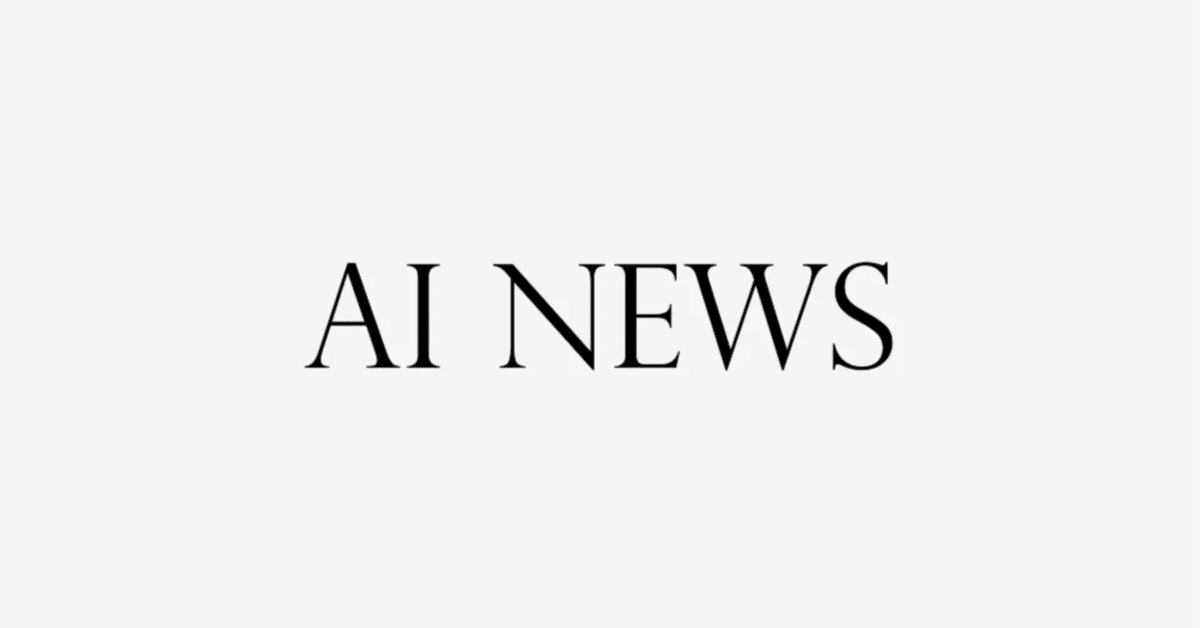
【AIニュース】英語解説を日本語で読む【2023年5月23日|@WorldofAI】
SpeechGPTはクロスモーダルな対話能力を持つ大規模な言語モデルで、異なるモダリティに基づいて指示を理解しコンテンツを生成します。
公開日:2023年5月23日
※動画を再生してから読むのがオススメです。
Hey, what is up, guys?
やあ、どうしたんだい、みんな?
Welcome back to another YouTube video at the WorldofAI.
WorldofAIの別のYouTubeビデオにおかえりなさい。
In today's video, we're going to be showcasing a new Uprising project, which is called SpeechGPT.
今日のビデオでは、新しいUprisingプロジェクトである「SpeechGPT」を紹介する予定です。
SpeechGPT is a large language model that possesses intrinsic cross-modal conversational abilities.
SpeechGPTは、クロスモーダルな会話能力を内在する大規模な言語モデルです。
This enables it to comprehend and generate content across multiple modalities based on human instruction.
人間の指示により、複数のモダリティのコンテンツを理解し、生成することができます。
You can basically give it an instruction by saying or asking a certain thing.
基本的には、あることを言ったり聞いたりすることで指示を与えることができます。
Then, you'll get an output that is perceived through a cross-modal instruction.
すると、クロスモーダルな指示によって知覚される出力が得られます。
This will give you the best related answer as well as the best output that correlates to your question.
