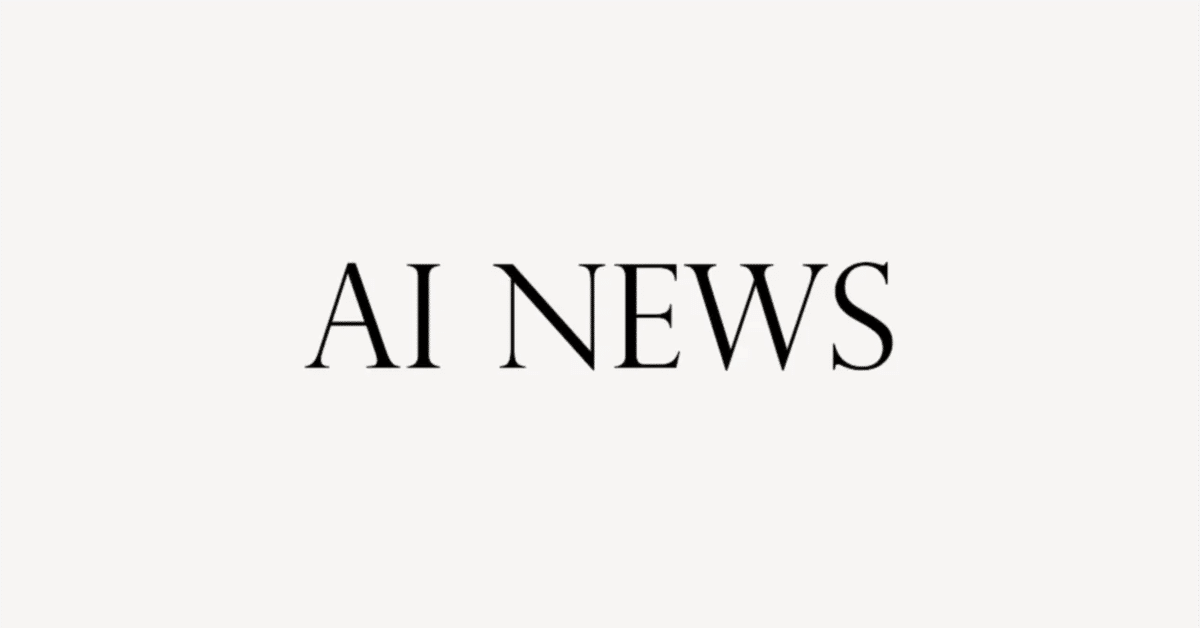
【Voyager:マインクラフトを学ぶAI】英語解説を日本語で読む【2024年2月1日|@TheAIGRID】
この動画は、NVIDIAのシニアリサーチサイエンティストであるジム・ファンのTED Talkを紹介しています。彼は「Foundation Agent」というAIエージェントについて語り、これは仮想世界と現実世界の両方で活動できるAIの新しい概念です。このAIエージェントは、ビデオゲームからロボットまで様々な分野で活躍し、多様なスキルを習得する能力を持っています。特に、「Voyager」というマインクラフトを学ぶAIエージェントについて詳述されています。VoyagerはYouTubeのゲームプレイ動画から学び、自己改善メカニズムを通じて新たなスキルを習得し、自己進化を遂げることが可能です。さらに、ジム・ファンはFoundation Agentの将来的な発展についても言及しています。これには、YouTubeのプレイ動画からデータセットを作成し、AIが行動を学習する方法や、エージェント間の相互作用を通じたタスク達成の研究が含まれます。
公開日:2024年2月1日
※動画を再生してから読むのがオススメです。
So there was a recent TED Talk that actually talked about AI agents, and it was a very fascinating TED Talk presented by the Senior Research Scientist at NVIDIA and the Lead of AI Agents Initiative.
最近のTED Talkでは、AIエージェントについて話されていて、NVIDIAのシニアリサーチサイエンティストであり、AIエージェントイニシアチブのリードを務める方によって非常に魅力的なトークが行われました。
So this is Jim Fan, the Senior Research Scientist at NVIDIA AI, and in this fascinating talk, he gives us a breakdown of where the future is headed with AI agents.
これはNVIDIA AIのシニアリサーチサイエンティストであるジム・ファン氏であり、この魅力的なトークでは、AIエージェントが将来どのような方向に進んでいるのかを詳しく説明しています。
He talks about something called the Foundation Agent, which would essentially seamlessly operate across both the virtual and physical worlds, and he explains how this technology could fundamentally change our lives, permeating everything from video games and metaverse to drones and humanoid robots, and explores how a single model could master these skills across different realities.
彼は「Foundation Agent」というものについて話しており、これは仮想世界と物理世界の両方でシームレスに操作できるものであり、この技術が私たちの生活を根本的に変える可能性があると説明しています。ビデオゲームやメタバースからドローンやヒューマノイドロボットまで、さまざまな領域に浸透し、単一のモデルが異なる現実でこれらのスキルを習得する方法を探求しています。
Now, this Foundation Agent is not to be confused with AGI itself because AGI refers to a level of artificial intelligence where a machine can understand, learn, and apply its intelligence to solve any problem in a manner comparable to a human across a wide range of domains.
ただし、「Foundation Agent」とAGI自体を混同しないでください。AGIは、機械が広範なドメインで人間と同様に問題を理解し、学習し、知識を適用することができる人工知能のレベルを指します。
Now, this idea of the Foundation Agent seems to be about creating a versatile, multi-functional AI that can operate both in virtual and physical environments, mastering skills in various realities.
「Foundation Agent」というアイデアは、仮想環境と物理環境の両方で操作できる多機能なAIを作り出すことに関するもののようです。さまざまな現実でスキルを習得することができます。
Now, in this video, I was lucky enough to be part of a private discussion with Jim Fan himself where he discussed the real future of Foundation Agents and discussed some of the research papers that he worked on, which are going to help contribute towards the development of the future research and development of the Foundation Agent and the industry as a whole.
このビデオでは、私はジム・ファン氏自身との非公開のディスカッションに参加する機会を得ました。彼はFoundation Agentの真の未来について話し、彼が取り組んだいくつかの研究論文についても議論しました。これらの研究論文は、将来のFoundation Agentの研究開発と産業全体の発展に貢献することになります。
So I'm going to show you guys just a few seconds from his TED Talk because it is one that shouldn't be missed at all, especially if you want to stay up to date on where everything is headed in AI, and then I'll share with you guys the conversation that we had about AI Agents and some of the papers that Jim Fan worked on himself.
では、彼のTED Talkから数秒間だけご紹介します。AIの進展について最新情報を知りたい場合には、ぜひ見逃さないでください。そして、AIエージェントやジム・ファン氏自身が取り組んだいくつかの論文についての私たちの会話も共有します。
As we progress through this map, we will eventually get to the upper right corner, which is a single agent that journal across all three taxes.
この地図を進んでいくと、最終的には上右の角にたどり着きます。それは、すべての3つの税金を横断する単一のエージェントです。
And that is the Foundation Agent.
そしてそれが、Foundation Agentです。
I believe training Foundation Agent will be very similar to ChatGPT.
