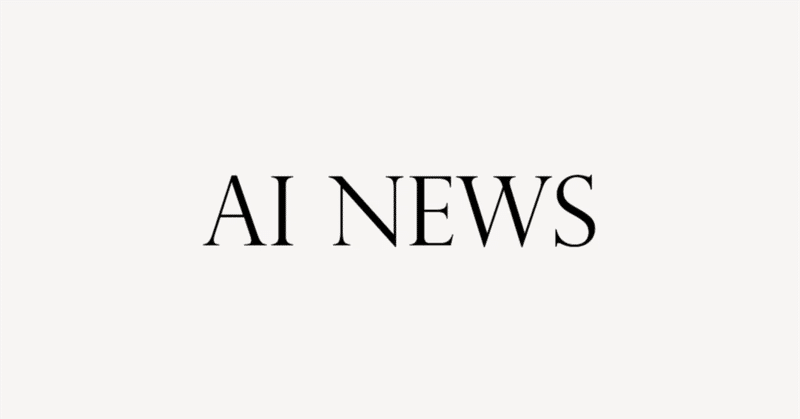
【Stable Diffusion XL Turbo: 革新的なAI画像生成技術】英語解説を日本語で読む【2023年12月24日|@Two Minute Papers】
この動画では、新しいAI技術「Stable Diffusion XL Turbo」について説明しています。この技術は、通常多くのステップを要する高品質な画像生成を、わずか数ステップで実現します。また、テキストや画像からの変換も可能です。自動運転車のトレーニングにも応用でき、NVIDIAのAIを使って実際の運転データから新しいシナリオを生成することが可能です。リアルなシミュレーションを作成し、従来の技術を上回る速さと品質で注目されています。
公開日:2023年12月24日
※動画を再生してから読むのがオススメです。
Great paper today, fellow scholars!
素晴らしい論文でした、同僚の学者の皆さん!
Stable Diffusion XL Turbo.
Stable Diffusion XL Turbo。
Why?
なぜかって?
Well, because today, we have these amazing computer games and simulations that run quickly, and we measure this in frames per second.
というのも、今日、われわれは素晴らしいコンピューターゲームやシミュレーションを高速で実行している。
Then, we have off-line simulations that run much slower, in seconds per frame.
一方、オフラインのシミュレーションは、1フレームあたり秒単位で、より低速で実行されます。
And today, we have an AI technique that we can measure in cats per second.
そして現在では、1秒間に何匹という単位で計測できるAI技術があります。
You can create so many cats per second, and it can do this too.
1秒間に何匹もの猫を作ることができるのです。
And, surprisingly it may even help us train self-driving cars we’ll look into that.
驚くことに、これは自動運転車の訓練にも役立つかもしれません。
And there’s more, you can also try this new tool right now.
さらに、この新しいツールを今すぐ試すこともできます。
And we will talk about this amazing new technique too, you can also try this for free too!
そして、この素晴らしい新しい技術についても話しましょう。それも無料で試すことができます!
How cool is that?
なんてクールなんだ
So, what was this cat thing?
さて、この猫のようなものは何でしょう?
This is Stable Diffusion XL Turbo, a supposedly quicker version of Stable Diffusion, the popular open source text to image AI.
これはStable Diffusion XL Turboで、人気のオープンソーステキスト画像変換AI、Stable Diffusionの高速バージョンと言われています。
The original version can do absolutely amazing things, but it takes a bit.
オリジナルのバージョンは本当にすごいことができるんだけど、ちょっと時間がかかるんだ。
About 20 to 60 seconds for an image.
画像で20秒から60秒くらい。
And this depends on this setting.
そして、これはこの設定に依存する。
The number of sampling steps.
サンプリングのステップ数。
We typically need 20 to 50 steps to create a high-quality image.
高品質の画像を作成するには、通常20から50のステップが必要です。
The more steps, the more computation we have to do, and thus, the longer we have to wait.
ステップ数が多ければ多いほど、より多くの計算が必要になり、その結果、待たされる時間も長くなる。
And here is an amazing new paper that promises…what?
そして、ここに驚くべき論文が発表された。
Can that really be?
本当にそんなことができるのだろうか?
1-4 sampling steps, often in a single step.
1-4サンプリングステップ、しばしばシングルステップで。
That sounds incredible.
信じられないような話だ。
I mean, if this was true, we would be able to perform text to image in… real time.
つまり、もしこれが本当なら、私たちはテキストから画像への変換を...リアルタイムで行えることになる。
Yes.
そうです。
Real time!
そう、リアルタイムだ!
But wait a second.
