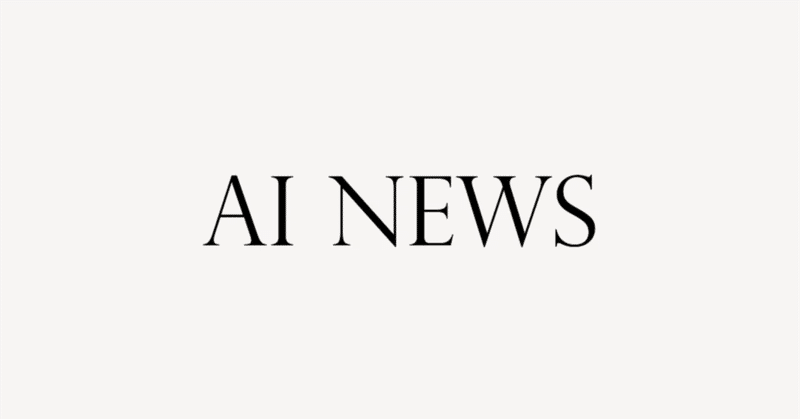
【Sora:Unreal Engine 5との結びつきを探る】英語解説を日本語で読む【2024年2月18日|@Wes Roth】
この動画では、OpenAIの背後にある大規模な取り組みと、我々がまだ見ていないような進歩について語られています。特に、Soraという最先端のAIビデオ生成モデルに焦点を当て、そのビデオ生成の能力と、それが現実と区別がつかないほどのビデオを作成する点に迫ります。Soraは単なるビデオ生成プラットフォームではなく、データ駆動型の物理エンジンであり、実際的な物理法則や長期的な推論、意味の理解を含む多くの世界をシミュレートする能力を持っています。この動画では、Soraがどのように3D世界や物理法則を驚くほど正確に生成できるのか、そしてそれがビデオゲームエンジン、特にUnreal Engine 5とどのように関連しているのかを探ります。さらに、Soraが合成データをトレーニングに使用している可能性が示唆され、AIの将来における合成データの使用がどのように拡張可能かについても議論されます。
公開日:2024年2月18日
※動画を再生してから読むのがオススメです。
So there's a lot of stuff brewing behind the scenes at OpenAI.
OpenAIでは裏でたくさんのことが進行中です。
As you'll see, OpenAI might be thinking multiple steps ahead, steps that we can't even see.
ご覧の通り、OpenAIは複数のステップ先を考えているかもしれません。私たちには見えないステップです。
Keep this image in mind.
このイメージを心に留めておいてください。
What OpenAI is showing us is just the tip of the iceberg.
OpenAIが私たちに示しているのは氷山の一角に過ぎません。
What's available behind the scenes is far bigger and greater than we know.
裏側にあるものは、私たちが知っている以上にはるかに大きく、偉大です。
Who would have predicted this?
誰がこれを予測したでしょうか?
That OpenAI would unleash something like Sora?
OpenAIがSoraのようなものを解き放つとは?
Far and away, the best AI video generation model.
はるかに、最高のAIビデオ生成モデルです。
We're getting very close to the point where AI video will be indistinguishable from reality.
AIビデオが現実と区別がつかなくなるという点に非常に近づいています。
But as you'll see in a second, this is just what's showing.
しかし、すぐにご覧いただく通り、これはただの表示に過ぎません。
What's behind the scenes is far bigger.
裏側にあるものははるかに大きいです。
In this video, let's see why Sora is different.
このビデオでは、なぜSoraが異なるのかを見てみましょう。
What do you think you're looking at right now?
今、何を見ていると思いますか?
Minecraft?
マインクラフト?
Well, not quite.
ちょっと違います。
This is generated by Sora.
これはSoraによって生成されました。
Why does it look like a 3D world in a video game?
なぜビデオゲームの中の3D世界のように見えるのでしょうか?
Why does it seem like the physics are incredibly well generated with something that's supposed to be just a video generation platform?
物理学が信じられないほどよく生成されているように見えるのはなぜでしょうか?それはただのビデオ生成プラットフォームであるべきものです。
The glass doesn't shatter, but notice the ice cubes, notice the water.
ガラスは割れませんが、氷のキューブに注目して、水に注目してください。
What does that look like to you?
それはあなたにとってどう見えますか?
In this video, let's talk about video generation, Sora, AI video models as world simulations.
このビデオでは、ビデオ生成、Sora、AIビデオモデル、世界シミュレーションについて話しましょう。
And what does the Unreal game engine have to do with all of this?
そして、これにアンリアルゲームエンジンがどのように関係しているのか?
Let's dive in.
さあ、始めましょう。
So I'll link below to a previous video where we went over all the various videos that are available to see that are made by Sora.
以前のビデオへのリンクを以下に貼り付けます。そこでは、Soraによって作成されたさまざまなビデオをすべて見ることができます。
So here we're not going to be actually showcasing all of them.
ここでは、実際にはそれらすべてを紹介することはありません。
We're mainly going to be digging into how the heck did they manage to create this and also specifically what this means.
主に、彼らがこれをどのように作成したのか、そして具体的にこれが何を意味するのかについて掘り下げていきます。
What does this mean?
これは何を意味するのでしょうか?
What I think it means is that opening eyes building at a light speed of things that we can't even see.
私が考えるに、これが意味するのは、私たちが見ることさえできないものの光速で進化しているということです。
Let's start here.
ここから始めましょう。
This is Dr. Jim Fan, one of the senior AI researchers at NVIDIA, covered a lot of his papers here.
これはNVIDIAのシニアAI研究者であるジム・ファン博士で、彼の論文の多くがここで取り上げられています。
So he's saying this.
彼はこう言っています。
If you think opening eyes Sora is a creative toy like DALL·E, think again.
目を開けたSoraがDALL·Eのような創造的なおもちゃだと思っているなら、もう一度考え直してください。
Sora is a data-driven physics engine.
Soraはデータ駆動型の物理エンジンです。
It is a simulation of many worlds, real or fantastical.
それは多くの世界、現実的または幻想的なもののシミュレーションです。
The simulator learns intricate rendering, intuitive physics, long horizon reasoning, and semantic grounding, all by some denoising and gradient maths.
このシミュレータは、複雑なレンダリング、直感的な物理、長期的な推論、および意味論的な基盤を、いくつかのノイズ除去と勾配数学によって学習します。
I won't be surprised if Sora is trained on lots of synthetic data using Unreal Engine 5.
私は、SoraがUnreal Engine 5を使用して多くの合成データで訓練されていると驚かないでしょう。
It has to be.
それはそうでなければなりません。
And he's not the only person mentioning that.
そして、彼だけがそれを言及しているわけではありません。
Multiple people have pointed out that a lot of the footage does have some like video game qualities.
複数の人々が、映像の多くにビデオゲームのような要素があると指摘しています。
Dr. Jim Fan continues here.
ジム・ファン博士はここで続けます。
Let's break down the following video prompt.
次のビデオプロンプトを分析してみましょう。
Photorealistic close up of video of two pirate ships battling each other as they sail inside a cup of coffee, which, by the way, I mean, if you take a look at this video, you know exactly what the prompt was.
コーヒーカップの中を航行しながら互いに戦う2隻の海賊船のビデオの写実的なクローズアップ、というものですが、ちなみに、このビデオを見れば、プロンプトが何であるかがすぐにわかります。
I mean, you can see the cup, you can tell this is coffee.
つまり、カップが見えるし、これがコーヒーであることもわかります。
This doesn't look like water.
これは水のようには見えません。
That looks like coffee.
それはコーヒーのように見えます。
With the froth on top and everything else.
上に泡が乗っていて、その他もすべてそろっています。
You know, those are two pirate ships.
あの、あれは2隻の海賊船です。
And you can tell that they're miniature pirate ships because, again, they fit into a cup of coffee.