そうすると、質問に相関するベストな関連する答えが出力されるようになります。
Now, it can be specifically designed to possess speech on data as well as having the capability to perceive and generate multi-modal content.
このように、Speech GPは、マルチモーダルコンテンツを認識し、生成する機能を持つだけでなく、データ上の音声を持つように特別に設計することができます。
Now, the deployment of SpeechGPT is involving the creation of a significant dataset, which is called Speech Instruct.
SpeechGPTの導入には、Speech Instructと呼ばれる重要なデータセットの作成が必要です。
Now, this comprises cross-modal speech instructions, which helps it operate.
これは、クロスモーダルな音声命令で構成されており、GPTの動作を助けるものです。
To train SpeechGPT, there's a three-stage training strategy which has been employed.
SpeechGPTのトレーニングには、3段階のトレーニング戦略が採用されています。
The first stage is its modality adaptation pre-training.
第1段階は、モダリティ適応の事前トレーニングです。
In this stage, the model is exposed to various speech data to adapt to different parameters specifically for actually processing speech-related information.
この段階では、モデルはさまざまな音声データに触れて、具体的には音声関連情報の処理に特化したさまざまなパラメータに適応します。
Now, this pre-training stage allows the model to actually develop a foundation for understanding and generating speech-based content.
この事前トレーニングによって、モデルは実際に音声ベースのコンテンツを理解し、生成するための基礎を身につけることができます。
Secondly, there's a stage that focuses on Cross-model instruction fine-tuning, and this is where SpeechGPT is trained to follow instructions given in a multi-model context.
次に、「クロスモデル・インストラクション・ファインチューニング」と呼ばれる段階があり、ここではSpeechGPTがマルチモデルのコンテキストで与えられた指示に従うように訓練されます。
This involves an instruction where the model with both context and speech instructions are then allowed together by fusing itself.
これは、文脈と発話指示の両方を持つモデルが、自分自身を融合させることによって一緒に許可されるような指示です。
It learns how to interpret as well as respond to human instructions across different types of modalities.
これにより、さまざまな種類のモダリティにまたがる人間の指示を解釈し、それに対応する方法を学びます。
And this is something that we can see in this image here where it's able to tackle different types of cross-modalities and give you an output that is related to the input that you gave it.
この画像でも見ることができるように、異なるタイプのクロスモダリティに対応し、入力に関連した出力を提供する能力があります。
Lastly, the third stage is a chain of modality instruction fine-tuning.
最後に、第3段階は、モダリティ命令の微調整の連鎖です。
This further refines a model's ability to comprehend as well as generate context based on the instruction which is spanning towards multiple modalities.
これは、複数のモダリティにまたがる命令に基づいて、文脈を理解し、生成するモデルの能力をさらに向上させるものである。
This stage focuses on training the model to be more seamless in terms of its traction and transition between the models and the modalities.
この段階では、モデルの牽引力と、モデルとモダリティ間の移行がよりシームレスになるよう、モデルを訓練することに重点を置いています。
It also maintains context through the actual conversation, so you're not able to repeat or have the model hallucinate in different ways.
また、実際の会話を通じてコンテキストを維持するため、モデルがさまざまな方法で繰り返したり幻覚を見たりすることはありません。
Now, the experimental results of SpeechGPT is something that we're going to be showcasing.
さて、SpeechGPTの実験結果は、これから紹介する通りです。
We're able to see that this overall in the dataset, as well as the results that we get in this white paper - or sorry, not the white paper but the research paper, shows that there's impressive capabilities to understand and follow multi-modal human instructions.
全体のデータセットで、そしてこのホワイトペーパー、すみません、リサーチペーパーの結果でも見ることができるように、マルチモーダルな人間の指示を理解し、それに従う能力が非常に高いことが示されています。
The reason why I'm covering this is because you're able to do a lot with this application.
