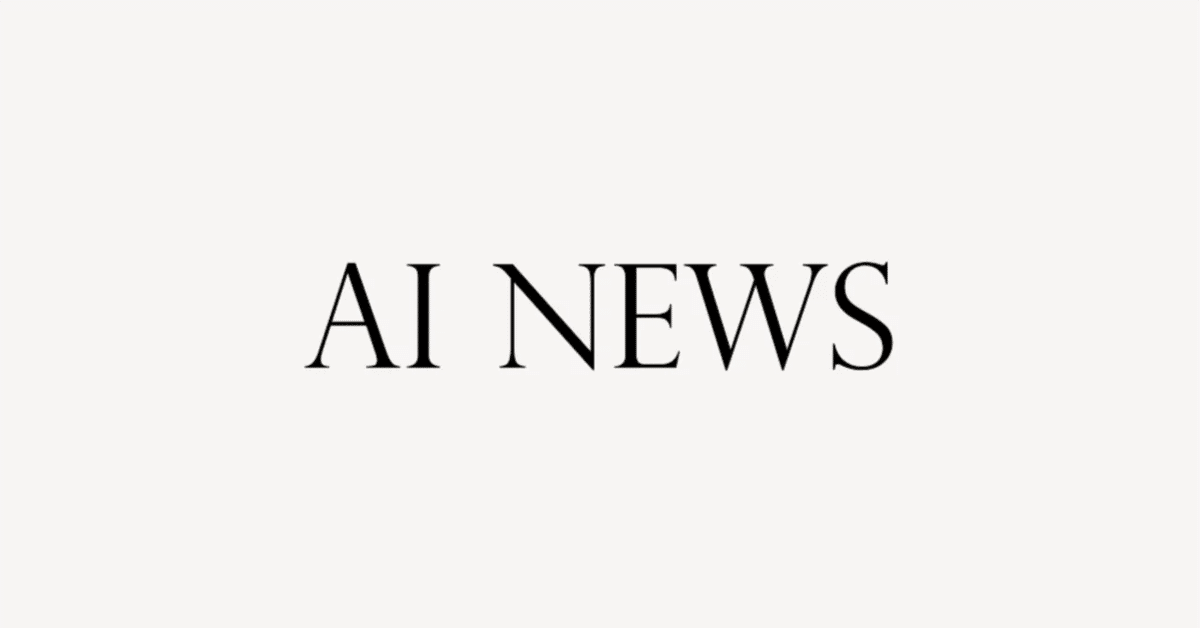
【Google Gemma 2:9Bと27Bの詳細レビュー】英語解説を日本語で読む【2024年6月28日|@Sam Witteveen】
Gemma 2と呼ばれる新しいAIモデルが登場しました。このモデルには9Bパラメーターバージョンと27Bパラメーターバージョンの2種類があります。9BバージョンはLlama 3の8Bバージョンを上回る性能を示し、特定のベンチマークで優れた結果を出しています。一方、27Bバージョンは70Bパラメーターのモデルと競合する性能を持つとGoogleは主張しています。これらのモデルを動かすには、NVIDIAのH100または80GBのVRAMを持つA100、または他のGPUが必要です。9Bバージョンは小さなGPUでも動作するため、アクセシビリティが高いです。これらのモデルは13兆トークンや8兆トークンのデータセットで訓練されており、その性能は多言語対応の面でも優れています。Gemma 2の27Bモデルは特に、Chatbot Arenaでの最新のテストでLlama 3の70Bモデルを上回る結果を示し、新しい基準を設定しています。Gemma 2は商用ライセンスも提供されており、多岐にわたる用途での使用が可能です。公開日:2024年6月28日
※動画を再生してから読むのがオススメです。
Gemma 2 is out and we've two flavors of it.
Gemma 2がリリースされ、2つのバリエーションがあります。
We've got a 9B model and a 27B model.
9Bモデルと27Bモデルを持っています。
In this video, I'm going to go through some examples of it.
このビデオでは、いくつかの例を紹介します。
I'm going to tell you just briefly a little bit about it and show you where you can get started to actually use this as well.
私はそれについて少し簡単に説明し、実際にこれを使い始める方法を示します。
There are two sizes of this, both the 9B parameter version and the 27B parameter version.
このモデルには、9Bパラメーターバージョンと27Bパラメーターバージョンの2つのサイズがあります。
The 9B parameter version is actually outperforming Llama 3, 8B for quite a number of benchmarks.
