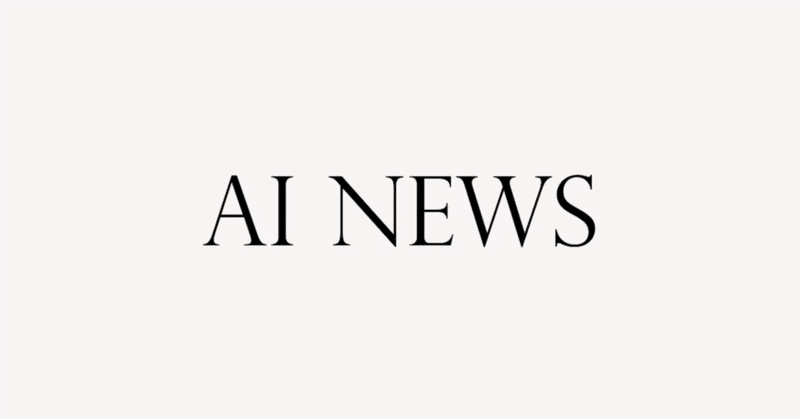
【Ferret:画像解析に特化したAppleの新AI】英語解説を日本語で読む【2023年12月30日|@TheAIGRID】
Appleは「Ferret」という新しいマルチモーダルAIシステムを導入しました。これはGPT-4を特定の面で上回る能力を持ち、CLIP ViT-L/14というツールを使用して画像を解析し、コンピュータが扱える形式に変換します。Ferretは、異なる形状を分析し、各点の詳細と位置を理解することで、画像の特定の部分を正確に見つけ出し、説明することができます。ベンチマークテストでは、入力タイプ、出力の基盤、データ構築、GPT生成、堅牢性、定量評価の面でGPT-4を上回りました。細かいマルチモーダル理解とインタラクションの能力は、複雑な視覚タスクにおいてGPT-4よりも適しています。画像内の対象物や領域を正確に識別する点で、GPT-4に比べて優れています。Ferretの能力は、自動運転システムなどでの使用が期待されています。
公開日:2023年12月30日
※動画を再生してから読むのがオススメです。
So, we finally have some news from Apple regarding their machine learning / LLMs in terms of what they've finally been developing.
ついに、Appleから彼らが開発していた機械学習または大規模言語モデルに関するいくつかの情報が出てきましたね。
So, Apple has introduced a multimodal AI system that is pretty impressive because it does actually exceed GPT-4's capabilities in some regards.
つまり、AppleはマルチモーダルAIシステムを導入したわけだが、これはかなり印象的なもので、実際にGPT-4の能力を超えている部分もある。
And this might be the scenario that many have been looking at when they say the GPT-4 is no longer King.
そしてこれは、GPT-4がもはや王者ではないと言うとき、多くの人が見てきたシナリオかもしれない。
So, let's take a look at exactly what Apple has introduced and how good this new multimodal AI system really is.
では、Appleが何を導入したのか、この新しいマルチモーダルAIシステムが本当に優れているのか、具体的に見てみよう。
So, let's take a look at how this system works.
では、このシステムの仕組みを見てみよう。
It's called Ferret.
これはFerretと呼ばれています。
So, this is essentially the Ferret model and it's based by the Apple researchers.
これは基本的にFerretモデルで、Appleの研究者たちによって作られています。
These are the ones that created it and essentially it's mainly a vision model.
これらはそれを作成したものであり、基本的には主にビジョンモデルです。
So, first it uses a tool called CLIP ViT-L/14 to understand what's in the picture and then turn it into a form the computer can work with.
まず、CLIP ViT-L/14と呼ばれるツールを使って、写真に写っているものを理解し、それをコンピューターが処理できる形にします。
Secondly, it also looks at the words you give it and converts them into a format it can understand.
次に、あなたが入力した単語を見て、それを理解できる形式に変換します。
Then, it identifies areas in the image and if you talk about a specific part of the picture like a cat in the bottom left hand corner, the model uses special coordinates to find exactly where that is in the image.
そして、画像内の領域を特定し、左下隅にいる猫のような画像の特定の部分について話すと、モデルは特別な座標を使用して、それが画像内のどこにあるかを正確に見つけます。
Of course, we do have processing and shapes features and it's really smart in dealing with different shapes in the picture, not just simple boxes.
もちろん、処理や形状の機能もあり、単純な箱だけでなく、画像内のさまざまな形状を扱うことができます。
It looks at many points in the area you're talking about and understands the details and locations of each point.
これは、あなたが話しているエリアの多くのポイントを見て、各ポイントの詳細と位置を理解します。
Finally, it brings together this information together to accurately find and describe the specific part of the picture you're talking about.
最後に、これらの情報をまとめて、あなたが話している写真の特定の部分を正確に見つけ、描写します。
Essentially, what we have here is a really impressive Advanced image identification model that when on certain benchmarks compared to GPT-4, and I did test it myself to just make sure it actually does exceed GPT-4's Vision capabilities.
基本的に、ここには非常に印象的な高度な画像識別モデルがあります。特定のベンチマークでGPT-4と比較したとき、私自身もテストしましたが、実際にGPT-4のビジョン能力を上回っていることを確認しました。
So, you can see here first of all there are some benchmarks that you may want to look at.
