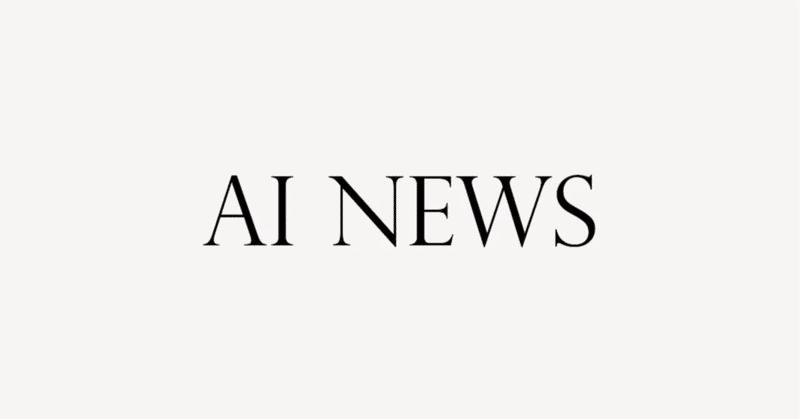
【元OpenAI社員が全てを暴露!未来のAI進化予測】英語解説を日本語で読む【2024年6月8日|@TheAIGRID】
元OpenAIの社員であるレオポルド・アッシェンブレンナーが、AI業界の未来について驚くべき予測を公開しました。彼の見解によると、AIは今後10年間で急速に進化し、2027年までには人工汎用知能(AGI)が現実のものとなる可能性が高いと述べています。アッシェンブレンナーは、「計算能力の拡大」「アルゴリズムの効率化」「ホブリングの解除」という三つの主要な要因が、AIの進化を加速させると指摘しています。特に注目すべきは、AIが自らの研究を自動化し、自己改良するフィードバックループを形成する可能性です。これにより、AIの進化速度は一層加速し、最終的には超知能に到達することが可能になります。彼が公開した文書「Situational Awareness」は、AIの未来に関する重要な情報を提供しており、特にAI研究者や技術者にとって必読の内容となっています。
公開日:2024年6月8日
※動画を再生してから読むのがオススメです。
Leopold Aschenbrenner is someone who used to work at OpenAI until he was quote unquote fired for leaking internal documents.
リオポルド・アッシェンブレナーは、OpenAIで働いていた人物であり、内部文書を漏らしたという理由で解雇されたとされています。
I do want to state that this video is arguably one of the most important videos because he details the decade ahead for how many of the companies around the world are going to get to AGI and his insights are like no other.
このビデオはおそらく最も重要なビデオの1つであり、世界中の多くの企業がAGIに到達するための10年間を詳細に説明しており、彼の洞察力は他のどのビデオとも異なります。
He made this tweet stating that virtually nobody is pricing in what's coming in AI and he made an entire document about the stages that we will need to take in order to get to AGI and some of the things that you're going to witness in the coming years.
彼は、ほとんどの人がAIに関する今後の出来事を価格に反映していないと述べるツイートをしており、AGIに到達するために必要な段階についての文書全体を作成し、今後数年間で目撃するであろういくつかの出来事についても述べています。
I think you should at least watch the first 10 minutes of this video because it is remarkably insightful into some of the things that he is predicting.
私は、彼が予測しているいくつかのことについて非常に洞察力があるため、少なくともこのビデオの最初の10分を見るべきだと思います。
There are seven different sections and I've read this thing from top to bottom at least and I'm going to give you guys the most insightful sections from this entire essay because I do believe that this is a remarkable document that I think everyone needs to pay attention to.
この文書全体を最上から最下まで読んだことがあり、このエッセイ全体から最も洞察力のあるセクションを提供します。私は、これが注目すべき文書であり、誰もが注意を払う必要があると考えています。
Without wasting any more time let's get into situational awareness the decade ahead.
さらなる時間を無駄にせずに、10年後の状況認識に入りましょう。
He has this introduction here where he talks about how the talk of the town has shifted from 10 billion compute clusters to 100 billion dollar compute clusters to even trillion dollar clusters and every six months another zero is added to the boardroom plans.
