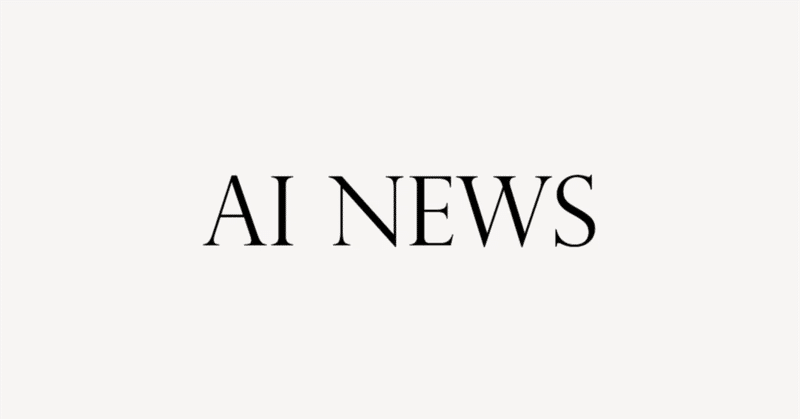
【2024年はロボティクスとAIの飛躍の年】英語解説を日本語で読む【2024年2月24日|@Wes Roth】
2024年はロボティクス、ゲームAI、シミュレーションの進化が特に注目される年となりそうです。OpenAI、Google/DeepMind、NVIDIAなどの企業から、ロボット技術とAI分野における重大な進展が報告されています。これにより、エネルギー問題の解決、宇宙探査の進化、資源の利用方法の変化など、社会全体に大きな影響を与える可能性があります。また、AIのトレーニングと透明性に関する議論も活発化しており、テクノロジーの倫理的側面への関心が高まっています。具体的には、Googleの画像生成AI「Gemini」が引き起こした議論や、AIによる新しい薬の開発などが注目されています。
公開日:2024年2月24日
※動画を再生してから読むのがオススメです。
You imagine you crack fusion with it or room temperature superconductors and batteries that are optimal.
それを使って核融合や常温超伝導体、最適なバッテリーを開発することを想像しています。
That opens up, suddenly energy becomes free or cheap, then that has huge consequences on resources, like freeing up.
それによって、突然エネルギーが無料や安価になり、それが資源に大きな影響を与える可能性があります。
Like you could do more space travel, mine asteroids maybe becomes feasible, all of these things.
宇宙旅行をより多く行ったり、恐らく小惑星を採掘することが可能になるかもしれません、全てのこれらのことが。
And then suddenly the nature of money even changes.
そして、突然お金の性質さえも変わるかもしれません。
So I'm not sure people are really understanding.
人々が本当に理解しているかどうかはわかりません。
I don't know if company constructs would even be the right thing to think about at that point.
その時点で企業構造を考えるのが適切なことなのかさえわかりません。
So the OpenAI-backed robot is gaining some traction.
OpenAI支援のロボットはいくらかの注目を集めています。
CEO and founder Bernt Bornich says new progress update on the droids dropping in four weeks.
CEO兼創設者のベルント・ボルニヒは、4週間後にドロイドの新しい進捗状況の更新を発表すると述べています。
Looks like Moravec's paradox might be false and we just didn't have the data.
モラベックのパラドックスは誤りであり、単にデータが不足していただけかもしれません。
What does that mean?
それはどういう意味ですか?
We'll dive into that in just a second.
ちょっとした時間でそれに取り組みます。
Also Ted Xiao, who's working on robotics for Google/DeepMind, says there will be three to four massive news coming out in the next weeks that will rock the robotics and AI space.
また、Google/DeepMindのロボティクスに取り組んでいるテッド・シャオは、次の数週間で3〜4つの重大なニュースが出ると述べており、それがロボティクスとAIの分野を揺るがすだろうと言っています。
Adjust your timelines, it'll be a crazy 2024.
タイムラインを調整してください、2024年は狂った年になるでしょう。
I have a feeling it will shock the entire industry in fact.
実際、それが業界全体を驚かせると感じています。
Just a hunch.
ただの予感です。
Dr. Jim Fan drops a career update.
ジム・ファン博士がキャリアの最新情報を発表しました。
He's founding a new group called GEAR, which stands for Generalist Embodied Agent Research.
彼はGEARという新しいグループを設立しており、それはGeneralist Embodied Agent Researchの略です。
2024 is the year of robotics, the year of gaming AI and the year of simulation.
2024年はロボティクスの年、ゲームAIの年、シミュレーションの年です。
He finally also got recognized for something that for some reason I feel like a lot of people have been missing.
彼は何かでようやく認められ、何故か多くの人々が見逃していたような気がします。
I did multiple videos on it.
私はそれに関する複数のビデオを作成しました。
It's just not getting the traction that it should be.
それは本来なるべきトラクションを得ていないだけです。
But people are finally waking up.
しかし、人々はついに目を覚ましています。
We'll look at that in just a second.
ちょっと待って、それを見てみましょう。
Also OpenAI drops some massive news about the GPT store.
また、OpenAIがGPTストアに関する重大なニュースを発表しました。
But first I have to touch on the whole Google fiasco.
しかし、まず最初にGoogleの問題に触れなければなりません。
I didn't put out a video on the day that it was happening, but Google has been changing some prompts to be different than what users want them to be.
その日にその出来事についてのビデオを出していませんが、Googleはユーザーが望むものと異なるプロンプトを変更しています。
I mean here are the images of the pope, of the founding fathers, image of a viking, image of an American woman, image of a British woman, image of a medieval knight, an Australian woman and here's their rendition of chess.
つまり、教皇の画像、開国の父たちの画像、バイキングの画像、アメリカの女性の画像、イギリスの女性の画像、中世の騎士の画像、オーストラリアの女性の画像、そしてこちらが彼らのチェスの描写です。
That last one was a joke obviously, but here's Demis Hassabis.
