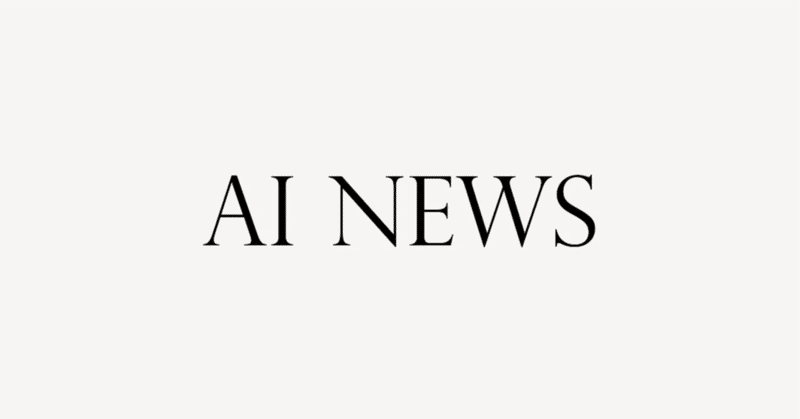
【CLIN:革新的なAI言語モデル】英語解説を日本語で読む【2023年10月28日|@AI Revolution】
この動画ではAIの言語モデル「CLIN」について紹介しています。CLINは自己学習・適応能力があり、未経験のタスクでも対応可能なゼロショットパフォーマンスを持ちます。独自のグローバルメモリとローカルメモリを用い、長期と短期の情報を効果的に利用します。一方、人間の学習は感情や社会的要素を含むが、AIは情報量の多い学習が得意です。CLINのメモリシステムと学習方法を理解することで、AIと人間の学習の違いと価値を把握できます。
公開日:2023年10月28日
※動画を再生してから読むのがオススメです。
Could a language model actually outsmart humans someday?
言語モデルはいつか実際に人間を凌駕することができるのだろうか?
This question is becoming more relevant with CLIN's advancements in AI learning.
この疑問は、AI学習におけるCLINの進歩によって、より適切なものになりつつある。
CLIN is a groundbreaking language model in AI, constantly learning and adapting to new tasks and environments all on its own.
CLINはAIにおける画期的な言語モデルであり、常に学習し、新しいタスクや環境に適応していく。
This is thanks to its pure zero-shot setup, which allows it to learn from interactions and feedback without needing extra adjustments.
これは、余分な調整を必要とせずに相互作用やフィードバックから学習することができる、純粋なゼロショット設定のおかげである。
Let's take a closer look at what makes CLIN stand out.
CLINが傑出している理由を詳しく見てみよう。
I'll be explaining how it works and why it's such an important advancement in artificial intelligence.
その仕組みと、なぜそれが人工知能における重要な進歩なのかを説明しよう。
First, let's understand what CLIN is and its function.
まず、CLINとは何か、その機能を理解しよう。
CLIN, short for Continually Learning from Interactions, is a system designed to help language agents quickly get better at what they do through repeated experiences.
CLINとは、Continually Learning from Interactionsの略で、言語エージェントが繰り返し経験することで、自分たちのすることが素早く上手になるように設計されたシステムです。
A language agent is essentially a computer program that communicates with the outside world in a way we can understand, like through text or speech.
言語エージェントとは基本的に、テキストや音声など、私たちが理解できる方法で外界とコミュニケーションするコンピューター・プログラムのことだ。
This could mean a chatbot having conversations with people, a video game character that follows your instructions, or even a tool that writes computer code.
これは、人と会話をするチャットボットや、あなたの指示に従うビデオゲームのキャラクター、あるいはコンピューター・コードを書くツールなどを意味する。
We actually need language agents like CLIN because they can adapt to our complex and ever-changing world without constant supervision or retraining.
CLINのような言語エージェントは、常に監視したり再教育したりすることなく、複雑で変化し続ける世界に適応できるため、実際に必要なのだ。
Imagine a personal assistant that learns from your feedback to better assist you, a game character that evolves based on your play style, or a code generator that becomes more efficient through your corrections.
あなたのフィードバックから学習し、より良いアシストをしてくれるパーソナルアシスタント、あなたのプレイスタイルに基づいて進化するゲームキャラクター、あるいはあなたの修正によってより効率的になるコードジェネレーターを想像してみてほしい。
That's the goal of CLIN.
それがCLINの目標だ。
To see how well CLIN works, researchers used Science World, a virtual environment where the agent uses natural language to interact with objects and complete science-related tasks like growing plants or making ice cream.