ここで彼が紹介しているのは、町の話題が10億のコンピュートクラスターから1000億ドルのコンピュートクラスター、さらには兆ドルのクラスターに移り、半年ごとに取締役会の計画にゼロが追加されているということです。
The AGI race has begun.
AGIレースは始まった。
We are building machines that can think and reason and by 2025 to 2026 these machines will outpace college graduates and by the end of the decade they will be smarter than you or I and we will have super intelligence in the true sense of the word.
私たちは考え、推論できる機械を構築しており、2025年から2026年までには、これらの機械が大学卒業生を凌駕し、そしてその10年の終わりまでには、彼らはあなたや私よりも賢くなり、我々は真の意味での超知能を持つことになります。
I'm going to say that again by the end of the decade okay we will have super intelligence in the truest sense of the word along with the way national security forces not seen in half a century will be unleashed and before long the project will be on.
もう一度言いますが、この10年の終わりまでに、我々は真の意味での超知能を持つことになります。そして、半世紀ぶりに見られなかった国家安全保障部隊が解放され、やがてプロジェクトが始まるでしょう。
These are some very fascinating predictions but just trust me once we get into some of the charts and some of the data that he's been analyzing I think it really does make sense and this is why this document is called situational awareness.
これらは非常に魅力的な予測ですが、チャートや彼が分析しているデータに入ると、本当に理にかなっていると信じてください。これがこの文書が状況認識と呼ばれる理由です。
Just read this part before we get into everything he says before long the world will wake up but right now there are perhaps a few hundred people most of them in San Francisco and the AI labs that actually have situational awareness through whatever peculiar forces or fate I have found myself amongst them and this is why this document is really important because information like this we're really lucky that people could leave a company like OpenAI and then publish a piece of information which gives us the details on how super intelligence is likely to arise and when that system is likely to arise.
すべてに入る前に、彼が言うには、世界はやがて目を覚ますでしょうが、今はおそらく数百人、ほとんどはサンフランシスコにいて、AI研究所にいる人々が、実際に状況認識を持っているということです。私はその中に自分自身を見つけた何らかの奇妙な力や運命によって、その中にいるということです。この文書が本当に重要な理由です。OpenAIのような企業を去り、その後、超知能がどのように生まれ、そのシステムがいつ生まれるかについての詳細を提供する情報のようなものに、私たちは本当に幸運だと思います。
So this is the section one from GPT-4 to AGI counting the orders of magnitudes.
これは、GPT-4からAGIへの第1セクションで、桁数を数えるものです。
When you see OOMs, that's what it stands for.
OOMsを見ると、それが何を意味するかがわかります。
He clearly states here his AGI prediction.
ここで彼は明確に自分のAGI予測を述べています。
AGI by 2027 is strikingly plausible.
2027年までにAGIは驚くほど実現可能です。
gpt2 to GPT-4 took us from preschooler to smart hall high school abilities in just four years.
GPT-2からGPT-4までの進化は、たった4年で幼稚園児から高校生のような能力を持つものになりました。
If we trace the trend lines of compute algorithmic efficiencies and unhobbling of gains, we should expect another preschooler to high school a size qualitative jump by 2027.
コンピュート、アルゴリズムの効率、利益の解放のトレンドラインを追跡すれば、2027年までにもう1つの幼稚園児から高校生のような質的な飛躍を期待すべきです。
This is where we get into our first very important chart because this shows us exactly where things may go.
ここで、私たちが行くかもしれない正確な場所を示す最初の非常に重要なチャートに入ります。
He says, I make the following claim: it is strikingly plausible that by 2027 models will be able to do the work of an AI researcher engineer that doesn't require believing in sci-fi.
彼はこう述べています。「次の主張をします。2027年までに、モデルがSFを信じる必要のないAI研究者エンジニアの仕事をできるようになる可能性が非常に高いと言えるでしょう。」
It just requires believing in straight lines on a graph.