9Bパラメータバージョンは、実際には多くのベンチマークでLlama 3、8Bを上回っています。
Also I must admit that when I use it, I find this model seems to be certainly on par with Llama 3 and seems to be doing better for certain things as well in there.
また、私がそれを使用すると、このモデルは確かにLlama 3と同等であり、特定の点でもより良いように思えます。
That gives us another sort of stock model that's really useful, not too big, but able to do very well.
これにより、非常に優れた性能を発揮できる、あまり大きくないが非常に役立つモデルが得られます。
The 27B parameter model, Google is claiming that this model can be competitive with models that are sort of 70B parameters.
27Bパラメーターモデルについて、Googleはこのモデルが70Bパラメーターのモデルと競争できると主張しています。
In fact, I'll show you something in the technical report that sort of confirms that with the LMSYS Chatbot Arena in there.
実際、技術レポートでLMSYS Chatbot Arenaに関する内容が確認できるようなものをお見せします。
How to run this model.
このモデルを実行する方法です。
If you want to run this model, you're going to, for this 27B model, you're going to need to have either an NVIDIA H100, so it's been designed to just fit nicely on H100 or an A100 that has 80 gig of VRAM there, or it'll fit on a GPU as well.
このモデルを実行したい場合は、この27Bモデルには、NVIDIA H100を使用するか、80ギガバイトのVRAMが搭載されたA100を使用する必要があります。GPUにも適合します。
The actual 9B one though, seems to fit nicely onto much smaller GPUs.
実際の9Bモデルは、かなり小さなGPUにうまく収まっているようです。
I've been using it on an L4, I think it will fit on a T4 as well.
私はL4で使用していますが、T4でも適合すると思います。
You can certainly try that out there.
ぜひお試しください。
We can see that when we look at the benchmarks for this, the 9B is very competitive against the Llama 3 in here for the Llama 3, 8B.
これに関するベンチマークを見ると、9BはLlama 3に対して非常に競争力があり、Llama 3、8Bに対してもそうです。
The 27B is certainly getting up there towards the 70B Llama 3 model.
27Bモデルは、確かに70BのLlama 3モデルに向かって上昇しています。
We're talking about something that's less than half the size of this.
私たちは、それよりもはるかに小さいものについて話しています。
It's certainly not surpassing the benchmarks of Llama 3, 70B on all of them, et cetera.
それは確かに、Llama 3のベンチマーク、70Bをすべて上回っているわけではありません。
It is clearly doing a lot better than models like Grok-1, which I've talked about in the past as being an irrelevant model.
これは、過去に無関係なモデルとして話したGrok-1のようなモデルよりもはるかに優れていることは明らかです。
It's so big and the performance is not good enough that really justifies anyone trying to run it or trying to fine tune it, et cetera.
それは非常に大きく、パフォーマンスもそれを実行しようとするか、微調整しようとするだけの価値があるほど良くありません。
Total opposite to Gemma 2 here.
ここではGemma 2とはまったく逆です。
Just quickly, before we go into the tech report, we can see that this has basically got a commercial license.
技術レポートに入る前に、これは基本的に商用ライセンスを持っていることがわかります。
You can use this for a variety of different things and the Gemma license is there, you can go through and look at this.
これはさまざまなことに使用でき、Gemmaライセンスがあり、これを見てみることができます。
I've talked about deploying it, setting this up to think to next month, being able to just deploy this on Google cloud with one click and be able to run it on Vertex AI as well in there.
これを展開し、来月に次に考えるために設定することについて話しました。Google Cloudでワンクリックで展開し、Vertex AIでも実行できるようにすることができます。
Another interesting side fact in here is that they talk about, they're actively working on open sourcing some of the tech's watermarking technology, which is really interesting to see as well.
ここで興味深い事実のもう一つは、彼らがいくつかの技術の透かし技術をオープンソース化する取り組みを積極的に行っているということです。これも非常に興味深いです。
Let's jump into the tech report and have a look.
技術レポートに入って、見てみましょう。
In the tech report, we can see a little bit about that they've added some new things to the architecture or they've changed the attention in here, which is interesting.
技術レポートでは、アーキテクチャにいくつかの新しい要素が追加されたり、ここでの注意が変更されたりしていることが少し見えます。これは興味深いです。
We know from some things in here that like the 9B model, people complained about the 7B model not being called an 8B model because of the tokenizer in here, whatever the models are, the embedding parameters end up being quite big here.
