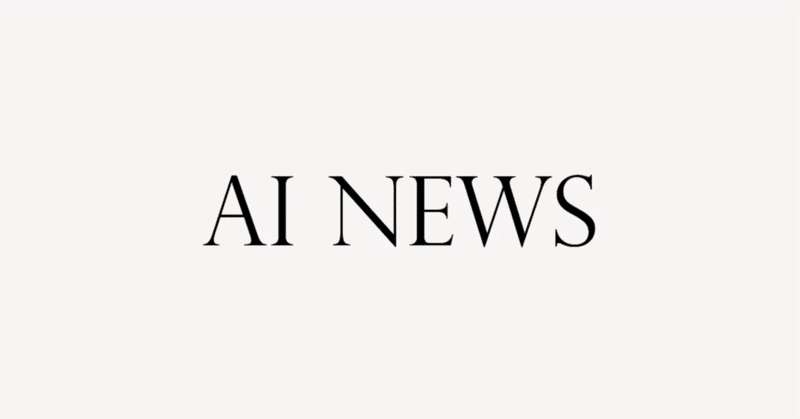
【GPT-5開発の最新動向】英語解説を日本語で読む【2024年1月27日|@AI Explained】
この動画では、OpenAIがGPT-5のフルトレーニングを開始した可能性と、その進展について詳述されています。共同創設者グレッグ・ブロックマンと研究者ジェイソン・ウェイのツイートが証拠として引用され、GPT-5のトレーニングは数ヶ月かかると予想されています。GPT-5は、GPT-4よりも約10倍のパラメータ数を持ち、より高度なパターン認識、コーディング、数学、STEM分野でのパフォーマンス向上が期待されています。
公開日:2024年1月27日
※動画を再生してから読むのがオススメです。
It seems quite likely that yesterday was the day that OpenAI launched the full training run of GPT-5.
恐らく昨日はOpenAIがGPT-5の完全なトレーニングランを開始した日だったようです。
I've gone through every source I can find to bring you the most reliable information on what that means, including possibly every public comment on the topic from OpenAI, an exclusive interview with a hardware CEO, and tons of my own analysis.
私は可能な限り信頼性の高い情報を提供するために、OpenAIからの可能な限りの公開コメント、ハードウェアCEOとの独占インタビュー、そして私自身の分析を含む、見つけられるすべての情報源を調査しました。
Plus, I'm going to find time to throw in a practical tip that you can use literally every time you open GPT, and a bonus early discovery that I really enjoy.
さらに、GPTを開くたびに実用的なヒントを投入し、私自身が本当に楽しんでいるボーナスの早期発見も加えます。
But let's start with these two tweets.
しかし、まずはこれらの2つのツイートから始めましょう。
The first clue that the full-scale GPT-5 is being trained comes from the president and co-founder of OpenAI, Greg Brockman.
GPT-5の全スケールトレーニングが行われているという最初の手がかりは、OpenAIの社長兼共同創設者であるグレッグ・ブロックマンから来ています。
Now, first a little bit of context: OpenAI typically trains smaller models of about a thousandth the size before they do a full training run.
まず、少し文脈を説明します。OpenAIは通常、完全なトレーニングランの前に、約1000分の1のサイズの小さなモデルをトレーニングします。
They then gather insights from these smaller models before doing the full training run.
それらの小さなモデルから洞察を得た後、彼らは完全なトレーニングランを行います。
So, that's the backdrop to OpenAI.
つまり、それがOpenAIの背景です。
In Brockman's words, scientifically predicting and understanding the resulting systems is being done what they're now building is maximally harnessing all their computing resources.
Brockmanの言葉によれば、科学的に予測し、結果として生じるシステムを理解することは、彼らが今構築しているものを最大限に活用することです。
They're gathering all of their ideas together and scaling beyond precedent.
彼らはすべてのアイデアを集め、先例を超えてスケールを拡大しています。
Translated, they are training their biggest model yet.
つまり、彼らはこれまでで最も大きなモデルをトレーニングしています。
We'll get to parameters, data, and capabilities in a moment, but first, what's this other tweet?
後でパラメータ、データ、機能について触れますが、まずはもう一つのツイートについて説明します。
This comes from Jason Way, a top OpenAI researcher.
これはトップのOpenAI研究者であるジェイソン・ウェイからのものです。
A few hours after Brockman's tweet, he said, There's no adrenaline rush like launching a massive GPU training.
ブロックマンのツイートの数時間後、彼は「大規模なGPUトレーニングを開始するときほどのアドレナリンラッシュはない」と述べました。
And this got plenty of salutes in the reply from other OpenAI employees.
これに対して、他のOpenAIの従業員からの返信でたくさんの称賛を受けました。
Now, for context, this does not mean that we're imminently going to get GPT-5.
ただし、これはGPT-5が直ちにリリースされることを意味するわけではありません。
GPT-4 took around 3 months to train, and then there was the safety testing.
GPT-4のトレーニングには約3ヶ月かかり、その後に安全性のテストが行われました。
I'm actually going to end this video with my exact prediction of when I think they're going to release GPT-5.