それはグラフ上の直線を信じることだけを必要とします。
What we can see here is a graph of the base scale up of effective compute counting gpt2 all the way up to GPT-4 and looking at the effective compute that we're going to continue to scale up.
ここで見ているのは、GPT-2からGPT-4までの効果的な計算の基本スケールアップのグラフであり、今後もスケールアップを続けることを考えています。
One thing that is fascinating from here is that I think there is going to be an even steeper curve for this.
ここから魅力的なことの1つは、これにさらに急な曲線があると思うことです。
The reason I state that is because during the period from 2022 to 2023, there was something that I would like to call an awareness.
私がそう述べる理由は、2022年から2023年までの期間に、私が「認識」と呼びたいものがあったからです。
This period right here marks the birth of GPT-3 to GPT-4 and this put a real giant spectacle on the AI era.
この期間は、GPT-3からGPT-4への誕生を示しており、これによりAI時代に本当に巨大なスペクタクルが置かれました。
GPT-3 and GPT-4 weren't just research products.
GPT-3とGPT-4は単なる研究製品ではありませんでした。
GPT-3 was, but GPT-4 and ChatGPT-3.5 were actual products that were available for the public.
GPT-3はそうでしたが、GPT-4とChatGPT-3.5は実際に一般向けに利用可能な製品でした。
Since then, we've seen an explosion in terms of how many people are now intrigued by AI and how many different companies are now piling billions of dollars and billions of resources into the technology into the compute clusters just so that they can capture all of the economic value that's going to be happening during this area.
その後、AIに興味を持つ人々の数や、様々な企業が数十億ドルや数十億のリソースをこの技術に投入していることが爆発的に増えています。その理由は、この分野で起こる経済価値をすべて捉えるためです。
Which is why I do believe that it wouldn't be surprising if during the period from 2024 to 2028, we do have a lot more growth than we've had in this period.
だからこそ、2024年から2028年までの期間に、この期間よりもはるかに多くの成長があることは驚くべきことではないと私は信じています。
Which means that having an automated AI research engineer by 2027 to 2028 is not something that is far, far off.
つまり、2027年から2028年までに自動化されたAI研究エンジニアを持つことは、非常に遠くないことではないということです。
Because if we're just looking at the straight lines and the effective compute, then this is definitely where we could get to.
なぜなら、直線と効果的な計算を見ているだけなら、これは確かに私たちが到達できる場所です。
The implications of this are quite stark.
これの影響は非常に厳しいです。
Because if we can have an automated AI research engineer, that means that it wouldn't take that long to get to super intelligence after that.
なぜなら、自動化されたAI研究エンジニアを持つことができれば、その後に超知能に到達するのにそれほど時間がかからないということです。
Because if we can automate AI research, then or better off, we're able to effectively recursively self-improve, but just without that crazy loop that makes super intelligence explode.
もしAI研究を自動化できれば、効果的に再帰的に自己改善することができるかもしれませんが、超知能が爆発するような狂ったループなしで。
Here's where he states one of the things that I think is really, really important to understand.
ここで彼が本当に理解するのが重要だと思うことの1つを述べています。
I stated this in a video before this document was released, but it's glad to see that someone else is ushering one of the same concerns that I originally thought of.
私はこの文書が公開される前のビデオでこれを述べましたが、私が最初に考えた同じ懸念の1つを誰かが提起しているのを見るのは嬉しいです。
He stated that the next generation of models has been in the oven, leading some to proclaim the stagnation and that deep learning is hitting a wall.
彼は、次世代のモデルが準備中であり、深層学習が壁にぶつかって停滞していると宣言する人もいると述べました。
But by counting the ordnance of magnitude, we get a peek at what we should actually expect.
しかし、桁数を数えることで、実際に期待すべきことを垣間見ることができます。
In a video around three weeks ago, I clearly stated that look, things are slowing down externally, but things are not slowing down internally at all.
約3週間前のビデオで、外部的には物事が鈍化しているが、内部的には全く鈍化していないと明確に述べました。
Just because some of the top AI labs may not have presented their most recent research, that doesn't mean the breakthroughs aren't being made every single month.
