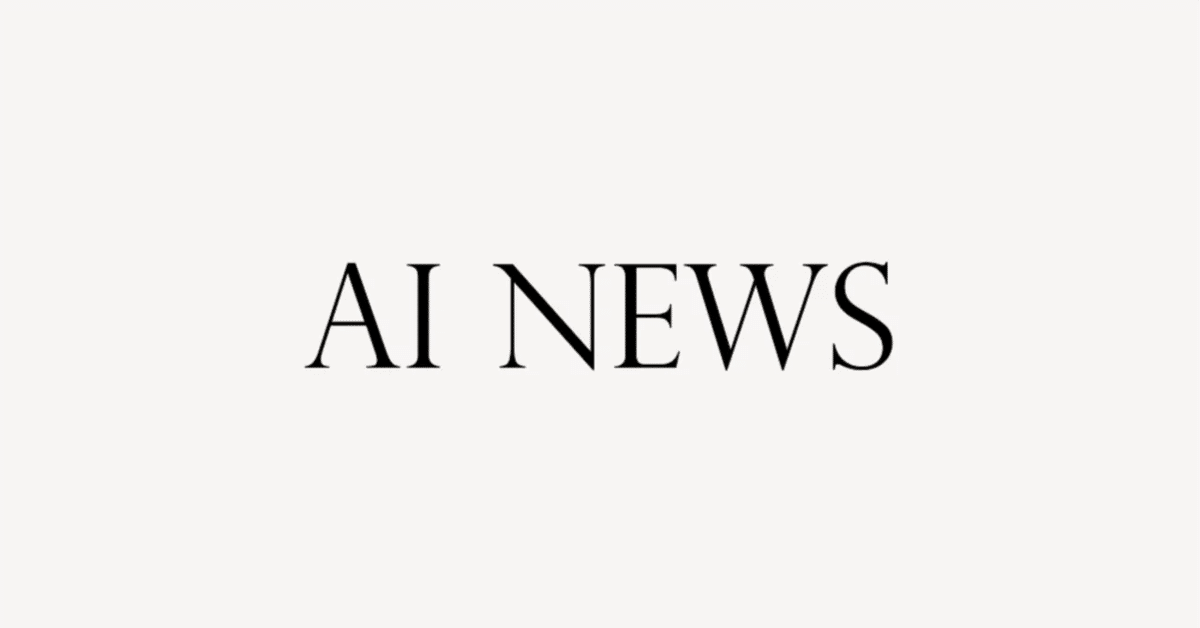
【GeminiとFAn:GoogleとMIT/ハーバードの最先端AI技術】英語解説を日本語で読む【2023年8月25日|@AI Revolution】
この動画では、Googleが開発中の新しいAIアシスタント「Gemini」と、MITとハーバード大学の研究者によって開発された追跡システム「FAn」について紹介されています。Geminiは、テキスト、画像、音声などの異なるデータタイプを同時に処理できる大規模な言語モデルで、現在のGoogleツールや製品の改善、多様で革新的な結果の生成、そしてビジネスや開発者のプロジェクトに活用されることを目指しています。一方、FAnは、カメラとクエリだけでリアルタイムにオブジェクトを追跡し、セグメンテーションすることができるシステムで、既存のメソッドに比べて正確で堅牢です。両方のシステムの開発は進行中で、Geminiの詳細は秋に公開され、FAnのコードとモデルはオンラインで公開されています。
公開日:2023年8月25日
※動画を再生してから読むのがオススメです。
As we all know, Google recently launched a new AI assistant which many believe is a trial run for an upcoming project named Gemini.
周知のように、グーグルは最近、新しいAIアシスタントを発表したが、これはジェミニと名付けられた次期プロジェクトの試験運用だと多くの人が考えている。
They're trying out various AI features to see how users respond.
ユーザーの反応を見るために、様々なAI機能を試しているのだ。
Gemini is anticipated to integrate everything from AlphaGo to Google's AI search.
ジェミニは、アルファ碁からグーグルのAI検索まですべてを統合すると予想されている。
It aims to be the most powerful AI system ever made, potentially transforming the internet and our daily lives.