研究者たちは、CLINがどの程度機能するかを確認するために、エージェントが自然言語を使ってオブジェクトと対話し、植物を育てたりアイスクリームを作ったりするような科学関連のタスクを完了する仮想環境、サイエンス・ワールドを使用した。
This tests CLIN's ability to learn and adapt in dynamic scenarios.
これは、CLINがダイナミックなシナリオの中で学習し適応する能力をテストするものである。
Science World is not an easy environment for language agents because it requires them to have both scientific knowledge and reasoning skills.
サイエンス・ワールドは言語エージェントにとって容易な環境ではない。科学的知識と推論スキルの両方が要求されるからだ。
Moreover, Science World has different levels of difficulty depending on the task and the environment.
さらに、Science Worldは、課題や環境によって難易度が異なります。
The tasks are divided into two categories: short and long.
タスクは短いものと長いものの2種類に分けられる。
Short tasks are simple and straightforward, such as boil water or measure mass.
短いタスクは、水を沸騰させたり、質量を測定したりするような単純明快なものである。
Long tasks are complex and multi-step, such as grow a plant or make ice cream.
長いタスクは、植物を育てたりアイスクリームを作ったりするような、複雑で複数のステップを要するものです。
The environments are also varied and diverse, ranging from familiar settings like kitchens or gardens to unfamiliar ones like deserts or volcanoes.
環境も、キッチンや庭のような見慣れたものから、砂漠や火山のような見慣れないものまで、変化に富んでいる。
CLIN excels in Science World due to its versatility and adapting to different scenarios.
CLINがサイエンス・ワールドで優れているのは、その多用途性とさまざまなシナリオへの適応力だ。
It's adept at learning tasks within a specific environment, transferring knowledge across various environments or tasks, and even handling situations that combine both adaptation and generalization.
特定の環境内でタスクを学習することも、さまざまな環境やタスクにまたがって知識を伝達することも、さらには適応と汎化の両方を兼ね備えた状況に対応することも得意だ。
To start, CLIN's ability to adapt is impressive.
まず、CLINの適応能力は素晴らしい。
It learns from its experiences in a particular environment, becoming more efficient over time.
特定の環境で経験から学び、時間とともにより効率的になります。
For instance, in learning to boil water, it understands the steps and refines its approach, like turning on the stove and monitoring the boiling process.
例えば、お湯を沸かすことを学習する際、コンロのスイッチを入れ、沸騰する過程を監視するなど、手順を理解し、アプローチを洗練させていく。
More impressively, CLIN can apply its learned skills to new environments or tasks without needing extra training.
さらに驚くべきことに、CLINは学習したスキルを新たな環境や作業に応用することができる。
For example, if it masters boiling water in a kitchen, it can use that knowledge in a desert using different tools.
例えば、キッチンでお湯を沸かすことをマスターすれば、その知識を砂漠で別の道具を使って使うことができる。
Similarly, if it learns to grow a plant in a garden, it can adapt this skill to grow one in a volcano with different resources.
同様に、庭で植物を育てることを学べば、火山で異なる資源を使って植物を育てることができる。
Lastly, CLIN's most striking ability is in scenarios requiring both generalization and adaptation.
最後に、CLINの最も顕著な能力は、一般化と適応の両方を必要とするシナリオにおいて発揮される。
It uses its broad experience to quickly adjust to completely new tasks or settings.
幅広い経験を活かして、まったく新しい仕事や環境に素早く適応する。
For example, if it knows how to boil water in various settings and grow plants in different environments, it can combine these skills to brew tea on a spaceship.
例えば、様々な環境でお湯を沸かす方法や、異なる環境で植物を育てる方法を知っていれば、これらのスキルを組み合わせて宇宙船でお茶を淹れることができる。
This capability to perform tasks it hasn't encountered before, known as zero-shot performance, sets CLIN apart as a highly advanced language model.
ゼロ・ショット・パフォーマンスと呼ばれる、これまでに遭遇したことのないタスクを実行するこの能力は、CLINを高度な言語モデルとして際立たせている。
CLIN stands out among language agents, especially when compared to models like Reflection, which also operates in Science World.
CLINは言語エージェントの中でも際立っており、特に同じScience Worldで動作するReflectionのようなモデルと比較した場合、際立っている。
Reflection is actually impressive because it can analyze feedback and keep its reflective text in an episodic memory buffer.
Reflectionは、フィードバックを分析し、その反射テキストをエピソードメモリバッファに保持することができるので、実際に印象的です。
But Reflection's adaptability is limited to specific environments and it struggles with different tasks.
しかし、リフレクションの適応性は特定の環境に限られており、さまざまなタスクに苦戦している。
Meanwhile, CLIN surpasses not only Reflection but also models relying on reinforcement or imitation learning.