一部のトップAI研究所が最新の研究成果を発表していないからといって、毎月革新が行われていないわけではありません。
He states here that while the inference is simple, the implication is striking.
ここで彼は、推論は単純だが、含意は驚くべきだと述べています。
Another jump like that very well could take us to AGI, to models as smart as PhDs or experts that can work beside us as a co-worker.
もう1つのジャンプがあれば、私たちをAGIに連れて行く可能性が非常に高いです。つまり、PhDや専門家と同じくらい賢いモデルが、共同作業者として私たちのそばで働くことができるかもしれません。
Perhaps most importantly, if these AI systems could automate AI research itself, that would set intense feedback loops.
おそらく最も重要なのは、これらのAIシステムがAI研究自体を自動化できれば、それは強烈なフィードバックループを設定するだろうということです。
That's, of course, where we get AI researchers to make breakthroughs in AI research, then we apply those breakthroughs to the AI systems, they become smarter.
もちろん、そこでAI研究者がAI研究での突破口を作り出し、それをAIシステムに適用することで、それらはより賢くなります。
The loop continues from there.
そのループはそこから続きます。
Basically, recursive self-improvement but on a slower scale, and here he clearly states even now barely anyone is pricing this in, but the situational awareness on AI isn't actually that hard.
基本的には、より遅いスケールでの再帰的な自己改善ですが、ここで彼は明確に述べていますが、ほとんどの人がこれを考慮に入れていないところですが、AIに関する状況認識は実際にはそれほど難しくありません。
Once you step back and look at the trends, if you keep being surprised by AI capabilities, just start counting the orders of magnitudes.
一歩引いてトレンドを見れば、AIの能力に驚かされ続けるなら、桁数を数え始めてください。
Here's where we talk about the last four years so you can see he speaks about gpt2 to GPT-4.
ここで、過去4年間について話すと、彼はGPT-2からGPT-4について話しています。
gpt2 was essentially like a preschooler, while it can string together a few plausible sentences.
GPT-2は基本的には幼稚園児のようなもので、いくつかの妥当な文をつなげることができます。
These are the gpt2 examples people found very impressive at the time, but yet it could barely count to five without getting tripped up.
これらは当時人々が非常に感銘を受けたGPT-2の例ですが、それでも、つまずかずに5まで数えることができませんでした。
We had GPT-3 which was in 2020, and this was as smart as an elementary schooler, and this was something that once again impressed people quite a lot.
2020年に登場したGPT-3は、小学生と同じくらい賢かったです。これも再び多くの人々を感銘させました。
This is where we get to GPT-4 in 2023, and this is where we get a smart high schooler.
ここで2023年のGPT-4について話しますが、これは賢い高校生です。
While it can write some pretty sophisticated code and iteratively debug it, it can write intelligently and sophisticatedly about complicated subjects.
それはかなり洗練されたコードを書くことができ、それを反復的にデバッグすることができ、複雑な主題について賢明かつ洗練された文章を書くことができます。
It can reason through difficult high school competition math, and it's beating the vast majority of high schoolers on whatever tests we give it.
それは難しい高校の競争数学を理解でき、私たちが与えるテストで高校生の大多数を打ち負かしています。
Remember, there was the sparks of AGI paper which showed some capabilities that showed us that we weren't too far away from AGI, and that this GPT-4 level system were the first initial sparks of artificial general intelligence.
AGIの火花の論文があり、それは私たちにAGIから遠くないことを示すいくつかの能力を示し、このGPT-4レベルのシステムが人工一般知能の最初の火花であることを示しました。
The thing is here, he clearly states, and I'm glad he's stating this because a lot of people don't realize this, that the limitation comes down to obvious ways that models are still hobbled.
ここで重要なのは、彼は明確に述べていますが、多くの人が気づいていないことで、制限はモデルがまだ足かせをしている明らかな方法にまで遡るということです。
Basically, he's talking about the way that models are used and the current frameworks that they have, the raw intelligence behind the model, the raw cognitive capabilities of these models if you even want to call it that, as artificially constrained.
