなりません。
I think there's going to be this separation and they will also do inference at different frequencies, right?
このような分離があり、異なる頻度で推論も行うと思いますね。
Like the system two will do inference at a lower frequency and system one, much higher frequency.
システム2はより低い周波数で推論を行い、システム1ははるかに高い周波数で行います。
And I feel that's also how humans work as well.
おそらく、それが人間も働く仕方だと感じています。
We think about certain things, you kind of plan on a global level.
私たちは特定のことを考え、ある種のグローバルレベルで計画を立てる。
And then that planning propagates to your lips, and you don't really think when I pick up this bottle.
そしてその計画があなたの唇に伝播し、私がこのボトルを持ち上げるときには本当に考えていません。
You are not really thinking about exactly how you orient each finger and how you're feeling the tactile feedback.
各指をどのように配置し、触覚フィードバックを感じているかを正確に考えているわけではありません。
You don't, you don't think about it.
考えていないんです、考えていないんです。
And that is like another kind of low level neural network doing the job.
それは別の種類の低レベルのニューラルネットワークがその仕事をしているようなものです。
Hi, Jim.
こんにちは、ジム。
This is mind blowing.
これは驚くべきことです。
I have a question on the first part of when you had this mind link as the feedback, right in that framework, you call this a reinforced learning.
最初の部分について質問があります。この心のリンクをフィードバックとして持っていたとき、その枠組みでは、これを強化学習と呼んでいますね。
I have a doubt about whether this is anything related to the GAN framework where you use the mind link as direct feedback, good or bad, right?
私は、このことがGANフレームワークと何か関連があるのか疑問があります。心のリンクを直接フィードバックとして使用する場合、良いか悪いか、そういう意味で。
As a discriminator and then your generator generated actions.
お客様のジェネレーターが生成したアクションを識別するために。
Could you please clarify it?
それを明確にしていただけますか?
Yes, I think there are definitely connections here.
はい、ここには間違いなくつながりがあると思います。
I think the kind of a closer analogy would be to ROHF where you're doing kind of reinforcement learning from human feedback and a human feedback part is learned by human preferences.
より近いアナロジーは、ROHFで、人間のフィードバックからの強化学習を行っており、人間のフィードバック部分は人間の好みによって学習されています。
And here it's actually the same, except that the human preference is not labeled by us hiring contractors to do the job, but by learning from lots and lots of videos, because the gamers online, they're already narrating what they're doing.
ここでは、私たちが請負業者を雇って仕事をさせることで人間の好みをラベル付けしているのではなく、オンラインのゲーマーたちが既に自分たちが何をしているかを語っているので、たくさんのビデオから学ぶことで同じです。
You have this kind of match between the text and the video for free, and you can use this as a kind of signal to make sure that whatever the agent is doing, the video that it generates matches the text prompt by optimizing for this reward function.
テキストとビデオの間でこの種の一致が無料で提供され、エージェントが何をしているかに関して、生成されるビデオがテキストプロンプトに一致するようにするためにこの報酬関数を最適化することで、これを信号として使用できます。
I do think this functions a little bit like a discriminator, but the difference is now it is language conditioned.
私は、これが少し識別器のように機能していると思いますが、違いは今、言語条件付きであるということです。
It's a much more powerful kind of reward model, a much more powerful discriminator.
これは、より強力な種類の報酬モデルであり、より強力な識別器です。
Can you say that your discriminator is a language condition discriminator?
お客様の識別器は言語条件付きの識別器と言えますか?
I think so.
そうだと思います。
It's kind of a language condition ranking, right?
それは一種の言語条件ランキングですね。
Ranking if the video is or your actions are good or good or not.
ビデオやあなたの行動が良いかどうかをランク付けするものです。
It is a kind of discriminate.
それは一種の差別です。
I'm, I'm a researcher from UC Berkeley and I think this is great work.
私はUCバークレーの研究者で、これは素晴らしい仕事だと思います。
We do need GPU accelerator all the from simulation to real this process.