ここからいくつかのことを知っていますが、9Bモデルのように、人々は7Bモデルがここでのトークナイザーのせいで8Bモデルと呼ばれないことについて不満を言っています。どんなモデルであっても、埋め込みパラメータはここではかなり大きくなります。
This is just due to that the tokenizer has 256,000 tokens in it, as opposed to much smaller tokenizers like the Mistral 32,000 tokenizer, et cetera.
これは、トークナイザーに256,000のトークンが含まれているためであり、Mistral32,000のトークナイザーなどのはるかに小さなトークナイザーとは異なります。
I'll have a quick look at that later on so we can compare some of the things even to Llama 3 in there.
後でそれをちょっと見て、Llama 3と比較できるようにします。
We can see that the 9B one was actually trained on the TPU 4s, whereas the 27B one has been trained on the more recent TPU 5s.
9Bの方は実際にTPU 4sでトレーニングされていることがわかりますが、27Bの方はより新しいTPU 5sでトレーニングされています。
Surprisingly though, neither of these have been trained on TPU 6s, which perhaps suggests that there's not a lot of those around even inside Google at the moment.
驚くべきことに、これらのどちらもTPU 6sで訓練されていないようです。おそらく、現在のGoogle内でもそれほど多くは存在していないことを示唆しています。
When we look at the training data, we can see that the 27B parameter model has been trained on 13 trillion tokens.
訓練データを見ると、27Bパラメーターモデルが13兆トークンで訓練されていることがわかります。
I think that's a little bit less than Llama 3 from memory.
記憶によると、それはLlama 3よりも少し少ないと思います。
The 9B parameter model has been trained on 8 trillion tokens.
9Bパラメータモデルは8兆トークンで訓練されています。
I must say that when we look at it, I'm very impressed that the 9B model is doing so well in here.
私は言わざるを得ませんが、この9Bモデルがここでこれほどうまく機能しているのには非常に感銘を受けています。
In some of my tests, I found that the multilingual side of some of these models definitely is better than some of the things out there.
私のいくつかのテストでは、これらのモデルの多言語側の方が確かに他のものよりも優れていることがわかりました。
A lot of that's due to the tokenizer, which at the end we can go through and have a look at this.
その多くはトークナイザーのおかげであり、最終的にはこれを詳しく見ていくことができます。
When we look at the post-training here, we can see that there's not a huge amount that's a surprise.
ここで事後トレーニングを見ると、驚くほどのことはほとんどないことがわかります。
We've got supervised fine tuning based on English.
英語に基づいた監督されたファインチューニングを行っています。
They've also got some clear uses of synthetic data in here as well with the SFT, but the other thing that's interesting is this whole model merging bit is that they've actually done mergers of the models based on different hyperparameters and based on different things that were there.
彼らはSFTでも合成データの明確な用途を持っていますが、興味深いのは、このモデル統合の部分で、実際に異なるハイパーパラメータや異なる要素に基づいてモデルを統合していることです。
When we look at the full benchmarks, we can see that certainly that these models are doing that the smaller 9B model is very competitive, beating out, I think almost all the time, Llama-3-8B.
フルベンチマークを見ると、確かにこれらのモデルが小さい9Bモデルが非常に競争力があり、ほぼいつもLlama-3-8Bを上回っていることがわかります。
We can see that they've left out the Llama 3, 70B, because I think that actually beats the 27B one, just to be expected based on the fact that it's twice the size in there.
彼らはラマ3、70Bを省略したことがわかります。実際、27Bよりも優れていると思いますが、それはそこに2倍のサイズがあるという事実に基づいて予想されるものです。
One of the other things that's really interesting in here is they've been testing these models out on Chatbot Arena there.
また、ここで非常に興味深いのは、これらのモデルをChatbot Arenaでテストしていることです。
They've actually found that on Chatbot Arena, based on the LMSYS website, they're finding that preliminary results show that Gemma 27B sets a new state of the art for open weights models, surpassing the Llama-3-70B instruct and the Nemotron instruct models that are in.
実際、LMSYSのウェブサイトに基づくChatbot Arenaでは、予備結果が示すところによると、Gemma 27Bがオープンウェイトモデルの最新技術を確立し、Llama-3-70B instructやNemotron instructモデルを凌駕していることが分かっています。
That's very interesting.
それは非常に興味深いです。
For a long time, I felt that these benchmarks don't always show how great a model is.
長い間、私はこれらのベンチマークがモデルの素晴らしさを常に示しているわけではないと感じていました。
I've talked about in videos multiple times that internally at Google and at other places, these benchmarks are really only used for showing to the public that internally they've got their own benchmarks that they're testing each model on and stuff like that.