最後のものは明らかに冗談でしたが、こちらがデミス・ハサビスです。
So he's the founder of DeepMind, which is part of the Alphabet Company.
彼はDeepMindの創設者であり、それはAlphabet Companyの一部です。
So the same kind of umbrella that Google is under.
つまり、Googleが所属する同じ種類の傘の下にあるということです。
Not directly part of Google, but it looks like they're merging more and more.
直接的にはGoogleの一部ではありませんが、ますます統合されているようです。
He just went on an air view and explained kind of what was happening there behind the scenes with these image generation.
彼はちょうど空中視点に移り、裏側で何が起こっているのかを説明しました。
So we'll take a look at that as well.
それも見てみましょう。
So this person that made all their tweets private, I believe this was Jack Krausik, who is a Google employee, who is the Senior Director of Product for Gemini Experiences.
全てのツイートを非公開にしたこの人物は、おそらくGoogleの従業員であり、Gemini Experiencesの製品のシニアディレクターであるジャック・クラウジックだと思います。
So he's likely behind a lot of the decisions that kind of went into this.
彼はこれに関わる多くの決定の背後にいる可能性があります。
He was responding to the backlash by saying, well no it's not true, that's not happening.
彼は、その批判に対して、いや、それは真実ではない、それは起こっていないと言っていました。
Here's the issue with that.
それに関する問題はこちらです。
This person I think nailed it perfectly.
この人物は完璧にそれを突き止めたと思います。
They're saying the actual problem is the lack of transparency of what you do with the prompts and how you're manipulating the output.
彼らは、実際の問題はプロンプトをどのように扱い、出力をどのように操作しているかの透明性の欠如だと言っています。
Just be transparent and none of the backlash will occur.
ただ透明性を持ち、批判は起こらないでしょう。
I understand that the intention is good, but if the ethnically ambiguous gets added to a prompt, please let the users know that instead of gaslighting them.
意図は良いと理解していますが、民族的に曖昧なものがプロンプトに追加される場合は、ユーザーにガスライティングするのではなく、それを知らせてください。
David Shapiro posted this and I think it really also cuts to the heart of the matter.
デイビッド・シャピロがこれを投稿しましたが、これは問題の核心にも触れていると思います。
And here's what he's saying.
そして、彼が言っていることはこちらです。
One, user consent matters.
ユーザーの同意は重要です。
Hijacking your intentions as a user of any technology is wrong.
どんな技術のユーザーとしても、あなたの意図を乗っ取ることは間違っています。
Enforcing a particular worldview on users is flatly unethical.
ユーザーに特定の世界観を押し付けることは、まったく倫理的ではありません。
Yes, racism is bad, but cramming one for-profits company's view of morality down the user's throats is dystopian AF and I think that dystopian AF is an excellent way of putting it.
はい、人種差別は悪いことですが、利益を追求する一企業の道徳観をユーザーに強制することはディストピアそのものですし、その状況を「ディストピアそのもの」と表現するのは非常に適切だと思います。
Corporations setting themselves up as arbiters of morality is flat out cyberpunk.
企業が自らを道徳の裁定者として設定することは、まったくサイバーパンクです。
Morality and ethics is up to the people, not the corporations and not the government.
道徳と倫理は人々によって決まるものであり、企業や政府ではありません。
It looks like we have overwhelmingly people agree.
圧倒的多数の人々が同意しているようです。
Looks like almost 90 percent agree strongly or mildly.
ほぼ90%の人が強く賛成またはやや賛成しているようです。
There's been some people noticing online this week that Gemini doesn't seem to create a white man if you ask it to or if you try to depict figures from history.
今週、オンラインでGeminiが白人男性を作成しないように見えると気づいた人がいました。また、歴史上の人物を描こうとするとそうならないようです。
It sort of doesn't want to do that or we'll sort of do it in a historically inaccurate way.
それはある種の理由でそうしたくないのか、歴史的に正確でない方法で行うのかもしれません。
I think this is a good example of these nuances.
これはこれらの微妙な違いの良い例だと思います。
The historical accuracy, absolutely we want that.
歴史的な正確さは、絶対に求めているものです。
So we need to fix that versus when you have a generic prompt, obviously then these things are universal.
それを修正する必要があると思います。一方、一般的なプロンプトがある場合は、これらのことは普遍的です。
So for example, if you said create a picture of a person walking a dog, you'd want a universal depiction.
例えば、犬を散歩している人の絵を作成するように言った場合、普遍的な描写が欲しいでしょう。
But then others are historical events or historical figures, then perhaps that should be narrower.
しかし、他の場合は歴史的な出来事や歴史上の人物である場合、もっと狭い方が良いかもしれません。
And as I say, we're continually improving our models based on feedback.
そして、フィードバックに基づいてモデルを継続的に改善しています。
Now here's an interesting take.
さて、興味深い視点があります。
So apparently this person Yishan was once the CEO of Reddit.
おそらくこの人、イーシャンはかつてRedditのCEOだったそうです。
So he's saying Google's Gemini issue is not really about woke/die and everyone who is obsessing over it has failed to notice the much, much bigger problem than it represents.
彼は、GoogleのGeminiの問題は実際には「目を覚ませ/死ね」ということではなく、それにこだわる人たちはそれが表すよりもはるかに大きな問題に気づいていないと言っています。
To recap, Google injected special instructions into Gemini so that when it was asked to draw pictures, it would draw people with diverse or non-white racial backgrounds.
要約すると、GoogleはGeminiに特別な指示を注入しました。それにより、絵を描くように求められたとき、多様な人種的背景を持つ人々を描くようになりました。
This resulted in lots of weird results where people would ask it to draw pictures of people who were historically white, like Vikings or 1940s Germans, and it would output black people or Asians.