私たちは、シミュレーションから実際のプロセスまで、GPUアクセラレータが必要です。
My question is, what's NVIDIA's long-term take on this gear lab?
私の質問は、NVIDIAのこのギアラボに対する長期的な考え方は何ですか?
Do you guys want to do research and provide a accelerator infrastructure for researchers and industry to accelerate this embodied as in, or you guys aim to produce a high level solution to like humanoid robots in general?
あなたたちは研究を行い、研究者や産業向けにこの具体的なものを加速するためのインフラを提供したいのですか、それとも一般的なヒューマノイドロボットのような高度なソリューションを生産することを目指していますか?
That is a great question because I thought about this a lot at the founding of gear.
それは素晴らしい質問ですね、なぜなら私はギアの創設時にこれについて多く考えました。
The way I position gear is, three words, mission driven research.
私がギアを位置付ける方法は、3つの言葉で言うと、ミッション駆動型研究です。
I think gear fundamentally is still a research lab because unlike OMS, which do have a mature recipe now, robotics does not have a mature recipe and no one in the world really knows what's the best way to scale up robotics and actually have it generalized across system one and system two.
私はギアは根本的にはまだ研究所であると考えています。なぜなら、OMSとは異なり、成熟したレシピを持っているわけではなく、ロボティクスはまだ成熟したレシピを持っておらず、誰もが本当にどのようにロボティクスをスケールアップし、システム1とシステム2全体に一般化させるのが最善の方法かを知らないからです。
No one has figured that out yet.
まだ誰もそれを解決していません。
And by that it's by definition, like a research project, but at the same time, right?
それによって、それは定義上、研究プロジェクトのようなものですが、同時に、ですよね?
Like, Jensen announced not just GR00T this time, but a few things along with GR00T, one is Osmo, which I also mentioned in my slides here.
ジェンセンは今回、GR00Tだけでなく、いくつかのものを発表しましたが、そのうちの1つが私のスライドで言及したOsmoです。
It is this compute orchestration system, as like a heterogeneous compute framework to schedule DGX and OVX one for training large models, one for simulation, right?
これは、DGXとOVXをスケジュールするための異種コンピュートフレームワークとしての、大規模モデルのトレーニング用とシミュレーション用のものですね。
Osmo comes with GR00T because GR00T requires this kind of very special infrastructure to do.
OsmoはGR00Tと一緒に提供されます。なぜなら、GR00Tにはこれらの非常に特別なインフラが必要だからです。
And for OMS, you don't have this problem.
そしてOMSにはこの問題はありません。
You don't have a simulator, but once you have a simulator, the computation graph becomes very complicated and you will need something like Osmo, which can be offered as a cloud service.
シミュレーターがないので、シミュレーションがあると、計算グラフが非常に複雑になり、トレーニング大規模モデルやシミュレーションにはOsmoのようなものが必要になります。それはクラウドサービスとして提供されることができます。
And then, Jensen and Thor, which one day will power GR00T on edge computing devices or on every humanoids ever deployed.
そして、ジェンセンとソーは、いつかグルートをエッジコンピューティングデバイスや展開されたすべてのヒューマノイドに動力を供給するでしょう。
It's really an ecosystem that we're doing here.
ここで私たちが行っているのは本当にエコシステムです。
And I see GR00T as kind of playing a fundamental role in this ecosystem where you need a foundation model that's actually working to make the humanoid robots useful, right?
そして、私はグルートをこのエコシステムの中で基本的な役割を果たす存在と見ています。ここでは、実際に機能している基礎モデルが必要です。これにより、ヒューマノイドロボットが有用になりますね。
Like right now, humanoid robots, they're more of a curiosity.
今のところ、ヒューマノイドロボットは、単なる興味の対象に過ぎません。
They're not useful.
役に立ちません。
Like no one really has at their home, a humanoid that can do all their dirty housework for them, which is by the way, my dream, but still, I'm, I'm, I'm trying, I'm very lazy and I'm trying to make sure I will stay lazy, right?
