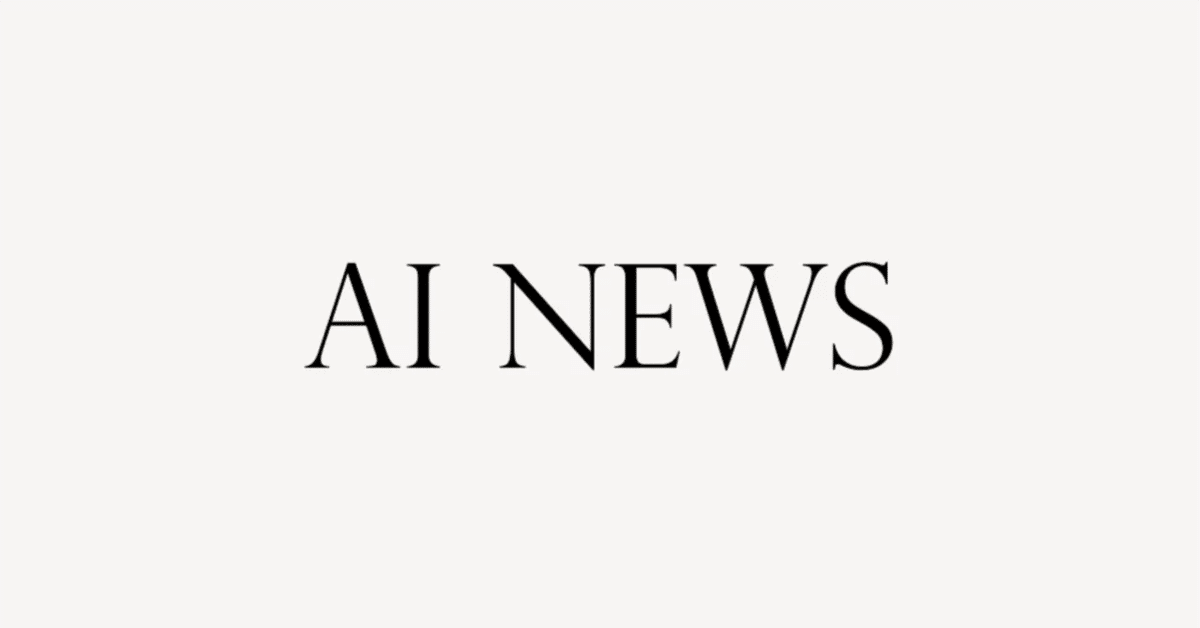
【人間を超えた?NVIDIAの最新AI、驚異の身体能力を獲得】英語解説を日本語で読む【2024年7月27日|@Two Minute Papers】
現在のテキストから画像生成AIシステムには、キャラクターの一貫性という根本的な問題がありますが、NVIDIAの新しい論文はこの課題に対する解決策を提示しています。この技術により、同一人物を異なる状況で生成することが可能になり、さらにControlNetを使用することで、スティックフィギュアから詳細な人物像を生成することもできます。これにより、一貫したキャラクターを維持しながら完全なストーリーを作成することが可能になります。さらに、テキストから3Dジオメトリやアニメーションを生成する技術も紹介されています。特に注目すべきは、テキストから物理ベースのアニメーションを生成する新しい論文です。この技術は約5000の異なる動きから学習し、単純な移動から複雑な動きまで生成することができます。シミュレーション分野でも革新的な進展があり、メッシュ、点群、神経放射場、ガウシアンスプラットなど、さまざまな形式のデータに対して単一のアルゴリズムでシミュレーションを行うことができる新技術が紹介されています。これにより、NASAの火星探査機キュリオシティの熱解析など、これまで困難だった高度な解析が可能になりました。さらに、光の波動性を考慮した完全な波動光学シミュレーションも可能になり、より正確な電波伝搬シミュレーションなどに応用できます。
公開日:2024年7月27日
※動画を再生してから読むのがオススメです。
I am currently at the NVIDIA headquarters to visit their researchers and CEO, Jensen Huang, to learn a bit more about their research works at SIGGRAPH, the most prestigious computer graphics conference and lots of AI things too, and yes, before you ask, of course, I made him hold on to his papers.