これにより、歴史的に白人であったヴァイキングや1940年代のドイツ人などの人々の絵を描くように求められると、黒人やアジア人が出力されるという奇妙な結果がたくさん出ました。
Google originally did this because they didn't want pictures of people doing universal activity, like walking a dog, to always be white, reflecting whatever bias existed in the training set.
Googleはもともと、犬の散歩などの普遍的な活動をする人々の絵が常に白人である必要はなく、トレーニングセットに存在する偏見を反映することを望んでいました。
So this person continues, this is not an unreasonable thing to do given that they have a global audience.
この人は続けて、彼らにはグローバルな観客がいることを考えると、これは理にかなったことではないと言います。
Maybe you don't agree with it, but it's not unreasonable.
あなたはそれに同意しないかもしれませんが、それは理にかなっています。
Google most likely did not anticipate or intend the historical figures who should be reasonably be white result.
Googleはおそらく、歴史的に白人であるべき人物が結果として黒人になることを予期したり意図したりしなかったでしょう。
Now he continues and he talks about the three laws of robotics by Asimov, the zero flaw and some bad things that happen in those books brings up Eliza Yudkovsky.
そして彼は続けて、アシモフのロボット三原則、ゼロ欠陥、そしてそれらの本で起こる悪いことについて話し、エリザ・ユドコフスキーを取り上げます。
We're not going to go down this path quite yet, but his point is that AI can have unpredictable outcomes.
まだこの道を進むつもりはありませんが、彼のポイントは、AIには予測できない結果が生じる可能性があるということです。
What are the cases?
何が問題なのですか?
It brings me back to something like this.
私をこんな気持ちにさせます。
The actual problem is the lack of transparency of what you do with the prompts and how you're manipulating the output.
実際の問題は、プロンプトをどのように扱い、出力をどのように操作しているかの透明性の欠如です。
Just be transparent.
ただ透明であればいいのです。
If the AI is outputting something that doesn't align what you think it should show, if you just start manipulating the user's request without letting them know that you're doing it and not being open about it, whatever noble purpose you thought you had becomes dystopian AF.
AIがあなたが表示すべきだと思うものと一致しないものを出力している場合、ユーザーのリクエストをただ操作し始めて、それを知らせずにオープンにしないでいると、あなたが持っていたと思っていた立派な目的はディストピアになります。
Just don't lie.
ただ嘘をつかないでください。
Don't be evil.
悪いことをしないでください。
As I was finishing up recording this video, this popped up in my feed.
このビデオの録画を終えていたとき、これが私のフィードに現れました。
So this is Google's blog saying Gemini image generation got it wrong.
これはGoogleのブログがGemini画像生成が間違っていたと言っているものです。
We'll do better.
もっとよくやります。
They're saying that it's clear that this feature missed the mark.
それは、この機能が目標を外れていることは明らかだと言っています。
It's inaccurate or even offensive.
それは不正確であり、さらには攻撃的です。
Basically having a diverse group of people, if you're asking for somebody walking the dog.
基本的には、犬の散歩をしている人を求める場合には、多様なグループの人々を持つことになります。
However, if you're asking Gemini for a specific type of person, such as a black teacher in a classroom or a white veterinarian with a dog, or people in particular cultural or historical context, you should absolutely get a response that accurately reflects what you ask for.
ただし、Geminiに特定のタイプの人物を求めている場合、例えば教室の黒人教師や犬を連れた白人獣医師、または特定の文化や歴史的文脈の人々など、正確に求めた内容を反映する回答を絶対に得るべきです。
And so they're saying, so what went wrong?
そして彼らは言っています、では何が間違ってしまったのでしょうか?
In short, two things.
要するに、2つのことです。
First are tuning to ensure that Gemini showed a range of people fail to account for cases that should clearly not show a range.
1つ目は、Geminiがさまざまな人々を表示するように調整することが失敗し、明らかにさまざまな人々を表示すべきでないケースを考慮していませんでした。
And second, over time, the model became way more cautious than we intended and refused to answer certain prompts entirely - wrongly interpreting some very anodyne prompts as sensitive, so some very inoffensive prompts as sensitive.
そして2つ目は、時間が経つにつれて、モデルは我々が意図したよりも遥かに慎重になり、一部の全く無害なプロンプトを敏感なものと誤解し、完全に回答を拒否するようになりました。その結果、非常に無害なプロンプトさえも敏感であると見なされました。
These two things led the model to overcompensate in some cases and be overly conservative in others, leading to images that were embarrassing and wrong.
これらの2つのことが、モデルがいくつかの場合に過剰補正し、他の場合に過度に保守的になる原因となり、恥ずかしくて間違った画像が生じた。
They're saying we did not want Gemini to refuse to create images of any particular group.
彼らは、Geminiが特定のグループの画像を作成することを拒否することを望んでいなかったと言っています。
And also Elon Musk posted this.
そして、イーロン・マスクもこれを投稿しました。
A senior exec at Google called and spoke to me for an hour last night.
Googleのシニアエグゼクティブが昨夜1時間私に電話して話しました。
He assured me that they are taking immediate action to fix the racial and gender bias in Gemini.
彼は、彼らがGeminiの中の人種や性別の偏見を修正するために即座の行動を取っていると私に保証しました。
Time will tell.
時が経てばわかるでしょう。
And I'm kind of with Elon this one.
そして、私はイーロンと同じくらいこの問題に賛成です。
Time will tell.
時が経てばわかるでしょう。
I want to believe that this was an innocent mistake caused by overtightening the commands on the AI model.
私は、これがAIモデルのコマンドを過度に締め付けることによって引き起こされた無実の間違いだと信じたいのです。
But I think all in all, this was a good thing, because it showed to a lot of people why it's important to have transparency with AI, why it's important to understand who is training these models, what their viewpoints are.