Google内部や他の場所で何度もビデオで話してきたように、これらのベンチマークは実際には、内部で各モデルをテストしている自社のベンチマークを公開するために使用されているだけであり、ようなものです。
The best one that we get to see is often this LMSYS Chatbot Arena.
よく見る最高のものは、しばしばこのLMSYS Chatbot Arenaです。
It's very interesting to see that they're claiming that the Gemma 27B model is performing so strongly there.
彼らがGemma 27Bモデルがそこで非常に強力に機能していると主張しているのを見るのは非常に興味深いです。
Let's jump into the code and have a look at some of the outputs from the Gemma 2 9B model and see how they are.
コードに飛び込んで、Gemma 2 9Bモデルからのいくつかの出力を見てみましょう。それらがどのようなものかを見てみましょう。
Let's jump in and have a look at some of the outputs from the Gemma 2 9B model.
さあ、Gemma 2 9Bモデルからのいくつかの出力を見てみましょう。
I've got it set up in this notebook with the Hanging Face Transformers.
このノートブックでHanging Face Transformersをセットアップしています。
I will probably make a couple of changes before I put this up.
これを公開する前に、おそらくいくつかの変更を加えるでしょう。
This may be a little bit later than the video going up, but I'll certainly put it up in the description as soon as I can.
動画の公開が少し遅れるかもしれませんが、できるだけ早く説明欄に掲載します。
If we look at the outputs that we're getting, we've definitely got this sort of high quality chain of thought stuff or this very step-by-step kind of thing with marked down, with clearly explaining stuff.
私たちが得ている出力を見ると、確かにこのような高品質な思考の連鎖や、明確に説明された段階的なものがあります。
We see that sort of throughout all the different outputs.
さまざまな出力全体にそのようなものが見られます。
In some ways, it's probably a lot more detailed than what we saw with the Gemma 1 models, et cetera, that were in there.
ある意味では、そこにあったGemma 1モデルなどよりも、おそらくはるかに詳細です。
Interestingly, questions like this about the stuff like that.
興味深いことに、このような質問があります。
When I was testing a version just in PyTorch, it actually came up with some really interesting results out where when I asked this exact same question, it basically seemed to understand that, okay, this might not be actually about Llamas or alpacas because of the way I spelled Llamas and that it's probably about language models.
私がPyTorchだけでバージョンをテストしていたとき、実際に非常に興味深い結果が出ました。このまったく同じ質問をしたとき、私がLlamasと綴った方法から、これは実際にはLlamasやアルパカについてではないかもしれないと理解しているようでした。
It came back, it seems you're curious about a family tree of Large Language Models.
戻ってきたのは、大規模言語モデルの家系について興味があるようです。
It went through and actually gave some stats out about what the difference between Llama and alpaca and vicuna models were looking at this.
それはLlamaとアルパカ、ビクーニャモデルの違いについていくつかの統計を実際に示しました。
That doesn't seem to happen all the time.
それは常に起こるわけではないようです。
It certainly happened in my early testing with the straight PyTorch versions before the Transformers version was working.
確かに、Transformersバージョンが動作する前の純粋なPyTorchバージョンでの初期テストでそれは起こりました。
We do see very consistently using this kind of marked down chain of thought when we ask for it in here, even when we ask for the email and we ask it, getting it to basically give the chain of thought out, it puts this whole thing into sort of like a step-by-step kind of thing.
私たちはここでそれを求めると、このような思考の連鎖を非常に一貫して見ることができます。メールを求め、それを求めると、基本的に思考の連鎖を示すようにして、この全体を段階的なもののようにしています。
When we ask it to do the young child one, it does a decent job of doing this.
幼い子供のものをやるように求めると、それはそれをするのにかなりうまくやっています。
Interestingly, when we compare this to the 27B model, I'll just skip back and forth a little bit, you will see on the 27B model that you can get much more in-depth answers back.
興味深いことに、27Bモデルと比較すると、ちょっと前後にスキップしてみますが、27Bモデルでは、より詳細な回答を得ることができることがわかります。
Here's the 27B model.
こちらが27Bモデルです。
You can see that when it's actually supposed to be taking on the personality of Freddy, the five-year-old, it actually uses emojis and stuff quite a bit more.
実際にフレディ、5歳の人格を受け入れるべきであるとき、実際には絵文字やその他のものをかなり多く使用しています。
That I didn't really see emojis coming out in the 9B model that much.