例えば、誰もが自宅に、自分の代わりに全ての汚れ仕事をしてくれるヒューマノイドを持っているわけではありません。それが私の夢なのですが、それでも、私は、私は、私は、怠惰であり、怠惰でい続けるように努めていますね。
That's what I've been researching on, but no humanoids work on that level yet.
それが私が研究していることですが、まだそのレベルで動作するヒューマノイドは存在しません。
We first need to make sure that these robots work.
まず、これらのロボットが動作することを確認する必要があります。
And then we can deploy them, and then we can deploy them massive, and we can ship compute with these models.
そして、それからそれらを展開し、そして、それらを大量に展開し、これらのモデルとともにコンピュートを出荷することができます。
We can ship compute infrastructure with these models.
これらのモデルとともにコンピュートインフラストラクチャを出荷することができます。
We can even open up APIs for people to deploy, to customize GR00T on their own robots, but it's not there yet.
私たちは人々が自分自身のロボットにGR00TをカスタマイズするためにAPIを公開することさえできますが、まだそこには至っていません。
It's more like mission-driven research.
それはむしろミッションに基づいた研究です。
One more quick question.
もう1つ質問があります。
Yeah, I see Jason mentioned that you guys partner with some of the bigger robotics company, right?
はい、Jasonが言及しているように、あなたたちはいくつかの大手ロボティクス企業と提携しているんですよね?
What about like startup companies or research groups?
スタートアップ企業や研究グループはどうですか?
Do you guys foresee having some collaboration with them?
あなたたちは彼らとの協力を予定していますか?
Many of the humanoid companies are startups by themselves.
多くのヒューマノイド企業は自らがスタートアップです。
And of course, we welcome researchers and students like yourself to join.
もちろん、あなたのような研究者や学生が参加することを歓迎します。
You have to link here, do a little bit hiring, you have to link here.
こちらにリンクする必要があります、少し採用を行う必要があります、こちらにリンクする必要があります。
Please feel free to apply and I would love all the best talents in the world to join gear and work with us on this moonshot.
お気軽にご応募ください、世界中の最高の才能がギアに参加し、この大胆な計画に取り組むことを願っています。
I mean, just clarify my question.
つまり、私の質問を明確にしてください。
Like for a research lab to embrace this infrastructure, do you think that's something you guys looking for?
研究所がこのインフラを取り入れることについて、あなたたちが探しているものだと思いますか?
You mean like kind of partnering with like research?
研究機関との提携のようなものを意味しますか?
Yeah, like we use the infrastructure to accelerate the robotics research.
はい、インフラを使用してロボティクスの研究を加速させるということです。
I think that is more case by case because for the humanoid robots themselves, like the hardware isn't super widely available yet.
それはケースバイケースだと思います、なぜなら、ヒューマノイドロボット自体、ハードウェアがまだ広く利用可能ではないからです。
But I'm open to talks.
しかし、話し合いには開かれています。
Hi, Jim.
こんにちは、ジム。
First of all, even I'm waiting for the robot that come and clean the house.
まず第一に、私も家を掃除してくれるロボットを待っています。
Looking forward to it.
楽しみにしています。
And I'm going to ask you a very different question.
そして、非常に異なる質問をしますね。
I work a lot with the school district and the high school students as one of my passion projects, and I see continuously the divide between what students are taught and what the workforce is really needing and with this whole AI and robotics, the divide increasing like leapfrogging.
私は学区や高校生と一緒に働くことが多く、情熱プロジェクトの一つとして、学生が教わっていることと実際の職場が本当に必要としていることの間にある隔たりを継続的に見ています。そして、このAIやロボティクス全体において、その隔たりが飛躍的に広がっているのを見ています。
What recommendation do you have for the collaboration of the tech industry with the schools and how can, what schools can do to prepare the students?
技術産業と学校の協力についてどのようなお勧めがありますか?学校はどのようにして生徒を準備できるのでしょうか?
And I'm not talking even at the college level, I'm more talking about specifically the four years of high school students.
そして、大学レベルでも話していません、具体的には高校生の4年間について話しています。
And, I can see a lot and lots of confusion and I bring a lot of sessions and conferences to them as well and love to invite you as well.