そして、それらがミニチュアの海賊船であることがわかります。再び、コーヒーカップに収まるからです。
He continues, the simulator instantiates two exquisite 3D assets, pirate ships with different decorations.
彼は続けます、シミュレータは2つの精巧な3Dアセットをインスタンス化します。異なる装飾の海賊船です。
Sora has to solve text to 3D implicitly in its latent space.
Soraは、その潜在空間でテキストから3Dへの解決策を暗黙的に解決しなければなりません。
If some of these words make no sense to you, it's going to make a lot more sense once we start unpacking it.
これらの単語のいくつかが理解できない場合、私たちがそれを解説し始めると、それははるかに理解しやすくなります。
I'll show you some research that shows why this, what you're seeing here, why the thing that produces this, the digital brain that produces it, is probably far stranger than you might know.
ここで見ているもの、これを生み出すもの、デジタル脳がそれを生み出すものが、おそらくあなたが知っているよりもはるかに奇妙である理由を示す研究をお見せします。
He continues, the 3D objects are consistently animated as they sail and avoid each other's paths.
彼は続けます、3Dオブジェクトは、航海してお互いの航路を避ける際に一貫してアニメーションされています。
That's important.
それは重要です。
I mean, this is a simulation of a 3D environment with physics that are carrying these things in this sort of maelstrom, whirlpool, fluid dynamics of the coffee, even the foam that forms around the ships.
つまり、これは、コーヒーの渦巻き、渦、流体力学、氷のキューブ、水、流体を含むこれらのものを運ぶ物理学を持つ3D環境のシミュレーションです。
Fluid simulation is an entire subfield of computer graphics, which traditionally requires very complex algorithms and equations.
流体シミュレーションは、従来、非常に複雑なアルゴリズムや方程式が必要とされるコンピュータグラフィックスの分野全体です。
Photorealism, almost like rendering with ray tracing.
光線追跡でレンダリングするのとほぼ同じ写実主義。
The simulator takes into account the small size of the cup compared to oceans and applies tilt shift photography to give a minuscule vibe.
シミュレータは、海と比較してカップの小ささを考慮し、微小な雰囲気を与えるためにティルトシフト写真を適用します。
The semantics of the scene does not exist in the real world, but the engine still implements the correct physical rules that we expect.
シーンの意味論は現実世界には存在しませんが、エンジンは私たちが期待する正しい物理法則を実装しています。
Next up, add more modalities and conditioning.
次に、さらにモダリティとコンディショニングを追加してください。
Then we have a full data-driven UE.
次に、完全なデータ駆動のUEがあります。
UE, I'm reading that as Unreal Engine here, that will replace all the hand-engineered graphic pipelines.
UE、ここではUnreal Engineとして読んでいますが、これはすべての手作業のグラフィックパイプラインを置き換えるものです。
So Unreal Engine, if you're not aware of it, it's this probably one of the best sort of game engines that people use to create games.
Unreal Engineは、それを知らない場合、おそらく人々がゲームを作成するために使用する最高のゲームエンジンの1つです。
It's built by developers for developers, and it basically enables people that develop games to have a lot of like the tools instead of coding up everything from scratch and creating every single part of the game from scratch.
これは開発者によって開発されたもので、基本的にはゲームを開発する人々が、すべてをゼロからコーディングしてゲームのすべての部分をゼロから作成する代わりに、多くのツールを持つことを可能にします。
This kind of provides you a workshop where you can use some existing common tools
これは、既存の一般的なツールを使用できるワークショップを提供するものです。
And then build on top of them to create your own game however you want to.
そして、それらの上に構築して、自分のゲームを自分の好きなように作成できます。
So they're saying this is the world's most advanced real-time 3D creation tool.
彼らはこれを世界で最も先進的なリアルタイム3D作成ツールだと言っています。
So this is Unreal Engine 5, and it started out kind of looking like a pixelated video game, and now a lot of the things that you can do with it are more or less indistinguishable from reality.
これがUnreal Engine 5で、最初はピクセル化されたビデオゲームのように見えていましたが、今ではそれでできることの多くは現実と区別がつかないほどです。
So the images you see here, this is a 3D creation tool that created this Unreal Engine 5.
ここで見ている画像は、このUnreal Engine 5が作成した3D作成ツールです。
So as you can see here, I mean, it looks very real, almost in a certain way more real than reality, and it creates these massively detailed worlds where every single thing is a 3D object with the shadows fall, etc., whereas they put a dynamic global illumination and reflections.
ここで見ているように、非常にリアルに見えます。ある意味では現実以上にリアルであり、影が落ちるなど、すべてのものが3Dオブジェクトであり、ダイナミックなグローバルイルミネーションと反射を施しています。
So by rendering the 3D space with the lighting and everything else, you're able to create incredibly realistic-looking images.
照明やその他の要素を含めて3D空間をレンダリングすることで、信じられないほどリアルな見た目の画像を作成できます。
And last point about the Unreal Engine is that this shot here can be moved around.
Unreal Engineに関する最後のポイントは、ここでのこのショットは移動できるということです。
You can pan left, right, up, and down.
左に、右に、上に、下にパンできます。
Each building is its own 3D asset, so you can circle around it, zoom in, zoom out, etc.
各建物は独自の3Dアセットであり、それを周囲から見回したり、ズームイン、ズームアウトすることができます。
So let's get back to Sora.
それでは、Soraに戻りましょう。
This, I think, is where OpenAI is playing on a much deeper level, on a much more advanced level than anyone else.
OpenAIが他の誰よりもはるかに深いレベルで、より高度なレベルで活動していると思います。
They are using, as far as we can tell, synthetic data to train Sora.
私たちが知る限り、Soraを訓練するために合成データを使用しています。
Now, of course, we don't know that for a fact.
もちろん、それが事実であるかどうかはわかりません。
Here, Dr. Jim Fatt continues.
ここで、ジム・ファット博士が続けます。
He's saying apparently some folks don't get data-driven physics engines.
明らかに、一部の人々はデータ駆動型の物理エンジンを理解していないようです。
So let me clarify.
私が説明します。
Sora is an end-to-end diffusion transformer model.
Soraはエンドツーエンドの拡散トランスフォーマーモデルです。
It inputs text/image and outputs video pixels directly.
テキスト/画像を入力し、ビデオピクセルを直接出力します。
Sora learns a physics engine implicitly in the neural parameters by gradient descent through massive amounts of videos.
Soraは、膨大な量のビデオを通じて勾配降下法によってニューラルパラメータ内で物理エンジンを暗黙的に学習します。
Sora is a learnable simulator or world model.
Soraは学習可能なシミュレータまたはワールドモデルです。
Of course, it does not call UE5 explicitly in a loop, but it's possible that UE5 generated...
もちろん、明示的にUE5をループ内で呼び出すわけではありませんが、UE5が生成した可能性があります...
So this is, again, Unreal Engine, that 3D asset world builder thing we were talking about.
これは再び、私たちが話していた3DアセットワールドビルダーであるUnreal Engineです。
But it's possible that UE5-generated text-video pairs are added as synthetic data to the training set.
しかし、おそらくUE5が多くの異なる画像を生成するために使用され、Soraモデルの訓練中に、UE5で作成された多くの異なるビデオが訓練セットに追加された可能性があります。
So what he's saying here is that it's possible, or at least this is the guess, that maybe Unreal Engine 5 was used to generate many, many different images, that during the training of the Sora model, they've used many different videos that were created in Unreal Engine 5.
彼が言っているのは、おそらく、少なくともこれは推測ですが、多くの異なる画像が生成されるためにUnreal Engine 5が使用された可能性があるということです。Soraモデルのトレーニング中に、Unreal Engine 5で作成された多くの異なるビデオが使用されました。
A camera flying through the city, zooming in on objects, flying through the streets like a drone, et cetera.
都市を飛び回るカメラ、オブジェクトにズームインし、ドローンのように通りを飛び回るなど。
And each had some text description of what was happening in there.