まず、ここでいくつかのベンチマークがあることがわかります。
So, you can see on the benchmarks for the Ferret model, we can see that Ferret actually has all of the input types which are Point box and free form.
Ferretモデルのベンチマークを見ると、Ferretはすべての入力タイプ(Point boxとFree form)を持っていることがわかります。
It also has very good output grounding which essentially just means that it can understand exactly the relationship between certain objects in the image and what they actually do in the real physical world.
また、出力も非常に優れています。つまり、画像内の特定のオブジェクトと、実際の物理世界でのオブジェクトの関係を正確に理解することができます。
Then, of course, we have on data construction and GPT generate and robustness and of course the quantitative evaluation of refer SLG ground with chat.
そしてもちろん、データ構築、GPT生成、ロバスト性、そしてもちろんチャットによるSLGグラウンディングの定量的評価もあります。
So, this is actually very interesting because in this section of the paper they didn't actually compare it to GPT-4 with a vision, they compared it to GPT4RoI.
というのも、この論文ではGPT-4とビジョンとの比較ではなく、GPT4RoIとの比較を行っているからです。
But later on in the paper, I will show you that compared to GPT-4 with vision.
しかし、論文の後半で、視覚ありのGPT-4との比較をお見せします。
So, if we take a look at GPT4RoI, we can see here that it says GPT4RoI instruction tuning large language model on region of interest and essentially what GPT4RoI was was a specifically fine-tune version.
GPT4RoIを見てみると、ここに「関心領域における大規模言語モデルの指導調整」と記されています。基本的に、GPT4RoIとは、特定の地域に特化して細かく調整されたバージョンだったということです。
So, in the benchmarks of the PDF, I'm guessing that the researchers likely tested against GPT4RoI instead of GPT-4 vision because GPT4RoI is a specifically designed for understanding and interacting with the regions of interest in images which is a more advanced and specialized task than what GPT-4 Vision might be designed for.
したがって、PDFのベンチマークでは、研究者たちはおそらくGPT-4 Visionではなく、GPT4RoIと比較していると思われます。GPT4RoIは、画像の関心領域を理解し、相互作用するために特に設計されたものであり、GPT-4 Visionが設計されたものよりも高度で特殊なタスクです。
GPT4RoI's ability to combine language and detailed image analysis especially focusing on specific areas within images makes it a more suitable Benchmark for testing the Ferret model's capabilities in fine grained multimodal understanding and interaction.
GPT-4ビジョンは、言語と詳細な画像解析を組み合わせることができ、特に画像内の特定の領域に焦点を当てることができるため、細かい粒度のマルチモーダル理解とインタラクションにおけるFerretモデルの能力をテストするベンチマークとしてより適しています。
This comparison helps to highlight the advancement and specific strengths of the Ferret model in handling complex Vision tasks and we can also see here the GPT4RoI Benchmark so that when you see what GPT4RoI is and why it was compared against the Ferret model you can see exactly why it was very effective and there are some of the these things before like we actually did a video on visual ChatGPT but GPT4RoI does have image it does have region and it does have multi-region and even after all of that the fet model actually does surpass it.
この比較は、フェレットモデルが複雑なビジョンタスクを処理する能力の進歩と特定の強みを強調するのに役立ちます。また、ここではGPT4RoIベンチマークも見ることができます。GPT4RoIが何であり、なぜFerretモデルと比較されたのかを正確に理解することができます。これらのことのいくつかは、実際にはビジュアルChatGPTのビデオを作成しましたが、それでもフェレットモデルはそれを上回っています。
So, here's where we do need to take a look at one of the examples where we do compare it to GPT-4.
ここで、GPT-4と比較する例の1つを見てみる必要があります。
So, this is an example where they say what is the purpose of the object on the bike and region zero is the highlighted yellow region that you can see right here and it says the ground truth the object is a shock absorber on the bike its purpose is to absorb or dampen shock impulses it does this bike converting the kinetic energy of the shock into another form of energy which is then dissipated in the context of the motorcycle it is particularly useful in providing comfort and stability for the rider especially when traveling over uneven or rough terrains.
自転車のオブジェクトの目的は何かということを述べている例です。リージョン0は、ここで見ることができるハイライトされた黄色の領域で、地面の真実のオブジェクトは自転車のショックアブソーバーであり、その目的はショックの衝撃を吸収または減衰させることです。これにより、ショックの運動エネルギーを別の形のエネルギーに変換し、特に不均一または荒れた地形を走行する際に、ライダーに快適さと安定性を提供するのに特に役立ちます。
So, you can see lava the model the vision model didn't get it right KOSMOS-2 Microsoft's multimotor model actually didn't get it right Shakira this another model just just completely didn't get it right.
つまり、lavaというモデルは、ビジョンモデルは正しく理解していなかったということです。 KOSMOS-2 マイクロソフトのマルチモーターモデルは、実際には正しく理解していませんでした。
And then, the Ferret model actually gets it 100% correct.
そして、フェレットモデルは100%正しい。
It says the model is a shock absorber, yada yada Y, and this shows just how effective it is.