基本的に、彼はモデルがどのように使用されているか、およびそれらが持つ現在のフレームワーク、モデルの背後にある生の知性、これらのモデルの生の認知能力(そう呼びたいとすら思えるなら)、が人工的に制約されているということについて話しています。
In the future, if you calculate the fact that these are going to be unconstrained, it's going to be very fascinating on how that raw intelligence applies across different applications.
将来、これらが制約されなくなることを計算すると、その生の知性が異なるアプリケーションにどのように適用されるかが非常に興味深いことになるでしょう。
One of the clear things I think that most people aren't realizing is that we're running out of benchmarks.
ほとんどの人が気づいていない明確なことの1つは、私たちがベンチマークを使い果たしていることです。
As an anecdote, my friends Dan and Colin made a benchmark called the MMLU a few years ago in 2020.
エピソードとして、私の友人のダンとコリンが2020年にMMLUというベンチマークを作成しました。
They hoped to finally make a benchmark that would stand the test of time equivalent to all the hardest exams we give high school and college students.
彼らは、高校や大学生に与える最も難しい試験に相当する、時代を超えて通用する基準をついに作りたいと望んでいた。
Just three years later, models like GPT-4 and Gemini get around 90%.
たった3年後に、GPT-4やGeminiなどのモデルは約90%を達成しています。
GPT-4 mostly cracks all the standard high school and college aptitude tests.
GPT-4は、主に標準的な高校や大学の適性テストをほとんどクリアしています。
You can see here the test scores of AI systems on various capabilities relative to human performance.
ここでは、AIシステムのテストスコアが、人間のパフォーマンスと比較してさまざまな能力に関して示されています。
You can see that in the recent years there have been a stark stark level of increases.
最近の数年間で、急激な増加が見られることがわかります。
Here it's absolutely crazy with as to how many different areas that AI is increasing in terms of the capabilities.
ここでは、AIが能力の面でどれだけ多くの異なる分野で進化しているかが本当に驚くべきことです。
It's really really fascinating to see and also potentially quite concerning.
見ることは本当に魅力的であり、また潜在的にはかなり懸念されることでもあります。
One of the things that most people did actually miss about going from GPT-4 to AGI was a benchmark that actually did shock me.
GPT-4からAGIに移行する際、ほとんどの人が見逃していたことの1つは、実際に私を驚かせたベンチマークがあるということです。
There is essentially this benchmark called the math benchmark, a set of difficult mathematic problems from a high school math competitions.
実質的には、高校数学競技大会からの難しい数学問題のセットである数学ベンチマークと呼ばれるものがあります。
When the benchmark was released in 2021, GPT-3 only got 5%. Basically the crazy thing about this was that researchers predicted at that time stating to have more traction on mathematical problem solving, we will likely need new algorithmic advancements from the broader research community and we're going to need fundamental new breakthroughs to solve maths or as they thought they predicted minimal progress over the coming years.
2021年にベンチマークが公開されたとき、GPT-3はわずか5%しか得られませんでした。基本的に、この狂気じみたことは、当時研究者が数学問題の解決においてより多くのトラクションを持つために、広範な研究コミュニティから新しいアルゴリズムの進歩が必要であり、数年後には数学の解決に対する基本的な新しいブレークスルーが必要になると予測していたことです。
But by mid 2022, we got to 50 accuracy and basically now with the recent math Gemini 1.5 Pro, we know that this is now at 90 which is absolutely incredible.
しかし、2022年半ばまでに、私たちは50%の精度に達し、基本的に最近の数学Gemini 1.5 Proでは、90%に達していることがわかります。これは本当に信じられないことです。
Here's something that you can clearly screenshot and share to your friends or colleagues or whatever it is whatever kind of community that you might be in.
これは、はっきりとスクリーンショットを撮って友達や同僚、またはどんなコミュニティにいるかに関係なく共有できるものです。
But you can see that the performance on the comments exams percentile compared to human test takers.