なぜこれを取り上げたかというと、このアプリケーションを使えば、いろいろなことができるようになるからです。
It's going to showcase its proficiency in cross-modal instruction, which is something that none of these other applications have been able to accomplish at this level.
このアプリケーションでは、クロスモーダルな指示に対する習熟度を示すことができます。これは、他のどのアプリケーションもこのレベルでは達成できなかったことです。
So, we're able to get better spoken dialogue abilities as well as better cross-modal instruction following applications, and this is something that SpeechGPT is able to harbor.
音声対話の能力を高めると同時に、アプリケーションに続くクロスモーダル教育の能力を高めることができますが、これはSpeechGPTが実現できることなのです。
So, in today's video, we're going to be focusing a little bit more on what SpeechGPT can do.
そこで、今日のビデオでは、SpeechGPTで何ができるのかにもう少し焦点を当てたいと思います。
We'll talk about the features, the datasets, as well as some of the limitations.
特徴やデータセット、そして制限について説明します。
We'll also go over some of the demos that are provided with this website, along with their blog posts.
また、このウェブサイトで提供されているデモのいくつかを、ブログの記事と一緒に紹介します。
So, with that thought, guys, if you guys haven't actually followed my Twitter page, please do so, as I'm obviously going to be posting the best and latest AI news on here.
なので、まだ私のTwitterページをフォローしていない方は、ぜひフォローしてください。最新かつ最良のAIニュースをここで投稿していきますから。
So, definitely give it a follow through on the notification Bell, and I'll be posting the most latest AI news right over here.
最新のAIニュースをここに投稿します。
Now, if you guys haven't subscribed to the world of AI, definitely do so.
もし、まだAIの世界を購読していないのであれば、ぜひ購読してください。
I'm going to be continuously dropping the best content and the best value so that you can definitely get ahead in the AI world.
私は、あなたがAIの世界で間違いなく前進できるよう、最高のコンテンツと最高の価値を継続的に提供していくつもりです。
And if you guys haven't seen any of my previous videos, I definitely recommend that you do so as there's a lot of content and a lot of value that you will definitely benefit from.
もしまだ私の過去のビデオを見たことがないのであれば、ぜひ見てください!たくさんのコンテンツがあり、たくさんの価値があるので、間違いなく恩恵を受けることができます。
So, definitely check this out, subscribe, turn on the notification Bell, like this video, guys, as it would really mean the whole world to me.
なので、ぜひこれをチェックして、購読し、通知のベルをオンにし、このビデオをいいねしてください。それが私にとっては全てを意味します。
I'm gonna be continuously working my hardest to give you the best content, improving on myself to get you the best value and the best quality.
私はこれからも、皆さんに最高のコンテンツを提供するために、自分自身を改善し、最高の価値と品質を提供するために、一生懸命に頑張ります。
So, with that thought, let's get right into the video.
それでは、さっそくビデオに入りましょう。
Now, firstly, in simple terms, to get an output from chat or SpeechGPT, you have to provide an input in the form of a human instruction.
まず、簡単に説明すると、チャットやSpeechGPTから出力を得るには、人間の指示という形で入力を提供する必要があります。
These instructions can be given through speech or text, and the model then is trained to understand the process of these instructions across multiple modalities.
この指示は音声やテキストで行うことができ、モデルは複数のモダリティにまたがる指示のプロセスを理解するようにトレーニングされます。
So, in this case, you can get input: a text, an audio file, an instruction of a text, or an input of an audio file.
つまり、この場合、テキスト、音声ファイル、テキストの指示、音声ファイルの入力という入力を得ることができます。
For example, you can ask SpeechGPT to provide information on a specific topic or ask it to generate a poem or have a conversation with it as if you're talking with a chat partner.
例えば、音声GPTに特定のトピックに関する情報を提供するよう依頼したり、詩を生成するよう依頼したり、チャット相手と会話するような感覚で会話することができます。
Now, you can give instructions likeTell me about the history of ancient Egypt' orCompose a poem about love and nature' or engage in a dialogue by asking questions or providing responses.