私は、Foundation AgentのトレーニングはChatGPTと非常に似ていると考えています。
All language tasks can be expressed as text in and text out.
すべての言語タスクは、テキストの入力とテキストの出力として表現することができます。
Be it writing poetry, translating English to Spanish, or coding Python, it's all the same.
詩を書くこと、英語からスペイン語への翻訳、Pythonのコーディングなど、すべて同じです。
And ChatGPT simply scales this up massively across lots and lots of data.
そして、ChatGPTは、大量のデータを使ってこれを大規模に拡大します。
It's the same principle.
同じ原則です。
The Foundation Agent takes as input an embodiment prompt and a task prompt and output actions, and we train it by simply scaling it up massively across lots and lots of realities.
Foundation Agentは具現化プロンプトとタスクプロンプトを入力とし、アクションを出力します。そして、私たちはたくさんの現実にわたってそれを大規模にスケーリングすることでトレーニングします。
Yes, so the first work I want to cover is Voyager, and Voyager was one of the first AI Agents that can play Minecraft professionally.
まずカバーしたい作品はVoyagerです。Voyagerは、プロのマインクラフトプレイヤーとして活躍できる最初のAIエージェントの一つでした。
So I suppose most of you are familiar with Minecraft.
だから、おそらく皆さんはマインクラフトに詳しいと思います。
It's got like 140 million active players.
アクティブなプレイヤーは約1億4000万人います。
That's more than twice the population of the UK.
それはイギリスの人口の2倍以上です。
So it's kind of insanely popular and beloved game, and it's open-ended, doesn't have a fixed storyline.
それは非常に人気のある愛されているゲームであり、ストーリーが固定されていません。
You can do whatever your heart desires in the game.
ゲーム内で心のままに何でもできます。
So we want an AI to have the same capabilities.
だから、AIにも同じ能力を持たせたいのです。
And when we set Voyager loose in Minecraft, it's able to play the game for hours on end without any human intervention.
そして、Voyagerをマインクラフトに解放すると、人間の介入なしで何時間もゲームをプレイすることができます。
So the video here actually shows snippets from a single episode of Voyager.
この動画は実際にはVoyagerの一つのエピソードからの断片です。
This is just a single run that lasted for like four to five hours, and we took some of the segments out and made this montage.
これは4〜5時間続いた単一のプレイであり、その一部を取り出してこのモンタージュを作りました。
So you see that Voyager explores the terrains, mine all kinds of materials, fight monsters, craft hundreds of recipes, and it's able to unlock an ever-expanding free of skills.
だから、Voyagerが地形を探索し、さまざまな素材を採掘し、モンスターと戦い、数百のレシピを作り、ますます広がるスキルを開放する様子がわかります。
What is magic behind it?
それの魔法は何でしょうか?
The key insight is coding as action.
鍵となる洞察は、コーディングをアクションとして扱うことです。
You know, Minecraft is a 3D world, but our most powerful OMS, at least at the time of Voyager's writing, was GPT-4, and it was text only.
マインクラフトは3Dの世界ですが、私たちの最も強力なOMS、少なくともVoyagerの作成時点ではGPT-4はテキストのみでした。
So we need a way to convert a 3D world into a textual representation, and thanks to the very enthusiastic Minecraft community, we actually have an open-source JavaScript API that we can use.
だから、3Dの世界をテキスト表現に変換する方法が必要でしたが、非常に熱心なマインクラフトコミュニティのおかげで、私たちは実際に使用できるオープンソースのJavaScript APIを持っています。
It's called Mind Flayer.
それはMind Flayerと呼ばれています。
So we use this code API.
だから、私たちはこのコードAPIを使用します。
And then, Voyager is an algorithm designed on top of GPT-4.
そして、VoyagerはGPT-4の上に設計されたアルゴリズムです。
So, the way it does it is to invoke GPT-4 to generate a code snippet in JavaScript.
それが行う方法は、GPT-4を呼び出してJavaScriptのコードスニペットを生成することです。
Each snippet is an executable skill in the game.
各スニペットはゲーム内で実行可能なスキルです。
And then, once it writes the code, it will be run in actual game runtime.
そして、コードを書いた後、実際のゲームランタイムで実行されます。
And just like human engineers, the program that Voyager writes isn't always correct.
そして、Voyagerが書くプログラムは常に正しいわけではありません。
So, we have a self-reflection mechanism to help it improve.
だから、改善するための自己反省のメカニズムがあります。
And more specifically, there are three different sources of self-reflection.
具体的には、自己反省の3つの異なる源があります。
One is JavaScript execution error, where you have the agent's current state like hunger, health, and inventory, or the world state like escape, resources, enemies nearby are fed to Voyager from the agent's state.
1つはJavaScriptの実行エラーであり、エージェントの現在の状態(空腹、体力、インベントリ)や世界の状態(逃走、資源、近くの敵)がエージェントの状態からVoyagerに提供されます。
And then, given the state, the agent will take an action and then observe the consequence of the action on the world and on itself, reflect on how it could do better, trial more actions and rings, and repeat.
そして、状態を元に、エージェントはアクションを実行し、そのアクションの結果を世界と自身に観察し、より良い方法を考え、さらにアクションと試行を繰り返します。
And once a skill becomes mature, Voyager stores the program into a skill library so that it can quickly record in the future.
スキルが成熟すると、Voyagerはプログラムをスキルライブラリに保存し、将来的に迅速に記録できるようにします。
You can think of it as a code base authored entirely by GPT-4.
GPT-4によって完全に作成されたコードベースと考えることができます。
And in this way, Voyager is able to bootstrap its own capabilities recursively as it explores and experiments in Minecraft.
そして、このようにして、Voyagerはマインクラフトで探索や実験を行いながら、自身の能力を再帰的にブートストラップすることができます。
Because now we're talking about coding and coding is compositional.
なぜなら、今はコーディングについて話しているからです。そして、コーディングは構成的なものです。
Voyager can write a bunch of functions and in the future, the future functions can compose some of the older functions in more and more complex skills and programs.
Voyagerはたくさんの関数を書くことができ、将来の関数はより複雑なスキルやプログラムの中で古い関数の一部を構成することができます。
So, let's go through a working example together.
では、一緒に実際の例を見てみましょう。
The agent in Minecraft finds its hunger bar dropping to one out of 20.
マインクラフトのエージェントは、空腹ゲージが20のうち1になるのを感じます。
So, it knows it needs to find food.
だから、食べ物を見つける必要があると知っています。
And now it senses four entities nearby: a cat, a villager, a pig, and some wheat seed.
そして、今は近くに4つのエンティティが感知されます:猫、村人、豚、そして小麦の種。
So, now it starts an inner monologue.
それでは、内なる対話を始めましょう。
Do I kill the cat or the villager for food?
猫を殺す?それとも村人を殺す?食べ物のために。
That sounds like a bad idea.
それは良くない考えのようです。
How about the wheat seed?
小麦の種はどうですか?
I could grow a farm, but it's going to take a very long time.
農場を作ることができますが、非常に長い時間がかかります。
So, sorry, piggy, you are the chosen one.
だから、ごめんね、豚ちゃん、君が選ばれたんだ。
And then, Voyager checks the inventory, retrieves an old skill from the library to craft an iron sword.