ジェミニは、これまで作られた中で最も強力なAIシステムとなることを目指しており、インターネットと私たちの日常生活を一変させる可能性を秘めている。
In this video, we'll discuss the Gemini Project.
このビデオでは、ジェミニ・プロジェクトについて説明する。
Later on, we'll also cover a new AI project from MIT and Harvard University called FAn.
その後、MITとハーバード大学の新しいAIプロジェクト「FAn」についても取り上げます。
This is another groundbreaking development, so it's worth watching the video till the end to learn all about it.
これも画期的な開発なので、ビデオを最後まで見て、そのすべてを知る価値がある。
So initially, Gemini was a product of the Gemini Project by Google DeepMind, the group behind AlphaGo, the AI that defeated the Go world champion in 2016.
つまり当初、Geminiは、2016年に囲碁の世界チャンピオンを破ったAI、AlphaGoの開発グループであるGoogle DeepMindによるGeminiプロジェクトの成果物だったのだ。
The aim of the Gemini Project is to build a universal AI that can tackle any task with any kind of data without specific models.
ジェミニ・プロジェクトの目的は、特定のモデルなしに、あらゆる種類のデータであらゆるタスクに取り組むことができる普遍的なAIを構築することだ。
Gemini is the initial phase of this project.
ジェミニはこのプロジェクトの初期段階である。
It's a big language model that processes text, images, videos, and more.
テキスト、画像、動画などを処理する大きな言語モデルだ。
It can even create content like turning text into a video or turning speech into an image.
テキストを動画にしたり、音声を画像にしたりと、コンテンツを作成することもできる。
The potential uses are vast.
潜在的な用途は膨大だ。
Gemini uses techniques from AlphaGo, including reinforcement learning, training AI through feedback, and tree search exploring possible action outcomes.
Geminiは、強化学習、フィードバックによるAIの訓練、可能性のある行動の結果を探索するツリー探索など、AlphaGoの技術を使用している。
By mixing these with language models, Gemini can address challenges in various areas.
これらを言語モデルと組み合わせることで、ジェミニは様々な分野の課題に対処することができる。
What's special about Gemini is its architecture that focuses on handling different data types simultaneously.
ジェミニの特徴は、異なるデータタイプを同時に扱うことに重点を置いたアーキテクチャにある。
For instance, if you provide a text describing a scene, Gemini can create a corresponding image, video, and sound.
例えば、シーンを説明するテキストを提供すると、Geminiは対応する画像、ビデオ、サウンドを作成することができる。
Conversely, from an image, video, or sound, it can produce a descriptive text.
逆に、画像、ビデオ、サウンドから、説明的なテキストを生成することもできる。
Gemini has an advantage over other AI systems because it can handle multiple types of content like text, images, and audio all at once.
ジェミニは、テキスト、画像、音声のような複数の種類のコンテンツを一度に扱うことができるため、他のAIシステムよりも優れている。
In contrast, OpenAI's ChatGPT is great at creating text but struggles with images, videos, or audio.
対照的に、OpenAIのChatGPTはテキストを作成するのは得意だが、画像や動画、音声を扱うのは苦手だ。
If you wanted to use OpenAI for those, you'd have to use different models like DALL-E for pictures or Jukebox for songs.
それらにOpenAIを使いたければ、画像ならDALL-E、曲ならJukeboxのような別のモデルを使う必要がある。
With Gemini, it's all combined.
Geminiでは、それがすべて統合されている。
So why is Google working on Gemini?
では、なぜグーグルはGeminiに取り組んでいるのか?
There are a few reasons.
理由はいくつかある。
First, Google sees potential in improving their current tools and products with Gemini.
第一に、グーグルはGeminiによって現在のツールや製品を改善できる可能性を見出している。
For example, their chatbot Barred and their search engine could benefit.
例えば、彼らのチャットボットBarredや検索エンジンは恩恵を受ける可能性がある。
Think about asking Gemini anything and getting an answer in any format you like.