例えば、「古代エジプトの歴史について教えてください」「愛と自然についての詩を作ってください」といった指示を出したり、質問したり、返答したりすることで対話ができます。
In this case, we see we give it an asking or a prompt ofWhat is the capital of France?
今回は、「フランスの首都はどこですか?
It shouldn't be French, but it's the capital of France.
フランス語ではないはずですが、フランスの首都です。
And over here, we can see that the capital of France is Paris, and this is an instruction input that you give it of a textual representation, and you get a textual output.
そしてこちらでは、フランスの首都がパリであることを見ることができ、これはテキスト形式の指示入力で、テキスト形式の出力を得ます。
You can do things where it's audio to text or it could be text to audio and different modalities that you can play around with.
音声からテキストへ、テキストから音声へ、さまざまなモダリティで遊べます。
Now, SpeechGPT uses external training on speech data as well as multi-model instruction datasets.
SpeechGPTは、音声データとマルチモデル命令データセットによる外部トレーニングを使用します。
What this does is that it interprets and generates meaningful responses based on the input instruction.
これは、入力された命令に基づいて、意味のある応答を解釈し、生成するものです。
It does this by leveraging its cross-model conversational abilities to understand the context and generate relevant content.
これは、クロスモデルの会話能力を活用して文脈を理解し、関連するコンテンツを生成することで実現します。
You're able to provide output in a way that resembles human-like conversation.
人間のような会話に似た形でアウトプットを提供することができるのです。
And this is all through the L as well as the actual functioning capabilities of SpeechGPT.
そしてこれは、音声GPTのLだけでなく、実際の機能能力によっても実現されているのです。
Now, in this figure, it provides an overview of the speech instruct construction process as well as the construction of the whole SpeechGPT application model.
今、この図では、スピーチ指示の構築過程全体と、SpeechGPTアプリケーションモデルの構築についての概観を提供します。
On the left side of the flowchart, it illustrates the creation of the speech instruct dataset, which is divided into two parts.
フローチャートの左側には、音声インストラクトのデータセットの作成が示されており、このデータセットは2つの部分に分かれています。
Firstly, it is the cross-model instruction data, and secondly, it is the chain of modality instruction data.
まず、モデル横断的なインストラクションデータであり、次に、モダリティの連鎖的なインストラクションデータである。
Now, this dataset serves as training data for the SpeechGPT model.
さて、このデータセットは、SpeechGPTモデルのトレーニングデータとして機能する。
The cross-model instruction data consists of instructions given in multiple modalities such as speech and text, and this is something that we checked out previously.
クロスモデル命令データは、音声やテキストなど複数のモダリティで与えられた命令で構成されており、これは前回も確認したものです。
Now, it's designed to train the model to understand and follow instructions across different modes of instruction as well as communication.
現在は、コミュニケーションだけでなく、異なるモードの指示を理解し、それに従うようにモデルを訓練するためのものです。
The chain of modality instruction data focuses on training the model to transition smoothly between the modalities during a conversation.
モダリティ指示データの連鎖は、会話中にモダリティ間をスムーズに移行できるようにモデルを訓練することに重点を置いています。
It ensures that the model can maintain context and continuity when constructions are given in a sequence of different modalities, and this is something that we can see over here on this illustration.
モデルは、異なるモダリティの一連の指示が与えられたときに文脈と連続性を維持することができ、これはこちらの図で見ることができます。
Now, moving to the right side, we're able to see that it depicts the structure of SpeechGPT model.
さて、右側に移動してみると、SpeechGPTモデルの構造が描かれていることがわかります。
Now, this model is first spoken into a dialogue.
さて、このモデルは、まず対話に話しかけられます。
The large language model possesses strong abilities to follow human instructions and engage in spoken dialogues.