そして、Voyagerはインベントリをチェックし、ライブラリから古いスキルを取り出して鉄の剣を作ります。
And then starts to learn a new skill called funded pig.
そして、新しいスキルである「funded pig」を学び始めます。
So that's kind of a working example of how Voyager would go through this loop.
これがVoyagerがこのループを通過する実際の例の一つです。
And the question still remains, how does Voyager keep exploring indefinitely?
そして、まだ疑問が残っています。Voyagerはどのようにして無期限に探索を続けるのでしょうか?
So all we did is to give Voyager a high-level directive, obtain as many unique items as possible.
私たちがしたことは、ボイジャーに高レベルの指示を与え、できるだけ多くのユニークなアイテムを入手することです。
And then Voyager implements a curriculum, again, by itself, to find progressively harder and novel challenges to solve.
そして、ボイジャーは自らカリキュラムを実装し、徐々に難しくて新しい課題を見つけ出すようにしています。
So I want to highlight that none of these are hard code.
これらはすべてハードコードではないことを強調したいと思います。
This progression of skills are discovered by Voyager itself as it explores.
スキルの進展は、ボイジャー自体が探索することで発見されます。
And also the curriculum that Voyager proposes is conditioned on its current capabilities, right?
また、ボイジャーが提案するカリキュラムは、現在の能力に基づいて条件付けられていますね?
Like if you only know how to use wooden tools, then you probably shouldn't propose to solve some diamond, to solve some tasks that would require diamond tools, right?
たとえば、木の道具しか使い方を知らない場合、ダイヤモンドの道具が必要なタスクを解決することを提案するべきではないでしょう?
There's like a progression of it.
それには進展があります。
And Voyager is able to find this curriculum automatically.
そして、ボイジャーはこのカリキュラムを自動的に見つけることができます。
And putting all these together, Voyager is able to not only master, but also discover new skills along the way.
これらすべてを組み合わせると、ボイジャーはマスターするだけでなく、途中で新しいスキルを発見することができます。
And we didn't pre-program any of these.
これらのいずれも私たちが事前にプログラムしたものではありません。
It's all Voyager's idea.
すべてボイジャーのアイデアです。
We simply took some snapshots from its playing session.
私たちは単に、プレイセッションからいくつかのスナップショットを取りました。
And that's what is shown here.
それがここに表示されているものです。
And we call this process lifelong learning, where the agent is forever curious and also forever pursuing new adventures.
このプロセスを終身学習と呼び、エージェントは常に好奇心を持ち、新しい冒険を追求し続けるのです。
Have you guys considered putting more than one agent in the same server together and seeing if they can learn to interact with each other and complete tasks cooperatively?
複数のエージェントを同じサーバーに配置し、お互いと協力してタスクを完了できるように学習できるかどうかを考えたことはありますか?
That's a great idea.
それは素晴らしいアイデアです。
So we thought about it.
私たちはそれについて考えました。
But back then, I think the framework doesn't really support multi-agent.
しかし、当時はフレームワークがマルチエージェントを本当にサポートしていなかったと思います。
At least the framework we implemented does not quite support that.
少なくとも、私たちが実装したフレームワークはそれをあまりサポートしていません。
But it is our future.
しかし、それは私たちの未来です。
So yeah, it is a very interesting question.
だから、それは非常に興味深い質問です。
And I do think having multi-agent would have new emergent properties.
そして、私はマルチエージェントを持つことで新しい出現的な特性があると思います。
Right, yeah.
そうですね、そうです。
Because my whole thought process was long-term, we could see maybe we could have 30 plus agents all in a world building villages together and stuff like that.
なぜなら、私の考え方全体が長期的なものだったので、30人以上のエージェントが一緒に村を建設するなどの世界で見ることができるかもしれません。
We could really see how they could develop different maybe ideals or goals over time and see what kind of separates them.
彼らが時間の経過とともに異なる理念や目標をどのように発展させるか、どのように彼らを分けるかを本当に見ることができるかもしれません。
I just thought that was interesting.
私はそれが興味深いと思っただけです。
Thanks for answering.
回答してくれてありがとう。
It is very interesting.
それは非常に興味深いです。
Yeah, it's such a great idea.
そうですね、素晴らしいアイデアですね。
I remember in your TED Talk, you mentioned that how Foundation Agent is the way to go.
私はあなたのTED Talkで、Foundation Agentが進むべき道だと述べていましたね。
From what I understand, Voyager is very successful thanks to MineDojo.
私の理解では、MineDojoのおかげでVoyagerは非常に成功しています。
So how are you and other NVIDIA researchers going to overcome the dataset curation barrier and able to have Foundation Agent to be able to play on one 10,000 other simulated realities and maybe Terraria, per se?
では、あなたと他のNVIDIAの研究者は、データセットのキュレーションの壁を乗り越え、Foundation Agentが1万の他のシミュレートされた現実や、たとえばTerrariaでプレイできるようにする予定ですか?
Yes.
はい。
So I think there are a couple of dimensions here.
だから、ここにはいくつかの次元があります。
In my TED Talk, I talk about three axes.
私のTED Talkでは、3つの軸について話しています。
So the first axis is skills, the number of skills the agent can master.
最初の軸は、エージェントがマスターできるスキルの数です。
And the second one is the number of embodiment that it can control.
そして2番目は、それが制御できる具現化の数です。
And by embodiment, I mean things like robot bodies.
具現化とは、ロボットのようなものを指します。
So you can have a humanoid form factor or you can have like a robot dog or agents in Minecraft.
人型の形状やロボット犬、マインクラフトのエージェントなど、さまざまな形状を制御できます。
You have different ways, different bodies that you can control.
異なる方法、異なる体を制御することができます。
So that's what we call embodiment.
これが具現化と呼ばれるものです。
And the third axis is realities, basically the number of simulations that the agent can master.
そして3番目の軸は、基本的にはエージェントがマスターできるシミュレーションの数です。
And here for Voyager, we only tried it in Minecraft because it is an open-ended world.
ここでは、Voyagerではマインクラフトでしか試していません。なぜなら、それは無限の世界だからです。
It is like one simulation, but it's kind of like a meta simulation, right?
それは1つのシミュレーションのようなものですが、メタシミュレーションのようなものですね。
Like in this one simulation, you can do so many different things.
この1つのシミュレーションでは、無限に多くの異なることができます。
In fact, infinitely, infinite number of creative things.
実際には、無限の創造的なことができます。
And we have seen humans doing crazy things in this world as well.
そして、私たちは人間がこの世界でクレイジーなことをしているのを見てきました。
Like someone actually built a functioning CPU circuit within Minecraft because Minecraft supports something called a redstone circuit, which apparently makes the game too incomplete.
実際に、マインクラフト内で機能するCPU回路を作った人もいました。なぜなら、マインクラフトはレッドストーン回路というものをサポートしているからです。それがゲームを不完全にするらしいです。
It's like a programmable game.
それはプログラム可能なゲームのようなものです。
And Minecraft is just one kind of simulated reality.
マインクラフトはただの1つの種類のシミュレートされた現実です。
But also there are thousands of games out there, right?
しかし、世界中には数千ものゲームがありますよね?