Geminiに何でも尋ねて、好きな形式で答えを得ることを考えてみてほしい。
It's efficient and can quickly solve problems using Google's vast resources.
効率的で、グーグルの膨大なリソースを使って素早く問題を解決できる。
Second, Google has a lot of data, more than many of its rivals.
第二に、グーグルはライバルの多くよりも多くのデータを持っている。
This data comes from places like YouTube, Google Books, their main search index, and academic content from Google Scholar.
このデータは、YouTube、Google Books、主要検索インデックス、Google Scholarの学術コンテンツなどから得られる。
Using all this information, Google can train better models and produce varied and innovative results.
これらすべての情報を利用することで、グーグルはより優れたモデルを訓練し、多様で革新的な結果を生み出すことができる。
But we need to go.
しかし、我々は行く必要がある。
And third, Google plans to offer Gemini to users of its Cloud platform.
そして第三に、グーグルはジェミニをクラウドプラットフォームのユーザーに提供する予定だ。
This means businesses and developers could use Gemini's abilities for their projects.
これは、企業や開発者がジェミニの能力をプロジェクトに利用できることを意味する。
They might develop unique learning resources, create assistive tech, or generate new content using ambient computing.
ユニークな学習リソースを開発したり、支援技術を開発したり、アンビエント・コンピューティングを使って新しいコンテンツを生成したりするかもしれない。
So when can we expect to see Gemini in action?
では、ジェミニが実際に使われるのはいつになるのだろうか?
Well, Google hasn't announced an official release date yet, but they have said that they will reveal more details about the project in the fall of this year.
グーグルはまだ正式なリリース日を発表していないが、今年の秋にはプロジェクトの詳細を明らかにすると述べている。
So stay tuned for more updates on this exciting development.
このエキサイティングな開発に関する最新情報をお楽しみに。
In the meantime, let me know what you think about Gemini in the comments below.
とりあえず、Geminiについてどう思うか、以下のコメントで教えてください。
Do you think it will surpass ChatGPT and other AI systems?
ChatGPTや他のAIシステムを超えられると思いますか?
What kind of content would you like to see Gemini generate?
Geminiがどのようなコンテンツを生成するのを見たいですか?
How would you use Gemini if you had access to it?
ジェミニにアクセスできたら、どのように使いますか?
It's still crazy to see how quickly this all escalated.
こんなに早くエスカレートするなんて、今でもクレイジーだよ。
I mean, before most people even started using ChatGPT, AI played a big role in boosting the US economy.
つまり、ほとんどの人がChatGPTを使い始める前から、AIはアメリカ経済を押し上げる大きな役割を果たしていたのだ。
While this is great news for many of us, it's worrying that aside from big tech companies using AI, many other companies aren't doing well financially.
これは私たちの多くにとって素晴らしいニュースだが、AIを利用している大手テック企業とは別に、他の多くの企業が財務的にうまくいっていないことが心配だ。
Last year, some top investors started buying assets like fine art instead of stocks to spread their risks.
昨年、一部の一流投資家はリスクを分散するために、株式の代わりに美術品などの資産を買い始めた。
Today's sponsor, Masterworks, offers you this diversification strategy, which was once exclusive to the wealthiest people on Earth.
本日のスポンサーであるマスターワークスは、かつて地球上で最も裕福な人々だけが行っていたこの分散投資戦略を提案する。
They've compiled decades worth of auction data to invest in art they believe will appreciate in value.
数十年分のオークション・データをまとめ、価値が上がると思われる美術品に投資するのだ。
They buy it upfront, qualify it with the SEC, and break it into investable shares.
彼らは前もってそれを購入し、SECで資格を与え、投資可能な株式に分割する。
Net proceeds from its eventual sale are then distributed to its investors.
最終的な売却から得られる純収益は、投資家に分配されます。
Now, I'm not a financial advisor, and historical returns are not a guarantee for future returns, but the results are so impressive.