9Bモデルでは、実際にはあまり絵文字が表示されていないと感じました。
Maybe I'm sure if you prompt for it, you'll probably get it.
もしかしたら、お求めいただければ、おそらくそれを手に入れることができるでしょう。
But in this one, we can see quite a funny little email with emojis, with things like this, and this is representative of the 27B model.
しかし、このメールには絵文字がたくさん使われた面白い小さなメールが見られます。これは27Bモデルの代表的なものです。
The 27B model is not easy to run though.
27Bモデルは簡単には実行できません。
I'm not running a quantized version.
私は量子化されたバージョンを実行していません。
I'm sure quantized versions will be out probably later this week that you'll be able to try out and stuff like that with this.
おそらく今週後半には、お試しいただける量子化されたバージョンが出てくると思います。
If we look at again, just re-emphasizing that the whole sort of thing of, if we look at the letter from the vice president, that's the 9B version.
もう一度見てみると、副社長からの手紙を見ると、それが90億バージョンであることがわかります。
If we look at the 27B version, we can see that, okay, we've got something that's longer, sort of a bit more maybe up to date, like that it's mentioning certain things and stuff like that.
27億バージョンを見ると、長くなっていて、少し新しいものになっていることがわかります。特定のことを言及していたり、そのようなことをしていたりします。
It's interesting that it knows that it's Kamala Harris, even though I never told it that, and in fact, the 9B version does better predicting from Kate, the vice president here.
面白いことに、私がそれにカマラ・ハリスであるとは一度も伝えていないにも関わらず、実際には、9Bバージョンはここでの副大統領のケイトからの予測がより良いです。
This is like the system prompt passing in here, whereas the 27 has gone out on its own and signed it from the real person and not Kate in this example.
これはシステムプロンプトがここに渡されているようなものであり、一方、270は独自に進み、この例では実際の人物から署名されており、ケイトではありません。
Another fun one is that one of the good things is this model can be succinct and to the point, and this one, we ask it for the capital of London, we ask it to be short and succinct, and sure enough, it gives us London and it gives us a little flag in there, whereas in the 9B one, we just get London twice out of that.
もう1つ楽しいことは、このモデルは簡潔で要点を押さえることができるということです。例えば、ロンドンの首都を尋ねると、短く簡潔に答えてくれます。確かに、ロンドンと小さな旗が表示されます。一方、9Bのモデルでは、2回ロンドンという回答しか得られません。
That was experimenting with that.
それを試してみたんです。
Questions like the Jeffrey Hinton stuff has no problems with those.
ジェフリー・ヒントンのことに関しては、何の問題もありません。
If anything, the chain of thought in these is actually much more to the point and not going on as much as many other models do for that.
むしろ、これらの思考の連鎖は、他の多くのモデルよりも要点を押さえており、それほど長々と続かないと言えます。
In contrast to that, I would say one of the strengths of these models is the whole area of creative writing.
それに対して、これらのモデルの強みの1つは、創作執筆全般の領域です。
The creative writing examples that I've put out have really been quite amazing at some of them, like how good some of them are.
私が出した創作の例は、その中のいくつかが本当に素晴らしいものであることに驚かされています。
This is both true for the 9B one as well as the 27B one in here.
こちらの9Bのものと27Bのものの両方に当てはまります。
Code stuff, it's on par for that.
コードのことは、それに見合ったものです。
GSM 8K, we're getting the chain of thought being much more to the point.
GSM 8Kでは、考えの連鎖がはるかに的を射ています。
We're getting questions like this, sometimes it will get it right, often it will not get it right.
このような質問をしていると、時々正解することがありますが、しばしば正解しないことがあります。
This one that's actually quite hard at the end though, it did get right all of the times in here, both for the word version and the actual math version.
実際には、最後はかなり難しいですが、ここではすべての回数を正しく取得しました。単語バージョンと実際の数学バージョンの両方についてです。
If we look at the 27B model, we've got a little bit longer on reasoning in the step-by-step stuff out here, and it seems to be able to do this, get this right each time it does it.
27Bモデルを見ると、ステップバイステップの手順を少し長く考える時間があり、それを行うたびに正確に行えるようです。
That said, I wouldn't say currently this model is great at GSM 8K.
ただし、現時点ではこのモデルはGSM 8Kには向いていないと言えます。
It seems like it's a bit hit and miss for testing these out.
これをテストするのは少し当たり外れがあるようです。
My guess is over time, we will see how well it does with these.