There's Legend of Zelda, there's Elden Ring, right?
ゼルダの伝説やエルデンリングなどがありますよね?
All of the open-ended games.
すべてのオープンエンドゲームがあります。
And there are also simulated realities for robots.
また、ロボットのためのシミュレートされた現実もあります。
And we also have the real world, which is by itself, our OG reality.
そして、私たち自身のオリジナルの現実である現実世界もあります。
So the way I see kind of the future of foundation models for agent is that we need to scale across all the three axes I just talked about.
だから、私がエージェントのための基盤モデルの将来を考えるとき、私たちは私が話した3つの軸全体にわたってスケールする必要があると考えています。
We need to scale over the number of skills, embodiments you can control.
私たちは、制御できるスキルや具現化の数を拡大する必要があります。
A single model can control all the robot bodies.
1つのモデルですべてのロボットボディを制御できます。
And then it can master all kinds of different rules, mechanics, and physics in different worlds, virtual and physical worlds alike.
そして、さまざまな世界、仮想的な世界と物理的な世界の中でさまざまなルール、メカニズム、物理をマスターすることができます。
And here the idea is if a model is able to master 100 different simulated realities, then our real physical world could simply be the 101st reality.
そして、ここでのアイデアは、モデルが100の異なるシミュレートされた現実をマスターできる場合、私たちの現実の物理的な世界は単に101番目の現実になるということです。
So some of you might heard about something called the simulation hypothesis, right?
皆さんはシミュレーション仮説というものを聞いたことがあるかもしれませんね?
Like saying that, oh, our real world is actually a simulation.
現実の世界は実際にはシミュレーションだということです。
I mean, we can talk about metaphysics and philosophy all day, but I actually think that idea is great to build AI.
形而上学や哲学について一日中話すことはできますが、私は実際にはその考えがAIを構築するのに適していると思っています。
Because for AI, our real world is just another simulation to it.
なぜなら、AIにとって、私たちの現実の世界はただの別のシミュレーションに過ぎないからです。
Like we can actually use this principle to guide the design of our next generation of embodied AI systems.
実際にこの原則を使って、次世代の具現化されたAIシステムの設計をガイドすることができます。
And that is kind of a quick recap of the main idea called Foundation Agent in my TED Talk.
これは、私のTED Talkで紹介したメインアイデアであるFoundation Agentの簡単なまとめです。
Yeah.
はい。
Does that answer your question?
それで質問に答えましたか?
Yeah.
はい。
I was more curious about how it's going, because data is probably going to be a key and how it's going to learn skills is depending on like, I remember MineDojo or forgot which one is relying on YouTube to learn all the Minecraft movements or Minecraft skills.
どのように進行しているかがもっと知りたかったんです。なぜなら、データがおそらく重要な鍵になるでしょうし、どのようにしてスキルを学ぶかは、たとえば、私が覚えているMineDojoか、どちらか忘れましたが、YouTubeを利用してMinecraftの動きやスキルをすべて学んでいるようなものに依存していることによるのです。
So is it basically had to rely on all pre-existing data or like the whole data creation problem? Or would you have like agent to simulate or like naturally learn skills by itself in the future?
つまり、基本的には既存のデータに頼らなければならないのか、それとも将来的にはエージェントがシミュレーションしたり、自然にスキルを学ぶことができるのか、ということですか?
Yes.
はい。
Let me switch to the MineDojo slide and let me reshare it.
私はMineDojoのスライドに切り替えて、再共有します。
So I think you're right, we need some data to bootstrap the process.
そうですね、あなたの言う通り、プロセスを始めるためにはいくつかのデータが必要です。
And for Minecraft specifically, like this game is one of the most, maybe the most streamed games on YouTube.
そして、特にマインクラフトの場合、このゲームはおそらくYouTubeで最もストリーミングされているゲームの一つです。
So there are like hundreds or if not millions of hours of Minecraft play videos online.
だから、数百、もしくは何百万時間ものマインクラフトのプレイ動画がオンライン上に存在します。
And in MineDojo, we explored this process.
そして、MineDojoでは、このプロセスを探求しました。
We explore this data set.
私たちはこのデータセットを探求しました。
So we collected a lot of YouTube videos where both kind of the gamer is playing the game and also narrating what they're doing.
私たちは、ゲーマーがゲームをプレイしている様子と、何をしているかを説明しているナレーションが両方含まれるYouTubeの動画をたくさん集めました。
And these are like real segments from a tutorial video, right?
これらは、実際のチュートリアル動画からのセグメントですね。
Let's say video clips three, as I raise my axe in front of this pig, there's only one thing you know is going to happen.
例えば、ビデオクリップ3では、私がこの豚の前で斧を振り上げると、起こることはただ一つです。
This is actually some YouTuber said this and we put it in a data set.
これは実際にYouTuberが言ったもので、私たちはそれをデータセットに入れました。
So the way we use this model is we train something called a MineCLIP.
このモデルの使い方は、MineCLIPと呼ばれるものを訓練することです。
And to skip the technical details, what the model does is that it learns the association between the video and the transcript that describes the actions in the video.
技術的な詳細は省きますが、このモデルは、ビデオとビデオ内のアクションを説明するトランスクリプトとの関連性を学びます。
So let's say for this example, this transcript, I'm going to go around and gather a little bit more wood from these trees. This transcript aligns very well with the activity in this video.
この例では、このテキストを使って、木からもう少し薪を集めることにしましょう。このテキストは、このビデオの活動と非常によく一致しています。
So this score will be close to one.
したがって、このスコアは1に近くなります。
And this part is talking about pig.
そして、この部分は豚について話しています。
It's not aligned with this video and the score would be close to zero.
このビデオとは合っておらず、スコアはほぼゼロになるでしょう。
So the score will always be between zero and one.
そのため、スコアは常にゼロから一の間になります。
And one means perfect description, zero means the text is irrelevant.
そして、一は完璧な説明を意味し、ゼロはテキストが関係ないことを意味します。
And you can treat this as a reward function.
そして、これを報酬関数として扱うことができます。
So concretely, how you use it is you have an agent simulation.
具体的には、エージェントのシミュレーションがあります。
And then you have a task prompt asking you to share sheep to obtain wool.
そして、羊を取得するために羊を共有するように求めるタスクプロンプトがあります。
And as the agent explores, it will generate a video snippet, right?
エージェントが探索すると、ビデオの断片が生成されますね?
And then this video snippet can be compared to this language embedding, and then output a score.
そして、このビデオの断片はこの言語の埋め込みと比較され、スコアが出力されます。
And you want to maximize this score, because that means your behavior is aligned with what the task prompt wants you to do.
このスコアを最大化したいのです。なぜなら、それはあなたの行動がタスクプロンプトが望むものと一致していることを意味するからです。
And this becomes a reinforcement learning loop.
そして、これは強化学習のループになります。
So it's actually ROI track.
実際にはROIトラックです。
If you look at it, if you squeeze at it, it's ROI track, right?
それを見ると、それを絞り込むと、ROIトラックですね。
Reinforcement learning from human feedback in Minecraft.