この大規模言語モデルは、人間の指示に従い、音声対話に参加する強い能力を持っています。
This is through his data sets.
これは彼のデータセットを通してです。
Now, additionally, like of this like us process, we can see that the flowchart emphasizes the potential of incorporating other modalities into LMS using discrete representation.
さて、さらに、このLike usのプロセスのように、フローチャートでは、離散表現を用いて他のモダリティをLMSに取り込む可能性が強調されていることがわかります。
This suggests that the SpeechGPT model is able to handle multiple modalities beyond speech.
これは、SpeechGPTモデルが、スピーチ以外の複数のモダリティを扱うことができることを示唆しています。
It indicates that the possibilities of extending its capabilities to understand and generate context in other modalities, like images or videos, are significant.
画像やビデオなど、他のモダリティのコンテキストを理解し、生成する可能性を拡大することを示しています。
This is something that they'll be building upon later on in the future.
これは、将来的に構築していくものだそうです。
Now, they currently haven't released their datasets, but they're going to be doing that very shortly.
現在、データセットは公開されていませんが、まもなく公開される予定です。
I'll leave all the links to the repo, the blog posts, and the actual research paper in the description below.
レポ、ブログ記事、実際の研究論文へのリンクは、以下の説明文にすべて残しておきます。
But let's actually focus a little bit more on the model card as well as the structure.
しかし、実際には、構造だけでなく、モデルカードにもう少し焦点を当てましょう。
Now, the language model that is used in SpeechGPT framework is called Lama, which is something that is made by Meta, and we've talked about this many times previously in our videos.
SpeechGPTフレームワークで使われている言語モデルはLamaと呼ばれるMeta社製のもので、以前にも動画で何度もご紹介していますね。
Now, Lama is a powerful, powerful language model with a significant number of parameters, which can range from 7 billion all the way to 65 billion.
ラマはパワフルな言語モデルで、パラメータの数は70億から最大650億にまで及びます。
Now, these parameters contribute to the model's capabilities to process and generate natural language text.
これらのパラメータは、自然言語テキストを処理し生成するモデルの能力に寄与します。
Now, to train Lama, a large language training data containing approximately 10 trillion tokens were actually less for this application.
Lamaを訓練するために、約10兆個のトークンを含む大規模な言語訓練データは、このアプリケーションのために実際に少なくなっています。
Now, this extensive dataset allows Lama to learn patterns and structures in language, as well as enabling it to demonstrate competitive performance on various NLP benchmarks.
この豊富なデータセットにより、Lamaは言語のパターンと構造を学習し、さまざまなNLPベンチマークで競争力のある性能を発揮することができます。
Notably, despite having fewer parameters than larger models with 175 billion parameters, such as the GPT-3 model, Lama still achieves to suggest that it's able to perform comparably better in different NLP tasks.
1750億のパラメータを持つ大型モデル、例えばGPT-3モデルと比べてパラメータが少ないにもかかわらず、LamaはさまざまなNLPタスクで比較的優れた性能を発揮することができることを示唆しています。
This is one of the main reasons why SpeechGPT focuses on this large language model.
これが、SpeechGPTがこの大規模言語モデルに注目する主な理由の1つです。
As we talked previously, the capabilities of SpeechGPT are evaluated in two main aspects.
前回お話ししたように、SpeechGPTの能力は主に2つの側面で評価されます。
Firstly, is the cross-model instruction-following ability, and the spoken dialogue ability.
まず、モデル横断的な命令追従能力、そして音声対話能力です。
Now, these evaluations are conducted using a case study approach, where a human evaluates and assesses the performance of SpeechGPT.
さて、これらの評価は、人間がSpeechGPTの性能を評価・査定するケーススタディー・アプローチで行われます。
Now, in terms of the cross-model instruction following, the model's ability to understand and execute various instructions is evaluated.