でもちょっと待って。
This is not new.
これは新しいことではない。
Creating an image in 1-4 sampling steps has never been a problem.
1~4サンプリングのステップで画像を作成することは決して問題ではない。
You can do it any time you want with Stable Diffusion, but, unfortunately then you get this.
Stable Diffusionを使えばいつでもできるが、残念なことにこうなる。
A blurry image.
ぼやけた画像。
No detail.
ディテールがない。
So, why is this interesting?
では、なぜこれが面白いのか?
Dear Fellow Scholars, this is Two Minute Papers with Dr. Károly Zsolnai-Fehér.
親愛なる研究者の皆さん、カーリー・ゾルナイ・フェヘール博士のTwo Minute Papersです。
Well, it is interesting because this new paper allows you to create images quickly, but also at the same time, give you high-quality images.
この新しいペーパーを使えば、素早く画像を作成することができ、同時に高品質な画像を得ることができるからです。
Now let’s have a look at the new technique.
では、新しい技術を見てみましょう。
Wow, that is as fast as you can type.
うわぁ、タイピングが速い。
The results update almost immediately.
結果はほとんどすぐに更新されます。
No more blurry images!
もうぼやけた画像はありません!
Wow.
すごい。
So, how quick is it?
で、どれくらい速いかって?
Well, hold on to your papers Fellow Scholars, because it can create an image in 9-10 milliseconds.
さあ、学者の皆さん、論文を手放さないでください。なぜなら、それは9-10ミリ秒で画像を作成することができるからです。
Yes, that is a hundred cats per second.
そう、1秒間に100匹の猫だ。
The resolution is 512x512 and the quality is not bad at all - it typically loses only against a slower version of itself, SDXL.
解像度は512x512で、画質はまったく悪くない-通常、より遅いバージョンのSDXLに対してのみ負ける。
But SDXL has been surpassed by a new text to image technique, yes, we will have a look at that too in a moment.
しかし、SDXLは新しいテキストから画像への技術によって超えられました。はい、それについてもすぐに見てみましょう。
So quality, checkmark, but following your prompts closely is also super important.
だから、品質にはチェックマークが必要だが、プロンプトに忠実に従うことも超重要だ。
And in that area, checkmark too.
その部分でもチェックマーク。
Excellent.
素晴らしい。
And there is so much more here.
そして、ここにはもっとたくさんのことがあります。
It can also perform not only text to image, but image to image translation.
テキストから画像への変換だけでなく、画像から画像への変換も可能です。
One image goes in, and it comes out transformed.
一つの画像が入り、それが変換されて出てくる。
We have seen this in Stable Diffusion before, and this helps you unleash your creativity like never before.
これは以前にもStable Diffusionで見たことがありますが、これまでにない創造性を発揮するのに役立ちます。
Remember this earlier NVIDIA paper where you could draw a landscape, and it would almost immediately give you a nearly photorealistic image?
以前NVIDIAが発表した、風景を描くとほぼ即座にフォトリアリスティックな画像が得られるという論文を覚えていますか?
Now it can do that too, and not only with landscape images, but with Apple’s memojis too.
今では、風景画像だけでなく、アップルのメモ帳でもそれができる。
I love the quick iteration speed here.
私はこの素早い反復スピードが大好きだ。
And in fact, people are already using it out there in the wild.
そして実際、人々はすでにこの方法を実際に使っている。
Let’s see how.
その方法を見てみよう。
Here you see an incredible example of real-time urban planning, prototyping and visualization.
リアルタイムの都市計画、プロトタイピング、ビジュアライゼーションの素晴らしい例をご覧ください。
And you can even create animations with it.
そして、それを使ってアニメーションさえ作成することができます。
All this for free and open source.
すべて無料でオープンソースだ。
So, how is this even possible?
では、なぜこんなことが可能なのか?
It is possible through a technique called Adversarial Diffusion Distillation.
それは、Adversarial Diffusion Distillation(逆説的拡散蒸留)と呼ばれる技術によって可能なのだ。
Luckily, we have the paper with a detailed description of the phenomenon.
幸運なことに、この現象について詳しく説明した論文がある。
So here’s how you do it: first, train a complex diffusion model.
では、やり方を説明します。まず、複雑な拡散モデルをトレーニングします。
This starts out from a noisy image, then over time, learns to reorganize this noise into an image that depicts our text prompt.
まず、複雑な拡散モデルを訓練する。これはノイズの多い画像からスタートし、時間をかけて、このノイズをテキストプロンプトを描写する画像に再編成するよう学習する。
But it does this slowly.
しかしこれはゆっくりと行われる。
Let’s call it the teacher model.