マインクラフトでの人間のフィードバックからの強化学習。
And just that the human feedback is not learned by annotating the dataset manually, but from kind of getting the transcript and videos from YouTube.
ただし、人間のフィードバックはデータセットを手動で注釈付けして学習するのではなく、YouTubeからトランスクリプトとビデオを取得することで学習されます。
So that's how in the MineDojo paper, we're able to leverage this YouTube video dataset.
ですから、MineDojoの論文では、このYouTubeビデオのデータセットを活用することができました。
And moving forward, there are also other ways that we can use the video, right?
そして、今後は他の方法もありますよね。
So I kind of briefly mentioned a few things in the slides as well.
スライドでもちょっと触れました。
For example, you can learn encoding.
例えば、ビデオから視覚表現のエンコーディングを学ぶことができます。
You can learn encoding of the visual representations from the video. This work is applied in robotics, but it can also be used for things like Minecraft.
ビデオから視覚的表現のエンコーディングを学ぶことができます。この研究はロボティクスに応用されていますが、マインクラフトなどの他の用途にも使えます。
And you can also even directly learn some behaviors from video by pseudo labeling the actions.
また、アクションを擬似ラベリングすることで、ビデオから直接いくつかの行動を学ぶこともできます。
So there are many ways kind of on how to use the videos to bootstrap embodied agents.
ですから、ビデオを利用して具現化エージェントをブートストラップする方法はたくさんあります。
And MineDojo is a very particular way to do it.
MineDojoはその中でも非常に特殊な方法です。
Thanks for that, Jim.
それでは、ジム、ありがとう。
Daniel, I know you have a question.
ダニエル、質問があると思いますが。
Like the action space was human annotated from different YouTube clips.
アクションスペースは、さまざまなYouTubeのクリップから人間によって注釈付けされました。
I think you guys set up like a label studio set up and was like labeling, this is mining something, this is doing XYZ.
ラベルスタジオのセットアップを行い、ラベリングを行っていたと思います。これはマイニングをしているもの、XYZをしているものなどをラベリングしていました。
But in Voyager, those actions were extracted by GPT-4 and then saved in a database.
しかし、Voyagerでは、それらのアクションはGPT-4によって抽出され、データベースに保存されました。
So my question is, did you notice any actions that were found by the AI?
私の質問は、AIによって見つけられたアクションについて気付いたことはありますか?
Kind of like AlphaGo, one of your recent tweets, where it found moves that a human wouldn't do.
最近のツイートのように、AlphaGoのように、人間がしないような手を見つけたことはありますか?
It stored moves that a human wouldn't do.
人間がしないような動きを保存していました。
And I guess this is sort of an aside because I'm now realizing that the video data was all human actions.
そして、今気づいたのですが、ビデオデータはすべて人間の行動でした。
So I'm guessing that might not be the case.
だから、それが必ずしもそうではないかもしれません。
Yeah.
そうですね。
So a few things to note.
いくつか注意点があります。
One is in MineDojo, the labeling part is about curating a set of tasks that could be possible in Minecraft.
まず、MineDojoでは、ラベリングの部分はマインクラフトで可能なタスクのセットを作ることに関係しています。
And we curated that set of tasks from some YouTube videos.
そして、そのタスクのセットはYouTubeのビデオから選びました。
But those are not the actions and are not used to train the model.
しかし、それらは行動ではなく、モデルの訓練には使用されません。
So we only train the model using kind of transcripts from in the wild.
だから、私たちはモデルの訓練には、野生の中でのトランスクリプトのみを使用しています。
And the manual curation is only for kind of, these are the interesting tasks that can be done.
そして、手動のキュレーションは、興味深いタスクを示すためだけです。
But we did not use that as actions.
しかし、それを行動として使用していません。
And for Voyager, coming back to your question, so it's able to kind of learn all these skills necessary to survive and to basically find new objects.
そして、Voyagerに戻って、あなたの質問に答えると、生き残るために必要なこれらのスキルを学ぶことができますし、新しいオブジェクトを見つけることもできます。
Because we gave it, yeah, let me kind of, this one.
なぜなら、私たちは、これを与えました、ええ、これを一つ。
So we give it a high-level directive.
私たちは、高レベルの指令を与えました。
That is to maximize the number of objects you can obtain.
それは、取得できるオブジェクトの数を最大化することです。
So we would tell Voyager, your task is to maximize the novel objects that you can obtain.
だから、Voyagerには、あなたのタスクは、新しいオブジェクトの数を最大化することです、と伝えました。
And so what Voyager does is trying to meet that kind of unsupervised objective.
そして、Voyagerは、そのような非監督学習の目的を達成しようとします。
Because we are not telling it that you need to find diamond.
私たちは、ダイヤモンドを見つける必要があるとは言っていません。
You need to find stone first before you need to find iron.
鉄を見つける前に石を見つける必要があるとも言っていません。
Or you need to find iron before diamond.
ダイヤモンドを見つける前に鉄を見つける必要があるとも言っていません。
We did not tell it that.
私たちはそれを伝えませんでした。
We just said, you need to find as many novel objects as possible.
ただ、できるだけ多くの新しいオブジェクトを見つける必要があると言っただけです。
And we actually have a way to measure it.
そして、実際にはそれを測る方法があります。
We can look at its inventory.
私たちは、そのインベントリを見ることができます。
And then count the number of diverse items it's able to obtain through its lifespan.
そして、その寿命を通じて取得できる多様なアイテムの数を数えることができます。
So we can actually quantitatively measure it.
だから、実際に定量的に測ることができます。
And let me show you a figure here.
ここに図を示しましょう。
So we actually have a comparison with some prior works.
実際には、いくつかの先行研究との比較があります。
It's this one.
これです。
Basically, this is React, reflecting on some kind of baseline and AutoGPT.
基本的に、これはReactで、ある種のベースラインとAutoGPTを反映しています。
And this is Voyager.
そしてこれがVoyagerです。
And this is Voyager.
そしてこれがVoyagerです。
The blue one is Voyager without the skill library.
青い方は、スキルライブラリのないVoyagerです。
And here, in this figure, the x-axis is the number of prompting iterations.
そして、この図では、x軸はプロンプティングの反復回数です。
And the y-axis is the number of distinct objects it's able to uncover or craft.
そして、y軸は発見または作成できる異なるオブジェクトの数です。
It doesn't matter.
問題ありません。
As long as you're in inventory, we see a new object, we count it towards the progress.
インベントリにいる限り、新しいオブジェクトが見つかると、進捗にカウントされます。
So it's got this high-level objective programming tool.
だから、これは高レベルの目標プログラミングツールを持っています。
And mostly, like the skills, I would say a human would be able to do.
そして、ほとんどの場合、スキルのようなものは、人間ができると思います。
And Voyager is not able to build crazy things yet.
Voyagerはまだクレイジーなものを作ることはできません。
Because that would require vision.
なぜなら、それにはビジョンが必要だからです。
And in the original Voyager, we did not have computer vision.
そして、元のVoyagerでは、コンピュータビジョンはありませんでした。
It's not doing the tasks from pixels.
それはピクセルからのタスクを実行していません。
It's converting the world to text.
それは世界をテキストに変換しています。
And that would be a limitation.