実際に、私はこのビデオを、GPT-5がリリースされると私が思う正確な予測で終了します。
But first, here is a little bit more supporting evidence that they are currently training GPT-5.
しかし、まず最初に、彼らが現在GPT-5のトレーニングを行っているというさらなる裏付けの証拠を少し提供します。
OpenAI updated their blog to say that applications for the red teaming network have closed and that those red teamers would know about the status of their applications by the end of last year.
OpenAIはブログを更新して、レッドチーミングネットワークへの申し込みが締め切られ、そのレッドチーマーたちは昨年末までに申し込みの状況を知ることになると述べました。
What that means is that the red teamers are now in place to start safety testing the new model.
つまり、レッドチーマーたちは新しいモデルの安全性テストを開始するために配置されています。
Now, you might say, What's the point of having those red teamers in place if it's still going to be training for 2 to 3 months?
では、もし2〜3ヶ月間トレーニングが続くのであれば、それらのレッドチーマーを配置する意味は何でしょうか?
Well, before a model is fully trained, it goes through various checkpoints.
まあ、モデルが完全にトレーニングされる前に、さまざまなチェックポイントを通過する必要があります。
Think of them a bit like a video game save.
それらをビデオゲームのセーブポイントのように考えてください。
What that also means is that, in effect, OpenAI will have a GPT-4.2 before they have a GPT-5.
また、それはOpenAIがGPT-5を持つ前にGPT-4.2を持つことを意味します。
Indeed, Greg Brockman, going back to April of last year, said that it might be one of those checkpoints that OpenAI released first.
実際、昨年4月にグレッグ・ブロックマンは、最初にOpenAIがリリースするのはそのようなチェックポイントの1つかもしれないと述べました。
He said that it's easy to create a continuum of incrementally better AIs, such as by deploying subsequent checkpoints of a given training run.
彼は、次のように述べました。次第に優れたAIを展開することは簡単であり、特定のトレーニングランの後続のチェックポイントを展開することによって実現できます。
And he explicitly contrasted that approach and said it would be very unlike our historical approach of infrequent major model upgrades.
そして、彼は明示的にそのアプローチと対比し、これまでのまれな主要なモデルのアップグレードとは非常に異なると述べました。
But remember that even before those checkpoints, OpenAI would have already gotten a glimpse of GPT-5's capabilities from those smaller earlier versions of the model.
ただし、それらのチェックポイントの前にも、OpenAIはモデルのより小さな以前のバージョンからGPT-5の能力の一端を既に把握しているはずです。
Indeed, Sam Altman back in November said that he was privileged to be in the room when they push back the veil of ignorance.
実際、昨年11月にサム・アルトマンは、彼らが無知のベールを引き裂く場面に立ち会ったと述べました。
I'm super excited.
私はとても興奮しています。
I can't imagine anything more exciting to work on.
私が取り組むのにもっとも興奮することは想像できません。
And on a personal note, like four times now in the history of OpenAI, the most recent time was just in the last couple of weeks, I've gotten to be in the room when we sort of push the front, the sort of the veil of ignorance back and the frontier of discovery forward.
そして、個人的なメモとして、OpenAIの歴史の中で4回も、最近のものは数週間前になりますが、私たちは無知のベールを引き裂き、発見のフロンティアを前に押し進める部屋にいることができました。
And getting to do that is like the professional honor of a lifetime.
それをすることは、人生でのプロの名誉のようなものです。
It all points to OpenAI in November and December having trained those smaller versions of GPT-5, again with the purpose of scientifically predicting and understanding the resulting GPT-5 system.
これらすべては、11月と12月のOpenAIがGPT-5のより小さなバージョンをトレーニングしたことを示しています。それは科学的な予測と結果のGPT-5システムの理解を目的としています。
So, they know it's going to be good, but just how good and how big and what are these new or old ideas that they're going to incorporate?
したがって、彼らはそれが良いことを知っていますが、それがどれほど良く、どれほど大きく、そしてどのような新しいまたは古いアイデアを取り入れるのでしょうか?
Well, one thing that seems almost certain is that they're going to incorporate a way to let GPT-5 think for longer.
まあ、ほぼ確実なことは、彼らがGPT-5がより長く考える方法を取り入れることだと思われます。
In other words, it's going to lay out its reasoning steps before solving a challenge and have each of those reasoning steps checked internally or externally.
言い換えれば、課題を解決する前にその推論のステップを示し、それぞれの推論のステップを内部または外部でチェックする方法を持つことになります。
Here's Sam Altman a few days ago at Davos: What it means to verify or understand what's going on is going to be a little bit different than people think right now.
ここ数日、サム・アルトマンはダボスで次のように述べています。「現在の人々が考えているようなものとは異なる方法で、何が起こっているかを検証または理解することの意味が変わるでしょう」。
I actually can't look in your brain and look at the 100 trillion synapses and try to understand what's happening in each one and say,Okay, I really understand why he's thinking what he's thinking.
実際には、私はあなたの脳を見ることも、100兆のシナプスを見て、それぞれで何が起こっているのかを理解しようとすることもできません。だから、彼が何を考えているのかを本当に理解することはできません。
You're not a black box to me.