私は現在、NVIDIA本社に滞在しており、彼らの研究者やCEOである黃仁勳を訪ねて、SIGGRAPHという最も権威あるコンピュータグラフィックスのカンファレンスや多くのAI関連の研究について少し詳しく知るために来ています。そして、はい、質問される前に、もちろん、彼には自分の論文を持っているように頼みました。
I tried using a current text-to-image AI system to create more images of us based on this as a reference, and I get…well, not quite what I am looking for.
この内容を参考に、現在のテキストから画像を生成するAIシステムを使用して、私たちのより多くの画像を作成しようとしましたが、うーん、私が求めているものとは少し違います。
It understands that there are two people holding on to a paper, a leather jacket here and there, but the people are completely different.
それは、紙を持っている2人の人物や、ここにあるレザージャケットなどを理解していますが、人物はまったく異なっています。
This is one of the most fundamental problems in text-to-image AI systems, character consistency.
これは、テキストから画像へのAIシステムにおける最も基本的な問題の1つであり、キャラクターの一貫性です。
Generating the same characters twice is almost impossible, and they just published an amazing paper that, in the future, could help us with exactly that.
同じキャラクターを2度生成することはほとんど不可能であり、彼らはまさにそれを解決するのに役立つ、素晴らしい論文を発表しました。将来的には、私たちに正確にその点を助けてくれるかもしれません。
When you ask for the same person to be in different situations, that really is the same person.
同じ人物に異なる状況を求めると、それは本当に同じ人物です。
It gets better.
状況は良くなります。
It also supports ControlNet.
それはControlNetもサポートします。
Or in other words, we can draw a stick Figure, and…look at that!
言い換えれば、私たちは棒人形を描くことができ、そして…それを見て!
Exactly the same person will take these poses.
まさに同じ人物がこれらのポーズを取ります。
All this takes 10 seconds per image.
全ての画像には10秒かかります。
Insanity!
狂気です!
With this, we can finally create a full story and never lose a character that we generated before.
これで、私たちはついに完全なストーリーを作成し、以前に生成したキャラクターを失うことはありません。
So cool.
とても素敵です。
This is text-to-image, and in the age of AI, text-to-video is also possible, and get this, text-to-3D geometry is possible too, so how about an even crazier idea?
これはテキストから画像への変換であり、AIの時代にはテキストからビデオへの変換も可能であり、さらに驚くべきことに、テキストから3Dジオメトリへの変換も可能です。ですので、もっと狂ったアイデアはどうでしょうか?
Text-to-animation.
アニメーションへのテキスト変換。
That is exactly right!
それはまさにその通りです!
Dear Fellow Scholars, this is Two Minute Papers with Dr. Károly Zsolnai-Fehér.
尊敬する研究者の皆様、私はカーロイ・ゾルナイ=フェール博士とともにお届けするTwo Minute Papersです。
Hold on to your papers, Fellow Scholars, because this new paper is from just about a week ago and allows us to just write a piece of text and it synthesizes that motion on this virtual character.
研究者の皆様、お手元の論文をお持ちください。なぜなら、この新しい論文はわずか1週間前のもので、テキストを書くだけで仮想キャラクター上でその動きを合成することができます。
It can go from simple locomotion type animations to more complex movements such as dancing and martial arts moves.
それは、単純な移動タイプのアニメーションから、ダンスや武道の動きなど、より複雑な動きまで可能です。
It looked at and learned from approximately 5000 different motions, and you are experienced Fellow Scholars.
約5000種類の異なる動きを見て学びました。そして、あなたは経験豊富な研究者です。
So you know that in works like this, the most important part is looking for movements that are not the simplest ones, but ones that are more complex than what is in the training dataset for the AI.
このような作業では、最も重要な部分は、単純なものではなく、AIのトレーニングデータセットにはないより複雑な動きを探すことです。
I have to say, they did not go easy on this little AI.
率直に言わせていただきますと、彼らはこの小さなAIに手加減しませんでした。
These movements have complexity to them.
これらの動きには複雑さがあります。
Very impressive.