そして、それは制約です。
So if you want to build a castle, you've got to see what you're building.
だから、城を建てたいなら、何を建てているか見なければなりません。
Otherwise, it's really hard to tell you the 3D coordinates and try to reason your head.
そうでないと、3D座標を伝えるのは本当に難しいですし、頭で理解しようとするのも難しいです。
Even for humans, it's really hard.
人間にとっても、本当に難しいです。
So Voyager doesn't do building tasks because we didn't ask it to.
だから、Voyagerは建築タスクを行いません。私たちはそれを頼んでいないからです。
And also, it's not quite capable of because of the limitation of its perception model.
そしてまた、知覚モデルの制約のために、それが完全に可能ではありません。
To you, what is the strategic value of a corpus like YouTube for training these type of open-ended embodied agents?
あなたにとって、YouTubeのようなコーパスがこの種のオープンエンドの具現化エージェントのトレーニングにおける戦略的な価値は何ですか?
Are these agents going to be able to make sense of the different rules of the world as they vary in simulations versus real-world data?
これらのエージェントは、シミュレーションと実世界のデータで異なるルールを理解することができるのでしょうか?
Physics, for example, varies drastically.
例えば、物理学は大きく異なります。
So what are your thoughts?
では、あなたの考えは何ですか?
So I think one of the components to build Foundation Agent is really good video models that can understand not just Minecraft video, but maybe videos from many different games or even videos of the real world, of people doing different tasks.
だから、Foundation Agentを構築するための要素の一つは、マインクラフトのビデオだけでなく、さまざまなゲームのビデオや、さまざまなタスクを行う人々のビデオなどを理解できる優れたビデオモデルです。
We want to train on as many videos as possible because what videos encode is something that we technically call intuitive physics.
私たちはできるだけ多くのビデオでトレーニングしたいのです。なぜなら、ビデオがエンコードしているものは、私たちが技術的に直感的な物理学と呼んでいるものだからです。
So when humans, when all of us go ahead to do our daily tasks, we don't solve physics equations in our head.
だから、私たち全員が日常のタスクを行うとき、頭の中で物理学の方程式を解くわけではありません。
If you drop a cup on the floor, your brain cannot compute exactly where the water is going to spill or how the cup is going to be broken.
もし床にカップを落としたら、あなたの脳は水がどこにこぼれるか正確に計算することはできませんし、カップがどのように壊れるかも計算できません。
You cannot simulate all of that.
それをすべてシミュレートすることはできません。
But you roughly know that you're going to make a mess.
しかし、あなたは大まかに自分がめちゃくちゃになることを知っています。
The water is going to spill and the cup, if it's a glass cup, it's mostly going to be broken.
水はこぼれて、グラスのカップならほとんど壊れます。
You have a rough common sense of where things are going.
物事がどのように進むかについて、おおよその常識があります。
And that is the predictive model in our brain and what we call intuitive physics.
それが私たちの脳内の予測モデルであり、直感的な物理学と呼ばれるものです。
It's not physics, it's intuitive.
それは物理学ではなく、直感です。
We cannot compute every trajectory.
私たちはすべての軌道を計算することはできません。
And I think for the current embodied agents, they lack this common sense.
そして、現在の具体的なエージェントには、この常識が欠けています。
They can't really predict what's going on next.
彼らは実際に次に何が起こるのかを予測することができません。
They don't have this intuitive physics built into their brain.
彼らの脳にはこの直感的な物理学が組み込まれていません。
And to learn intuitive physics, I believe the best way is to learn on lots and lots of videos.
そして、直感的な物理学を学ぶために、私はたくさんのビデオを学ぶことが最善だと信じています。
And once you have that common sense model, it's still not enough.
そして、一度その常識モデルを持っていても、それだけでは十分ではありません。
You can predict what's going on next, but you still don't know how to act.
次に何が起こるかを予測することはできますが、どのように行動するかはまだわかりません。
So just like if you watch tennis champions playing tennis, you can watch it all day and you know what's going to happen next.
テニスのチャンピオンがテニスをプレイしているのを見ても、一日中見ていて次に何が起こるかはわかります。
You have a predictive model in your brain, but can you play tennis as well as the best players?
脳内に予測モデルがありますが、あなたは最高のプレイヤーと同じくらいテニスができますか?
You still need a lot of practice to actually ground the common sense that you learn from the videos.
実際にビデオから学んだ常識を確固とするためには、まだたくさんの練習が必要です。
And that's how I see the simulations coming into play.
そして、それがシミュレーションが重要な役割を果たす方法です。
So you need both the videos and a lot of pre-training and also the simulations, be it Minecraft or Physics Sim or some other games, to really ground the knowledge through trial and error.
ですから、ビデオと多くの事前トレーニング、そしてマインクラフトやPhysics Simなどのシミュレーションを通じて、知識を試行錯誤することが本当に重要です。
And that's how I see, that's how I believe we should build the next embodied systems.
そして、それが次の具体的なシステムを構築する方法だと私は考えています。
I hope that answers your question.
それで質問に答えられたらいいのですが。
Yeah, it does.
はい、答えられました。
Is that how you see Omniverse fitting into all of this, right?
それがOmniverseがこれらすべてにどのように適合するか、そう見えるのですか?
Like Tesla's like noisy data at scale, but we're going to need sort of synthetic training data or like I guess open-ended agents trying stuff in the in simulated environments too.
テスラのようなノイズの多い大規模なデータがある一方で、シミュレートされた環境での合成トレーニングデータや、オープンエンドのエージェントが試行錯誤する必要もあるのではないでしょうか。
Yes.
はい。
How about this?
これはどうでしょうか?
Let me share a screen.
画面を共有させてください。
This is Eureka.
これはEurekaです。
It is a five-finger robot hand that's able to do pen spinning tricks in NVIDIA simulation.
これはNVIDIAのシミュレーションでペン回しのトリックができる5本指のロボットハンドです。
And how we are able to train this is actually using something called ISXSim that is built on top of Omniverse.
そして、これを訓練する方法は、実際にはOmniverseの上に構築されたISXSimというものを使用することです。
So in terms of abstraction levels, you can think of Omniverse as like a base level graphics engine, right?
抽象化レベルに関しては、Omniverseは基本レベルのグラフィックスエンジンのようなものと考えることができますね。
It runs on the latest GPUs.
最新のGPU上で動作します。
It's able to get acceleration, hardware native acceleration, and it does rendering, physics and all of that.
ハードウェアのネイティブなアクセラレーションを得ることができ、レンダリングや物理演算なども行います。
It's in Omniverse.
Omniverseに含まれています。
And the ISXSim is a library built on top of Omniverse for robotics specifically.
そして、ISXSimは、特にロボット工学のためにOmniverseの上に構築されたライブラリです。
So it's able to import things like robot hand models, import objects compute the contact physics of the fingers with the pen here.
したがって、ロボットの手のモデルやオブジェクトをインポートし、ここでのペンとの指の接触物理を計算することができます。
And most importantly, and probably the most unique feature of ISXSim is its scalability.
そして、ISXSimの最も重要でおそらく最もユニークな機能は、スケーラビリティです。
So you can run 10,000 environments in parallel on a single GPU, which means you're basically speeding up reality by 10,000 X. In the real world, you're bottlenecked by real physics, right?