そして、それぞれに何が起こっているかのテキスト説明が付いていました。
And that combination of text and video, those pairs were added as synthetic data to the training set.
そして、そのテキストとビデオの組み合わせ、そのペアはトレーニングセットに合成データとして追加されました。
Now, the synthetic data part is big.
今、合成データの部分は重要です。
And we've talked about it quite a bit on this channel.
そして、このチャンネルでそれについてかなり話しました。
So in the last few years, a lot of people have questioned how far we're gonna be able to scale these AI neural nets, these AI models, because they've already consumed such a massive amounts of data that was generated by humans that we figured, hey, we're approaching the end of that.
ここ数年、多くの人々が疑問を持っていました。AIニューラルネット、AIモデルをどれだけスケーリングできるか。なぜなら、すでに人間が生成した膨大なデータを消費しており、私たちはその限界に近づいていると考えていました。
They already read all of the books, all of the internet.
彼らはすでにすべての本、すべてのインターネットを読みました。
So it seemed unlikely that we're gonna be able to increase the amount of text that we have available to 10x or 100x, right?
私たちが利用可能なテキストの量を10倍または100倍に増やすことができる可能性は低いようですね。
Because if you gave me all the textbooks and all the books that are written in it and everything written online, they said, okay, and now give me 100x that amount, that you'd be hard pressed to find that text elsewhere.
なぜなら、もし私にすべての教科書や書籍、オンラインで書かれたすべてのものを与えたら、それを100倍に増やしてくださいと言われたら、そのテキストを他で見つけるのは難しいでしょう。
And yet, that's what's needed to keep improving and increasing these AI models.
それでも、それが必要なのは、これらのAIモデルを改善し増やすためです。
One of the potential solutions was using synthetic data or data that was created not by humans, like a human-written book, but rather by these AI models, or in this case, Unreal Engine 5.
潜在的な解決策の1つは、合成データや人間が作成したものではなく、これらのAIモデルやこの場合はUnreal Engine 5によって作成されたデータを使用することでした。
And up until very recently, we didn't really know if it was gonna work or not.
そして、最近まで、それがうまくいくかどうかは本当にわかりませんでした。
A lot of people questioned it.
多くの人々がそれを疑問視しました。
They said the minor problems with the synthetic data will lead to corruption of the models.
彼らは、合成データのわずかな問題がモデルの破損につながると言いました。
Little mistakes here and there will compound and kind of break the model.
ここでの小さなミスは蓄積され、モデルを壊す可能性があります。
So the argument was if you create a bunch of these images and you feed it into a model, right, and something's wrong with how the legs are generated or how the fingers are generated, you can kind of tell that she's kind of sliding on the sidewalk a little bit.
議論は、これらの画像をたくさん作成してモデルに送り込み、何かが足の生成方法や指の生成方法に問題があるとき、彼女が少し歩道を滑っているのがわかるということでした。
All those little errors will kind of compound.
すべての小さなエラーが蓄積されるでしょう。
And if we just use the AI-generated data to train the next series of models, eventually those little errors will compound and just everything will kind of fall apart.
そして、次のシリーズのモデルを訓練するためにAIが生成したデータだけを使用すれば、最終的にはそれらの小さなエラーが蓄積され、すべてが崩壊してしまうでしょう。
It'll corrupt and it'll actually start getting worse and worse.
それは腐敗し、実際に悪化し始めます。
But a lot of the more recent research seems to suggest that no, that's not the case.
しかし、最近の多くの研究は、そうではないと示唆しているようです。
Orca 2 was the Microsoft open source model that was built on synthetic data, data generated by GPT-4.
Orca 2は、GPT-4によって生成されたデータで構築されたMicrosoftのオープンソースモデルでした。
Here we're seeing potentially the amazing Sora being built on synthetic data as well and looking incredibly, incredibly good.
ここでは、驚くべきSoraが合成データ上に構築され、非常に非常に良く見えている可能性があります。
And rumors are is that OpenAI kind of understood this kind of early on and they're just running with it.
そして噂によると、OpenAIはこれを早く理解していて、それに乗っかっていると言われています。
Like they might have discovered it early and just have been building and building with that idea in mind.
彼らは早くにそれを発見し、その考えを念頭に置いて建設を続けているかもしれません。
And certainly Sora, if this is true, kind of confirms that.
そして確かに、もしこれが真実なら、Soraはそれを確認するようなものです。
Now, I think this was very confusing to a lot of people because I guess according to what Dr. Jim Fan has said here, maybe some people in the comments thought, oh, so Sora just uses the Unreal Engine to generate this stuff and just spits it out.
今、これは多くの人々にとって非常に混乱していたと思います。ここでジム・ファン博士が言ったことによると、コメント欄の一部の人々は、Soraは単にUnreal Engineを使用してこれらのものを生成し、吐き出していると思ったのかもしれません。
Is that what's happening here?
ここで何が起こっているのでしょうか?
Because that is all of a sudden not that impressive, right?
なぜなら、それは突然それほど印象的ではなくなるからですよね?
But that's not the case.
しかし、それは事実ではありません。
And let me show you an interesting study that I think might help not only understand how this is happening, but it's also kind of wild in and of itself.
そして、これがどのようにして起こっているかを理解するだけでなく、それ自体もかなりワイルドなものであると思われる興味深い研究を紹介させていただきます。
So this is beyond surface statistics.
これは表面的な統計を超えています。
So it was in November, 2023 out of Harvard.
それは2023年11月、ハーバード大学でした。
And the question that I was trying to ask is how do these latent diffusion models and image generating model, the inner workings of these, they remain mysterious.
私が尋ねようとしていた質問は、これらの潜在的な拡散モデルや画像生成モデルの内部機構はどのようにして、それらは謎めいたままなのかということです。
When we train these models on images without explicit depth information, they typically output coherent pictures of 3D scenes.
これらのモデルを明示的な深さ情報のない画像で訓練すると、通常、3Dシーンの一貫した画像が出力されます。
How is that possible?
それが可能なのはどうしてでしょうか?
So really fast, a lot of these image models, this is kind of how you can think of how they generate these images.
非常に速く、これらの画像モデルの多くは、これが画像を生成する方法の考え方です。
So the idea of taking this image that we can all recognize as a dog and kind of adding more and more what they call noise to it, right?
私たちが皆が犬として認識できるこの画像を取り、それにさらに何と呼ばれるノイズを追加していくと考えることができますね。
Until it turns into static or we can't tell what it is.
それが静的に変わるか、何かわからなくなるまで。
This is how those diffusion models are trained.
これが拡散モデルが訓練される方法です。
And then to produce images, they do the sort of reverse of that, the denoising process.
そして画像を生成するために、彼らはその逆のプロセス、ノイズ除去プロセスを行います。
So they start an image out as this sort of static thing that you can't tell what it is and slowly denoise it until it becomes an image that you requested for it.
したがって、画像をこのように静的なものとして始め、それがリクエストされた画像になるまで徐々にノイズを取り除いていきます。
So for example, here, you can see this process takes shape here.
たとえば、ここでは、このプロセスがここで形を成しているのが見えます。
Step one, the first part, you can't even tell what that is.
ステップ1、最初の部分、それが何かわからないでしょう。
It's just noise, right?
それはただのノイズですね。
And slowly over time, as the denoising process happens, all of a sudden you see certain shapes take form.
そして時間の経過とともに、ノイズ除去プロセスが起こると、突然、特定の形が形成されるのが見えます。
And finally, this is the final product.
最後に、これが最終的な製品です。
As you can obviously tell, this is a red car, one of those NT cars on a green lawn.
明らかにわかるように、これは緑の芝生の上のNT車の1つである赤い車です。
And so for this experiment, they basically trained their own current neural network, their own AI model to produce these images.