非常に印象的です。
Loving it.
楽しんでいます。
Don't forget that this is a physics-based animation system.
これが物理ベースのアニメーションシステムであることを忘れないでください。
What does that mean?
それはどういう意味ですか?
It means good news and bad news.
それは良いニュースと悪いニュースを意味します。
Good news because it is accurate.
正確であるということが良いニュースです。
The movements are not just made up from thin air, they have to relate to reality, and the bad news is a great deal more amusing because if you make the slightest mistake, you might lose your balance, which can still happen here.
動きは空中からでっち上げられたものではなく、現実と関連していなければなりません。そして悪いニュースは、わずかな間違いをするとバランスを失う可能性があるため、より面白いものです。それはここでも起こり得ます。
Or even worse, look.
それどころか、見てください。
It can even fall over.
それは倒れるかもしれません。
Don't go too crazy with those kicks, little scholar.
蹴りに関してあまり無茶をしないでください、小さな学者さん。
Also, it is quite sensitive to the phrasing we are using in our prompts.
また、私たちのプロンプトで使用している表現にはかなり敏感です。
That means two very similar prompts can lead to results that are not similar at all.
それは、非常に似ている2つのプロンプトが全く似ていない結果につながる可能性があることを意味します。
But the potential is huge, and in cases like this, always invoke the first law of papers, which says do not look at where we are, look at where we will be, two more papers down the line.
しかし、その可能性は巨大であり、このような場合には常に論文の第一法則を引用するべきです。それは、今いる場所を見るのではなく、2つの論文をさらに進めた先の場所を見るというものです。
To think that all this runs in real time on a consumer graphics card, super impressive.
すべてが消費者向けグラフィックカードでリアルタイムで実行されていると考えると、非常に印象的です。
Note that we agreed that NVIDIA would not pay us anything beyond the trip itself, and this video is not sponsored by them, and we also agreed that I would talk about the limitations of the techniques too.
NVIDIAは旅行費用以外には何も支払わないことに同意したこと、そしてこのビデオは彼らによってスポンサーされていないことに留意してください。また、私たちは技術の限界についても話すことに同意しました。
That is the way of the scholar.
それが学者の道です。
No year is complete without a bunch of simulation papers, and I have to say, this year is particularly tasty.
1年がシミュレーションの論文なしで完了することはありませんが、私は言わせていただきます、今年は特に興味深いです。
For instance, if you have a triangle mesh, you need one technique tailored to meshes to work with them.
たとえば、三角形メッシュを持っている場合、メッシュに合わせた技術が必要です。
If you have a neural radiance field, you need another method that is specialized to that.
ニューラル放射輝度場を持っている場合、それに特化した別の方法が必要です。
However, here is a new technique that can simulate meshes, point clouds, nerves, Gaussian splats, anything you can possibly wish for, even tomography scans, and all this with one algorithm.
しかし、ここには、メッシュ、ポイントクラウド、神経、ガウススプラット、あなたが望む可能性のあるもの、さらにはトモグラフィーのスキャンまでをシミュレートできる新しい技術があります。すべてこれを1つのアルゴリズムで実現します。
Note that a limitation here is that these are not the highest fidelity simulations, the ones that can run on Gaussian splats get the closest to the visual fidelity to a production setting, but this still needs one or two more papers to get there.
ここでの制限は、これらが最高の忠実度のシミュレーションではないことです。ガウススプラットで実行できるものが、視覚的な忠実度を製品設定に最も近づけますが、それでも1つまたは2つの論文が必要です。
Check this out, previous simulation techniques, for instance, finite element simulations often do not work that well with highly detailed geometry.
これをご覧ください。例えば、有限要素シミュレーションなど、従来のシミュレーション技術は、非常に詳細なジオメトリにはあまり適していません。
Here is their new technique doing something that was previously almost impossible or too costly to do, and that is doing a thermal analysis of NASA's Curiosity Mars rover.
こちらが彼らの新しい技術で、以前はほとんど不可能または費用がかかりすぎるとされていたこと、つまりNASAの好奇心の火星ローバーの熱解析を行っている様子です。
You, astute fellow scholars, immediately recognize that these are noise patterns that clear up over time, exactly as you see in light transport simulations, ray tracing if you will, so why is that?