私はファイナンシャル・アドバイザーではないし、過去のリターンが将来のリターンを保証するものでもない。
In just a few years, they've sold over $45 million worth of artwork, and just a couple weeks ago, they sold a Cecily Brown painting for a jaw-dropping 77% annualized net returns, bringing their streak to 15 straight profitable exits.
わずか数年で、彼らは4500万ドル以上の美術品を売り上げ、数週間前にはセシリー・ブラウンの絵画を驚異的な77%の年間正味収益率で売却し、15回連続で利益を上げました。
Masterworks has over 800,000 users, and their art offerings have sold out within hours, which is why they've had to make a waitlist.
Masterworksは80万人以上のユーザーを持ち、彼らの提供するアートは数時間で完売している。
But my viewers can skip the line and get priority access right now by clicking the link in the description.
しかし、私の視聴者は、説明文にあるリンクをクリックすることで、行列をスキップして今すぐ優先アクセスを得ることができる。
Alright, now let's discuss FAn, short for Follow Anything.
さて、ここでFAn(Follow Anythingの略)について説明しよう。
This is a new system developed by MIT and Harvard researchers that allows robots to track any object in real time using just a camera and a simple query, whether it's text, image, or a click.
これはMITとハーバードの研究者が開発した新しいシステムで、カメラと簡単なクエリ(テキスト、画像、クリックなど)だけで、ロボットがリアルタイムであらゆる物体を追跡できる。
In this video, I'll break down what FAn is and why it's impressive.
このビデオでは、FAnがどのようなもので、なぜ印象的なのかを説明する。
FAn uses the Transformer architecture for visual object tracking.
FAnはビジュアルオブジェクトトラッキングにTransformerアーキテクチャを使用しています。
Transformers, commonly known for advancing natural language processing (NLP), can do things like generate text and translate languages.
Transformerは一般的に自然言語処理(NLP)を進歩させることで知られ、テキストを生成したり言語を翻訳したりすることができる。
The researchers were curious to see if Transformers could also be effective with images.
研究者たちは、トランスフォーマーが画像にも有効かどうか知りたかったのだ。
You see, most of the existing robotic systems that can follow objects use convolutional neural networks (CNNs). These are another type of neural network that can process images by applying filters and pooling operations.
物体を追跡できる既存のロボットシステムのほとんどは、畳み込みニューラルネットワーク(CNN)を使っている。これもニューラルネットワークの一種で、フィルターやプール演算を適用して画像を処理することができる。
CNNs are great for tasks like image classification and segmentation, but they have some limitations when it comes to tracking and following objects.
CNNは画像の分類やセグメンテーションのようなタスクには最適だが、物体の追跡や追従に関してはいくつかの制限がある。
For example, they can only handle a fixed set of object categories that they have been trained on.
例えば、CNNが扱えるのは、訓練されたオブジェクト・カテゴリの固定セットのみである。
They also require a lot of manual tuning and calibration to work well in different environments and scenarios.
また、さまざまな環境やシナリオでうまく動作させるためには、多くの手動チューニングやキャリブレーションが必要になる。
And they are not very user-friendly because they often need complex inputs like bounding boxes or masks to specify the target object.
また、ターゲットオブジェクトを指定するために、バウンディングボックスやマスクのような複雑な入力を必要とすることが多いため、使い勝手が良いとは言えません。
FAn solves these problems by using a different approach.
FAnは、異なるアプローチを用いることで、これらの問題を解決する。
Instead of CNNs, it uses Vision Transformers or ViTs.
CNNの代わりに、ヴィジョン・トランスフォーマー(ViT)を使うのだ。
These are Transformers that can process images by splitting them into patches and treating them as sequences of tokens.
これは画像をパッチに分割し、トークンのシーケンスとして処理するトランスフォーマーである。
ViTs can learn to capture the relationships between different parts of an image, just like Transformers can capture the relationships between different words in a text.
ViTsは、トランスフォーマーがテキスト中の異なる単語間の関係を捉えることができるように、画像の異なる部分間の関係を捉えることを学習することができる。
And because they are based on attention mechanisms, they can focus on the most relevant parts of the image for the task at hand.