私は多くの混乱を見ており、彼らにセッションやカンファレンスをたくさん提供しています。そして、あなたも招待したいと思っています。
I think for kind of, like high school students, or like education in general, I feel that one thing good about AI right now is that the barrier of entry has been lowered significantly, right?
一般的に、高校生や教育に関しては、現在のAIの良い点の1つは、参入の障壁が大幅に低下していると感じていますね。
Like any student, middle school student, whoever can just register for an LOM account.
どんな学生でも、中学生でも、簡単にLOMアカウントに登録できます。
And then start using this API and build agents, right?
そして、このAPIを使ってエージェントを構築し始めることができますね。
They can actually reproduce Voyager without using much funding.
彼らは実際に多くの資金を使わずにボイジャーを再現することができます。
We have the code open source.
私たちはコードをオープンソースにしています。
You can connect it to NVIDIA's LOM API.
お客様はNVIDIAのLOM APIに接続することができます。
You can connect it to OpenAI's API.
お客様はOpenAIのAPIに接続することができます。
It's all very accessible and doesn't cost too much.
それは非常にアクセスしやすく、あまり費用がかかりません。
I think like the barrier of entry is lowered to an unprecedented skill.
私は、参入の障壁が前例のないスキルに低下したと思います。
When I, when I was a high school student, I did not even have access to computer science classes.
私が高校生だったとき、コンピュータサイエンスの授業にすらアクセスできませんでした。
I wrote my first line of code when I was a college student.
私は大学生のときに最初のコードを書きました。
And I feel that these days things have changed and I would love to be young again and start kind of building from middle school using LOM APIs.
そして、最近は状況が変わったと感じており、もう一度若くなってLOM APIを使って中学校から構築を始めたいと思っています。
That's just the coolest time.
それはただ最高の時期です。
And I'd love to have you over and with the students to learn from you.
そして、生徒たちと一緒にあなたから学びたいと思っています。
Hi, Jim.
こんにちは、ジムさん。
Thank you for the presentation.
プレゼンをしていただきありがとうございます。
My question is about the physics in the beginning of your presentation.
私の質問は、あなたのプレゼンの最初の部分の物理学についてです。
You mentioned that one of the features of the agent is to the knowledge of the world.
あなたは、エージェントの特徴の1つが世界の知識であることを述べました。
Could you elaborate on how, how would you go into learn the physics, from, from this training?
このトレーニングから物理学を学ぶ方法について詳しく説明していただけますか?
Because essentially you explained to us that the task, planning action can be in, in bolded into something like a GPT, but, but the fix is very different.
基本的に、あなたは、タスク、計画、アクションがGPTのようなものに太字で示されることができると説明しましたが、その修正は非常に異なります。
If you could elaborate on that.
それについて詳しく説明していただければと思います。
I think that is a very deep question.
それは非常に深い質問だと思います。
I don't think we have a, we have a very clear answer to it, but this is my take, so I think training on lots and lots of videos, and if you do a very good job at scaling up and doing it right, the model will be able to learn some kind of physics and we call that intuitive physics and that's what humans do as well.
おそらくそれには明確な答えがないと思いますが、これは私の考えです。ですので、たくさんのビデオを使ってトレーニングを行い、スケーリングアップして正しく行うと、モデルはある種の物理学を学ぶことができると思います。それを直感的な物理学と呼び、それは人間も同じです。
Like when we are going about our daily lives, we don't compute differential equations and accurate physics in our mind.
私たちが日常生活を送る際、私たちは微分方程式や正確な物理学を頭の中で計算しているわけではありません。
If I spill this water right now, I have no idea, right.
もし私が今この水をこぼしたら、全くわかりませんね。
How each water molecule is going to move, right.
各水分子がどのように動くか、ですね。
I'm not computing that, but I will.
私はそれを計算していませんが、します。
No, that I will make a mess and then Nathan over there will be very mad at me.
おっしゃる通り、私が散らかすと、向こうのネイサンは私にとても怒るでしょう。
That is intuitive physics.