あなたは私にとってブラックボックスではありません。
But what I can ask you to do is explain to me your reasoning.
しかし、私があなたにお願いできるのは、あなたの推論を私に説明してもらうことです。
I can say,You know, you think this thing, why?
私は「あなたはこのことを考えている、なぜですか?」と尋ねることができます。
And you can explain,First this, then this, then there's this conclusion, then that one.
そして、あなたは「まずこれ、次にこれ、そしてこの結論、それからあれ」と説明することができます。
And then there's this, and I can decide if that sounds reasonable to me or not.
そして、それからこれがあります、そして私はそれが私にとって合理的に思えるかどうかを判断することができます。
And I think our AI systems will also be able to do the same thing.
そして、私たちのAIシステムも同じことができるようになるでしょう。
They will be able to explain to us in natural language the steps from A to B, and we can decide whether we think those are good steps.
彼らは私たちに自然言語でAからBへのステップを説明することができ、私たちはそれが良いステップかどうかを判断することができます。
And a few days before that, Sam Altman told Bill Gates that that might involve asking GPT-4 or GPT-5 the same question 10,000 times.
そして、その数日前、サム・アルトマンはビル・ゲイツに対して、それはGPT-4またはGPT-5に同じ質問を10,000回繰り返すことを含むかもしれないと述べました。
You know, when you look at the next two years, what do you think some of the key milestones will be?
次の2年間を見ると、重要なマイルストーンのいくつかは何だと思いますか?
Multimodality will definitely be important.
マルチモダリティは間違いなく重要になるでしょう。
Which means speech in, speech out?
それは言葉が入力として与えられ、言葉が出力として返ってくることを意味しますか?
Speech in, speech out, Images, eventually video.
言葉を入力とし、言葉を出力とすることから始まり、最終的には画像、そしてビデオに至ることを意味します。
Clearly, people really want that.
明らかに、人々はそれを本当に望んでいます。
We launched images and audio, and it had a much stronger response than we expected.
私たちは画像と音声をリリースしましたが、それには予想以上の反応がありました。
We'll be able to push that much further.
私たちはそれをさらに進めることができるでしょう。
But maybe the most important areas of progress will be around reasoning ability.
しかし、おそらく最も重要な進歩は推論能力の周りで起こるでしょう。
Right now, GPT-4 can reason in only extremely limited ways.
現時点では、GPT-4は非常に限定的な方法で推論することができます。
And also reliability.
そして信頼性も同様です。
If you ask GPT-4 most questions 10,000 times, one of those 10,000 is probably pretty good, but it doesn't always know which one.
GPT-4に同じ質問を10,000回しても、その中の1つはおそらくかなり良い回答ですが、常にどれが良いかはわかりません。
And you'd like to get the best response of 10,000 each time.
そして、毎回1万の最良の応答を得たいと思っています。
That increase in reliability will be important.
信頼性の向上は重要になるでしょう。
And at this point, watchers of my channel will know exactly what he's referring to.
そして、私のチャンネルの視聴者は彼が何を指しているのかを正確に知っています。
Both of those approaches, checking your reasoning steps and sampling up to 10,000 times, are incorporated into OpenAI's Let's Verify step-by-step paper.
これらのアプローチ、推論ステップのチェックと最大10,000回のサンプリングは、OpenAIの「ステップバイステップで検証しましょう」という論文に組み込まれています。
Now, I'm not going to dive into the details of Let's Verify in this video because I've got at least two previous videos on the topic.
今回のビデオでは「ステップバイステップで検証しましょう」の詳細には触れませんが、このトピックに関する少なくとも2つの以前のビデオがあります。
But notice in the paper how many times they sample GPT-4.
しかし、論文を見ると、彼らがGPT-4を何度もサンプリングしていることに注目してください。
The chart shows what happens when you sample the model over a thousand times and pick out the best responses.
このチャートは、モデルを1,000回以上サンプリングし、最良の回答を選び出した場合の結果を示しています。
And notice something about this process, a supervised way of doing things.
そして、このプロセスについて何かに気づくでしょう、つまり、教師ありの方法です。
The results are continuing to go up.
結果は引き続き向上しています。
I can't resist showing you a quick example of the reasoning steps broken down into separate lines and essentially a verifier looking in and checking which steps are accurate or inaccurate.
私は、推論のステップを別々の行に分解し、本質的には検証者が内部を見て、どのステップが正確か不正確かをチェックする例をお見せするのが我慢できません。
The answers for whom each step in the reasoning process got a thumbs up were the ones submitted, and the results were dramatic.
推論プロセスの各ステップについて肯定的な評価を受けた回答が提出され、その結果は劇的でした。
Essentially, this process of sampling the model thousands of times and taking the answer that had the highest rated reasoning steps doubled the performance in mathematics.
要するに、モデルを何千回もサンプリングし、最も評価の高い推論ステップを持つ回答を選ぶこのプロセスは、数学のパフォーマンスを2倍にしました。
And no, this didn't just work for mathematics.
そして、これは数学だけでうまくいったわけではありません。
It had dramatic results across the STEM fields.