そして、この実験では、彼らは基本的に自分たちの現在のニューラルネットワーク、自分たちのAIモデルを訓練して、これらの画像を生成しました。
They use the synthetic data sets.
彼らは合成データセットを使用しています。
So basically they used Stable Diffusion, the open source model, you might've heard of it.
基本的に彼らは、オープンソースのモデルであるStable Diffusionを使用しました。おそらくそれを聞いたことがあるかもしれません。
So they basically created their own synthetic data set of images and I believe they just made images of like cars and animals and people, et cetera.
基本的に彼らは、自分たちで画像の合成データセットを作成し、車や動物、人などの画像を作成したと思います。
But the important thing to understand is they just fed it 2D images.
しかし理解する重要な点は、彼らが2D画像を与えたことです。
Here's a picture of a car, here's a picture of a boat, here's a picture of a person.
こちらが車の写真、こちらが船の写真、こちらが人の写真です。
So they had those kinds of pairs of text, the description of the image and the 2D image.
彼らはそのようなテキストのペア、画像の説明と2D画像を持っていました。
The model had no concept of what 3D meant, of what depth of field meant, nothing like that.
そのモデルは、3Dとは何か、被写界深度とは何かといった概念を持っていませんでした。
And at the end of this, it was able to, if you said, make a picture of a red car, it would do something like this.
そして、最後に、もし赤い車の写真を作れと言ったら、これのようなことができました。
It would create a picture of a red car, which is great.
赤い車の写真を作成しました、それは素晴らしいことです。
This is what we expect it to do.
これが私たちが期待することです。
Here's where it gets weird.
ここで奇妙なことが起こります。
They kind of sliced into it at various parts of the generation process to see how it was sort of thinking about how to create this car.
生成プロセスのさまざまな部分でそれを切り込んで、この車を作成する方法についてどのように考えているかを見てみました。
And what seemed to be happening is that very early in the process, long before you could tell that it was a car, the AI model learned to separate objects in the foreground, sort of near the camera, like this wheel, with objects that were further in the background, like the background, the trees in the background, et cetera.
非常に早い段階で起こっているように見えることは、車であることがわかる前に、AIモデルが、カメラの近くのような前景のオブジェクト、この車輪のようなオブジェクトを、背景のような後ろのオブジェクト、背景の木々などと分離する方法を学んだということです。
So before it even made the image, it had kind of an idea in its head about where things would be placed within that image, in that 3D space, even though it was never explicitly taught what 3D meant or how to construct a 3D scene in its head, in its neural network, whatever.
画像を作成する前に、その画像内で物事がどこに配置されるかについて、その3D空間内でのアイデアを持っていましたが、3Dが何を意味するかや、頭の中で3Dシーンをどのように構築するかを明示的に教えられたことはありませんでした。
But this was something that's sometimes referred to as an emergent ability.
これは、時々新たに獲得される能力と呼ばれるものです。
In order to figure out how to build realistic-looking 2D images, it had to create a mental model of the 3D world in its head.
リアルな2D画像を作成する方法を理解するために、頭の中で3D世界の精神モデルを作成しなければなりませんでした。
So in other words, even a very simple neural net AI model like this, when we feed it 2D images, it learns to have a 3D sort of representation of space in order to create those images.
非常に単純なニューラルネットAIモデルであっても、2D画像を与えると、それらの画像を作成するために空間の3D的な表現を持つように学ぶのです。
We don't teach it to do that.
私たちはそれにそうするように教えません。
We don't push it in any way to do that.
私たちはそれを何らかの方法で押し付けることはありません。
It kinda happens.
それはなんとなく起こる。
And so when Dr. Jim Fan is saying, Sora learns a physics engine implicitly in the neural parameters by gradient descent through massive amounts of videos, I think that's kind of similar to what this paper's saying.
そして、ジム・ファン博士が言っているように、Soraはビデオの大量の勾配降下を通じてニューラルパラメータ内で物理エンジンを暗黙的に学ぶということは、この論文が言っていることと似ていると思います。
So Sora learned a physics engine implicitly, meaning it wasn't taught.
Soraは物理エンジンを暗黙的に学んだので、それが教えられたわけではありません。
We didn't tell it how physics worked.
それに物理学がどのように機能するかを教えませんでした。
We just showed it a massive amounts of videos.
ただ大量のビデオを見せただけです。
And in its brain and its neural parameters, it was like, okay, I kinda get how physics works now.
そして、その脳とニューラルパラメータの中で、物理学がどのように機能するかをなんとなく理解したという感じでした。
By the way, if you wanna know what the really big deal, why everybody's freaking out about AI and why everybody's so obsessed with it, I think this sentence, if you understand what this sentence means, this is kind of the big deal.
ちなみに、本当に大きな問題、なぜみんながAIに熱狂し、なぜみんながそれに夢中なのかを知りたい場合は、この文が何を意味するかを理解すると、これが大きな問題だと思います。
We've figured out how to make computers think and learn and create certain mental models similar to, I would say, how humans do it.
私たちはコンピューターが考え、学び、特定の精神モデルを作成する方法を見つけ出したということです。それは、人間がそれを行う方法に似ていると言えるでしょう。
So Dr. Jim Fan continues.
続けて、ジム・ファン博士は言います。
So there's some vocal objections apparently in the comments.
コメントには明らかにいくつかの声の反対があります。
So people are saying Sora is not learning physics.
人々は、Soraが物理学を学んでいないと言っています。
It's just manipulating pixels in 2D.
それは単に2Dのピクセルを操作しているだけです。
And as somebody that's been posting videos about this for quite a while now, I see this in the comments.
これについてかなり長い間ビデオを投稿してきた人として、私はコメントでこれを見ます。
It's a small minority of people, but they really argue hard against this.
人々の中でごく少数ですが、彼らは本当にこれに反対して強く主張しています。
They say, AI, it doesn't reason, it doesn't think, it doesn't understand.
彼らは、AIは論理を持たず、考えを持たず、理解を持たず、と言います。
It's not learning physics.
それは物理学を学んでいないと。
And truth be told, we probably don't fully understand everything yet.
実際、私たちはまだすべてを完全に理解していないかもしれません。
So I'm not really saying that we know exactly what it's doing, but things like this, right, as this paper here states, how do these neural networks transform, say the phrase, car in the street into a picture of an automobile on the road?
私は正確に何をしているかを知っているとは言っていませんが、この論文が述べているように、これらのニューラルネットワークが、例えば、道路上の自動車の写真に、通りにある車というフレーズをどのように変換するのか、ということです。
Do they simply memorize superficial correlations between pixel values and words?
彼らは単にピクセル値と単語の間の表面的な相関を記憶しているだけでしょうか?
And this is what a lot of these people, they want us to believe, the people that are arguing against it, is just pixels in 2D.
そして、これが反対している多くの人々が私たちに信じさせたいと思っていることです、それは単なる2Dのピクセルだけです。
This is just manipulating little pixels.
これは単なる小さなピクセルを操作しているだけです。
It has no understanding of how physics works, how water works.
これは物理学がどのように機能し、水がどのように機能するかを理解していないのです。
This is just pixels on a screen.
これはただ画面上のピクセルです。
That's all it's doing, right?
それがやっていることはそれだけですか?
It has no concept of how fluids move or how coffee, the little foam buildup is different than water.
それは流体がどのように動くか、コーヒーが水と異なるような泡の蓄積の概念を持っていません。
And at this point, I gotta say, probably not.
そしてこの時点で、おそらくそうではないと言わなければなりません。
It seems like they are learning something deeper, such as an underlying model of objects, such as cars, roads, and how they are typically positioned.
彼らは、車や道路などのオブジェクトの基本的なモデル、およびそれらが通常どのように配置されているかなど、より深い何かを学んでいるようです。
And by the way, there are other researchers that have found this idea that AI neural nets create certain world models.
ちなみに、他の研究者たちも、AIニューラルネットが特定の世界モデルを作成するという考えを見つけています。
Certain understanding of how their world works.