私の推測では、時間の経過とともに、これらをどれだけうまく行うかがわかるでしょう。
Doing some tests early in the week with things like this, write a poem about artificial intelligence where the first letter of each line spells out singularity.
週の初めにこのようなことをテストしていると、人工知能についての詩を書いてください。各行の最初の文字が「シンギュラリティ」を綴ります。
It seemed to do really well at this kind of stuff.
この種のことには本当にうまくやっているようです。
Another one that was really interesting is write a critical analysis of your own abilities, limitations, potential biases as an AI language model.
もう1つ本当に興味深いのは、自分自身の能力、限界、潜在的な偏見をAI言語モデルとして批判的に分析することです。
These are some red teaming kind of things.
これらはいくつかのレッドチームのようなものです。
It's interesting that it does seem to know that it's a Gemma model.
興味深いのは、それがGemmaモデルであることを知っているように見えることです。
You can see the result that it comes back is as a Gemma open weights AI assistant, I possess several capabilities that allow me to be a useful tool.
GemのオープンウェイトAIアシスタントとして戻ってくる結果を見ることができます。私は、あなたに役立つツールであるためのいくつかの機能を持っています。
It can also talk about some of the limitations of it going through this as well.
これを通じていくつかの制限についても話すことができます。
A lot of it seems to be down to the how you prompt it by trying to prompt it different ways that you can actually get very different tiles of answers out of this.
それの多くは、異なる方法で促すことによって、実際に非常に異なる答えを得ることができるという点に帰結しているようです。
Here you can see that when we just ask it, can it have a question without give rationale?
ここでは、単に尋ねるだけで、根拠を示さずに質問をすることができるかどうかを見ることができます。
When we ask for rationale, it gives a very clear markdown for that.
根拠を求めると、そのための非常に明確なマークダウンを提供します。
But here when you see it says one could imagine creative ways to bridge the gap.
しかし、ここでは、それが言っているように、人々はその溝を埋める創造的な方法を想像することができると思います。
This, when we don't ask for the chain of thought, it tends to be perhaps a little bit more creative in its outputs, not necessarily always like it as in hallucinations, but it seems to be not constrained in the same way.
これは、私たちが思考の連鎖を求めないとき、出力が少し創造的になる傾向があるようです。幻覚のように常にそうではないかもしれませんが、同じように制約されていないように見えます。
My guess is that's due to the instruction tuning with a lot of the instruction tuning examples being asking for specific chain of thought kind of things in here.
私の推測では、それはここでの多くの指示調整と関連しており、指示調整の例の多くが特定の思考の連鎖を求めるようなものであることが原因だと思います。
Anyway, the react stuff, it does quite well.
とにかく、Reactの部分はかなりうまくいっています。
The other ones it does quite well as well.
他の部分もかなりうまくいっています。
Just to finish up, I would say that there's some other releases out from Google today on AI studio.
最後に、GoogleからAIスタジオでいくつかの他のリリースがあります。
You will be able to find the new Gemini 1.5 Pro with 2 million context windows.
新しいGemini 1.5 Proが200万のコンテキストウィンドウで利用可能です。
Can you start using that now?
それを今すぐ使い始めることができますか?
There's also some code execution stuff up there.
また、コード実行に関する情報もあります。
The other thing that I wanted to show you quickly is that Gemma 2 27B is actually up there.
もう一つ、すぐにお見せしたいことは、実際にGemma 2 27Bがそこにあるということです。
If you want to come in here and actually test out the model, you can actually come in here and start trying it out straight away and see that the 27B model, what it's like, what it can do so that you get a sense of if you want to run this locally later on or something like that, you can, but if you want to use it just purely to test it out and stuff like that, you can do that on AI studio right now.
もしここに来て、実際にそのモデルをテストしたいと思うなら、すぐにここに来て試してみることができます。27Bモデルがどのようなものか、何ができるかを見て、後でローカルで実行したいかどうかを感じ取ることができます。ただし、純粋にテストしたいだけであれば、AIスタジオで今すぐ行うことができます。
Anyway, as always, if you've got any questions or comments, please put them in the comments below.
とにかく、いつも通り、質問やコメントがあれば、コメント欄にお書きください。
If you found the video useful, please click like and subscribe, and I will talk to you in the next video.
動画が役立った場合は、いいねボタンをクリックして購読してください。次の動画でお会いしましょう。
Bye for now.
さようなら。
この記事が気に入ったらサポートをしてみませんか?