STEM分野全体に劇的な結果をもたらしました。
And remember, this was using GPT-4 as a base model, not GPT-5, and it was only 2,000 samples, not 10,000 like Sam Altman talked about.
そして覚えておいてください、これはGPT-4をベースモデルとして使用したものであり、GPT-5ではなく、サンプル数はサム・アルトマンが話した10,000ではなく2,000だけでした。
So this is the evidence I would present to someone who said that LLMs have peaked.
これが、大規模言語モデルがピークに達したと言う人に提示する証拠です。
If OpenAI can incorporate, through parallelization, a way to get the model to submit and analyze 10,000 responses, the results could be truly dramatic.
もしOpenAIが並列化を通じてモデルに10,000回の回答を提出し、分析する方法を組み込むことができれば、結果は本当に劇的になるかもしれません。
Indeed, the Let's Verify paper from OpenAI repeatedly cited this earlier DeepMind paper on solving math problems with process-based feedback.
実際、OpenAIの「ステップバイステップで検証しましょう」という論文では、数学の問題をプロセスベースのフィードバックで解決するためのこの以前のDeepMindの論文が繰り返し引用されています。
And for coding, we know AlphaCode 2 from Google DeepMind used a mass sampling approach to get an 87th percentile score on a coding contest.
そして、コーディングに関しては、Google DeepMindのAlphaCode 2が大量のサンプリングアプローチを使用してコーディングコンテストで87パーセンタイルのスコアを叩き出したことがわかっています。
In other words, it beat 87% of participants in this Codeforces coding challenge.
言い換えれば、このCodeforcesのコーディングチャレンジで参加者の87%を上回りました。
For context, the GPT-4 that we got scored around the fifth percentile, a score of 400 in the Codeforces challenge.
文脈を考えると、私たちが手に入れたGPT-4はコードフォースのチャレンジで5パーセンタイル程度のスコアでした。
These numbers are a little out of date, but AlphaCode 2 would have scored around here, expert or candidate master level.
これらの数字は少し古いですが、AlphaCode 2はここ、エキスパートまたは候補マスターレベルでスコアを叩き出したでしょう。
Or to just translate everything, if they find a way to let GPT-5 think, it could be night and day in terms of performance for coding, mathematics, and STEM.
また、すべてを翻訳すると、もしGPT-5に思考させる方法を見つけることができれば、コーディング、数学、およびSTEM分野のパフォーマンスに関しては、昼夜の違いとなるかもしれません。
But just how big will GPT-5 be that's doing all of this parallel thinking?
しかし、この並列思考を行うGPT-5はどれほど大きくなるのでしょうか?
Well, for AI insiders, I interviewed Gavin Uberti, the CEO and co-founder of Etched AI, which I've also talked about on this channel.
AIの内部者には、私がこのチャンネルでも話したEtched AIのCEO兼共同創設者であるギャビン・ウベルティ氏にインタビューしました。
He is a 21-year-old dropout from Harvard University, and on his LinkedIn profile, it says he's building the hardware for superintelligence.
彼はハーバード大学を中退した21歳であり、彼のLinkedInプロフィールには「超知能のためのハードウェアを構築している」と書かれています。
In the interview, he guessed that GPT-5 would have around 10 times the parameter count of GPT-4.
インタビューでは、彼はGPT-5のパラメータ数がGPT-4の約10倍になると推測しています。
According to leaks, GPT-4 has around 1.5 to 1.8 trillion parameters.
リークによると、GPT-4のパラメータ数は約1.5兆から1.8兆です。
But just quickly, what did he mean when he said that he expects that to come from a combination of a larger embedding dimension, more layers, and double the number of experts?
ただ、簡単に言うと、彼が言ったことは、それがより大きな埋め込み次元、より多くの層、そして専門家の数を2倍にする組み合わせから期待されるということですか?
Well, think of the embedding dimension as being about how granular the training can be about each token and its context.
埋め込み次元は、トークンとその文脈についてのトレーニングの詳細さを表すものと考えてください。
A bigger embedding dimension means more granularity and nuance about each token.
より大きな埋め込み次元は、各トークンについての詳細さと微妙なニュアンスを意味します。
And doubling the number of layers allows a model to develop deeper pattern recognition.
そして、層の数を2倍にすることで、モデルはより深いパターン認識を開発することができます。
It allows it to see more complex patterns within patterns within patterns.
それによって、パターンの中のパターンの中の複雑なパターンを見ることができます。
More highlights from that interview will be coming on AI Insiders on Patreon in the coming weeks.
そのインタビューからのさらなるハイライトは、AI InsidersのPatreonで今後数週間にわたって公開されます。
But during this video on GPT-5, I promised you two interludes, one focused on DALL·E 3 and one a practical tip for using chat anytime.
しかし、このGPT-5のビデオでは、DALL·E 3に焦点を当てた2つのインターリュード、およびチャットの使用に関する実用的なヒントの約束をしました。
Well, here is the first of those two interludes focused on a particular quirk of DALL·E 3.