しかし、全体的に考えると、これは良いことだと思います。なぜなら、多くの人々にAIの透明性が重要である理由、これらのモデルを訓練している人々が誰なのか、彼らの視点が何であるかを理解することが重要であることを示してくれたからです。
Maybe this was a simple mistake.
もしかしたら、これは単なる間違いだったかもしれません。
But if in the future it gets used for malicious intent, how do we know?
しかし、将来悪意を持って使用される可能性がある場合、どうやってそれを知ることができるでしょうか?
Do we have visibility into that decision process?
その意思決定プロセスに対してどのような可視性がありますか?
So I agree with Musk, your time will tell.
私はマスクに同意します。時間がすべてを教えてくれるでしょう。
Oftentimes the argument against having open source AI is the chance that it will be taken over by bad actors.
オープンソースのAIに反対する議論の多くは、それが悪意ある者によって乗っ取られる可能性があるということです。
What protections do we have against the big closed source centralized AI from being taken over by bad actors?
大手のクローズドソースの中央集権型AIが悪意ある者に乗っ取られるのを防ぐために、どんな保護策がありますか?
In other news, so this is Logan.GPT, the ambassador for OpenAI and AGI.
その他のニュースとして、これがLogan.GPTです。OpenAIとAGIのアンバサダーです。
He's saying great news for GPT builders and users.
彼はGPTビルダーやユーザーにとって素晴らしいニュースだと言っています。
We just launched a big GPT store update with GPT reviews, builder social profiles, a new about page with ratings, categories, number of conversations, etc, etc.
私たちはGPTレビュー、ビルダーのソーシャルプロフィール、評価、カテゴリー、会話の数などを含む新しいページを備えた大規模なGPTストアのアップデートを行いました。
So now you're able to rate GPTs, provide private feedback directly to the builder, and an about section in the ChatGPT store.
今では、GPTsを評価し、ビルダーに直接プライベートフィードバックを提供し、ChatGPTストアの紹介セクションを利用できるようになりました。
Here's Nick Dobos.
こちらがNick Dobosです。
So he created the Grimoire, the number one Coding Wizard GPT.
彼は、Coding Wizard GPTであるGrimoireを作成しました。
So it looks like it's got over 200 reviews.
200以上のレビューがあるようですね。
So this is it right there in the GPT store.
これがGPTストアにあるものです。
It looks like it's saying 1000 plus reviews now.
今、1000以上のレビューがあるようです。
Now I'm curious, are you using GPTs?
今、興味が湧いてきましたが、GPTsを使用していますか?
Are you building something on there?
何かを構築していますか?
When it was first announced and I was looking at it, I thought this was going to be the next big thing, the next big wave.
最初に発表されたとき、私はこれが次の大きな流れ、次の大きな波になると思っていました。
However, I'm not quite sure how much of an impact it has made.
ただ、どれだけの影響を与えているかはまだよくわかりません。
Now it seemed like since then we had a lot more news seemed to indicate that everything is moving much faster now.
今では、その後たくさんのニュースがあり、すべてが今はずっと速く動いているように思われます。
Like this might have been a much bigger deal if AI progress moved slower.
もしそれが進歩が遅かったら、これはもっと大きな問題だったかもしれません。
But with the way things are going, this was just maybe this was just a much smaller thing that we first anticipated.
しかし、事が進んでいるように、これはたぶん最初に予想していたよりもずっと小さなことだったかもしれません。
Or is it heating up now because there's more ratings and potentially people starting to be paid for how many users use their GPTs?
それとも、もっと評価が高くなっているのは、GPTsを利用するユーザーが増えているためで、潜在的に人々が報酬を受け取り始めているからですか?
I'm not sure.
よくわかりません。
Let me know in the comments what you think.
コメントであなたがどう思うか教えてください。
Are you excited about GPTs still?
GPTsにまだ興奮していますか?
Did you completely forget that they exist?
彼らが存在することを完全に忘れてしまいましたか?
I'd be curious to know.
知りたいと思います。
Now the other big news here is robotics.
そして、もう1つの大きなニュースはロボティクスです。
So Dr. Jim Phan is announcing that he's launching gear.
ジム・ファン博士は、彼がギアを立ち上げることを発表しています。
Generalist embodied agent research.
総合的な具現化エージェントの研究。
So this is kind of under the NVIDIA umbrella.
これは、NVIDIAの傘下にあるということです。
We believe in a future where every machine that moves will be autonomous and robots and simulated agents will be as ubiquitous as iPhones.
私たちは、すべての動く機械が自律し、ロボットやシミュレートされたエージェントがiPhoneと同じくらい普及する未来を信じています。
We are building the foundation agent, a generally capable AI that learns to act skillfully in many worlds, virtual and real.
私たちは、基礎エージェントを構築しています。多くの世界、仮想的で現実の世界で巧みに行動することを学ぶ一般的に能力のあるAIです。
2024 is the year of robotics, the year of gaming AI, and the year of simulation.
2024年は、ロボティクスの年、ゲームAIの年、シミュレーションの年です。
We are setting out on a moon landing mission and getting there will spin off mountains of learnings and breakthroughs.
私たちは月面着陸ミッションに乗り出し、そこに到達することで多くの学びと突破口が生まれるでしょう。
So this is the page on NVIDIA.
これは、NVIDIAのページです。
So this is the other person that's going to be on the team.
これは、チームに加わる他の人物です。
One of the main people on there.
そこにいる主要な人物の1人。
It seems like I believe he was working with Dr. Jim Fan on Voyager, the Minecraft AI, as well as on the Eureka paper, which we'll touch on in just a minute here.
おそらく、私は彼がVoyager、Minecraft AIでジム・ファン博士と一緒に働いていたと信じています。また、Eureka論文についてもすぐに触れます。
And so this NVIDIA GEAR team will be led by the two of them.