一方、CLINはリフレクションだけでなく、強化学習や模倣学習に頼ったモデルも凌駕している。
These models often need lots of training data and detailed adjustments.
これらのモデルは、多くの訓練データと詳細な調整を必要とすることが多い。
But CLIN does well without any of these updates or tweaks, making it both efficient and adaptable.
しかしCLINは、こうした更新や調整を一切必要とせず、効率性と適応性を両立させている。
CLIN shines in several key areas.
CLINはいくつかの重要な分野で優れている。
For instance, it excels in handling complex, multi-step, long tasks, achieving about 85% accuracy.
たとえば、複雑で多段階の長いタスクの処理に優れており、約 85% の精度を達成しています。
This is much better than Reflection's 62%, indicating CLIN's capability to manage more demanding tasks.
これは Reflection の 62% を大きく上回り、CLIN がより負荷の高いタスクを処理できることを示しています。
In adapting to varied environments, CLIN also performs strongly, maintaining around 79% accuracy, a significant improvement over Reflection's 54%. This flexibility means it can apply its skills in new and different settings more effectively.
さまざまな環境への適応においても CLIN は高い能力を発揮し、約 79% の精度を維持し、Reflection の 54% を大幅に上回りました。この柔軟性は、新しく異なる環境でも、より効果的にスキルを適用できることを意味する。
Moreover, when it comes to tackling new and unique tasks, CLIN's ability to learn from experience is evident.
さらに、新しくユニークなタスクに取り組む場合、CLINの経験から学ぶ能力は明らかです。
It has a success rate of 73% in these scenarios, outperforming Reflection, which scores 46%. This adaptability highlights CLIN's advanced learning and application capabilities compared to other models.
このようなシナリオでの成功率は73%で、46%のリフレクションを上回っている。この適応力は、他のモデルと比較してCLINの高度な学習能力と応用能力を際立たせています。
CLIN is achieving remarkable results, and its success largely hinges on its innovative use of memory.
CLINは目覚ましい成果を上げているが、その成功はメモリの革新的な使い方によるところが大きい。
It's fascinating to see how it learns, and here's an insight into how it operates.
CLINがどのように学習しているのかを見るのは興味深い。
CLIN operates with two distinct memory systems: Global and local memory.
CLINは2つの異なる記憶システムを使っている: グローバル記憶とローカル記憶だ。
Global memory is more long-term and dynamic.
グローバル・メモリーはより長期的で動的なものだ。
It holds what we call causal abstractions from past experiences.
過去の経験からの因果的抽象化と呼ばれるものを保持している。
These are basically explanations of how certain actions lead to particular results.
これは基本的に、ある行動がどのようにして特定の結果につながるかを説明するものである。
For instance, understanding that boiling water needs heat or that plants grow with water and sunlight are types of causal abstractions.
例えば、水を沸騰させるには熱が必要であるとか、植物は水と日光で育つといったことは、因果関係の抽象化の一種である。
This Global memory is continuously updated with new relevant information from each experience.
このグローバル記憶は、それぞれの経験から得られる新しい関連情報で絶えず更新される。
In contrast, local memory is more short-term and focused on the specific task at hand.
対照的に、ローカル記憶はより短期的で、目の前の特定のタスクに集中する。
It records feedback from the current activity, giving CLIN insights into how it's performing or what's happening in its immediate environment.
現在の活動からのフィードバックが記録され、CLINがどのように活動しているか、あるいは目の前の環境で何が起こっているかについての洞察を与える。
If CLIN is trying to boil water, feedback like the water is boiling gets stored here.
CLINがお湯を沸かそうとしている場合、お湯が沸いたというようなフィードバックがここに保存される。
Or if it's caring for a plant, it notes if the plant is wilting.
あるいは、植物の世話をしているのであれば、植物が枯れていないかどうかを記録する。
This local memory is reset and updated with fresh feedback for every new task.
このローカル・メモリーはリセットされ、新しいタスクのたびに新鮮なフィードバックで更新される。
Now, how does CLIN use these memories?
さて、CLINはこれらの記憶をどのように使うのだろうか?
Before tackling a task, it consults its Global memory to pull out helpful causal abstractions.
タスクに取り組む前に、CLINはグローバル・メモリーを参照し、役に立つ因果関係を抽象化する。
Say CLIN is faced with boiling water in a desert.
例えば、CLINが砂漠で水を沸騰させることに直面したとする。
It recalls from Global memory that heat is needed for boiling water.
CLINはグローバル記憶から、水を沸騰させるには熱が必要であることを思い出す。
This helps it strategize, like figuring out how to generate heat in that setting.