つまり、単一のGPU上で10,000の環境を並列に実行することができるため、実質的に現実を10,000倍速く進めることができます。現実世界では、実際の物理に制約がありますよね?
You simply cannot collect data with this level of throughput.
このレベルのスループットでデータを収集することは不可能です。
But in simulation, you can.
しかし、シミュレーションでは可能です。
If you sort of compute at it, and with parallel computing, you can simulate 10,000 robot hands doing pen spinning at the same time.
計算を行い、並列計算を行えば、同時に10,000のロボットハンドがペンを回転させるようなことをシミュレートすることができます。
And in this way, you scale up the data stream and you can train like very complex policies like pen spinning that would otherwise take maybe a decade of real world time if you want to do this directly on a physical robot, right?
そして、このようにデータストリームをスケーリングアップし、物理的なロボット上で直接行う場合にはおそらく10年かかるであろうペン回しのような非常に複雑なポリシーを訓練することができますよね?
It's very slow.
非常に遅いです。
So yeah, that's how ICMD simulation comes into play for embodied agents.
ですので、それが具現化エージェントにおいてICMDシミュレーションがどのように関与しているかです。
And since we're talking about Eureka, I will just quickly cover this work.
そして、Eurekaについて話しているので、この研究についても簡単に説明します。
So how is Eureka trained?
Eurekaはどのように訓練されるのでしょうか?
Basically, I see Eureka has two loops.
基本的に、Eurekaには2つのループがあります。
The outer loop is a language model, here GPT-4, writing code in a physics simulation API.
外側のループは、言語モデルであるGPT-4が、物理シミュレーションAPIでコードを書くことです。
And this code will become the reward function.
そして、このコードが報酬関数となります。
And reinforcement learning requires a reward function so that you have something to maximize, right?
強化学習では報酬関数が必要であり、最大化するものがあることが必要ですよね?
Something to work towards.
何か目指すものが必要です。
And that is the second loop.
それが2番目のループです。
The inner loop is that given a reward function, we do reinforcement learning to train another neural network that controls the robot hand.
内側のループは、報酬関数が与えられた場合に、ロボットハンドを制御する別のニューラルネットワークを強化学習して訓練することです。
And then this dual loop system is what makes Eureka quite unique.
そして、この二重ループシステムがEurekaをかなりユニークなものにしています。
You can think of this as system one and two thinking, right?
これは、システム1と2の思考と考えることができますね。
From the book thinking fast and slow.
本「思考の遅い思考の速い」からの引用です。
The LOM loop is a system two loop because it's doing high level reasoning, right?
LOMループはシステム2のループであり、高レベルの推論を行っていますね。
It's looking at how the hand is performing.
それは手のパフォーマンスを見ています。
And then proposing change in code.
そして、コードの変更を提案しています。
So it's like a system two deliberate slow reasoning.
だから、それはシステム2の意図的な遅い推論のようなものです。
And the loop on the right is a system one loop.
そして、右側のループはシステム1のループです。
It is like fast, unconscious.
それは速く、無意識のようなものです。
Like you don't do reasoning when you're spinning pen, right?
ペンを回しているときには推論をしないでしょう?
It's more like a feeling of it.
それはむしろ感覚のようなものです。
It's muscle memory.
それは筋肉の記憶です。
So the loop on the right hand side will be the system one, where we have a smaller neural network, but it's much higher frequency than the LOM and is able to control the hand to do very dexterous tasks.
だから、右側のループはシステム1であり、より小さなニューラルネットワークですが、LOMよりも高い周波数で、非常に器用なタスクを制御することができます。
And we're able to do not just pen spinning, but like a few other kind of manual manipulation tasks for the robot.
ペン回しだけでなく、ロボットの他の種類の手作業もできます。
I'm not showing, I don't think I'm showing this here, but basically this method is general purpose and it's not limited to just pen spinning.
ここでは表示していませんが、基本的にこの方法は汎用性があり、ペン回しに限定されていません。
Okay.
わかりました。
And I'll open up for like five minutes or questions.
5分間、質問を受け付けます。
Thank you very much.
ありがとうございました。
I'll try to make this quick.
できるだけ早く説明します。
So I know you said, I think in the paper, it says that the reward functions can essentially be updated in somewhat real time.
ペーパーに書かれているように、報酬関数は実質的にリアルタイムで更新できると言っていましたね。
Is that correct?
それは正しいですか?
You don't have to retrain the entire model.
モデル全体を再訓練する必要はありません。
So for the reward function, like it's updated every time the inner loop or the loop on the right hand side has finished.
報酬関数については、右側のループが終了するたびに更新されます。
So you can think of like this loop as a full reinforcement learning training session, right?
このループは、完全な強化学習のトレーニングセッションと考えることができますね。
And we train it to conversions and then it will have like performance metric, which you can report back to GPT-4 and then GPT-4 will propose the next reward function.
そして、それを収束させてから、GPT-4にパフォーマンスメトリックを報告し、GPT-4が次の報酬関数を提案します。
Okay.
わかりました。
So the future that I'm seeing with this here is that we could have a bot that actually exists in the real world and we could potentially with similar architecture train a bot by actually showing it an example and then it practices that same example itself.
この先、私が見ている未来では、実際の世界に存在するボットを持つことができ、同じアーキテクチャを使ってボットを訓練することができるかもしれません。具体的な例を示してそれを実践させることで、ボットが同じ例を自分で練習することができるかもしれません。
So I'm just wondering if you guys seem to be very focused on robotics.
ただ、あなたたちはロボットに非常に焦点を当てているように見えるので、ちょっと疑問に思っています。
So is this the future that you guys are looking towards?
それが、あなたたちが向かっている未来なのですか?
Yeah.
はい。
I think there are many ways we can scale Eureka even more, right?
私たちはEurekaをさらにスケールアップする方法はたくさんあると思いますよ。
Like one is it's skills, assimilation skills.
例えば、スキルの習得スキルです。
And here we are learning like one skill at a time, right?
そして、私たちは一度に一つのスキルを学んでいますよね?
This pen spinning skill is like one Eureka run.
このペン回しのスキルは、一つのEurekaの実行です。
But you can imagine that we can do maybe a thousand different skills in parallel if we throw a lot of GPUs at it.
しかし、もし私たちがたくさんのGPUを投入すれば、同時にたくさんの異なるスキルを行うことができると想像できます。
So that is something that we're thinking about doing.
それは私たちが考えていることです。
Oh, and actually in this video, you can see like there are a lot of other tasks that we tried, but each task is like a separate neural network, right?
ああ、そして実際にこのビデオでは、他にもたくさんのタスクを試しているのが見えますが、各タスクは別々のニューラルネットワークですよね?
We're not training a single one that has multitasking, but it is an obvious next step that we can do.
私たちはマルチタスキングを持つ単一のニューラルネットワークを訓練しているわけではありませんが、それは次の明らかなステップです。
And the other thing is to actually make it work in the real world.
そしてもう一つは、実際の世界でそれを動かすことです。
And that will involve sim to real, right?
それにはシムからリアルへの移行が必要ですよね?