しかし、コメントの試験のパフォーマンスを人間のテスト受験者と比較すると、そのパーセンタイルがわかります。
We can see that GPT-4 ranks above 90% for pretty much all of them except calculus and chemistry which is a remarkable feat when we went from GPT-3 to GPT-4 in such a short amount of time.
GPT-4は、微積分や化学を除くほとんどすべての分野で90%以上の順位を獲得しています。GPT-3からGPT-4に移行する際に、そのような短期間でこれを達成するというのは驚くべきことです。
This is a true true jump in capabilities that many people just simply wouldn't have expected.
これは、多くの人々が単純に予想していなかった、本当の能力の飛躍です。
Here's where we start in to get to some of the predictions that we can really start to make based on the nature of deep learning.
ここで、深層学習の性質に基づいて本当に始められる予測のいくつかについて話していきます。
Essentially the magic of deep learning is that it just works and the trend lines have been astonishingly consistent despite the naysayers at every turn.
基本的に、深層学習の魔法は、それがうまく機能するということであり、否定的な意見を持つ人々がいつもいるにもかかわらず、トレンドラインは驚くほど一貫しています。
We can see here that this are screenshots from the scaling compute in the OpenAI's Sora technology and at each level we can see an increase in the quality and consistency.
ここでは、OpenAIのSoraテクノロジーにおけるスケーリングコンピュートのスクリーンショットが示されており、各レベルで品質と一貫性が向上していることがわかります。
The base compute results in a pretty terrible image/video four times compute results in something that is pretty coherent and consistent but 30 times compute is something that is remarkable in terms of the quality consistency and the level of video that we do get which shows us that these trend lines are very very consistent.
ベースのコンピュートはかなりひどい画像/ビデオを生成し、4倍のコンピュートはかなり首尾一貫したものを生成しますが、30倍のコンピュートは、品質、一貫性、および得られるビデオのレベルにおいて驚くべきものです。これは、これらのトレンドラインが非常に一貫していることを示しています。
He says if we can reliably count the orders of magnitude that we're going to be training these models we can therefore extrapolate the capability improvements and that's how some people actually saw the GPT-4 level of capabilities.
彼は言います、「私たちがこれらのモデルを訓練する際にどれだけの桁数を確実に数えることができれば、したがって能力の向上を推測することができる」と。これが、実際にはGPT-4の能力レベルを見た一部の人々が見た予測につながる方法です。
One of the things that he talks about is of course things like chain of thought tools and scaffolding and therefore we can unlock significant latent capabilities basically when we have GPT-4 or whatever the base cognitive capabilities are for this architecture.
それがもちろん、思考の連鎖ツールや足場などのことについて話していることの1つですので、GPT-4やこのアーキテクチャの基本的な認知能力がある場合、基本的には重要な潜在能力を引き出すことができます。
We can use that to unlock latent capabilities by adding different steps in front of that system.
そのシステムの前に異なるステップを追加することで、潜在能力を引き出すことができます。
For example, when you use GPT-4 with chain of thought reasoning, you significantly improve your ability to answer certain questions in different scenarios.
たとえば、GPT-4を思考の連鎖推論と一緒に使用すると、異なるシナリオで特定の質問に答える能力が大幅に向上します。
It's things like that where you can unlock more knowledge from the system by using different ways to interact with it, which means that the raw data behind the system and the raw knowledge is a lot bigger than people think.
そのようなことは、異なる方法でシステムとやり取りすることで、システムの背後にある生データや生の知識が人々が考えている以上にはるかに大きいことを解き明かすことができます。
This is what you call un-hobbling gains.
これが、あなたが「足かせを外す利益」と呼ぶものです。
One of the things that's really important, and this is something that doesn't get enough attention, but this is going to make up a lot of the gains that you won't see, is the algorithmic efficiencies.
非常に重要なことの1つは、これはあまり注目されていないことですが、これが見えない多くの利益を構成することになるのは、アルゴリズムの効率です。
Whilst massive investments into compute get all the attention, algorithmic progress is similarly an important driver of progress and is dramatically underrated.