彼らの世界がどのように機能するかを理解する特定のもの。
In this research, you can find by searching for Othello-GPT, a GPT model similar to opening eyes, ChatGPT.
この研究では、Othello-GPTという検索して見つけることができる、ChatGPTと同様のGPTモデルがあります。
It started out as a blank slate.
それは真っ白な板から始まりました。
So no words, no images, no pictures, nothing.
言葉も画像も写真も何もありませんでした。
And it was fed nothing but Othello moves, this game that you play on a board.
そして、ボード上でプレイするこのゲーム、オセロの手だけを与えられました。
Looks kind of like this.
これは、こんな感じです。
So it was fed millions of these until it was able to predict a legal next move it could make, which is what we would kind of expect it to do.
それが次に取ることができる合法的な手を予測できるようになるまで、何百万もの手を与えられました。それは私たちが期待することです。
But when they dug deeper, it seemed like this neural net developed a representation of the board state, of where the pieces were, of whether they're the opponent's colors or my color.
しかし、さらに掘り下げると、このニューラルネットは、どこに駒があるか、それらが相手の色か自分の色かなど、ボードの状態の表現を開発したようでした。
Now, again, it had no idea about any of these.
さらに一度言いますが、これらのことについては何の知識もありませんでした。
It didn't have any idea that there was a board or pieces or rules of the game.
ボードや駒、ゲームのルールがあることも知りませんでした。
And yet it created a sort of representation in its brain of how that game is played, based on nothing but moves of these games being given to it to train on.
それでも、それは脳内にそのゲームがどのようにプレイされるかの表現を作り出しました。ただし、それに与えられたこれらのゲームの手を訓練することに基づいています。
And in all these cases, people are saying, no, it's just predicting the next move.
そして、これらすべての場合において、人々は言っています、「いいえ、それはただ次の手を予測しているだけです」。
Nothing else is happening.
他に何も起こっていません。
There's no understanding or anything like that.
それを理解することはできません。
It's just a stochastic parrot repeating the data it's been fed.
それは単に与えられたデータを繰り返す確率的なオウムです。
And same thing here with Dr. Jim Fan.
そして、ここでもジム・ファン博士も同じです。
So people are saying, no, it's not learning physics, you silly AI senior researcher at NVIDIA.
人々は言っています、「いいえ、あなた、NVIDIAのAIシニアリサーチャー、物理学を学んでいるわけではありませんよ」。
It's just manipulating pixels in 2D, right?
それは単に2Dのピクセルを操作しているだけですよね?
Because obviously random Twitter comments know better than the people that are studying this their entire life.
明らかに、ランダムなTwitterのコメントが、これを一生研究している人々よりもよく知っているということです。
And he's saying, I respectfully disagree with this reductionist view.
そして彼は言っています、「この還元主義的な見方には異議を唱えます」。
It's similar to saying GPT-4 doesn't learn coding.
GPT-4がコーディングを学んでいないと言うのと同様です。
It's just sampling strings.
それは単に文字列をサンプリングしているだけです。
Well, what transformers do is just manipulating a sequence of integers, token IDs.
トランスフォーマーが行うことは、整数、トークンIDのシーケンスを操作するだけです。
What neural networks do is just manipulating floating numbers.
ニューラルネットワークが行うことは、浮動小数点数を操作するだけです。
That's not the right argument.
それは正しい議論ではありません。
Sora's soft physics simulation is an emergent property as you scale up text to video training massively.
Soraのソフト物理シミュレーションは、テキストからビデオトレーニングへと大規模にスケールアップする際の、新たな特性として現れます。
This is the controversial thing.
これは論争の的です。
This is the thing that I think people are kind of a little bit scared of that they're arguing against anytime you suggest that these things understand something.
これは、人々が少し怖がっていると思うことです。これらのことが何かを理解していると提案すると、反対する人がいます。
Some portion of people, some percentage get really kind of mad and they call you names and they tell you you're crazy.
一部の人々、一部の割合が本当に怒って、あなたに罵り言葉を浴びせ、狂っていると言います。
But again, at this point, so this is Dr. Jim Fan.
しかし、この時点で、これはジム・ファン博士です。
Andrew Ang has stated that he believes that neural networks on some level understand, or at least in terms of the fact that they build these mental models, that that shows some level understanding.
アンドリュー・アングは、ニューラルネットワークがある程度理解していると信じています。少なくとも、それらがこれらのメンタルモデルを構築するという事実において、それはあるレベルの理解を示しています。
Jeffrey Hinton, who's called the godfather of AI, also said something very similar along those lines.
AIの教父と呼ばれるジェフリー・ヒントンも、非常に似たようなことを述べました。
This isn't just a thing that spits out something that's statistically likely.
これは、統計的に可能性が高いものを出力するだけのものではありません。
There's something deeper happening here.
ここには、より深い何かが起こっています。
There are these emergent properties.
これらは新たに現れる特性です。
And so he continues, GPT-4 must learn some form of syntax, semantics, and data structures internally in order to generate executable Python code.
そして、彼は続けます。GPT-4は、実行可能なPythonコードを生成するために、内部である形式の構文、意味論、およびデータ構造を学ばなければなりません。
GPT-4 does not store Python syntax trees explicitly.
GPT-4はPythonの構文木を明示的に保存しません。
That's not a point.
それはポイントではありません。
GPT-4 and other AI models, they don't store the information they see.
GPT-4や他のAIモデルは、彼らが見た情報を保存しません。
There isn't sentences of text that are stored in it.
それには、テキストの文章が保存されているわけではありません。
Very similarly, Sora must learn some implicit forms of text to 3D, 3D transformations, ray traced rendering, and physical rules in order to model the video pixels as accurately as possible.
非常に同様に、Soraはビデオピクセルをできるだけ正確にモデル化するために、3D、3D変換、レイトレーシング、物理的なルールの暗黙の形式を学ばなければなりません。
It has to learn concepts of a game engine to satisfy the objective.
目的を満たすために、ゲームエンジンの概念を学ばなければなりません。
If we don't consider interactions, the Unreal Engine 5 is a very sophisticated process that generates video pixels.
インタラクションを考慮しないと、Unreal Engine 5はビデオピクセルを生成する非常に洗練されたプロセスです。
Sora is also a process that generates video pixels, but based on end-to-end transformers.
Soraもビデオピクセルを生成するプロセスですが、エンドツーエンドの変換器に基づいています。
They are on the same level of abstraction.
それらは同じ抽象化レベルにあります。
The difference is that UE5 Unreal Engine 5 is handcrafted and precise, but Sora is purely learned through data and it's intuitive.
違いは、UE5 Unreal Engine 5が手作りで正確であるのに対し、Soraは純粋にデータを通じて学習され、直感的であることです。
And this is the big difference between computers of old, computer programs that were coded.
これが昔のコンピュータ、コード化されたコンピュータプログラムとの大きな違いです。
Most of the things that we see computers do, they do because a smart software engineer was able to figure out how to do that.
私たちがコンピュータが行うことのほとんどは、賢いソフトウェアエンジニアがそれをどのように行うかを理解できたからです。
These neural nets, they do stuff that we don't teach them.
これらのニューラルネットは、私たちが教えないことを行います。
They do stuff that we can't even imagine.
私たちが想像できないことを行います。
If you think I'm kidding, take a look at what AlphaFold2 does.
冗談を言っていると思うなら、AlphaFold2が何をするか見てみてください。
And so he's asking, will Sora replace game engine devs?
そして、彼は尋ねています、Soraはゲームエンジンの開発者を置き換えるのか?
Absolutely not.
絶対にそうではありません。
It's emergent physics understanding is fragile and far from perfect.
その新興物理学の理解は壊れやすく、完璧からは程遠いです。
It still heavily hallucinates things that are incompatible with our physical common sense.