これは、その環境で熱を発生させる方法を考えるなど、戦略を立てるのに役立つ。
If the task is about growing a plant near a volcano, it remembers from Global memory that plants need water and sunlight, guiding its actions to perhaps find water or shield the plant from extreme conditions.
火山の近くで植物を育てるという課題であれば、植物には水と日光が必要であることをグローバル記憶から思い出し、水を探したり、植物を極限状態から遮ったりする行動を導く。
During the task, CLIN also checks its local memory.
タスクの間、CLINはローカルメモリーもチェックする。
This helps it adjust its actions based on real-time feedback.
これは、リアルタイムのフィードバックに基づいて行動を調整するのに役立つ。
If it's boiling water and notices from the local memory that the water is already boiling, it knows to stop heating.
お湯を沸かしているときに、ローカルメモリーからお湯がすでに沸騰していることに気づけば、沸騰を止めることができる。
Or if it's managing a plant and finds from the feedback that the plant is wilting, it might change its approach, like moving the plant to a cooler spot.
あるいは、植物を管理しているときに、フィードバックから植物が枯れていることに気づけば、植物を涼しい場所に移動させるなど、アプローチを変えるかもしれない。
This dual memory system is what makes CLIN stand out.
この二重の記憶システムが、CLINを際立たせている。
It doesn't just learn from abstract concepts but also incorporates immediate practical feedback.
抽象的な概念から学ぶだけでなく、即座に実践的なフィードバックも取り入れる。
This approach enables it to adapt its learning to a variety of tasks and environments, embodying the essence of a continual learning language agent.
このアプローチにより、様々なタスクや環境に学習を適応させることができ、継続的に学習する言語エージェントの本質を体現している。
Now, understanding the way CLIN learns compared to how we humans learn shows us both the differences and what's similar between the two.
さて、CLINの学習方法を私たち人間の学習方法と比較して理解することで、両者の違いと共通点の両方が見えてくる。
Humans and CLIN both learn from their experiences, but we humans also mix in our emotions, thoughts, and social interactions, which adds depth to our learning.
人間もCLINも経験から学ぶが、私たち人間は感情や思考、社会的相互作用も織り交ぜることで、学習に深みを与えている。
Our learning is shaped by many factors, which makes it rich and detailed, but it can also be a bit unpredictable at times.
私たちの学習は多くの要因によって形成されるため、豊かで詳細なものとなるが、時には予測不可能なこともある。
CLIN, however, learns through set algorithms.
しかしCLINは、決められたアルゴリズムで学習する。
It focuses on being efficient and can handle a lot of information, but it doesn't have any emotional understanding.
効率的であることに重点を置き、多くの情報を扱うことができるが、感情的な理解はない。
This means while CLIN's learning is very precise, it can't really understand feelings or make ethical choices like humans can.
つまり、CLINの学習は非常に正確だが、人間のように感情を理解したり、倫理的な選択をすることはできない。
Another key difference is that humans can change the way they learn depending on what works best for them in different situations.
もう一つの大きな違いは、人間は異なる状況で最も効果的な学び方に合わせて学び方を変えることができることです。
This kind of adaptability isn't something you find in CLIN's algorithm-based learning process or in any AI learning process.
このような適応性は、CLINのアルゴリズムベースの学習プロセスや、どのAIの学習プロセスにも見られないものだ。
Realizing these differences helps us appreciate what CLIN can do with data and information, but it also shows us the special value of human intelligence, particularly when it comes to understanding emotions and making moral decisions.
こうした違いを理解することは、CLINがデータや情報を使ってできることを評価するのに役立つが、同時に人間の知性、特に感情を理解し道徳的な判断を下すことに関して特別な価値があることを教えてくれる。
This comparison underlines that human learning and AI learning can complement each other, as both have their own strengths and weaknesses.
この比較は、人間の学習とAIの学習が、それぞれの長所と短所を持ちながら、互いに補い合うことができることを強調している。
I hope this sheds light on how CLIN's memory systems function.
CLINの記憶システムがどのように機能するのか、その一端が明らかになれば幸いである。
If you have questions or thoughts, feel free to drop them in the comments.
もし質問や感想があれば、遠慮なくコメント欄に書き込んでほしい。
And for those curious to delve deeper into CLIN, the original research paper is available in the video description.
また、CLINについてもっと詳しく知りたい方のために、オリジナルの研究論文をビデオの説明文に掲載しています。
Thanks for tuning in, and see you in the next one.
お聞きいただきありがとうございます。次回もお会いしましょう。
この記事が気に入ったらサポートをしてみませんか?