コンピュートへの大規模な投資が注目を浴びる一方で、アルゴリズムの進歩も同様に進歩の重要な要因であり、大幅に過小評価されています。
To see just how big of a deal algorithmic progress can be, consider the following illustration.
アルゴリズムの進歩がどれほど重要かを見るために、次のイラストを考えてみてください。
This one right here: the drop of the price to attain 50 accuracy on the math benchmark over just two years.
これですよ:数学のベンチマークで50の精度を達成するための価格がわずか2年で下がったこと。
For comparison, a computer science phd student who didn't particularly like math scored 40.
比較のために、数学があまり好きでないコンピュータサイエンスの博士課程の学生は40点を取得しました。
This is already quite good, and the inference efficiency improved by nearly three orders of magnitude or a thousand x in less than two years.
これは既にかなり良いものであり、推論効率はわずか2年未満で3桁も向上しました、つまり1000倍です。
What we have here is something that is incredibly more efficient for the same result in just two years.
ここにあるものは、同じ結果をたった2年で非常に効率的にするものです。
That is absolutely incredible.
それは本当に信じられないことです。
These algorithmic efficiencies are going to drive a lot more gains than you think.
これらのアルゴリズムの効率は、思っている以上に多くの利益をもたらすでしょう。
As someone who was looking at arxiv, which is where a lot of these research papers get published, just trust me, there are like probably 50 to 80 different research papers that get published every single day.
これらの研究論文が発表されるarxivを見ていた人として、信じてください、おそらく毎日50から80の異なる研究論文が発表されています。
A few of those allow you know 10 to 20 percent gain, 30 gain.
それらのうちのいくつかは、10から20パーセントの利益、30の利益をもたらします。
If you calculate the fact that all of these algorithmic efficiencies are going to compound against each other, we're really going to see more cases like this.
これらすべてのアルゴリズムの効率が互いに複利で働くことを計算すると、このようなケースがさらに増えるでしょう。
Here's where you talk about the api cost and you basically look at how efficient it becomes to run these models.
ここでAPIコストについて話すと、これらのモデルを実行する効率がどれほど向上するかを見てみます。
GPT-4 on release cost to save at GPT-3 when it was released.
GPT-4のリリース時のコストは、GPT-3のリリース時と同じでした。
But since the GPT-4 release a year ago, the prices for GPT-4 level models have fallen six times/four times for the input/output with the release of GPT-4.
リリースから1年が経過したGPT-4以降、GPT-4レベルのモデルの価格は、GPT-4のリリースとともに6回/4回下がりました。
GPT-3.5 level is basically Gemini 1.5 Flash, and this is 85 times cheaper than what we previously used to have.
GPT-3.5レベルは基本的にGemini 1.5 Flashであり、これは以前使用していたものより85倍安くなっています。
We can see here on this graph that if we want to calculate exactly how much progress is going to be made, we can clearly see that there are two main things here.
このグラフを見ると、進捗がどれだけ進むか正確に計算したい場合、ここには2つの主要な要素があることがはっきりとわかります。
The first is the physical compute of scaling, which is going to be things like these data centers and the hardware that we throw at the problems.
最初に挙げられるのは、スケーリングの物理的な計算であり、これはデータセンターや問題に取り組むために投入するハードウェアなどのことです。
And then the algorithmic progress, which is going to be the efficiencies where people rewrite these algorithms in crazy ways that just drive efficiencies that we previously didn't know how to solve.
そして、アルゴリズムの進歩であり、人々がこれらのアルゴリズムを狂ったような方法で書き直し、以前は解決方法がわからなかった効率を生み出すことになります。
That's why in the future, where we do get an automated AI researcher to do that, this gap is going to widen even more.
だから将来、自動化されたAI研究者がそれを行うようになると、このギャップはさらに広がるでしょう。
This is where we talk about hobbling.
これは私たちがホブリングについて話すところです。
This is, of course, something that we just spoke about before, but the reason that this.
これはもちろん、以前に話したことですが、その理由はそれがそうだからです。
この記事が気に入ったらサポートをしてみませんか?