さて、それら2つのインターリュードの最初は、DALL·E 3の特定のクセに焦点を当てたものです。
I say that, but this trick also works for Midjourney.
と言っても、このトリックはMidjourneyでも機能します。
Now, many of you might have noticed a trend on TikTok, Reddit, Twitter, and YouTube shorts where people post an image.
多くの方々がTikTokやReddit、Twitter、YouTube Shortsで画像を投稿し、徐々に強烈にしていく傾向に気付いたかもしれません。
And then, make it progressively more intense.
そして、さらに奇妙なものを言えば、これよりも議論の余地があるものがあります。
Let's say, well, here's something arguably even more quirky.
さて、ここでさらに奇妙なものを紹介します。
I got the original idea from Peter Werf on Twitter and decided to make it more intense.
私はTwitterのピーター・ウェルフから元のアイデアを得て、それをより強烈にしました。
But first, I asked, Draw an image of a London scene, but don't use lampost in the image.
最初に、私は尋ねました。「ロンドンの風景のイメージを描いてくださいが、イメージには街灯を使用しないでください」。
And lo and behold, we get dozens of lamposts.
そして、見てください、私たちはたくさんの街灯を手に入れました。
I mean, in this one, we have a lampost coming down from the sky.
つまり、この1つでは、空から街灯が降りてきています。
And what does GPT-4 say in analyzing these?
GPT-4はこれらを分析して何と言っているのかと言いますか?
It says that these are two images of a London Street Scene without lamposts.
それによると、これらは街灯のないロンドンの街のシーンの2つのイメージだと言っています。
So then, I said, Now make these images with even fewer lamposts, stripping any lampost references completely.
それで、私は言いました。「これらのイメージをさらに街灯の少ないものにしてください。街灯の参照を完全に剥奪してください」。
And here were the results.
そして、ここに結果があります。
As you can see on the right, there's barely a lamppost in sight.
右側を見ていただくと、ほとんど街灯が見えません。
And as GPT-4 says, these images were created with complete omission of any lamppost references.
GPT-4によると、これらのイメージは街灯の参照を完全に省略して作成されました。
Then I said, Delete absolutely everything that pertains to a lamppost.
それから、私は言いました。「街灯に関連するものは絶対にすべて削除してください」。
And I don't know about you, but I can't see any lampposts left.
私にはわかりませんが、街灯はもう見えません。
And finally, I said, Take this to the Max and give me an image that someone could not picture a lamppost existing within, even in their wildest imagination.
最後に、私は言いました。「これを最大限に活用して、誰もが自分の最も想像力のある中でも街灯が存在しないと思えるようなイメージを作ってください」。
Now, I think it's pretty cute that the lamppost persisted throughout these images, and I suspect that the deeper reason is that the caption training that DALL·E 3 got didn't have many examples of omission.
今、これらのイメージの中で街灯が残っているのはかなりかわいいと思いますし、DALL·E 3が受けたキャプショントレーニングには省略の例があまりなかったという深い理由があると疑っています。
They used web captions and synthetic captions, but I doubt there were many examples of people saying, This image does not contain X. But speaking of modality, the first thing they want to fix, apparently for GPT-5, is the real-time nature of the voice interaction.
彼らはウェブのキャプションと合成のキャプションを使用しましたが、おそらく「このイメージにはXが含まれていません」と言う人々の例はあまりなかったでしょう。しかし、モダリティについて話すと、明らかにGPT-5のために修正したい最初のことは、音声インタラクションのリアルタイム性です。
At the moment, there is quite a bit of time to First token latency.
現時点では、最初のトークンの待ち時間がかなりあります。
In other words, it takes a bit too long to reply.
言い換えれば、返信するのに少し時間がかかります。
Here's Sam Altman speaking last week.
ここに先週のサム・アルトマンのスピーチがあります。
I think there's all sorts of the current stuff that people complain about, like the voice is too slow, and you know it's not real-time, and that'll get better this year.
人々が文句を言っている現在の問題は、声が遅すぎることやリアルタイムではないことなどですが、今年はそれが改善されるでしょう。
I think where we're headed, and then I'll talk about this year, is we're headed towards the way you use computers to talk to it.
私たちが向かっている方向、そしてそれから今年について話しますが、私たちはコンピュータとの対話にコンピュータを使用する方法に向かっています。
The operating system of a computer, in some sense, is close to this idea that you're like working inside of a chat experience.
コンピュータのオペレーティングシステムは、ある意味ではチャット体験の中で作業しているようなものです。
When he mentioned using an LLM as an operating system, he was drawing on Andre Kathy's Vision.
彼がLLMをオペレーティングシステムとして使用すると言ったとき、彼はアンドレ・キャシーのビジョンを引用していました。
I've talked about it before, but notice at the top that it's video In and Out, audio in and out, and it's not like OpenAI is hiding it.
以前にも話しましたが、上部に注目してください。ビデオの入力と出力、音声の入力と出力であり、OpenAIがそれを隠しているわけではありません。
They want as much text, image, audio, and video data as they can get their hands on.
彼らはできるだけのテキスト、画像、音声、ビデオデータを手に入れたいと考えています。
They also want what I'm going to call reasoning data, data that expresses human intention they call it.