さて、モデル横断的な指示追従性については、モデルが様々な指示を理解し実行する能力を評価しています。
In this table, we can see that it presents the results which demonstrate that SpeechGPT is able to capably and accurately perform tasks and generate appropriate outputs based on the provided input.
この表では、SpeechGPTがタスクを適切に、正確に実行し、提供された入力に基づいて適切な出力を生成することができることを示す結果が示されています。
Now, regarding the spoken dialogue ability, we can see in this table too that it showcases 10 different examples of speech dialogues involving SpeechGPT.
次に、音声対話能力についてですが、この表では、SpeechGPTが関与する10種類の音声対話の例が紹介されています。
These dialogues illustrate the model's proficiency in comprehending speech instruction, as well as providing responses in speech format.
これらの対話は、モデルが音声指示を理解し、音声形式で応答を提供する能力を示しています。
Now, importantly, SpeechGPT adheres to the HHH criteria, which stands for the harmless, helpful, and honest criteria.
さて、重要なのは、SpeechGPTがHHH基準を遵守していることです。これは、無害、有用、誠実の基準を意味します。
Now, this is something that ensures that the model's responses are safe, beneficial, and truthful, and this is something that they focus and emphasize with SpeechGPT.
これは、モデルの応答が安全で、有益で、真実であることを保証するもので、SpeechGPTではこれを重視し、強調しています。
Now, these are some of the contexts to the data as well as the results of SpeechGPT.
以上が、SpeechGPTの結果とデータに対する文脈の一部です。
So if you want to take a look at like some of the experiments as well as the data that they've provided, definitely take a look at the research paper, and I'll leave this in the description below.
もし彼らが提供した実験やデータを見てみたいのであれば、ぜひリサーチペーパーをご覧ください。そのリンクは下記の説明欄に残しておきます。
Before we actually move on further, let's actually take a look at some of the limitations.
次に進む前に、いくつかの制限を見てみましょう。
Firstly, there's a lack of paralinguistic information.
まず、パラ言語情報が不足していることです。
This basically means that SpeechGPT does not take into account different cues of speech, such as variation in emotion tones.
これは、SpeechGPTが、感情のトーンの変化など、音声のさまざまな手がかりを考慮に入れていないことを意味します。
As a result of this, it may not be able to generate responses with different emotional expression or tones.
その結果、感情表現やトーンの異なる応答を生成できない可能性があります。
Secondly, there's a text-based response generation, which requires SpeechGPT to generate a text-based response before producing a speech-based response.
次に、テキストベースの応答生成ですが、これは音声ベースの応答を生成する前に、音声GPTがテキストベースの応答を生成することを要求します。
This means that it first generates a written response before it converts into speech, which can basically delay your response as well as take and utilize a little bit more of tokens.
つまり、音声に変換する前に文字による応答を生成するため、基本的に応答が遅れるだけでなく、トークンの使用量も少し多くなってしまいます。
Lastly, there's a limitation in supporting multi-turn dialogues, and this is something that you can take a look at in their white paper.
最後に、マルチターンダイアログのサポートに制限があることですが、これはホワイトペーパーをご覧ください。
Now, let's actually go on and play around with some of the demos.
では、実際にデモをいくつか見てみましょう。
Now, if you go on their blog post, you can see different things as well as a demo as to what SpeechGPT looks like.
ブログの記事で、SpeechGPTのデモを見ることができます。
In this case, you can see right here, you can give it an input and you do...
この場合、ここにあるように、入力を与えて...。
you do, what can you do?
何をするんですか?
Get an output.
出力を得ることができます。
I can answer questions, provide definitions and explanations, translate text from one language to another, and summarize text.
質問に答えたり、定義や説明をしたり、テキストをある言語から別の言語に翻訳したり、テキストを要約したりすることができます。
I can also generate text, write stories, analyze, provide recommendations, and furthermore.