あなた、鋭敏な研究者の皆さんは、すぐにこれらが時間の経過とともに解消されるノイズパターンであることに気づくでしょう。光輸送シミュレーション、例えばレイトレーシングで見られるように、それはなぜでしょうか?
Yes, that's right, it borrows some of the techniques used there and applies them to other simulation domains that were previously impossible or too slow and costly to do.
そうです、そこで使用されている技術の一部を借用し、以前は不可能または遅すぎて費用がかかりすぎる他のシミュレーション領域に適用しているのです。
Not This one is a real revolution and also won one of the best papers awards and SIGGRAPH.
これは本当の革命であり、SIGGRAPHのベストペーパーアワードの1つも受賞しました。
That is an honor very few researchers achieve.
それは非常に少数の研究者が達成する名誉です。
Congratulations!
おめでとうございます!
This one is also not without limitations, for instance, the handling of non-convex domains is possible, but more costly.
この方法にも制限があります。例えば、非凸領域の処理は可能ですが、よりコストがかかります。
Talking about ray tracing.
レイトレーシングについて話しています。
With ray tracing, we simulate the path of millions and millions of light rays to create beautiful photorealistic scenes like this one.
レイトレーシングでは、何百万もの光線の経路をシミュレートして、このような美しい写実的なシーンを作成します。
Did you notice that I keep saying rays and ray tracing?
私が「光線」と「光線追跡」という言葉を繰り返しているのに気づきましたか?
But, technically, light is not a ray, it is an electromagnetic wave.
しかし、技術的には、光は光線ではなく、電磁波です。
In most simulations, we just use a ray representation because it's simpler and often good enough for most practical cases.
ほとんどのシミュレーションでは、単に光線の表現を使用していますが、それはより簡単で、ほとんどの実用的なケースには十分です。
However, not always.
しかし、常にそうとは限りません。
A full wave optical light simulation is one of the holy grail problems in this area, and I can't believe I'm saying this, but this new technique proposes a way of doing just that.
光の完全な波光学シミュレーションは、この分野の聖杯の一つであり、私がこれを言っていることを信じられませんが、この新しい技術はそれを実現する方法を提案しています。
What can it do, and why is it useful?
それは何ができるのか、そしてなぜそれが役立つのか?
Get this, it can compute the propagation of cellular signal coverage across a whole city, and if we do it with a simple ray representation, we get this.
これを見てください、それは都市全体にわたる細胞信号カバレッジの伝播を計算することができ、単純な光線表現で行うと、このようになります。
However, these rays of light bend and diffract, and taking it into consideration makes a huge difference and yields much more realistic simulations.
しかし、これらの光線は曲がり、回折するため、それを考慮に入れることは非常に重要であり、より現実的なシミュレーションを生み出します。
This involves evaluating difficult and costly diffraction integrals, and thus, this is still very slow.
これには難解でコストのかかる回折積分の評価が含まれており、そのため、これはまだ非常に遅いです。
Consider this to be a proof of concept paper that made something almost impossible to be now possible.
これは、ほぼ不可能だったことを可能にした概念の証明論文と考えてください。
Great news, the full source code is available for this one.
素晴らしいニュースです、このプロジェクトの完全なソースコードが利用可能です。
Let the experiments begin!
実験を始めましょう!
The quality of these papers is just stunning!
これらの論文の品質はただ驚くべきものです!
Loving it!
大好きです!
By the way, the cafe at NVIDIA is something else.
ちなみに、NVIDIAのカフェは別格です。
I ordered a pizza and it arrived approximately two minutes later.
ピザを注文し、約2分後に届きました。
That's what I call Two Minute Papers Style.
これが私が「Two Minute Papersスタイル」と呼ぶものです。
What do you think?
どう思いますか?
What would you Fellow Scholars use these techniques for?
皆さん学者の方々は、これらのテクニックをどのように活用しますか?
Let me know in the comments below!
コメント欄で教えてください!
この記事が気に入ったらサポートをしてみませんか?