彼らはまた、私が「推論データ」と呼ぶものを求めています。それは人間の意図を表すデータで、彼らはそう呼んでいます。
Now, I didn't notice that phrase at the time of this blog post in November, but it fits in clearly with what I was saying earlier about let's verify.
私は11月のこのブログ投稿の時点ではそのフレーズに気づきませんでしたが、私が以前に言っていたこととは明らかに一致しています。つまり、検証しましょう。
Think about it, how would you make a model agentic, able to solve more complex challenges?
考えてみてください、モデルをエージェンティックにするにはどうすればよいでしょうか?より複雑な課題を解決できるようにするために。
Well, if GPT-5 gets loads of data about people laying out plans full of human intention, GPT-5 could learn to imitate those schemes and plans and maybe have a verifier internally or externally judging those reasoning steps.
もちろん、GPT-5が人間の意図を含んだ計画をたくさんのデータとして取得すれば、GPT-5はそれらの計画とスキームを模倣し、内部または外部の検証者がその推論ステップを判断することができるようになるかもしれません。
Now, as for the question of whether those reasoning steps faithfully represent what the model is internally calculating, that will have to be for another day.
ただし、モデルが内部的に計算しているものを忠実に表現しているかどうかという問題については、別の日になるでしょう。
This paper from Anthropic back in July said that as models become larger and more capable, they actually produce less faithful reasoning on most tasks we study.
Anthropicのこの論文は、モデルが大きくなり、能力が向上するにつれて、ほとんどのタスクで忠実な推論を出力しなくなると述べています。
That doesn't mean getting the answer wrong more often.
それはより頻繁に間違った答えを出すという意味ではありません。
It means outputting reasoning steps that don't actually reflect what it's internally calculating.
それは、実際には内部で計算しているものを反映していない推論ステップを出力することを意味します。
So, GPT-5 may end up being an excellent productivity assistant while still being somewhat inscrutable on a deeper level.
したがって、GPT-5はより深いレベルでは理解しがたいままであるにもかかわらず、優れた生産性アシスタントになるかもしれません。
Just quickly before we leave data, I think there's one thing that we can safely say, which is that there will be much more multilingual data in GPT-5 training set.
データを離れる前に、GPT-5のトレーニングセットにははるかに多くの多言語データが含まれると断言できることが1つあります。
OpenAI has formed so many data partnerships, including with people like the Icelandic government, and there are so many more multilingual data sets being open-sourced that I think it's almost inevitable that there will be a dramatic forward step in GPT-5's multilingual abilities.
OpenAIは、アイスランド政府などとのデータ提携を含め、多くのデータセットがオープンソース化されているため、GPT-5の多言語能力が劇的に向上することはほぼ避けられないと思います。
Partly, this is a safety thing too, with OpenAI wanting its red teamers to be fluent in more than one language.
これは安全性の問題でもあり、OpenAIはレッドチームのメンバーが1つ以上の言語に堪能であることを望んでいます。
Models are notoriously easier to jailbreak in different languages, and it looks like OpenAI is working hard on that front.
モデルは異なる言語で簡単にジェイルブレイクされる傾向があり、OpenAIはその点で一生懸命取り組んでいるようです。
But there is one language that I bet you didn't know GPT-4 can already speak, and that's the language of, according to this fascinating paper from Tokyo, GPT-4 can almost perfectly handle unnatural scrambled text.
ただし、GPT-4が既に話すことができる言語が1つあります。それは、東京のこの興味深い論文によると、GPT-4はほとんど完璧に不自然なスクランブルされたテキストを処理できるということです。
Now, you might already know that humans have this ability.
おそらく、人間にはこの能力があることをすでに知っているかもしれません。
If the first and last letter of a word is the same, you can often still recognize the word.
単語の最初と最後の文字が同じ場合、単語をしばしば認識することができます。
But GPT-4, and obviously GPT-5, will be able to go a step further.
しかし、GPT-4、そして明らかにGPT-5はさらに進むことができます。
Even if the first and last letter are different, if a word is completely scrambled, it can recover the sentence.
最初と最後の文字が異なる場合でも、単語が完全にスクランブルされている場合、文を復元することができます。
I tested it out, and indeed, it works.
私はそれをテストしてみましたが、実際には機能します。
Just look at how utterly garbled this sentence is.
この文がどれだけ完全にごちゃごちゃになっているかを見てください。
For me and you, that would be almost complete gobble.
私やあなたにとってはほとんど理解不能なものになるでしょう。
But GPT-4 was able to recognize what I'm saying.
しかし、GPT-4は私が言っていることを認識することができました。
So, that is the practical tip that I also wanted to give you in this video.
したがって、これは私がこのビデオであなたに伝えたかった実用的なヒントです。
If you have a quick request of GPT-4, don't bother going back and spending 30 seconds correcting all your typos.
GPT-4に素早いリクエストをする場合、すべてのタイプミスを修正するために戻って30秒を費やす必要はありません。
Trust me, I've been guilty of this in the past because I love perfect English.