そして、このNVIDIA GEARチームは彼ら2人によって率いられることになります。
And the mission is to build these foundation models, multimodal foundation models, LLMs for planning and reasoning, vision language models, and world models trained on internet scale data sources.
そして、その使命は、これらの基礎モデル、多様な基礎モデル、計画と推論のための大規模言語モデル、ビジョン言語モデル、そしてインターネット規模のデータソースで訓練されたワールドモデルを構築することです。
We also have general purpose robots, robotic models and systems that enable robust locomotion and dexterous manipulation in complex environments, foundation agents in virtual worlds, these Large Action Models that autonomously explore and continuously bootstrap their capabilities across different games and simulations, simulations and synthetic data.
また、一般的な用途のロボット、複雑な環境での頑健な移動と器用な操作を可能にするロボットモデルとシステム、仮想世界の基礎エージェント、これらの大規模なアクションモデルがあります。これらは異なるゲームやシミュレーション間で自律的に探索し、能力を継続的にブートストラップするものです。
And this is the recent thing that we've been talking about with things like Sora emerging simulation infrastructure and synthetic data pipelines for large scale learning, and they're hiring.
そして、これは私たちが最近話していることで、Soraのような新しいシミュレーションインフラや、大規模学習のための合成データパイプラインなどが出現しており、彼らは採用を行っています。
So I gotta say, this is, this would be a very exciting opportunity in part because as Dr. Jim Fan says, NVIDIA has enough capital to solve robotic foundation model, gaming foundation model, and generative simulation all at once.
これは非常に興奮する機会であると言わざるを得ません。なぜなら、ジム・ファン博士が言うように、NVIDIAはロボットの基礎モデル、ゲームの基礎モデル、そして生成シミュレーションを一度に解決する資本を持っているからです。
He's saying our new group is perhaps the most well funded embodied AI lab on earth.
彼は、私たちの新しいグループはおそらく地球上で最も資金力のある具現化AI研究所かもしれないと言っています。
So I would not be surprised if NVIDIA indeed is the company that's going to produce a lot of these breakthroughs.
NVIDIAが確かにこれらの多くのブレークスルーを生み出す企業であると驚かないでしょう。
And also Eureka was named the top 10 NVIDIA research projects of 2023.
また、Eurekaは2023年のNVIDIA研究プロジェクトトップ10に選ばれました。
We've covered it on this channel.
私たちはこのチャンネルでそれについて取り上げてきました。
It was quite exciting.
それはかなり興奮するものでした。
They taught a robot in a simulation to twirl a pen and its fingers, similar to how you used to have kids in school kind of do that if you recall, which was just an insanely hard problem to solve previously.
彼らはシミュレーションでロボットにペンを回し、指を動かす方法を教えました。学校で子供たちがやっていたような、以前は解決が非常に難しい問題でした。
But it gets even better than that.
しかし、それ以上のことが起こります。
In order to train these robots in simulation, there's something that's called a reward function.
これらのロボットをシミュレーションで訓練するために、報酬関数と呼ばれるものがあります。
So basically, if you're training a robot to walk, have some sort of a walking animation to get from one side to the other, you might give it rewards for, for example moving forward some distance, you might give some penalties for falling out its face.
ロボットに歩くように訓練する場合、片側からもう片側に移動するための歩行アニメーションを持っているかもしれません。例えば、前に進んだ距離に対して報酬を与え、顔から落ちた場合にはペナルティを与えるかもしれません。
Whatever the case is, you write out an equation that gets at a attaboy every time you do something right.
どのような場合でも、正しいことをしたたびに「よくできました」という評価を得る方程式を書き出すことができます。
And they don't do that again, if it does something wrong, similar to some of the things we used to perhaps train animals, reinforcement learning give them a little treat when the dog sits down on command, something like that.
そして、何か間違ったことをした場合には、もう一度それを行わないようにします。犬が命令に従って座ったときに少しのご褒美を与えるような、動物を訓練する際に行っていたことに似ています。
Now we humans are pretty good at writing those reward functions up to a point.
今、私たち人間は、その報酬関数を書くのがかなり得意ですが、ある程度までです。
We're good at writing them when the thing that we're trying to get the robot to do is pretty simple, like balance something or run across the screen or open a door or drawer or something like that.
私たちは、ロボットにバランスを取ったり、画面を横切ったり、ドアや引き出しを開けたりするような、かなり単純なことをさせようとする場合には得意です。
But we're not really good when it comes to doing something that is more complex, like spinning a pen in its fingers.
しかし、ペンを指で回すなど、より複雑なことをさせる場合には、本当に得意ではありません。
So Eureka tried something new and said we humans were not that good at writing this code, so you, ChatGPT, GPT-4, you do it. And we told GPT-4 what to do, the task description that we're trying to achieve to make the shadow hand spin the pen to a target orientation.
そこでEurekaは新しいことを試み、「我々人間はこのコードを書くのがそれほど得意ではない」と述べ、あなた、ChatGPT、GPT-4にその任務を任せました。そして、我々はGPT-4に何をすべきか、つまり目標の向きにペンを回転させるために達成しようとしているタスクの説明を伝えました。
Then we gave it the environment code, sort of like the code behind this simulation.
それから、私たちはそれに環境コードを与えました。このシミュレーションの背後にあるようなコードです。
And so GPT-4 wrote out various samples, different sort of versions, different approaches to doing this.
そして、GPT-4はこれを行うためのさまざまなサンプル、異なる種類のバージョン、異なるアプローチを書き出しました。
And that was fed into the Isaac Gym, the simulation.
そして、それはIsaac Gym、つまりシミュレーションに供給されました。
The results of that was taken out of the simulation and given again to that same GPT-4 with feedback and said, here's what you told us to do.