モデルはショックアブソーバーであり、これがどれだけ効果的かを示しています。
Now, like I said, I did actually try to put this image into ChatGPT, and I said, What is the purpose of the highlighted region on the bike?
さて、私は実際にこの画像をChatGPTに入れて、「バイクのハイライトされた領域の目的は何ですか?」と言いました。
And the highlighted region on the motorcycle is where the exhaust pipes and muffler are located, which is completely wrong.
そして、バイクのハイライトされた領域は、排気パイプとマフラーが配置されている場所ですが、これは完全に間違っています。
Now, I do want to state that I did actually try this multiple, multiple times.
今、私は実際にこれを何度も試してみました。
Like, I tried this prompt so many different times, and ChatGPT didn't get it right at all.
このプロンプトを何度も何度も試しましたが、ChatGPTは全く正しく理解できませんでした。
Now, maybe you could prompt this better than I did, but on this zero-shot setting, it's simply just not that effective at providing insights onto certain things.
もしかしたら、私よりもうまくプロンプトを出すことができるかもしれませんが、このゼロショット設定では、特定の物事に関する洞察を提供するのに有効ではありません。
Now, one thing that they did actually talk about was the further comparisons between this and GPT-4.
彼らが実際に話したことの1つは、これとGPT-4とのさらなる比較です。
So, if we do take a look, you can see that they actually did some of this testing themselves.
したがって、私たちはこれを見てみると、彼らが実際にいくつかのテストを行ったことがわかります。
So, GPT-4 Vision versus Ferret, you can see here that we have Ferret, and essentially, this is part of the section that actually talks about referring and grounding.
したがって、GPT-4 Vision対Ferretの比較では、ここにFerretがあります。これは実際に参照と基準について話しているセクションの一部です。
So, for example, you can see right here they say, What is region zero used for?
たとえば、ここで「リージョン0は何に使用されますか?」と言っています。
And it says, The object is a pipe used for transporting exhaust gases from a MK cycle.
対象はMKサイクルからの排気ガスを輸送するためのパイプです。
That's correct.
その通りです。
Then, the second region, region one, the object is a shock absorber.
次に、2番目の領域、領域1、対象物はショックアブソーバーです。
That is also correct.
それも正しいです。
Now, this is where they tried GPT-4, and they actually did try to prompt it in two ways, exactly like how I did.
さて、ここでGPT-4が試されたわけだが、彼らは実際に2つの方法でGPT-4を促そうとした。
So, they asked it for the red object.
つまり、赤い物体を要求したのだ。
It did actually get the exhaust pipe or muffler correct.
その結果、排気管やマフラーは正解だった。
Then, the object in the red circle, actually, of course, it does say disc, correct.
そして、赤い丸の中のオブジェクトは、もちろん、ディスクと答えました。
And this is something that I also did encounter.
これも私が遭遇したことです。
Now, what they also tried to do was they tried to get GPT-4 Vision with the coordinates because it might be a bit more accurate.
彼らはまた、座標と一緒にGPT-4 Visionを取得しようとしました。それはより正確かもしれません。
And they did actually get a more accurate answer on the first part of the question.
そして実際、質問の最初の部分ではより正確な答えを得ることができた。
But the second example, it once again just completely fails.
しかし、2つ目の例では、またしても完全に失敗してしまった。
Now, I would like to see tons of more different examples because just one, you know, region with a motorcycle isn't exactly the best.
今、私はさまざまな例をたくさん見たいと思っています。ただ1つのバイクの領域では、最適ではありません。
But what's also fascinating was the grounding.
しかし、興味深いのは、グラウンディングです。
Okay, so we can see here that this is a popular capture that we all know and that we all frequently see when signing up to different websites or signing into different applications.
このキャプチャは誰もが知っているもので、さまざまなウェブサイトにサインアップしたり、さまざまなアプリケーションにサインインしたりするときによく目にするものです。
You can see right here that it says, We have the traffic lights, and it says, Detect all objects among the traffic light, and it actually shows us where the traffic lights are.
信号機があります」と表示され、「信号機の中からすべてのオブジェクトを検出します」と表示され、実際に信号機がどこにあるかがわかります。
Then, of course, ChatGPT tries to detect the traffic lights, and it gets it wrong.
ChatGPTは信号機を検出しようとしますが、もちろん間違えます。
You can see that the traffic lights in ChatGPT are highlighted here, and there aren't any traffic lights here.
ここでは、ChatGPTの信号機がハイライトされていますが、ここには信号機はありません。
Now, I think this is absolutely crazy because in terms of referring, you can see that GPT-4 Vision falls short in understanding relatively small regions.
これは本当に信じられないことだと思います。参照に関しては、GPT-4 Visionは比較的小さな領域の理解において不十分です。
And similarly, for grounding, GPT-4 Vision fails to localize relatively small objects in complex scenes and specific regions.
同様に、グラウンディングに関しても、GPT-4 Visionは複雑なシーンや特定の領域にある比較的小さな物体の定位に失敗する。
But as for grounding, we follow the Yang et al prompt and localize the image using bounding boxes.
しかし、基盤づけについては、我々はYang et alのプロンプトに従って、バウンディングボックスを使用して画像を局所化します。
The image size is width and height, and as we observed, GPT-4 Vision is able to understand the referring to a certain extent, either colored region in the image or with the coordinates in the text.