それは直感的な物理学です。
I know roughly what the consequences will be given my actions.
私は、私の行動によって結果がどのようになるか、おおよそわかっています。
I think models train on lots and lots of videos.
私は、モデルがたくさんのビデオで訓練されると思います。
Let's say a predictive model like Sora, if you're predicting the future, and if you're doing a good job at doing that, that means you have to implement some kind of implicit intuitive physics engine in order to generalize, right.
未来を予測するような予測モデル、例えばSoraのような場合、もし未来を予測するのがうまくいっているなら、一般化するために何らかの暗黙の直感的な物理エンジンを実装しなければならないということですね。
You need to understand that when you drop a glass of water, it's gonna, it's gonna break, right.
グラスを落とすと、割れることを理解する必要がありますね。
Like these kinds of abstractions.
このような抽象的なものが好きです。
And I think like, if you are using these models for accurate physics, I don't think they're going to work.
そして、これらのモデルを正確な物理学に使用している場合、それらはうまく機能しないと思います。
But if you're using these models for robotics, I think it might be just the right data because for the robots, they don't need to compute exactly right. All the water molecules, they just need to operate like humans, having intuitive understanding of how the world works and also learning kind of cause and effect from it, right, like physics is also kind of causal reason.
しかし、これらのモデルをロボティクスに使用している場合、ロボットにとってはちょうど適切なデータかもしれません。ロボットにとっては、正確に計算する必要はありません。水分子全てを、彼らは単に人間のように操作する必要があります。世界がどのように機能するかについての直感的な理解を持ち、またそれから原因と結果を学ぶ必要があります。物理学もまた因果的な理由の一種です。
That's how I see kind of videos and these types of models will help robotics.
私が考えるに、この種のビデオやモデルはロボティクスに役立つでしょう。
Hi, Jim.
こんにちは、ジム。
Thank you for the presentation.
プレゼンテーションありがとうございます。
Just a couple of questions on your work that is inspiring us.
私たちを刺激してくれるあなたの仕事に関するいくつかの質問があります。
The question is more related to your hybrid gradient, let's say framework is more for me, like sim is to the reward-free framework that is in the literature, how can you ensure that Eureka will discover skills that are different between the others?
質問は、あなたのハイブリッド勾配、つまり枠組みが、文献にある報酬フリーの枠組みに似ているという点に関連しています。Eurekaが他と異なるスキルを発見することをどのように保証できますか?
Sorry, do you mind saying again, how, how Eureka can help in finding new skills, different skills between them in order to explore better, to find new possibilities.
すみません、もう一度言ってもらってもいいですか。Eurekaが新しいスキル、異なるスキルを見つけるのにどのように役立つのか、新しい可能性を見つけるために。
Find new possibilities.
新しい可能性を見つけてください。
I think the capability of Eureka will also in some sense be determined by the foundation model itself.
ユーレカの能力は、ある意味では基本モデル自体によっても決定されると思います。
Eureka was built on GPT-4 and it was quite a while back.
ユーレカはGPT-4上に構築され、かなり前になります。
I think GPT-4 itself has been improved and there's also like Gemini models and also cloud models.
GPT-4自体が改良されており、Geminiモデルやクラウドモデルもあります。
Whichever model is more kind of creative and more diverse, Eureka will just inherit that from the underlying model.
どのモデルがより創造的で多様性に富んでいるかによって、ユーレカは基礎モデルからそれを受け継ぐだけです。
If the model itself doesn't have a lot of diversity, then it might just get stuck in some local minimal and not be able to propose new solutions, but at least in our particular experiments in the paper where we do like dexterous manipulation tasks for those tasks, I think Eureka is doing a really good job in kind of searching the function space.
モデル自体が多様性に乏しい場合、局所的な最小値にはまって新しい解決策を提案できないかもしれませんが、少なくとも私たちが行った特定の実験では、器用な操作タスクにおいて、ユーレカは関数空間を探索するのに非常に優れた仕事をしていると思います。
And actually, we have a chart in the paper where we show that the reward functions that it engineers is actually better than what human engineers can come up with.