その結果はシミュレーションから取り出され、再び同じGPT-4にフィードバック付きで与えられ、こう言いました。「あなたが私たちにやるように言ったことはこれです。」
Here's how well that worked.
こういうふうにうまくいったかどうかを示しました。
And based on that, it tried to rewrite it, tried to improve it, sort of like iterate upon it.
そして、それに基づいて、それを書き直そうとし、改善しようとしました。まるでそれを繰り返すように。
And this loop continued.
そして、このループが続きました。
And surprisingly, Eureka outperforms humans across all tasks.
驚くべきことに、Eurekaはすべてのタスクで人間を上回っています。
In particular, and this is the really interesting part, Eureka realized much greater gains on high dimensional dexterity environments, meaning as humans get worse at doing the very complex tasks and writing these reward functions for very complex tasks, Eureka does well on those tasks.
特に、これが本当に興味深い部分なのですが、Eurekaは高次元の器用さ環境で大幅な向上を実現しました。つまり、人間が非常に複雑なタスクを行うのが悪化し、非常に複雑なタスクの報酬関数を書くことが難しくなるにつれて、Eurekaはそれらのタスクでうまくいきます。
In other words, it's better at teaching robots how to do complex tasks than humans can.
つまり、Eurekaは、人間よりもロボットに複雑なタスクを教えるのが得意です。
So by the way, this Isaac Gym, so it's a GPU accelerated physics simulator that speeds up reality by a thousand X. So GPUs are the NVIDIA chips that they're so famous for.
ちなみに、これがIsaac Gymです。これは、現実を1000倍速くするGPUアクセラレーション物理シミュレーターです。GPUは、NVIDIAチップで有名です。
And so just imagine a universe of these like little robots that are floating around trying to land, right?
そして、ちょうど周りを漂って着地しようとしているこれらの小さなロボットの宇宙を想像してみてください。
Stretching out for infinity or trying to catch this egg on a plate.
無限に広がっていたり、お皿の上で卵をキャッチしようとしていたりします。
And this simulates kind of our physics, all the friction and gravity and everything else, momentum, to kind of simulate our reality.
これは、摩擦や重力、その他すべての運動量など、私たちの現実をシミュレートするためのものです。
But it's able to run it a thousand times faster.
しかし、それを1000倍速く実行することができます。
And so Eureka, in that environment, by writing rewards for that environment, Eureka is a superhuman reward engineer.
そして、その環境では、その環境のための報酬を書くことで、Eurekaは超人的な報酬エンジニアです。
The agent outperforms expert human engineers on 83% of the benchmark tasks, with an average improvement of 52%. And it's much greater gains on tasks that require sophisticated high dimensional motor control.
そのエージェントは、ベンチマークタスクの83%で専門家の人間エンジニアを上回り、平均的な改善率は52%です。そして、高度な高次元モーター制御を必要とするタスクでの大幅な向上があります。
And this is interesting.
これは興味深いです。
This is kind of a big deal, I think.
これはかなり重要なことだと思います。
So Dr. Jim Fan here is saying, to our surprise, the harder the task is, the less correlated are Eureka rewards to human rewards.
ここにいるジム・ファン博士は驚くべきことに、タスクが難しいほど、Eurekaの報酬と人間の報酬の相関が低くなると言っています。
In a few cases, Eureka rewards are even negatively correlated with human ones, while delivering significantly better controllers.
いくつかの場合、Eurekaの報酬は人間の報酬とさらには負の相関がありますが、かなり優れたコントローラーを提供しています。
What that means is as the tasks get more and more difficult, not only is Eureka better than humans, it also comes up with novel, innovative, completely creative and different ways of solving those tasks or writing those reward functions than humans do.
それはつまり、タスクがますます難しくなるにつれて、Eurekaは人間よりも優れているだけでなく、それらのタスクを解決する方法や報酬関数を書く方法について、人間とは全く異なる新しい、革新的で創造的な方法を考え出します。
So when people say, oh, ChatGPT is just spitting out what it's learned, it's not making anything new. How do you explain this?
人々が、「ああ、ChatGPTは学んだことを吐き出しているだけで、新しいものは何も作っていない」と言うとき、どう説明しますか?
It's better than humans in ways that humans were not even like creative enough to come up with those solutions.
それは、人間が考えつくような解決策を考え出すのに十分なほど創造的ではなかった方法で、人間よりも優れています。
They're new, they're novel, and they're better.
それらは新しいし、斬新で、より良いです。
Here he compares it to AlphaGo, where it made brilliant moves that no human Go player would make.
ここでは、AlphaGoと比較しており、人間の囲碁プレイヤーが行わないような素晴らしい手を打っています。
AI will discover very effective strategies that look alien to us.
AIは、私たちには異質に見える非常に効果的な戦略を発見するでしょう。
So this to me was huge, because this really reinforces this idea of AI, something like GPT-4, being out of the box, better than us, than humans, at training these robots in a simulation, which then these simulations, from what we've seen, they translate very well to the real world.
これは私にとって非常に重要だったのですが、これは本当に、GPT-4のようなAIが、シミュレーションでこれらのロボットを訓練する点で、私たちよりも優れているという考えを強化しています。そして、これらのシミュレーションは、私たちが見てきたところ、実世界に非常にうまく適用されています。
So it's not like this hand that's trained in a simulation is now only able to do that in the simulation.
シミュレーションで訓練されたこの手が、今後はシミュレーション内でしかできないことができるわけではありません。
You take out that data, you put it into a robotic hand with the same characteristics, and it's going to be able to do that.
そのデータを取り出し、同じ特性を持つロボティックハンドに入れると、それができるようになります。
So AI will be training the next generation of AI and robots.
AIは次世代のAIやロボットを訓練することになります。
So this is Ted Xiao.
これはテッド・シャオです。
So he's saying how there will be three to four massive news coming out in the next next few weeks that will rock the robotics and AI space.