これを教師モデルと呼ぼう。
Now comes the magic!
ここからがマジックです!
We now create a smaller student model that tries to mimic its teacher.
今度は、先生を模倣しようとする小さな生徒モデルを作ります。
It learns how the teacher behaves and tries to reproduce its behavior.
教師がどのように振る舞うかを学習し、その振る舞いを再現しようとします。
But wait - we already have the teacher model, why copy it?
でも、ちょっと待ってください。すでに教師モデルを持っているのに、なぜそれをコピーするのですか?
Well, we are copying it with this student neural network, so we retain the quality, but at the same time, this student network will be much cheaper and faster.
まあ、この生徒ニューラルネットワークでそれをコピーするわけだから、質は保たれるが、同時にこの生徒ネットワークはずっと安くて速くなる。
So more corgis and cats cheaper and faster.
コーギーや猫をより安く、より早く、というわけだ。
Now, hold on to your papers Fellow Scholars, because perhaps this could also be used to train self-driving cars.
フェローの諸君、書類をしっかり持っていてくれ。自動運転車の訓練にも使えるかもしれない。
How?
どうやって?
Well, look at this cool new paper from NVIDIA, where they use real driving logs to analyze previous situations and even create new ones.
NVIDIAが発表したこのクールな論文を見てほしい。実際の運転ログを使って過去の状況を分析し、さらに新しい状況を作り出している。
Now here, all of these agents are controlled by NVIDIA’s AI, and…are you thinking what I am a thinking?
これらのエージェントはすべて、NVIDIAのAIによって制御されている。
Oh yeah, just imagine putting this into an image to image translator AI two more papers down the line, and bam, you have a simulation where you can safely train your cars in challenging situations that actually happened or may happen.
そうそう、これをもう2つ先の論文にある画像から画像への翻訳AIに組み込むことを想像してみてください。そうすれば、実際に起こった、あるいは起こるかもしれない困難な状況で、車を安全に訓練できるシミュレーションができあがります。
Imagine this in a similar manner to this earlier work where we can go from video game graphics to a real life, and back.
これは、ビデオゲームのグラフィックから実生活へ、そしてまた戻ることができるこの以前の研究と似たような方法を想像してみてほしい。
But this time with real driving situations.
しかし、今回は実際の運転状況である。
But this new paper can tokenize trajectories, meaning that it breaks down complex driving situations much like you would break down a sentence into words.
しかし、この新しい論文では、軌跡をトークン化することができます。つまり、複雑な運転状況を文を単語に分解するように分解することができます。
And then letters.
そして文字にする。
And it does it very, very well.
そして、それは非常に、非常にうまくいく
How well?
どのように?
Look.
見てください。
It is able to create more lifelike scenarios, outperforming many-many previous techniques.
よりリアルなシナリオを作成することができ、これまでの多くの技術を凌駕している。
Like a mini video game with intelligent AI players.
知能を持ったAIプレイヤーによるミニ・ビデオゲームのようだ。
What a time to be alive!
なんという時代だろう!
Now, as promised, we are going to have a look at this new text to image AI that looked at 1.1 billion images and learned to create incredibly high-quality outputs for your text prompts.
さて、約束通り、11億枚の画像を見て、あなたのテキストプロンプトのために信じられないほど高品質の出力を作成することを学んだ、この新しいテキストから画像へのAIを見てみましょう。
You can try it here, the link is available in the video description.
ここで試すことができます。リンクはビデオの説明欄にあります。
So, how good is it?
さて、その実力は?
Well, let’s test it against Stable Diffusion XL.
では、Stable Diffusion XLと比較してみましょう。
Look at that!
見てください!
Approximately 6 to 7 times out of 10, it is preferred over SDXL.
10回中6~7回は、SDXLより優先されています。
I think that is insane.
これは非常識だと思う。
Don’t forget, SDXL is a paper that came out approximately 5 months ago, and it has already been surpassed.
忘れてはならないのは、SDXLは約5ヶ月前に発表された論文であり、すでにそれを上回っているということだ。
Bravo!
ブラボー!
And while we are looking at some of these eye-poppingly beautiful images, just imagine that two more papers down the line, and I am sure that we are going to be looking at images and videos of these created in real time, and all you need to provide is just a text prompt.
そして、目を見張るような美しい画像をいくつか見ている間に、あと2つ先の論文を想像してみてほしい。私たちはきっと、リアルタイムで作成されたこれらの画像やビデオを見ているはずで、提供する必要があるのはテキストプロンプトだけなのだ。
この記事が気に入ったらサポートをしてみませんか?