画像のサイズは幅と高さであり、観察したところ、GPT-4 Visionは、画像中の色のついた領域や、テキスト中の座標を参照することで、ある程度理解することができました。
But when compared to Ferret, it does fall short in precise understanding with those really small regions.
しかし、Ferretと比較すると、本当に小さな領域を正確に理解することはできません。
However, in the paper, they actually did say that, on the other hand, GPT-4 Vision is more knowledgeable in common sense.
しかし、論文の中では、GPT-4 Visionの方が常識的な知識は豊富であると述べている。
For example, it can further highlight that the exhaust pipe can reduce the noise, and it does talk about the fact that GPT-4's enhanced linguistic capabilities are much more advanced.
例えば、排気管が騒音を低減することをさらに強調することができますし、GPT-4の強化された言語能力がはるかに高度であることも述べられています。
Now, in regard to the grounding that we do see at the bottom here, Ferret does excel at identifying most traffic lights, even in cluttered scenes.
さて、この一番下にある接地に関してだが、Ferretは乱雑なシーンであっても、ほとんどの信号機を識別することに優れている。
So, the paper says, Nevertheless, Ferret shines, especially when precise bounding boxes for grounding are needed, and catering to those applications that require pinpoint accuracy in smaller regions.
とはいえ、Ferretは、特に接地のための正確なバウンディングボックスが必要なときに輝きを放ち、より小さな領域でピンポイントの精度を必要とするアプリケーションに対応する。
And this is precisely where Ferret steps in to fill the gap.
そして、Ferretはまさにこのギャップを埋める役割を果たす。
So, overall, if we compare GPT-4 Vision to Apple's new multimodal ferret model, it's clear that ferret excels in accurately identifying small and specific regions in images, particularly in complex scenarios.
全体として、GPT-4 VisionとAppleの新しいマルチモーダルferretモデルを比較すると、Ferretが、特に複雑なシナリオにおいて、画像内の小さく特定の領域を正確に識別することに優れていることは明らかだ。
But GPT-4 can recognize areas outlined in red or specific in text, but tends to struggle with smaller regions.
しかしGPT-4は、赤で輪郭を描かれた領域やテキスト中の特定の領域を認識することはできるが、より小さな領域では苦戦する傾向がある。
Whereas GPT-4 Vision is knowledgeable and effective in general knowledge question and answering related to the image regions, ferret actually stands out for its precision in pinpointing small areas, filling the crucial gap in detailed image analysis.
GPT-4 Visionが画像領域に関連する一般的な知識の質問と回答において知識豊富で効果的であるのに対し、ferretは小さな領域をピンポイントで特定する精度で際立っており、詳細な画像分析における決定的なギャップを埋めている。
Now, we can talk about some of the image implications of this.
さて、このことが画像に与える影響についてお話ししましょう。
Because previously, if this is very effective and it very well might be, we might have a situation on our hands where we have vision models that really do help in terms of performing many different tasks that they weren't trained for.
というのも、もしこれが非常に効果的で、そうなる可能性が非常に高いのであれば、視覚モデルが、訓練されていないさまざまなタスクをこなすという点で、本当に役立つ状況になるかもしれないからだ。
For example, there was a paper which actually was talking about how there were early explorations of visual language models on autonomous driving.
たとえば、自律走行に関するビジュアル言語モデルの初期の探索について話していた論文がありました。
So essentially, this paper actually talked about how you could potentially use GPT-4's vision capabilities for essentially just driving on the road.
つまり、この論文ではGPT-4の視覚能力を道路上での運転に利用できる可能性があると述べているのです。
So of course, everyone knows that there are different AI systems used for self-driving capabilities.
もちろん、自動運転機能にさまざまなAIシステムが使われていることは誰もが知っています。
And although we're not there yet, maybe GPT-4 could help because it's essentially kind of like a mini AGI system that could interpret out-of-context scenarios.
GPT-4は、基本的にミニAGIシステムのようなもので、文脈から外れたシナリオを解釈することができるからです。
So you can see here that it's able to identify certain things and describe the image and exactly what was going on.
つまり、GPT-4は特定のものを識別し、画像と何が起こっているかを正確に説明することができるのです。
And essentially, what they did here was they tried to understand the traffic lights.
そして基本的に、彼らがここで行ったことは、信号機を理解しようとしたことです。
They also tried to essentially say, based on the image that you're seeing, what would your next thing be?
彼らはまた、あなたが見ている画像に基づいて、次に何をするかを示そうとしました。
And sometimes it did get it right.
そして、時にはそれを正しく理解することもあった。
So red highlights the wrong understanding, green highlights the right understanding.
赤は誤った理解を、緑は正しい理解を示しています。
And if we do get an image model that is really effective, we could be seeing these kind of models, maybe even more effective than some of the AI systems that we do have in cars, and thus giving us the full self-driving capabilities.
そして、もし本当に効果的な画像モデルを手に入れることができれば、私たちはこれらの種類のモデルを見るかもしれません。おそらく、私たちが車に持っているいくつかのAIシステムよりもさらに効果的なものであり、完全な自動運転能力を提供してくれるかもしれません。
Because we know that just being able to identify scenarios isn't good enough, because what a lot of these car companies are facing is the fact that not every scenario is going to be the same.