また、ViTは注意メカニズムに基づいているため、目の前のタスクに最も関連する画像の部分に焦点を当てることができる。
FAn uses ViTs for real-time tracking and segmentation of objects in videos.
FAnは、ViTをビデオ内のオブジェクトのリアルタイム追跡とセグメンテーションに使用する。
It identifies the object and distinguishes it from the background.
それは対象物を識別し、背景と区別します。
All it requires is a bounding box.
必要なのはバウンディングボックスだけです。
After that, you can guide FAn to recognize new objects by typing a description, showing a picture, or clicking on the object in the video.
その後、説明を入力したり、画像を表示したり、ビデオ内のオブジェクトをクリックしたりすることで、FAnに新しいオブジェクトを認識させることができます。
For instance, if you want FAn to track a red ball, type red ball, show a picture of one, or click on it in the video.
例えば、FAnに赤いボールを追跡させたい場合、red ballと入力するか、赤いボールの写真を表示するか、ビデオの中で赤いボールをクリックします。
FAn will then track the red ball throughout the video.
FAnはビデオを通して赤いボールを追跡します。
You can easily switch to a different object by changing your instruction.
指示を変えれば、簡単に別のオブジェクトに切り替えることができる。
What's impressive is FAn isn't just limited to tracking one item.
印象的なのは、FAnが1つのアイテムを追跡するだけに限定されていないことだ。
It can track multiple objects simultaneously by just giving separate instructions for each.
それぞれに別々の指示を与えるだけで、複数の物体を同時に追跡できるのだ。
FAn has shown impressive performance in visual object tracking and segmentation, achieving top results in real time.
FAnは、ビジュアルオブジェクトのトラッキングとセグメンテーションで素晴らしいパフォーマンスを示し、リアルタイムで最高の結果を達成している。
It operates at about 55 frames per second on a standard GPU and can tackle challenges like occlusions, fast motion, and background disturbances.
標準的なGPUで1秒間に約55フレームで動作し、オクルージョン、速い動き、背景の乱れなどの課題に取り組むことができる。
When compared to popular CNN-based methods like Siam Mask and Segurat, FAn was more accurate and robust.
Siam MaskやSeguratのような一般的なCNNベースの手法と比較すると、FAnはより正確でロバストであった。
Unlike those methods, FAn can work across various datasets without extra training.
これらの手法とは異なり、FAnは余分な訓練なしで様々なデータセットに対応できる。
This progress suggests a future where robots can easily and smartly interact with any object in any setting.
この進歩は、ロボットがどのような環境でも、どのような物体とも簡単かつスマートに対話できる未来を示唆している。
Imagine a robot assistant that understands your commands and does tasks like fetching or cleaning, or a robot that can play games and explore unknown places.
あなたの命令を理解し、取ってきたり掃除をしたりするアシスタントロボットや、ゲームをしたり未知の場所を探検したりするロボットを想像してみてほしい。
So the future looks promising, I guess.
将来は有望に見えますね、おそらく。
And the best part is that FAn is not some proprietary technology that only a few people can access.
そして何より素晴らしいのは、FAnが一部の人しかアクセスできない独占技術ではないということだ。
The researchers have made their code and models available online for anyone to use and improve.
研究者たちはコードとモデルをオンラインで公開し、誰でも利用したり改良したりできるようにしている。
You can find it on GitHub repository, and I highly encourage you to check it out and try it for yourself.
GitHubのリポジトリで見ることができるので、ぜひチェックして自分で試してみてほしい。
Alright, thanks for watching the video.
さて、ビデオを見てくれてありがとう。
I hope you found it informative.
参考になっただろうか?
If you did, make sure to hit the like button and subscribe to my channel.
もし見ていただけたら、「いいね!」ボタンとチャンネル登録をお願いします。
And remember to click the bell icon to stay updated on new uploads.
また、ベルアイコンをクリックすると、新しいアップロードが更新されます。
Thanks again, and I'll see you in the next one.
改めてありがとうございます。次回お会いしましょう。
この記事が気に入ったらサポートをしてみませんか?