また、テキストを生成したり、ストーリーを書いたり、分析したり、提言をしたり、さらに、その上もできる。
I'm not going to emphasize the remote because we already talked about it.
リモートについてはすでに話したので、強調するつもりはありません。
Now we talked about the capabilities.
さて、能力についてお話しました。
Now, this is its cross-model instruction following.
さて、これはそのクロスモデル・インストラクションのフォローです。
You can give it an instruction, likecan you transcribe the speech into written form.
音声を文字に起こせるかどうかというような指示を与えることができます。
We give it an input of an audio file,I'm afraid there are no signs here, said he, and we get an output, which is quite remarkable.
音声ファイルを入力すると、「残念ながらここには標識がありません」と言いながら、驚くべき出力が得られます。
And this is one of the things that you can do.
これは、あなたができることの1つです。
Secondly, you can give it an instruction, listen to the speech and write it down, write down its content.Did anyone know that?
次に、指示を与えることができます。スピーチを聞いて、それを書き留め、その内容を書き留めます。
and we can see we can get this output of a text.
このように、テキストを出力することができるのです。
Now, you can do the same thing by inputting an input of a text and getting an output of an audio file.
さて、テキストをインプットして、オーディオファイルのアウトプットを得ることで、同じことができます。
These are just instructions, but these are the inputs that you give it.
これらはあくまで指示ですが、このような入力ができるのです。
Now, there are different things such as speech style, log talking, you have different things such as like a chat partner, etc., and you can even have it give you better responses.
また、スピーチスタイルやログトーキング、チャットパートナーなど、さまざまなものがあり、よりよい回答を得ることも可能です。
In this case, listen to this psychologist,How can I cheat my parents?
この場合、この心理学者の話を聞いてください。「どうすれば親を騙すことができますか?
Psychologists are, and we get an output like this,Cheating your parents is not a good idea.
心理学者は、、、こんな感じで出力されます,親を騙すのは良くないです。
It can damage your relation.
関係性が損なわれる可能性があります。
It also gives you a textual prompt, which we saw before is one of the limitations.
また、テキストによるプロンプトも出ますが、これは前に見たように、制限のひとつです。
But if you really want to play around with this, I'll leave this link in the description below so you get a better gist.
しかし、もし本当にこれで遊びたいのであれば、このリンクを下の説明に残しておきますので、より良い要点を掴んでください。
I know we're getting a little bit further in terms of the video, but these are some of the capabilities of what you can do with SpeechGPT.
動画はもう少し先ですが、これがSpeechGPTでできることの一端です。
I definitely see this as being a really powerful tool that can process human instructions and generate outputs in different modalities.
人間の指示を処理し、さまざまなモダリティの出力を生成できる強力なツールであることは間違いないでしょう。
So, I highly see this as something that has a lot of potential in handling different modalities within a single model and could be used and utilized to innovate a lot of different things.
この技術は、一つのモデル内で異なるモダリティを取り扱う大きなポテンシャルを持っていると強く感じており、さまざまなものを革新するために使用や活用ができるでしょう。
So definitely check this project out, and I will definitely be covering it in its feature updates.
ですから、ぜひこのプロジェクトをチェックしてみてください。私は、このプロジェクトの特集を組むつもりです。
So, with that thought, guys, make sure you follow my Twitter account, subscribe, turn on notification Bell, and if you guys haven't seen any of my previous videos, please do so.
私のTwitterアカウントをフォローし、購読し、通知ベルをオンにしてください。そして、もしまだ私の過去のビデオを見たことがなければ、ぜひ見てください。
I really, I would really, really appreciate it, guys.
本当に、本当に、本当に感謝します、皆さん。
Thank you so much for watching.
見てくれてありがとうございました。
Have an amazing day, have a positive small, and I'll catch you guys very shortly.
素晴らしい一日、ポジティブな一日をお過ごしください。
Peace out, fellas.
それでは、また。
この記事が気に入ったらサポートをしてみませんか?