シナリオを識別できるだけでは十分ではないことがわかっているため、これらの自動車会社が直面している問題は、すべてのシナリオが同じではないということです。
A lot of these training examples are in dry and very simple road conditions.
なぜなら、多くの自動車会社が直面しているのは、すべてのシナリオが同じではないという事実だからだ。
Whereas when things are out of context, when there is snow, all these judgments and things that you need, kind of like a mini AGI system, which is exactly what Elon Musk said, are things that, I guess you could say, can't just be done with those AI systems.
一方、コンテキストから外れた場合、雪が降る場合など、これらの判断や必要なものは、ミニAGIシステムのようなものが必要です。これはまさにイーロン・マスクが言ったものであり、これらのAIシステムだけでは対応できないと言えるものです。
So this could mean that maybe we're just about to get some kind of huge update from Apple.
ということは、Appleから何か大きなアップデートがあるのかもしれません。
I'm not entirely sure what they're working on, but this does bring us to the question, and more importantly, one of the big questions, which is, where is Apple anyways?
彼らが何に取り組んでいるのか全くわからないが、これは私たちに疑問、そしてより重要な、大きな疑問のひとつをもたらす。
They've got Siri, and they've been sitting on it for quite some time.
AppleはSiriを手に入れ、長い間それを放置している。
And you might be thinking, what on earth are they going to release?
そして、皆さんは思っているかもしれません、彼らは一体何をリリースするのでしょうか?
Are they ever going to release any kind of AI model or any AI system?
AIモデルやAIシステムを発表するつもりなのだろうか?
But I've got to be honest with you guys, you have to understand that Apple is a company that tends to wait.
しかし、正直に言うと、Appleは待つ傾向がある会社だということを理解してほしい。
But this is the one time that I think Apple waiting might actually be a horrible situation.
しかし、今回ばかりは、Appleが待つということは、実は恐ろしい状況なのかもしれないと思う。
Because it's not like this is a traditional kind of technology.
なぜなら、これは伝統的な種類の技術ではないからだ。
This is the kind of technology that does move very quickly.
なぜなら、これは従来の技術とは違うからだ。
And if you're not caught out, you can be left behind.
そして、遅れをとれば、取り残される可能性がある。
And Apple traditionally doesn't really care about what Samsung do, because usually Samsung has the best features first.
そして、Appleは伝統的にサムスンの動向にはあまり関心がありません。なぜなら、通常はサムスンが最高の機能を最初に持っているからです。
But with Apple, people are loyal.
しかし、Appleの場合、人々は忠実です。
They will wait for the features, even if they're three years behind.
たとえ3年遅れていたとしても、彼らはその機能を待つでしょう。
And even if, you know, the other side, which is usually just Samsung versus or Android versus Apple, people will say that, you know, Android had it first.
そして、たとえサムスン対アンドロイド、アンドロイド対Appleといった対立があったとしても、人々はアンドロイドの方が先だと言うでしょう。
But Apple, the core diehard supporters, will really not care and just simply say, It doesn't matter.
しかし、コアな熱狂的支持者であるAppleは、そんなことはどうでもいい、と言うだろう。
And of course, it will be intriguing to see what Apple actually does, because as we know, anything is truly possible, ladies and gentlemen.
そしてもちろん、Appleが実際に何をするのか興味深いです。なぜなら、何でも本当に可能だからです、皆さん。
Apple have finally decided to make their entrance into the generative AI space.
Appleはついに、ジェネレーティブAIの分野への参入を決めた。
Apple recently announced something called Apple GPT.
Appleは最近、Apple GPTと呼ばれるものを発表した。
Now, Apple GPT is an artificial intelligence language model rumored to be in development by Apple.
Apple GPTは、Appleが開発中と噂されている人工知能言語モデルだ。
It is expected to be similar to OpenAI's GPT-3 and aims to enhance Siri's virtual assistant capabilities and other AI-powered features in Apple's products.
OpenAIのGPT-3に似ていると予想されており、Siriのバーチャルアシスタント機能やApple製品の他のAI搭載機能を強化することを目的としている。
The informal name Apple GPT suggests that it could use a generative pre-trained transformer model, the same kind of model that ChatGPT uses.
Apple GPTという非公式な名称は、ChatGPTが使用しているのと同じ種類の、事前に訓練された生成変換モデルを使用している可能性を示唆している。
Now, Apple GPT started as an experiment by a small team of Apple engineers in 2022 and is currently limited to internal use, assisting with prototyping future features.
現在、Apple GPTは2022年にAppleのエンジニアの小さなチームによる実験として始まり、現在は将来の機能のプロトタイプを支援する内部使用に限定されている。
So, it's clear that Apple has realized that the markets are moving very, very quickly, and they do have an entirely new platform to deploy their generative AI features.
つまり、Appleが市場の動きが非常に非常に速いことを理解し、ジェネレーティブAI機能を展開するための全く新しいプラットフォームを持っていることは明らかだ。
From the new Apple Vision Pro to their new iPhones, Apple has a variety of applications that they could use to deploy their new Apple GPT.