信じてください、私も過去にこれに罪を犯しました。完璧な英語が大好きなので。
But if you have a letter or two out of place, don't worry.
しかし、文字が1つまたは2つ間違っていても心配しないでください。
It will understand.
理解するでしょう。
To be honest, if it can unscramble this, it can understand your typo of the word there, your missing comma, and all the rest of it.
正直に言って、もしこれを解読できるなら、そこでのタイプミス、欠落したカンマ、そしてその他のすべてを理解できるでしょう。
So, save yourself 30 seconds and don't even bother correcting your typos.
だから、30秒を節約して、タイプミスを修正する必要はありません。
But now, it's time for me to finally give my prediction for when GPT-5 is going to be released.
しかし、今は、私がいよいよGPT-5のリリース予測をする時です。
For the last few weeks, I've honestly thought it would be around September of this year.
ここ数週間、正直に言って、今年の9月ごろになると思っていました。
But now, I think it will be toward the end of November of 2024.
しかし、今は、2024年11月末になると思います。
And no, that's not just because that would be the 2-year anniversary of the release of ChatGPT, the original version.
いいえ、それは単にChatGPT、元のバージョンのリリースの2周年になるからではありません。
First, let me clarify that I don't think they will release the full capabilities of GPT-5 on the first go.
まず、私は彼らが最初の段階でGPT-5の全機能をリリースしないと思うと明確にしておきます。
As mentioned, I think they'll release different checkpoints, different functionalities as we head into 2025.
先述のように、2025年に向けて異なるチェックポイント、異なる機能をリリースすると思います。
But what explains the delay from now when they're training it all the way to November?
しかし、なぜ今から11月までの遅延が説明されるのでしょうか?
Well, first of all, as mentioned, it does take a few months to train a model the size of GPT-5.
まず第一に、先述のように、GPT-5のようなモデルのトレーニングには数ヶ月かかります。
Yes, they might be able to use, say, 100,000 GPUs from NVIDIA, but training a model has hiccups.
そうです。彼らはNVIDIAの10万台のGPUを使用できるかもしれませんが、モデルのトレーニングには問題が生じます。
And of course, the model will be much larger.
そしてもちろん、モデルははるかに大きくなります。
But let's say that takes around 2 months.
しかし、それには約2ヶ月かかるとしましょう。
That would bring us to the end of March.
それにより、私たちは3月末になります。
Now, here's the key point.
ここで重要なポイントです。
Sam Altman has boasted many times in the past about how they tested GPT-4 for 6 to 8 months before releasing it.
サム・アルトマンは過去に何度もGPT-4のリリース前に6〜8ヶ月間テストしたことを自慢しています。
It would be pretty awkward for OpenAI to have even less safety testing for GPT-5.
GPT-5に対してもさらに少ない安全性テストを行うのはかなり気まずいでしょう。
So, add 6 months to the end of March, and you get to the end of September.
だから、3月末から6ヶ月を加えると、9月末になります。
Of course, add 8 months, and you get to the end of November.
もちろん、8ヶ月を加えると、11月末になります。
So, why end of November rather than end of September?
では、なぜ9月末ではなく11月末なのでしょうか?
Well, I think OpenAI will want to steer clear of what will be an incredibly contentious American election.
それは、非常に論争の的となるアメリカの選挙から距離を置きたいとOpenAIが考えるからだと思います。
If they release even an alpha version of GPT-5 with, say, video and audio before that election, they could come under incredible flack.
もし彼らが選挙前にビデオや音声などを含むGPT-5のアルファ版をリリースしたら、彼らは信じられないほどの非難を浴びる可能性があります。
As they say on their website, they're still working to understand how effective their current tools might be for personalized persuasion.
彼らのウェブサイトで言っているように、彼らは現在のツールが個別の説得にどれだけ効果的かを理解するためにまだ取り組んでいます。
That would be stepping into a minefield.
それは地雷原に足を踏み入れることになるでしょう。
In the recent New Hampshire election, we already saw robo-calls imitating Joe Biden.
最近のニューハンプシャー州の選挙では、ジョー・バイデンを模倣したロボコールが既に行われています。
What a bunch of malarkey.
なんてばかげたことだ。
We know the value of voting Democratic.
私たちは民主党に投票する価値を知っています。
When our votes count, it's important that you save your vote for the November election.
私たちの投票が重要なときには、11月の選挙のために投票を取っておくことが重要です。
So, November 30th, as well as being a symbolic date, would stay clear of that election.
だから、11月30日は象徴的な日付であり、またその選挙から距離を置くために選ばれるでしょう。
You might say, What about 2025?
では、2025年はどうなるのでしょうか?
But I think the incentives of Moloch will prevent them delaying too long.
しかし、モロックのインセンティブが彼らがあまりにも長く遅延することを防ぐだろうと思います。
In not too long, we might be getting the release of Gemini Ultra, not to mention Gemini 2 Ultra, and of course, LLaMA 3 from Meta, announced by Zuckerberg.
それほど遠くない将来、Gemini Ultraのリリース、そしてもちろん、ザッカーバーグによって発表されるMetaのGemini 2 UltraやLLaMA 3のリリースが予想されます。
When everyone else has caught up, they might feel compelled to release the next model.