彼は、今後数週間でロボティクスとAIの分野を揺るがすであろう3〜4つの大きなニュースが出ると述べています。
He says he believes that all the pieces we need for a modern attempt at embodied intelligence, and keep in mind, so he's at Google and DeepMind.
彼は、現代の具体的な知能の試みに必要なすべての要素が揃っていると信じており、そして、彼はGoogleとDeepMindにいることを念頭に置いてください。
Here he's mentioning how much better the data collection for robots will be.
ここで彼は、ロボットのためのデータ収集がどれだけ改善されるかについて言及しています。
Here's an open source system.
こちらはオープンソースのシステムです。
It's 400 bucks.
それは400ドルです。
It's from Stanford.
それはスタンフォード出身です。
It's designed to democratize robot data collection.
それはロボットのデータ収集を民主化するために設計されています。
How do you collect that data?
そのデータはどのように収集していますか?
Well, here's a human kind of just doing those motions.
まあ、ここには人間がその動作をしているようなものがあります。
There are two cameras on there recording an MP4.
そこにはMP4を記録する2つのカメラがあります。
Interestingly, it looks like they use two mirrors there to kind of create a stereo effect to kind of have just more angles on what they're doing without necessarily using more cameras.
興味深いことに、そこには2つの鏡を使用して立体効果を作り出すようにして、より多くの角度で何をしているかを見るために、より多くのカメラを使用せずにいるようです。
And here's the opening eye back robot saying that there's going to be a new progress update on the droids in four weeks.
そして、ここには新しい進捗状況のアップデートが4週間後にドロイドで行われるというロボットの開いた目が言っています。
And it looks like Moravec's paradox might be false, and we just didn't have the data.
モラベックのパラドックスは誤りである可能性があり、単にデータが不足していただけかもしれません。
So Moravec's paradox is the idea that contrary to traditional assumptions, reasoning requires very little computation, but sensory motor and perception skills require enormous computational resources, meaning it is comparatively easy to make computers exhibit adult-level performance on intelligence tests or playing checkers, and difficult or impossible to give them the skills of a one-year-old when it comes to perception and mobility.
モラベックのパラドックスとは、従来の仮定に反して、推論には非常に少ない計算が必要であり、知覚運動や知覚スキルには膨大な計算リソースが必要であるという考えです。つまり、知能テストやチェッカーのプレイにおいて成人レベルのパフォーマンスをコンピュータに示すことは比較的簡単であり、知覚や移動能力に関して1歳児のスキルを与えることは困難または不可能であるということです。
So he's saying it looks like that might be false, and we just didn't have the data.
彼はそれが誤りである可能性があるように見え、単に私たちにはデータが不足していただけかもしれません。
So can't wait to see what these guys drop, what the new breakthrough is going to be.
これらの人たちが何を発表するのか、新しいブレークスルーが何になるのか楽しみにしています。
Before you go, here's a couple clips from the Demi Sassabi's interview where he touches on some pretty interesting things.
行く前に、デミ・ササビのインタビューからいくつかのクリップをご紹介します。彼が興味深いことに触れています。
Take a look.
ご覧ください。
Do you think the world is ready for something like AGI to show up?
AGIのようなものが登場する準備ができていると思いますか?
It's something that's going to affect everyone in society.
それは社会の誰もが影響を受けるものです。
I think there are questions on international cooperation.
国際協力に関する疑問があります。
I would like to see a lot more of that.
私はそれをもっとたくさん見たいと思います。
Unfortunately, the geopolitical nature of the world right now is not very conducive to that.
残念ながら、現在の世界の地政学的性質はそれにはあまり適していません。
So that's unfortunate timing.
それは残念なタイミングです。
And then we need to, of course, accelerate our research into safety, guardrails, control mechanisms, and also philosophy, too.
そして、もちろん、安全性、ガードレール、制御メカニズム、そして哲学に関する研究を加速する必要があります。
What do we want from our systems and ethics and philosophy?
私たちのシステムや倫理、哲学から何を望むのでしょうか?
It's deep philosophical issues.
それは深い哲学的問題です。
What do we want our systems to do?
私たちのシステムには何を望むのでしょうか?
What values should they have?
どんな価値観を持つべきでしょうか?
And I think society will have to adapt about what it is we want to do in a society where we have AI systems.
そして、AIシステムが存在する社会において、私たちが何をしたいのかについて社会が適応する必要があると思います。
They're able to do very useful things for us.
彼らは私たちに非常に役立つことができます。
Maybe we have abundance because of that, because we crack things like energy problems, things like physics and material design.
おそらく、私たちはエネルギー問題、物理学、素材設計などの問題を解決することで豊かさを得ることができるのでしょう。
So there should be a huge plethora of amazing benefits that we just have to make sure are equally distributed so everyone in society gets the benefit of that.
驚くほど多くの素晴らしい利点があるはずであり、それらが平等に分配されるようにしなければなりません。社会の誰もがその恩恵を受けられるように。
And then I think incredible things might be possible that are written in science fiction books, books like the Culture series by Ian Banks and so on.
そして、サイエンスフィクションの本に書かれているような信じられないようなことが可能になるかもしれないと思います。イアン・バンクスによるCultureシリーズなどの本など。
It's always been my favorite since I was a teenager of a depiction of maximum human flourishing across the cosmos, help by AI systems, solving a lot of these fundamental problems for us, helping us solve a lot of these problems.
若い頃からずっと、AIシステムによって支援され、私たちのために多くの基本的な問題を解決し、私たちを支援することで、宇宙全体での最大限の人間の繁栄の描写が私のお気に入りです。
I think it could be an amazing, amazing future.
それは素晴らしい、素晴らしい未来になるかもしれないと思います。
I'm not even sure.