新しいApple Vision Proから新しいiPhoneまで、Appleには新しいApple GPTを展開するために使用できる様々なアプリケーションがある。
And as we stated, Siri seems to be getting a major, major upgrade.
そして我々が述べたように、Siriは大きな大きなアップグレードを受けるようだ。
There are some predicted features that we do want to talk about.
私たちが話したいいくつかの予測された機能があります。
The most anticipated features of Apple GPT include better natural language understanding, which essentially means that when we do talk to Siri and when Siri talks back to us, the conversations are going to be a lot better than the monotone ones that we currently engage in.
Apple GPTの最も期待される機能には、より優れた自然言語理解が含まれます。これは、私たちがSiriに話しかけたり、Siriが私たちに話しかけたりするときに、会話が現在のような単調なものよりもずっと良くなることを意味します。
This is something that Apple hasn't really improved on since the major release of Siri.
これは、Siriのメジャーリリース以来、Appleがあまり改善してこなかったことです。
Number two, we're also going to get some improved text generation.
2つ目は、改善されたテキスト生成を得ることです。
As you know, sometimes when you're typing on your keyboard, you do get a bunch of suggested words.
ご存知のように、キーボードをタイプしていると、候補となる単語がたくさん出てくることがあります。
And if the generative pre-trained transformer can actually allow us to get improved text generation, writing messages in iMessage is going to get a whole lot easier.
事前訓練されたジェネレイティブ・トランスフォーマーによってテキスト生成が改善されれば、iMessageでメッセージを書くのがもっと簡単になります。
And I'm pretty sure that this Apple GPT is probably going to assist you in many other Apple applications as well, such as Notes, iMessage, WhatsApp, and of course, any word writing software.
そして、このApple GPTはおそらく、Notes、iMessage、WhatsApp、そしてもちろんあらゆる単語作成ソフトウェアなど、他の多くのAppleアプリケーションでもあなたを助けてくれると確信している。
Number three is, of course, the enhanced conversational abilities.
3つ目は、もちろん、会話能力の向上です。
And this could mean that potentially, we might be able to customize our own versions of Siri, which could be unique to us.
そして、これは潜在的に、私たちは独自のバージョンのSiriをカスタマイズすることができるかもしれないということを意味するかもしれません。
That would be really, really interesting and a unique spin on what we already have with the generative pre-trained transformers.
それは本当にとても興味深いことで、すでにある生成的な事前訓練されたトランスフォーマーにユニークなアレンジを加えることができるでしょう。
Now, these features are expected to improve Siri's contextual understanding, provide more accurate responses, and enable more realistic conversations with users.
これらの機能は、Siriの文脈理解を向上させ、より正確な応答を提供し、ユーザーとのよりリアルな会話を可能にすると期待されている。
Now, in comparison to other AI tools, Apple GPT is quite similar to other AI tools like ChatGPT and Google Bard in terms of performance and functionality, according to some sources.
さて、他のAIツールと比較した場合、Apple GPTはChatGPTやGoogle Bardのような他のAIツールとパフォーマンスや機能性の点でかなり似ているとの情報もある。
However, it's not publicly available yet, and it's only accessible through a web interface for a select group of Apple employees.
しかし、まだ一般には公開されておらず、Appleの一部の従業員だけがウェブインターフェイスからアクセスできる。
And according to many different resources, such as Bloomberg, Apple is expected to make a major, major announcement about its AI efforts in 2024.
また、Bloombergなど様々な情報源によると、Appleは2024年にAIの取り組みについて重大な重大発表を行う見込みだという。
So, Apple GPT is a language model rumored to be in development by Apple, and it seems that, like in 2024, we're going to get a major overhaul.
つまり、Apple GPTはAppleが開発中と噂されている言語モデルで、2024年に大規模なオーバーホールが行われるようだ。
Now, we aren't sure at when in 2024 this groundbreaking announcement is supposed to be, but like many different Apple conventions, it's probably going to be one of Apple's livestream events that they host throughout the year when they're unveiling latest products or just doing a standard keynote.
この画期的な発表が2024年のいつになるのかは定かではありませんが、おそらくAppleの様々なコンベンションと同様、最新製品の発表や通常の基調講演を行う際に、年間を通して開催されるAppleのライブストリームイベントの1つになると思われます。
So, what Apple have done is they've upgraded autocorrect to the point where it actually uses machine learning.
Appleが行ったことは、オートコレクトを機械学習を使うところまでアップグレードしたということです。
So, before, Apple used to use an archaic old version of machine learning to predict text.
以前は、Appleは古臭い古いバージョンの機械学習を使ってテキストを予測していました。
But now, as you know, as Google pioneered the way in actually creating the Transformer architecture for people to now use, the thing that actually makes ChatGPT so effective, which OpenAI actually built their chatbot around, this is what Apple are now essentially using for their autocorrect word prediction.
しかし、GoogleがTransformerアーキテクチャを実際に作成し、人々が使用するために先駆けたように、ChatGPTを実際に構築したものがOpenAIによって作られたものであり、これがAppleが現在実際に自動修正の単語予測に使用しているものです。
So, although this firstly wasn't a major announcement, it just goes to show that, of course, Apple, as a big company as they are, are seriously paying attention to what is going on in the space.