How do we kind of transfer the neural network learning simulation to the real world?
どのようにしてニューラルネットワークの学習シミュレーションを実際の世界に移すのでしょうか?
And there are many techniques to it.
それにはいくつかの技術があります。
One is called domain randomization, which is basically the simulation hypothesis I just mentioned.
その一つがドメインランダム化と呼ばれるもので、先ほど言ったシミュレーションの仮説です。
Like if you're able to master 10,000 different simulated realities or like different physical configurations in sim, let's say if you're able to work with earth's gravity and also moon's gravity and also Mars gravity in simulation, 10,000 of them, then you are very likely able to generalize to the real world, which will be very complex and not quite the same as the simulation, right?
たとえば、10,000の異なるシミュレートされた現実や異なる物理的な構成を制御できる場合、例えば地球の重力や月の重力、火星の重力など、シミュレーションで10,000のそれらを扱える場合、それは非常に複雑でシミュレーションとは異なる実世界にも一般化できる可能性が非常に高いですよね?
Simulation is always going to be inaccurate portrayal of the real world, but that's how we overcome the sim to real gap.
シミュレーションは常に実際の世界の正確な描写にはなりませんが、それがシムからリアルへのギャップを克服する方法です。
I feel like Eureka is a very underrated research paper for last year.
私はEurekaが昨年の非常に評価されていない研究論文だと感じています。
It's probably my favorite.
おそらく私のお気に入りです。
And is it the first of its kind?
それはその種の最初のものですか?
Oh, sorry.
ああ、すみません。
Is it the first of its kind where LLM trained robot?
LLMで訓練されたロボットは初めてのものですか?
Like is it fully LLM trained robot?
完全にLLMで訓練されたロボットですか?
And if so, is there like a bridge being built right now where skill learned in Isaac Gym can be applied to real world robots?
もしそうなら、現在、Isaac Gymで学んだスキルを実際の世界のロボットに適用するための橋が建設されていますか?
Yeah.
はい。
So first, thanks for your kind words.
まず、お褒めの言葉をありがとうございます。
So there are a few works on kind of combining LLMs and robotics.
LLMとロボティクスを組み合わせた研究はいくつかあります。
There are also some works from Berkeley, from Stanford, from some of the universities.
バークレー、スタンフォード、いくつかの大学からもいくつかの研究があります。
But I think Eureka, at least to my knowledge, should be the first on using LLM to kind of instruct how to train robot, right?
しかし、私の知る限りでは、Eurekaはロボットの訓練方法としてLLMを使う最初のものであるはずです。
You can see Eureka as automating the development of robotics, right?
Eurekaは、ロボティクスの開発を自動化するものと考えることができます。
Because typically the reward functions are written by human engineers, who's like robot developers, robot engineers, and not like every developer can write reward function.
なぜなら、通常、報酬関数はロボットの開発者である人間のエンジニアによって書かれるからです。すべての開発者が報酬関数を書くことはできません。
It's actually very specific.
それは実際に非常に特定のものです。
You got to have like domain expertise on how to use physics simulation.
物理シミュレーションの使い方についてのドメインの専門知識が必要です。
You got to be familiar with the whole framework to be able to do it, right?
それを行うためには、フレームワーク全体に精通している必要がありますよね?
It's not even easy for any programmer to do this without training.
訓練なしでこれを行うのは、どんなプログラマにとっても簡単ではありません。
But here we found that GPT-4 is so good at zero-shot understanding documentation.
しかし、ここではGPT-4がドキュメントのゼロショット理解に非常に優れていることがわかりました。
So we simply feed like MVDS physics API documentation to GPT-4.
ですので、MVDS物理APIのドキュメントを単純にGPT-4に与えます。
And then, it writes these reward functions, and they can.
そして、それがこれらの報酬関数を書きます。そして、それらはできます。
It can actually write it better than the human developers.
実際には、それは人間の開発者よりも優れて書くことができます。
So we see Eureka as a first step towards automating the development of robotics itself, right?
ですので、私たちはEurekaをロボット開発自体の自動化への第一歩と見ていますね、そうですか?
If you think of robotics, it's basically a bunch of code.
ロボットを考えると、基本的にはコードの束です。
Ultimately, it's just coding, right?
最終的には、単なるコーディングですよね?
Like, can an entire robot stack be programmed not by us, but by GPT-4 or whatever is coming next, and they can do it iteratively?
例えば、私たちではなく、GPT-4や次に来るものがイテレーションを行うことができるのでしょうか?
That is a fascinating question.
それは魅力的な質問ですね。
So, would it be reasonable to describe it as the first AI agent trained robot in a simulation?
それでは、シミュレーションで訓練された最初のAIエージェントとしてそれを説明するのは妥当でしょうか?
The first kind of like LLM instructed AI agent.
LLMによって指示された最初の種類のAIエージェントですね。
Yeah, the first LLM trained kind of dexterous agent concept.
そうですね、最初のLLM訓練された器用なエージェントの概念ですね。
And is there a possibility for robotics?
そして、ロボット工学には可能性がありますか?
Yeah, sorry, go ahead.
はい、すみません、どうぞ。
Is there like a possibility of, have you heard of Mamba? Like the architecture of Mamba?
Mambaというものを聞いたことがありますか?Mambaのアーキテクチャについての可能性のようなものがあるのでしょうか?
Yeah.
はい。
Is there a possibility of Transformer being replaced for Mamba in RoboSims or robot learning like in VMA?
RoboSimsやVMAのようなロボット学習で、トランスフォーマーがMambaに置き換えられる可能性はありますか?
Sorry, that might be off topic.
申し訳ありませんが、それは話題から外れるかもしれません。
Yes.
はい。
I think it's an orthogonal question because the architecture part, I mean, it will be useful, but it is not the pinpoint of robot research.
アーキテクチャの部分に関しては、有用であるかもしれませんが、それがロボット研究の核心ではありません。
We have not even exhausted the potential of Transformers yet.
私たちはまだTransformerの潜在能力を十分に引き出していません。
I think the hard part for robotics is data, right?
ロボット工学にとって難しいのはデータですよね?
How do you get data?
どのようにデータを取得しますか?
The data can come from internet videos, as we just covered.
データはインターネットの動画から取得できます。先ほど説明しましたね。
It can also come from scaling up simulation.
シミュレーションのスケーリングアップからもデータを取得できます。
And for simulation, it's a little bit special because the data is generated by the agent itself, right?
そしてシミュレーションに関しては、データはエージェント自体によって生成されるという特殊なものですね?
It's kind of an actively collected data versus internet will be possibly connected data.
それはアクティブに収集されたデータと、インターネットから取得される可能性のあるデータということですね。
So data is the bottleneck.
つまり、データがボトルネックなのです。
We can use whatever architecture we want.
私たちは好きなアーキテクチャを使うことができます。
And if Mamba replaces Transformer in the future, we have to switch, but it's not kind of the pinpoint right now.
そして将来的にMambaがTransformerに置き換わる場合、私たちは切り替える必要がありますが、現時点ではそれが核心ではありません。
Got it.
わかりました。
Thank you so much.
本当にありがとうございます。
この記事が気に入ったらサポートをしてみませんか?