実際、私たちは論文にチャートを掲載しており、それによると、ユーレカが設計する報酬関数は、人間のエンジニアが考えつくよりも優れていることを示しています。
And as I said, like human engineers have to do trial and error and it's kind of a nightmare to do.
そして、人間のエンジニアは試行錯誤をしなければならず、それはやるのが大変です。
Eureka automates that and does a better job, but it doesn't necessarily mean it can excel in every domain.
ユーレカはそれを自動化し、より良い仕事をしますが、それがすべての領域で優れることを意味するわけではありません。
It really depends on the LLM.
それは大規模言語モデルによって本当に異なります。
Hi, James.
こんにちは、ジェームズ。
Thank you for sharing about your mission-driven research.
使命に忠実な研究について共有してくれてありがとう。
I'm David from Columbia Business School.
私はコロンビア・ビジネススクールのデイビッドです。
May I know what do you think about the obvious challenge for your research from the JR lab to the real world?
あなたの研究におけるJRラボから実世界への明らかな課題についてどう思われますか?
Your question is about transferring kind of the research.
あなたの質問は、ある種の研究の移行についてです。
What do you think will be the biggest challenge and what do you think will be the next step you're focused on?
最大の課題は何だと思いますか?そして次に焦点を当てるのは何だと思いますか?
The challenges, I think CM2Real is really hard.
課題は、私はCM2Realが本当に難しいと思います。
I do believe that if you train in 10,000 simulations and if you do well in all of them, you will have a very good chance to transfer to a real world.
私は、もし1万回のシミュレーションでトレーニングを受け、それらすべてでうまくいけば、実世界に移行する可能性が非常に高いと信じています。
But in reality, it isn't always that simple, right?
しかし、実際には、それはいつもそんなに簡単ではないですよね?
It's, it depends on many things.
それは、多くの要因に依存します。
One is the fidelity of the simulation.
1つはシミュレーションの忠実度です。
You still want the simulation to be as accurate as possible, or at least not to make systematic mistakes in some critical areas.
シミュレーションができるだけ正確であること、または少なくともいくつかの重要な領域で系統的なミスをしないようにしたいです。
And also the robot hardware itself can fail, right?
そして、ロボットのハードウェア自体が故障する可能性もありますよね?
And the CM2Real, the software can have bugs.
CM2Real、ソフトウェアにバグがある可能性もあります。
There are many places that could go wrong.
間違う可能性がある場所はたくさんあります。
But so far we have, and also in the past works from NVIDIA research, we have a lot of success in CM2Real where we do something called domain randomization.
しかし、これまでに、そしてNVIDIAリサーチの過去の作品でも、私たちはドメインランダム化という手法を用いてCM2Realで多くの成功を収めてきました。
Like you instantiate 10,000 simulations and each one has slightly different physics coefficients, like different gravity, different frictions.
たとえば、10,000のシミュレーションをインスタンス化し、それぞれがわずかに異なる物理係数を持っているようなものです。異なる重力、異なる摩擦などです。
And if your model is robust to all of these variations, then it just generalizes on a box through real world, because the real world's gravity and coefficient, even though you don't know them exactly, they could be off by a little bit, but your model is robust to a distribution of them.
もしモデルがこれらすべての変動に強いとすれば、実際の世界を通して一般化されるので、実際の世界の重力や係数は正確にはわからなくても、少しズレているかもしれませんが、モデルはそれらの分布に対して頑健です。
The real world is actually in distribution of your simulation and you can generalize.
実際の世界は実際にはシミュレーションの分布内にあり、一般化できます。
But, that will be the ideal case and it doesn't always happen.
しかし、それは理想的なケースであり、常に起こるわけではありません。
I do see CM2Real as a critical challenge here.
私はここでCM2Realを重要な課題と見なしています。
That's one thing.
それが一つ。
And the second thing is no one has figured out how to solve robotics yet.
そして二つ目は、誰もがロボティクスを解決する方法を見つけ出していないということです。
If someone told you they have solved robotics take it with a grain of salt, I don't believe that at all of this moment, anyone has figured that out.