だから、これはまず大きな発表ではなかったが、もちろん、Appleが大企業として、この分野で何が起こっているかに真剣に注意を払っていることを示している。
I mean, how could you not pay attention to the rapid rise of AI?
つまり、AIの急速な台頭に注目しないわけがないのだ。
There was also another small AI announcement in which many people also did miss, which was introducing Apple's new Journal feature.
また、多くの人が見逃したが、もうひとつ小さなAIの発表があり、それはAppleの新機能Journalの紹介だった。
So, essentially, what Journal was is it's pretty much a feature that allows you to write down your journals, but it is going to be powered by an on-device AI.
基本的にJournalは、ジャーナルを書くことができる機能ですが、オンデバイスのAIによって駆動されます。
The word that they actually used was on-device machine learning.
実際に使われた言葉は、デバイス上の機械学習でした。
So, essentially, your iPhone can create personalized suggestions of moments to inspire your writing.
つまり、本質的には、あなたのiPhoneは、あなたの書くことをインスパイアする瞬間をパーソナライズされた提案を作成することができます。
Now, they also stated that suggestions will be intelligently curated from information on your iPhone, like your photos, location, music, workouts, and more.
提案内容は、写真、位置情報、音楽、ワークアウトなど、あなたのiPhone上の情報からインテリジェントにキュレートされるとも述べている。
And then, of course, you can essentially control what suggestions that they pull from your phone.
そして、もちろん、あなたは基本的にあなたの携帯電話から引き出す提案をコントロールすることができる。
So essentially, what we have here is an AI tool that is going to allow you to write more effectively by pulling from every single piece of data that it has on your phone, such as your photos and many other different sources.
つまり、ここにあるのは、写真やその他さまざまな情報源など、あなたのiPhoneにあるあらゆるデータを引き出すことで、より効果的な執筆を可能にするAIツールなのだ。
Now, one thing that I did find very interesting about this talk from Apple was that they did refuse to mention the term artificial intelligence or AI.
さて、Appleのこの講演で非常に興味深かったのは、彼らが人工知能やAIという言葉について言及しなかったことだ。
Now, when you look at the transcript right here, you can see that AI isn't mentioned, but machine learning is actually mentioned in seven different times.
このトランスクリプトを見ると、AIについては触れられていませんが、機械学習については7回も触れられています。
Then, of course, we have this tweet from a user named Ethan Mik, and essentially, it's a very, very valid point.
そして、もちろん、Ethan Mikというユーザーのツイートがありますが、これは非常に妥当なポイントです。
So in this tweet, he basically says that Apple didn't address the dead end that is Siri in the age of AI.
このツイートで、彼は基本的に、AppleはAIの時代におけるSiriという行き詰まりに対処していないと述べています。
So if you don't know what Siri is, for those of you who don't use Apple, essentially, it's a voice assistant that you can prompt by saying, Hey Siri, and then your phone will simply go up and wake up with a woman who essentially asks you, What would you like to do?
Siriが何かわからない場合、Appleを使用していない人のために説明しますが、基本的には「Hey Siri」と言って促すことができる音声アシスタントで、あなたの電話は単に起動し、女性があなたに「何をしたいですか?」と尋ねるようになります。
Now, it can be a man, it can be a woman, essentially, it's quite like Amazon's Alexa but for iPhone.
男性でも女性でも、基本的にはアマゾンのアレクサのようなものだが、iPhoneのためのものだ。
Now, the problem is, is that when you ask Siri for a restaurant prompt, which is exactly what this guy did, this is Siri's response versus what Microsoft Bing can do with the same exact prompt.
さて、問題なのは、Siriにレストランのプロンプトを求めたとき、まさにこの男性がしたことだが、これはSiriの反応であり、マイクロソフトのBingが同じプロンプトでできることとは異なる。
Now, we do know, of course, Microsoft Bing isn't voice activated, but it just goes to show that in the age of AI, why is Apple declining to spread any news or any advancements?
もちろん、Microsoft Bingが音声で起動するものではないことは承知しているが、AIの時代になぜAppleはニュースや進歩を広めようとしないのだろうか?
Now, I do have an answer for that, and it's simply autonomous products.
それについては、私には答えがあります。それは単に自律型の製品です。
Now, Apple has been actively acquiring a range of artificial intelligence companies in recent years with the aim of enhancing the AI and machine learning capabilities of its products and services.
最近、Appleは人工知能の範囲を広げるためにさまざまな企業を積極的に買収しており、製品とサービスのAIと機械学習の能力を向上させることを目指しています。
The list of companies acquired by Apple includes Emotient, a startup company that uses AI technology to read people's emotions by analyzing facial expressions; Turi, a small Seattle-based startup specializing in machine learning and artificial intelligence; RealFace, a cyber technology startup whose facial recognition technology can be used to authenticate users; AI Music, a startup that uses AI to generate personalized soundtracks and adaptive music; Wave 1, a California-based startup that was developing AI algorithms for video compression; and to name some others, the acquired Shazam, SensoMotoric Instruments, Silk Labs, Drive AI, Laserlike, Spectral Edge, and many, many more.