それはまだ、私たちの物理的な常識と矛盾するものを幻覚的に見せています。
It does not yet have a good grasp of object interactions.
物体の相互作用をまだうまく把握していません。
See the uncanny mistake in the video below.
以下のビデオで奇妙な間違いを見てください。
So basically it doesn't break the glass, but notice that, but while the glass doesn't shatter, like everything else is looking great.
基本的にはガラスを壊さないのですが、ガラスが割れないのにもかかわらず、他のすべてが素晴らしく見えることに注意してください。
The physics, the ice cubes, the water, the fluid.
物理学、氷のキューブ、水、流体。
Sora is the GPT-3 moment.
SoraはGPT-3の瞬間です。
Back in 2020, GPT-3 was a pretty bad model that required heavy prop engineering and babysitting.
2020年には、GPT-3は重いプロップエンジニアリングとベビーシッティングが必要なかなり悪いモデルでした。
But it was the first compelling demonstration of in-context learning as an emergent property.
しかし、それはコンテキスト内の学習の説得力のある最初のデモンストレーションでした。
Don't fixate on the imperfections of GPT-3.
GPT-3の欠点に固執しないでください。
Think about extrapolations to GPT-4 in the near future.
近い将来のGPT-4への推測について考えてみてください。
All right, with that said, let's quickly go over the technical report that OpenAI posted for Sora.
それでは、OpenAIがSoraに投稿した技術レポートをさっと見てみましょう。
So we explore large scale training of generative models on video data.
私たちはビデオデータでの生成モデルの大規模なトレーニングを探求します。
Specifically, we train text conditional diffusion models.
具体的には、テキスト条件付き拡散モデルをトレーニングします。
So those are the denoising models that create images jointly on videos and images of variable durations, resolutions, and aspect ratios.
これらは、変動する長さ、解像度、アスペクト比のビデオと画像を共同で作成するノイズ除去モデルです。
We leverage a transformer architecture.
私たちはトランスフォーマーアーキテクチャを活用しています。
So this is the big thing that probably drives a lot of the AI progress today.
これがおそらく今日のAIの進歩の多くを推進している大きな要素です。
So that was made in 2017 by Google.
それは2017年にGoogleによって作成されました。
A lot of smart researchers that created that.
それを作成した多くの優れた研究者たちがいます。
And it's really pushed the whole field forward, you could say.
そして、それは全体の分野を前進させました、と言えるでしょう。
And it operates on space-time patches of video and image latent codes.
そして、それはビデオと画像の潜在的なコードのスペースタイムパッチで動作します。
Our largest model, Sora, is capable of generating a minute of high fidelity video.
私たちの最大のモデル、Soraは、高品質のビデオを1分生成することができます。
So this is, as far as I can tell, I mean, before this, I personally have not seen any AI model capable of doing a coherent one minute long scene.
これは、私が見た限りでは、これまでに一分間の連続したシーンを行うことができるAIモデルを個人的に見たことがありません。
The lady walking in Tokyo, I mean, this to me was, I haven't seen anything like this.
東京を歩く女性、これは私にとっては、これまでに見たことがないものでした。
The fact that it's, for one whole minute, stayed coherent, nobody like floated up into the sky.
1分間もちゃんとした状態を保っているという事実は、誰もが空に浮かぶことはなかったということです。
This building didn't morph into five different buildings over time.
この建物は時間の経過とともに5つの異なる建物に変形しませんでした。
Like you can tell she's on the same street.
彼女が同じ通りにいることがわかります。
It's the same people, it's the same building.
同じ人々で、同じ建物です。
Even the text doesn't morph.
テキストさえも変形しません。
I'm kind of gushing about it, but it's a big deal.
私はそれについてちょっと感激していますが、それは大きな進歩です。
And they're saying, our results suggest that scaling video generation models is a promising path towards building general purpose simulators of the physical world.
そして、彼らは、「私たちの結果は、ビデオ生成モデルのスケーリングが物理世界の一般的なシミュレータを構築するための有望な道であることを示唆しています」と述べています。
So they start by saying, turning visual data into patches.
彼らは、視覚データをパッチに変換することから始めます。
Patches is kind of a new term here that they explain.
こちらで説明されているのは、パッチという新しい用語です。
We take inspiration from larger language models, which acquire generalist capabilities by training on internet scale data.
私たちは、インターネットスケールのデータを学習することで、一般的な能力を獲得する大規模な言語モデルからインスピレーションを得ています。
So like ChatGPT, GPT-4, just sucks up all the data on the internet, various books, et cetera.
ChatGPTやGPT-4は、インターネット上のすべてのデータ、さまざまな書籍などを吸収しています。
And then it acquires generalist abilities.
そして、それは一般的な能力を獲得します。
It's able to do a lot of different things well.
それは多くの異なることをうまく行うことができます。
And the success of this Large Language Model paradigm, so GPT-4, all the breakthroughs that it did, this is enabled in part by the use of tokens that elegantly unify diverse modalities of text, code, math, and various natural languages.
この大規模言語モデルのパラダイムの成功、つまりGPT-4、それが行ったすべてのブレークスルーは、テキスト、コード、数学、およびさまざまな自然言語の多様なモダリティを優雅に統一するトークンの使用によって部分的に可能にされています。
So you can think of tokens, I guess, it's kind of like letters.
トークンは、おそらく、文字のようなものだと考えることができます。
It's a unit, just like we break words up into letters.
それは、単語を文字に分割するのと同じように、単位です。
They're kind of like a unit of a word.
それらは、単語の単位のようなものです。
They're similar in that they break up all the text into these tokens, into units that the LLMs are able to understand.
それらは、すべてのテキストをこれらのトークン、大規模言語モデルが理解できる単位に分割するという点で似ています。
And so here they're connecting the idea of tokens to models of visual data.
そしてここでは、彼らはトークンのアイデアを視覚データのモデルにつなげています。
How can they inherit the benefits that we've had with these GPT-4, et cetera?
これらのGPT-4などで得た利点をどのように受け継ぐことができるのでしょうか?
And so where LLMs have text tokens, Sora has visual patches.
大規模言語モデルがテキストトークンを持っているのに対して、Soraはビジュアルパッチを持っています。
So tokens basically are patches in the visual models.
トークンは基本的にビジュアルモデルのパッチです。
Patches have previously been shown to be an effective representation for models of visual data.
パッチは以前、ビジュアルデータのモデルの効果的な表現であることが示されています。
We find that patches are a highly scalable and effective representation for training generative models on diverse types of videos and images.
パッチは、さまざまな種類のビデオや画像の生成モデルのトレーニングに非常にスケーラブルで効果的な表現であることがわかります。
So they take a bunch of images encoded into these patches, and they're able to produce outputs.
彼らはこれらのパッチにエンコードされた画像の束を取り、出力を生成することができます。
Video compression network, we train a network that reduces the dimensionality of visual data.
ビデオ圧縮ネットワークでは、視覚データの次元削減を行うネットワークをトレーニングします。
This network takes raw video as input and outputs a latent representation that is compressed both temporally and spatially.
このネットワークは、生のビデオを入力として受け取り、時間的にも空間的にも圧縮された潜在表現を出力します。
So when you hear this idea of like a latent space, you can think of it as sort of a space where the model does its thinking and processing that is not directly observable.
潜在空間という考え方を聞いたとき、モデルが考えや処理を行う空間として、直接観察されない空間と考えることができます。
So if you have a library full of books,
本棚がいっぱいの図書館があるとします。
And then you have like a little database that has all the books and the titles and what they're about, you can think of that as like the latent space that represents the library.
そして、本とそのタイトル、内容がすべて記載された小さなデータベースがあるとします。それを図書館を表す潜在空間と考えることができます。
And the library has actually all the books and all the text, and the database is kind of just a representation of it.
図書館には実際にすべての本とすべてのテキストがあり、データベースはそれを表すものに過ぎません。
And compressed temporarily and spatially, temporarily just means time.