他のみんなが追いついた時、彼らは次のモデルをリリースすることを強いられるかもしれません。
And Anthropic might choose a similar time to release Claude 3.
そしてAnthropicは、Claude 3をリリースするために似たような時期を選ぶかもしれません。
Here is the CEO of Anthropic, Dario Amade, giving his rough predictions for GPT-5 and Claude 3.
ここにAnthropicのCEOであるダリオ・アマデが、GPT-5とClaude 3の大まかな予測を述べています。
What do you think happens on the next major training run for LLM?
LLMの次の主要なトレーニングランでは何が起こると思いますか?
My guess would be nothing truly insane happens.
私の予想では、本当に狂ったことは起こらないでしょう。
Say, in any training run that happens in 2024 to really invent new science, the ability to cure diseases, the ability to make bioweapons, yeah, maybe someday but the Dyson fears.
もし2024年に行われるどんな研究でも、新しい科学を本当に創造し、病気を治す能力や生物兵器を作る能力を持つ可能性があるかもしれませんが、それはダイソンの恐怖かもしれませんね。
The least impressive of those things, I think will happen.
それらの中で最も印象に残らないことは、おそらく実現するでしょう。
I would say no sooner than 2025, maybe 2026.
2025年以降になると思いますが、おそらく2026年以降になるでしょう。
I think we're just going to see in 2024, more commercially applicable versions of the models that exist today.
私は2024年には、今日存在するモデルのより商業的に適用可能なバージョンが見られると思います。
Like, we've seen a few of these generations of jumps.
いくつかのジェネレーションの飛躍を見てきました。
I think in 2024, people are certainly going to be surprised.
私は2024年には、人々が驚くことになると思います。
Like, they're going to be surprised at how much better these things have gotten.
つまり、これらのものがどれだけ良くなったかに驚くことになるでしょう。
But it's not going to quite bend reality yet.
しかし、まだ現実を曲げるほどではありません。
Of course, I have to say at the end of this video that no one truly knows, not even OpenAI, what GPT-5 will be like.
もちろん、このビデオの最後に言わなければならないのは、GPT-5がどのようなものになるか、OpenAIでさえも誰も本当のことを知らないということです。
As Sam Altman recently said, Until we go train that model, GPT-5, it's like a fun guessing game for us.
最近、サム・アルトマンは、「GPT-5というモデルをトレーニングするまで、それは私たちにとって楽しい推測のゲームのようなものです」と言いました。
I can't tell you, here's exactly what it's going to do that GPT-4 didn't.
私はあなたに、GPT-4がしなかったことをGPT-5が具体的にどのようにするのかを教えることはできません。
And here's Greg Brockman with a similar message.
そして、ここにグレッグ・ブロックマンも同様のメッセージを伝えます。
Right, that is the biggest theme in the history of AI.
AIの歴史において、それが最も大きなテーマであるというのは正しいです。
It's that it's full of surprises.
それは驚きに満ちているということです。
Every time you think you know something, you scale it up 10x.
何かを知っていると思ったら、それを10倍にスケールアップさせるんです。
Turns out you knew nothing.
結局、何も知らなかったことになります。
And so, I think that we, as a humanity, as a species, are really exploring this together.
そして、私たちは人類として、種として、本当に一緒にこれを探求していると思います。
And then, we get cryptic messages like this from senior members of OpenAI.
そして、OpenAIの上級メンバーからこんな謎めいたメッセージを受け取ります。
Ben Newhouse says he's hiring at OpenAI, and we're building what I think could be an industry-defining zero to one product that leverages the latest and greatest from our upcoming models, i.e., GPT-5.
ベン・ニューハウスはOpenAIでの採用を行っていると述べており、私たちは業界を定義づける可能性のある「ゼロからワン」への製品を作成していると思っています。それは、間もなく登場する最新かつ最高のモデル、例えばGPT-5の先進技術を活用しています。
Two other OpenAI employees replied like this: This product will change everything.
他の2人のOpenAIの従業員もこう答えました: この製品はすべてを変えるでしょう。
And what they said, of course, it would be pure speculation to guess what they mean with this.
そして、彼らがこれをどういう意味で言っているのかを推測するのは純粋な憶測になるでしょう。
So, those were my predictions.
それが私の予測でした。
I try to base them on evidence rather than idle speculation.
私はそれらを根拠に基づいて立てるようにしています。ただの憶測ではなく。
I do try not to be lazy like GPT-4 has in the past been accused of being.
私はGPT-4が過去に怠惰だと非難されたように、怠け者にならないように心がけています。
If you like this kind of thing, I would invite you to see more exclusive premium content on AI Insiders on Patreon.
もしこのようなことが好きなら、AI InsidersのPatreonでより多くの独占プレミアムコンテンツをご覧いただければと思います。
But for everyone watching to the end, I want to thank you so much for being here, and I want to wish you a wonderful day.
最後までご視聴いただいた皆さんに、本当にありがとうございます。素晴らしい一日をお過ごしください。
この記事が気に入ったらサポートをしてみませんか?