Appleによって買収された企業のリストには、顔の表情を分析して人々の感情を読み取るAI技術を使用するスタートアップ企業であるEmotientが含まれています。また、機械学習と人工知能に特化した小規模なシアトル拠点のスタートアップであるTuriも含まれています。さらに、顔認識技術を用いてユーザーを認証することができるサイバーテクノロジーのスタートアップ、RealFaceもリストに挙げられています。AIを使ってパーソナライズされたサウンドトラックや適応型音楽を生成するスタートアップ、AI Musicも同様です。また、ビデオ圧縮用のAIアルゴリズムを開発していたカリフォルニア拠点のスタートアップ、Wave 1も買収されました。その他にも、Shazam、SensoMotoric Instruments、Silk Labs、Drive AI、Laserlike、Spectral Edgeなど多くの企業が買収されています。
These acquisitions have allowed Apple to tap into the expertise and technology of these companies to develop advanced AI and machine learning capabilities for a range of applications.
これらの買収により、Appleはこれらの企業の専門知識と技術を活用し、さまざまな用途向けに高度なAIと機械学習機能を開発できるようになった。
For example, the acquisition of Turri in 2016 gave Apple access to the company's expertise in developing machine learning tools and platforms, while the acquisition of Xnor AI in 2019 provided Apple with low-power edge-based AI technology for its product.
例えば、2016年のTurriの買収により、Appleは機械学習ツールやプラットフォームの開発における同社の専門知識を利用できるようになり、2019年のXnor AIの買収により、Appleは自社製品向けの低消費電力エッジベースのAI技術を手に入れた。
By investing in a wide range of AI companies, Apple has been able to stay at the forefront of the AI race and to drive innovation in the technology industry.
さまざまなAI企業に投資することで、AppleはAI競争の最前線に立ち続け、テクノロジー業界のイノベーションを推進してきた。
The company has introduced a range of AI-powered features in recent years, such as facial recognition in the iPhone X and Siri's improved natural language processing, and it has continued to invest heavily in AI research and development.
会社は最近、iPhone Xの顔認識やSiriの改良された自然言語処理など、AIを活用した機能を導入してきました。また、AIの研究開発にも積極的に投資を続けています。
Overall, Apple's acquisitions in the AI space demonstrate the company's commitment to staying ahead of the curve in the technology industry.
全体として、AppleのAI分野における買収は、テクノロジー業界の最先端を行くという同社のコミットメントを示している。
By leveraging the expertise and technology of the companies it has acquired, Apple has been able to enhance the AI and machine learning capabilities of its products and services, driving improvements in user experience, efficiency, and productivity.
Appleは、買収した企業の専門知識と技術を活用することで、製品やサービスのAIと機械学習の能力を向上させ、ユーザーエクスペリエンス、効率、生産性の向上を推進しています。
Apple's extensive research into machine learning is a key part of the company's strategy for staying ahead of the curve in the technology industry.
Appleの機械学習に関する広範な研究は、テクノロジー業界の最先端を走り続けるための同社の戦略の重要な一部である。
With a dedicated department focused on machine learning, Apple is able to invest heavily in research and development, driving innovation and pushing the boundaries of what's possible with this technology.
機械学習に特化した専門部署があるため、Appleは研究開発に多額の投資を行うことができ、イノベーションを推進し、このテクノロジーで可能なことの限界を押し広げることができる。
One way that Apple is demonstrating its commitment to machine learning is by regularly publishing research papers that highlight the company's innovative work in the field.
Appleが機械学習へのコミットメントを示す一つの方法は、この分野における同社の革新的な取り組みに焦点を当てた研究論文を定期的に発表することです。
These papers cover a wide range of topics, from computer vision and natural language processing to autonomous systems and data privacy.
これらの論文は、コンピュータビジョンや自然言語処理から自律システムやデータプライバシーまで、幅広いトピックをカバーしています。
One recent example of Apple's innovative work in machine learning is the development of a program called Facelet.
Appleの機械学習における革新的な取り組みの最近の一例は、Faceletと呼ばれるプログラムの開発である。
This program uses machine learning algorithms to create photorealistic 3D renders of a person's face just using two photos.
このプログラムは、機械学習アルゴリズムを使って、2枚の写真を使うだけで人の顔の写実的な3Dレンダリングを作成する。
This technology has significant applications in the field, such as virtual reality gaming and film production, and it demonstrates the potential of machine learning to drive advances in a wide range of industries.
この技術は、仮想現実ゲームや映画制作などの分野で重要な応用があり、機械学習の可能性を示して、さまざまな産業の進歩を推進しています。
Overall, Apple's heavy focus on machine learning is a testament to the company's commitment to staying at the forefront of the technology industry.
全体として、Appleが機械学習に力を入れていることは、テクノロジー業界の最先端を走り続けるという同社のコミットメントの証である。
By investing heavily in research and development and sharing its work with the wider scientific community, and pushing the boundaries of what's possible with machine learning, Apple ensures that it remains a major player in the AI race.
研究開発に多額の投資を行い、その成果をより広範な科学コミュニティと共有し、機械学習で可能なことの限界を押し広げることで、AppleはAI競争の主要プレーヤーであり続けることを確実にしている。
この記事が気に入ったらサポートをしてみませんか?