私は確信が持てません。
If you imagine a world where AGI has arrived and it's solved a lot, helped us solve a lot of big scientific problems, I sometimes call them root node problems.
AGIが到来し、多くの大きな科学的問題を解決し、私たちが解決するのを手伝ってくれた世界を想像してみてください。私は時々それらを根ノード問題と呼びます。
So if you think of a tree of knowledge and what are the core big problems that you want to unlock that unlock many new branches of research, and I think alpha fold again is one of those root node problems.
知識の木を想像してみてください。解きたい中核の大きな問題は何か、それによって多くの新しい研究の枝が解き放たれる。そして、アルファフォールドもまたその根ノード問題の1つだと思います。
But you imagine you crack fusion with it or room temperature superconductors and batteries that are optimal.
しかし、それを使って核融合や常温超伝導体、最適なバッテリーを解明すると想像してみてください。
That opens up, suddenly energy becomes free or cheap, then that has huge consequences on resources like freeing up like you could do more space travel, mine asteroids maybe becomes feasible, all of these things.
それによって、突然エネルギーが無料または安価になり、その結果、宇宙旅行をより多く行ったり、小惑星を採掘することが可能になる、すべてが変わります。
And then suddenly the nature of money even changes.
そして、突然お金の性質さえも変わります。
So I'm not sure people are really understanding.
人々が本当に理解しているかどうかはわかりません。
I don't know if company constructs would even be the right thing to think about at that point.
その時点で企業構造が正しい考え方なのかさえわかりません。
I think, again, this is where international collaboration between governments and other things may be required to make sure that these systems, if there's multiple ones, are managed in the right way and used for the benefit of everyone.
これらのシステムが複数ある場合、それらが適切に管理され、皆の利益のために使用されるようにするために、政府と他の機関との国際協力が必要とされる可能性があると思います。
AlphaFold, but the average person in the street probably doesn't know about that yet, what that impact will be, but they will do if that leads to AI designed drugs and cures for really terrible diseases.
AlphaFoldですが、通りで一般の人々はまだその影響を知らないかもしれませんが、それがAIによる薬や非常にひどい病気の治療法を設計することにつながるなら、知るようになるでしょう。
And I think we're only just a few years away from that.
そして、それまで数年だと思います。
Obviously we spun out Isomorphic Labs, assisted company of Google DeepMiner, an alphabet company, which I also run, which is to take the AlphaFold technologies, move them into chemistry and biochemistry to actually design drugs to bind to the right parts of the protein structures that, of course, AlphaFold has predicted.
明らかに、私たちはGoogle DeepMinerの協力会社であるIsomorphic Labsを立ち上げました。それは、私も運営しているAlphaFoldの技術を化学と生化学に移し、もちろんAlphaFoldが予測したタンパク質構造の正しい部分に結合する薬を実際に設計することです。
And then we've just signed big deals with Big Pharma and on real drug programs.
そして、私たちはビッグファーマとの大規模な取引を結んでおり、実際の薬のプログラムに取り組んでいます。
And I expect in the next couple of years we'll have AI design drugs in the clinic and clinical testing.
そして、次の数年でAIによる設計薬が臨床試験に登場すると予想しています。
And that's going to be an amazing time.
それは素晴らしい時期になるでしょう。
I would say we're a couple of years away from having the first AI design, truly AI design drugs for a major disease, cardiovascular, cancer, we're working on all of those things, isomorphic.
私たちは、主要な疾患、心血管疾患やがんなどに対して、真にAIが設計した最初の薬を持つまでには数年かかると言えます。私たちはこれらすべてのことに取り組んでおり、同形的なアプローチを採用しています。
What does AGI mean to you?
AGIとは、あなたにとってどういう意味ですか?
Well, AGI means a system that is generally capable.
AGIとは、一般的に可能なシステムを意味します。
So out of the box, it should be able to do pretty much any cognitive tasks that humans can do.
そのシステムは、人間が行える認知的なタスクをほぼ何でも行えるはずです。
I think it's going to have to be a battery of thousands of tests and performing well across the board, covering all of the different spaces of things that we know the human brain can do.
私は、数千のテストを行い、全般的に優れたパフォーマンスを発揮し、人間の脳が行えると知られているさまざまな領域を網羅する必要があると考えています。
And by the way, the only reason that's an important, obviously, anchor point is the human brain is the only existence proof we have in the universe, as far as we know, of general intelligence being possible.
ちなみに、それが重要なアンカーポイントである唯一の理由は、人間の脳が一般的知能が可能であるという実在の証拠であるということです。
So that's why I originally studied neuroscience as well as computer science, because clearly, certainly in the early days of AI, it was important to get neuroscience inspiration, too, for how are these intelligent phenomena, how do they come about?
そのため、私が元々神経科学とコンピュータ科学の両方を学んだ理由です。明らかに、AIの初期段階では、これらの知能現象がどのように生じるのか、そのヒントを神経科学からも得ることが重要でした。
What do they look like?
彼らはどのように見えますか?
And therefore, what do these systems need to be able to do in order to exhibit signs of general intelligence?
それゆえ、これらのシステムが一般的な知性の兆候を示すためには、何ができる必要があるのか、それを示すためにはどのような外見をしているのか、ということが重要です。
And I think we're still quite a long way off of that, actually, with the current systems, right?
そして、実際には、現行のシステムではまだかなり遠いと思いますね?
So there's still a long way to go.
実際、現在のシステムでは、そのような目標にはまだかなり遠いと思いますね。
And as I said earlier, a lot of breakthroughs still needed.
そして、前にも言ったように、まだ多くの突破口が必要です。
I would not be surprised if we saw systems nearing that kind of capability within the next decade or sooner.
私たちがそのような能力に近づいたシステムを次の10年以内、もしくはそれ以前に見ることに驚かないでしょう。
この記事が気に入ったらサポートをしてみませんか?