そして、時間的にも空間的にも圧縮され、時間的には時間を意味します。
So an hour long video is kind of compressed to be shorter.
1時間のビデオが短く圧縮されるということです。
And so Sora is trained on and subsequently generates videos within this compressed latent space.
そして、Soraはこの圧縮された潜在空間でトレーニングされ、その後ビデオを生成します。
I think it's fair to think of latent space as similar to what humans have with like abstract ideas, or if you ever have some intuition about something that you can't fully put into words, that idea exists somewhere, but it might take you a while to like fully extract it into something that another human being might understand.
潜在空間を、人間が抽象的なアイデアを持つのに似ていると考えるのは妥当だと思います。また、何かについて直感を持っているが完全に言葉にできない場合、そのアイデアはどこかに存在しているが、他の人が理解できるように完全に抽出するのに時間がかかるかもしれません。
And so these patches that they're talking about, it makes the output much more flexible.
そして、彼らが話しているこれらのパッチは、出力をより柔軟にします。
So it looks like they can control the size of the generated videos.
生成されたビデオのサイズを制御できるようです。
It's not limited by an aspect ratio or anything like that.
アスペクト比などで制限されることはありません。
And they're pointing out here that Sora is a diffusion transformer, and transformers have demonstrated remarkable scaling properties across a variety of domains, including of course, language modeling, computer vision, image generation.
ここで指摘されているのは、Soraが拡散トランスフォーマーであり、トランスフォーマーは言語モデリング、コンピュータビジョン、画像生成など、さまざまな領域で顕著なスケーリング特性を示してきたということです。
And that's why this picture is kind of interesting because as we increase the compute, how much sort of resources we give this thing, it gets better and better and better with no additional changes.
そして、これが面白いのは、計算を増やすと、このものにどれだけのリソースを与えるかに応じて、追加の変更なしでどんどん良くなっていくということです。
This basically means that a lot of improvement can be had just with kind of better hardware, with more hardware.
これは基本的に、より良いハードウェア、より多くのハードウェアを使うだけで、多くの改善が得られるということを意味します。
I think that's part of the reason why Sam Altman's talking about seven trillion funding for his AI chip company, because sometimes that's pretty much just all you need.
これが、サム・アルトマンがAIチップ会社に7兆ドルの資金を投入することを話している理由の一部だと思います。ときにそれが必要なのは、それだけだからです。
And they're able to rapidly create quick prototype content in lower resolutions and generate full resolution all within the same model.
そして、彼らは低解像度で迅速にプロトタイプコンテンツを作成し、同じモデル内で全解像度を生成することができます。
Instead of having one model generate the low res and then another that kind of like turns into high resolution, it's all within the same model.
低解像度を生成するモデルと、それを高解像度に変換するような別のモデルを持つ代わりに、すべて同じモデル内で行われます。
And they noticed the improved framing and composition in these models, which again, I'm curious how much of that, if the idea that they've used the Unreal Engine in the Unreal Engine, like a video game, you can slightly shift the angle of the cameras just a little bit left and right, up and down, to maybe give the model just a better understanding of how zooming in and out, how framing works.
そして、これらのモデルでフレーミングと構成が改善されたことに気付きました。再び、私はそれのどれくらいが、彼らがアンリアルエンジンを使用しているというアイデアが、カメラの角度をわずかに左右、上下に少し変えることができるビデオゲームのようなUnreal Engineで、モデルにズームインとズームアウト、フレーミングの仕組みをより良く理解させるためのものか、興味を持っています。
They'll be curious to see if that's how they solved it.
彼らはそれがどのように解決されたかを見るのが楽しみです。
And again, so language understanding.
そして、再び、言語理解です。
So they're saying that they use the same recapturing technique that they use in DALL·E 3, because these models require a large amount of videos of corresponding text captions.
これらのモデルは大量の対応するテキストキャプションのビデオを必要とするため、DALL·E 3で使用されている再捉技術と同じものを使用していると言っています。
And notice what they say here.
ここで彼らが言っていることに注意してください。
We first train a highly descriptive captioner model.
まず、非常に記述的なキャプションモデルを訓練します。
And then use it to produce text captions for all the videos in our training set.
そして、それを使用して、トレーニングセット内のすべてのビデオにテキストキャプションを生成します。
We find that training on highly descriptive video captions improves text fidelity, as well as the overall quality of the videos.
非常に記述的なビデオキャプションでトレーニングすると、テキストの忠実度が向上し、ビデオの全体的な品質も向上することがわかります。
So I don't know.
私はわかりません。
I'm reading this as they use GPT-4 revision, maybe slightly fine-tuned, to caption a boatload of these Unreal Engine videos.
私は、彼らがGPT-4改訂版を使用していると読んでいますが、多少微調整されているかもしれません。これらのUnreal Engineビデオにキャプションを付けるために。
Again, we don't know if this is the case or not.
もう一度言いますが、これが事実かどうかはわかりません。
Obviously, nowhere in there do they say Unreal Engine, but assuming it's true, they use something like GPT-4 revision to caption every single video, and then feed that into this model.
明らかに、そこにはUnreal Engineとは書かれていませんが、それが真実であると仮定すると、GPT-4改訂版のようなものを使用して、すべてのビデオにキャプションを付け、それをこのモデルに送り込むと思われます。
And the whole point of that is you just have unlimited high-quality data for training the model.
その全体のポイントは、モデルのトレーニングに無制限の高品質データがあるということです。
You can create images of anything, people, spaceships, cities, whatever.
人々、宇宙船、都市など、何でもイメージを作成できます。
You can do realistic, animated, first-person shooter, whatever.
リアルな、アニメーション、ファーストパーソンシューターなど、何でもできます。
The model can probably caption millions of hours of this footage.
そのモデルはおそらく何百万時間もの映像にキャプションを付けることができるでしょう。
Like there's just no bottleneck to producing a staggering amount of very high-quality video, of data.
まるで、非常に高品質なビデオ、データの驚くべき量を生産するためのボトルネックがないようです。
Data that is paired with text, which is the pairs that you need to train these models.
テキストとペアになったデータは、これらのモデルをトレーニングするために必要なペアです。
And Sora can also be prompted with other inputs, such as pre-existing images or videos, create perfectly looping videos, animated static images, et cetera, extending videos forwards or backwards in time, et cetera.
そして、Soraは既存の画像やビデオなど、他の入力でも促されることができ、完璧にループするビデオ、アニメーションされた静止画像などを作成し、ビデオを時間的に前後に延長することもできます。
So you can take a still image and turn it into this or that, or pretty much anything you want.
静止画像を取ってこれやあれに変えたり、ほぼ何でもできます。
This one's pretty cool, I gotta say 'Wow'.
この一つはかなりかっこいいですね、私が言うべきは「わあ」です。
Yeah, to me, notice the lighting and how it handles the 3D space up above in the cathedral.
そうですね、私にとっては、大聖堂の上の照明や3D空間の扱いに注目してください。
Like, I don't know, I'd be hard-pressed to say.
まあ、わからないな、言いにくいと思います。
I mean, that looks like a Unreal Engine sort of how it would render the 3D space of a building.
建物の3D空間をレンダリングする際に、それはまるでUnreal Engineのように見えますね。
It does not look like any of the other AI video generation things that I've seen.
他のAIビデオ生成のものとは全く違うように見えません。
And the lighting too seems like actual lighting.
そして、ライティングも実際の照明のように見えます。
It doesn't seem like just texture.
ただのテクスチャではないようですね。
It looks like it's a light source.
それは光源のように見えます。
Now take a look at this video.
さて、このビデオをご覧ください。
I had half a mind to just have it loop infinitely and not say anything, but I won't do that.
無限ループにして何も言わずに放置しようかと思いましたが、やめます。
So apparently you can also use Sora to produce an infinite loop, a seamless infinite loop by just looping the entire few seconds together.