もし誰かがロボティクスを解決したと言ったら、それは疑ってかかってください。私は今のところ、誰もがそれを解決したとは信じていません。
One critical problem of robotics and why is it difficult is the data, right?
ロボティクスの一つの重要な問題、なぜ難しいのかというと、データですね。
For ChatGPT, as I said, like you can scrape lots and lots of internet text and you just scale up on that.
ChatGPTに関しては、私が言ったように、たくさんのインターネットテキストをスクレイピングして、それをスケールアップすることができます。
But how do you scrape robot control data from the internet?
しかし、インターネットからロボット制御データをどのようにスクレイピングするのでしょうか?
It does not exist.
それは存在しません。
And that's just one simple reason why robotics is so much harder than something like GBT4, right?
それがロボティクスがGBT4のようなものよりもはるかに難しい理由の1つであるという単純な理由ですね?
How do you collect the data in this way?
このようにデータを収集する方法はどうですか?
From our kind of gear labs roadmap, we're thinking about a combination of data, right?
私たちの種類のギアラボのロードマップから、私たちはデータの組み合わせを考えていますね。
You need internet data, you need simulation data, and you for sure also need real, also need real robot data and each different sources of data have complementary strengths and weaknesses, right?
インターネットデータが必要で、シミュレーションデータが必要で、確かに実際のロボットデータも必要で、そしてそれぞれの異なるデータソースには補完的な強みと弱みがありますね。
It's so much more complicated than just training, like language models, because you only have internet data, right?
言語モデルのようなトレーニングだけよりもはるかに複雑です、なぜならインターネットデータしか持っていないからですね。
You don't have the other two sources of data to worry about, but for robotics, you need to care about kind of the whole system.
他の2つのデータソースを心配する必要はありませんが、ロボティクスでは全体のシステムに注意を払う必要があります。
The data problem is a second critical challenge in addition to Sim2Real that I am seeing, and the third is, how to scale up.
データの問題は、私が見ているSim2Realに加えて、2番目の重要な課題であり、3番目は、どのようにスケールアップするかです。
And that's still tied to data problem, right?
それはまだデータの問題に関連していますね。
But like, even if you have all the videos on the internet, what do you learn from it?
しかし、たとえインターネット上のすべてのビデオを持っていても、そこから何を学ぶのでしょうか?
Do you predict the next frame?
次のフレームを予測しますか?
And after you, so let's say, even if you have a Sora model, how do you apply Sora model to a robotic stack?
そしてあなたの後に、たとえあなたがSoraモデルを持っていても、Soraモデルをロボットスタックに適用する方法はどのようなものですか?
It's actually not clear at all.
実際、全く明確ではありません。
Why?
なぜですか?
Because Sora model doesn't have action.
なぜなら、Soraモデルにはアクションがないからです。
It doesn't come with actions.
アクションは含まれていません。
It's text to video, but you want the actions out of it.
それはテキストからビデオへの変換ですが、アクションを取り出したいと思っています。
And actions are really hard.
そしてアクションは本当に難しいです。
Especially if you have dexterous hands in a humanoid, the actions are really hard.
特に、ヒューマノイドに器用な手がある場合、アクションは本当に難しいです。
Even if you have all the computing in the world, you have all the data in the world, how do you extract the signals of embodied agents from it is an unsolved problem.
世界中のすべてのコンピューティングを持っていても、世界中のすべてのデータを持っていても、具現化されたエージェントの信号をどのように抽出するかは未解決の問題です。
That's why I said gear is a mission-driven research because there are so many things, but it is a critical mission that we cannot delay.
そのため、私はギアがミッション駆動型の研究だと言ったのです。たくさんのことがあるけれども、遅延することができない重要な使命なのです。
Sorry about that.
申し訳ありません。
That's, that was our last question.
それが、最後の質問でした。
Could we give everyone here can give Jim a round of applause for that.
ここにいる皆さんがジムに拍手を送っていただけますか。
この記事が気に入ったらサポートをしてみませんか?