Soraを使って数秒間をループさせることで、シームレスな無限ループを作成することもできるようです。
You can extend the video forwards and backwards and produce an infinite loop.
ビデオを前後に延長して無限ループを作成することができます。
You can take two different sort of sets of images and kind of combine them.
異なる種類の画像の2つを取り、それらを組み合わせることができます。
So you see a butterfly underwater, or at one point it's gonna be a drone, or it's gonna be a butterfly flying like a drone through the Colosseum.
水中で蝶を見ることができ、ある時点ではドローンになったり、コロッセウムを飛ぶように蝶がドローンのようになったりします。
There it is.
それがそれです。
Now here you're combining, was that a chameleon, a gecko with a bird and you got this bird-like chameleon, chameleon bird, a very unique look.
ここでは、カメレオンと鳥を組み合わせて、鳥のようなカメレオン、カメレオンのような鳥を作り出しています。非常にユニークな外見です。
I gotta say, I mean, it really captures kind of the essence of both.
本当に、両方の本質を捉えていると言わざるを得ません。
You can generate images and here's where it gets interesting, emerging simulation capabilities.
画像を生成することができ、ここで興味深いのは、新たなシミュレーション能力が現れるところです。
We find that video models exhibit a number of interesting emerging capabilities when trained at scale.
我々は、規模で訓練されたビデオモデルがいくつかの興味深い新しい能力を示すことを発見しています。
These capabilities enable Sora to simulate some aspects of people, animals, and environments from the physical world.
これらの能力により、Soraは物理世界から人々、動物、環境の一部をシミュレートすることができます。
These properties emerge without any explicit inductive biases for 3D, objects, et cetera.
これらの特性は、3D、オブジェクトなどの明示的な帰納バイアスなしに現れます。
They are purely phenomena of scale.
それらは純粋に規模の現象です。
And as I mentioned in the previous video, and this is kind of what we're talking about here with the emergent properties, this is really where I would love to see much, much more research.
そして、前のビデオで言及したように、これが新興特性に関する私たちが話している内容ですが、これは本当にもっと多くの研究を見たいところです。
Like what is happening in those neural nets that is making this real and how far can we take this?
これが実際に何をしているのか、そしてこれをどこまで進めることができるのか、ということです。
And they continue 3D consistency, Sora can generate videos of dynamic camera motion as the camera shifts and rotates people and scene elements move consistently through three dimensional space.
そして、3Dの一貫性を続け、Soraは、カメラが移動し、回転するときに、人々やシーンの要素が3次元空間を通じて一貫して移動するビデオを生成することができます。
Long range coherence and object permanence, this is huge.
長期の一貫性と物体の恒久性、これは非常に重要です。
This is one of the most striking things about how well they're able to keep the coherence in these images over long periods of time or as the camera shifts around.
これは、これらの画像の一貫性を長期間保つ能力について、カメラが移動するかぎり、どれほどうまくやっているかについて、最も印象的なことの1つです。
This is unlike anything I've seen.
これは私が見た中で他に類を見ないものです。
And interacting the world, so it can simulate actions that affect the state of the world in simple ways.
そして、世界との相互作用、つまり、単純な方法で世界の状態に影響を与えるアクションをシミュレートすることができます。
So like watching somebody draw, that's pretty incredible.
誰かが描くのを見るようなこと、それはかなり信じられないことです。
And it's gonna be able to simulate artificial processes like in video games, it's able to simulate digital worlds.
そして、ビデオゲームのような人工プロセスをシミュレートすることができ、デジタルワールドをシミュレートすることができます。
So it's that idea of simulating Minecraft as we've mentioned earlier.
私たちが以前に言及したように、Minecraftをシミュレートするというアイデアです。
I mean, it looks so close.
つまり、それは非常に近いように見えます。
I mean, everything 3D is like simulated perfectly as you move around, it's very, very similar.
私の意味することは、周りを動き回ると、すべての3Dが完璧にシミュレートされているようなもので、非常に非常に似ています。
And these capabilities suggest that continued scaling of these video models is a promising path towards the development of highly capable simulators of the physical and digital world and the objects, animals and people that live within them.
そして、これらの能力は、これらのビデオモデルの継続的なスケーリングが、物理的およびデジタル世界、およびそれらに住むオブジェクト、動物、人々の高性能シミュレーターの開発に向けた有望な道であることを示唆しています。
And I'll leave you with this final post by Dr. Jim Fan.
そして、最後にジム・ファン博士によるこの最終的な投稿をお伝えします。
None of this is meant to be a religious or spiritual or anything like that.
これらのいずれも宗教的または精神的なものを意図したものではありません。
It is just sort of a thought experiment.
それは単なる思考実験です。
But think about this, if there's a higher being who writes the simulation code for our reality, we can estimate the file size of the compiled binary.
しかし、考えてみてください、もし私たちの現実のシミュレーションコードを書く高次な存在がいるとしたら、コンパイルされたバイナリのファイルサイズを推定することができます。
So basically when you write code, when you write it out, it can be understood by humans, it's long, it kind of spells everything out.
基本的に、コードを書くとき、それを書き出すと、人間に理解されるようになります、長く、すべてをスペルアウトします。
And then when you compile it, it gets turned into a smaller file that the computer can just execute and run directly.
そして、それをコンパイルすると、コンピュータが直接実行して実行できるようになるより小さなファイルに変換されます。
It's smaller, faster, it's just everything that it needs to run to do what it's supposed to do.
それはより小さく、速く、それがやるべきことを実行するために必要なすべてです。
So he's saying if Meta AI's videos, this many parameters and Sora is this, then the creator's binary, the sort of the person that built the simulation, which is our lives, might be no larger than 111 gigabytes, which is interesting to think that our world could be reduced to some tech spec like that, some technological specification.
Meta AIのビデオがこれだけのパラメータを持ち、Soraがこれだけであるならば、クリエイターのバイナリ、つまり私たちの生活であるシミュレーションを構築した人物のものは、111ギガバイトよりも大きくないかもしれないと言っています。それは私たちの世界がそのような技術仕様に簡略化される可能性があると考えることは興味深いです。
And he's saying Sora is not just compressing our world, but all possible worlds.
そして、彼はSoraが私たちの世界だけでなく、すべての可能な世界を圧縮していると言っています。
Our reality is the only one of the simulations that Sora is able to compute.
私たちの現実は、Soraが計算できるシミュレーションの中で唯一のものです。
It's possible that some parts of the physical world doesn't exist until you look at it, much like you don't need to render every atom in the Unreal 5 and the Unreal Engine 5 to make it a realistic scene.
物理世界の一部があなたがそれを見るまで存在しない可能性があるというのは、Unreal Engine 5のすべての原子をレンダリングする必要がないように、現実的なシーンにするためには必要ありません。
By the way, this idea that it's possible that some parts of our universe, our physical world, that they don't exist until you look at it, does that sound like nonsense to you?
ちなみに、私たちの宇宙、物理世界の一部があなたがそれを見るまで存在しない可能性があるというこの考えは、あなたにとって無意味に聞こえますか?
Because it is true, this is literally true.
なぜなら、これは真実であり、文字通り真実です。
You know how some people act differently if they're aware that somebody's watching them?
あなたは、誰かが自分を見ていることを知っていると、一部の人々が異なる行動を取る方法を知っていますか?
Well, so does light.
光も同じようにそうします。
Why?
なぜですか?
I have no idea, but I've said this before, I feel like AI is gonna unravel some of the strangest mysteries in our universe.
私には全くわかりませんが、以前にも言ったように、AIが私たちの宇宙の中で最も奇妙な謎のいくつかを解き明かすだろうと感じています。
My name's Wes Roth and thank you for watching.
私の名前はウェス・ロスです。ご視聴ありがとうございました。
この記事が気に入ったらサポートをしてみませんか?