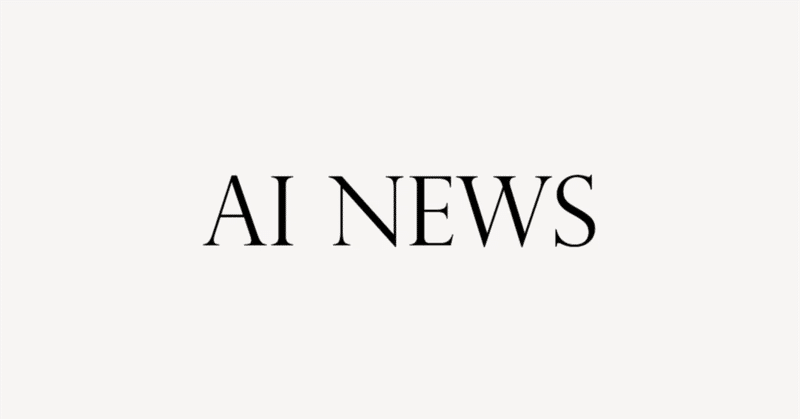
【Eureka:NVIDIAが開発した革命的AIロボットトレーニングツール】英語解説を日本語で読む【2023年10月28日|@TheAIGRID】
NVIDIA ResearchがEurekaというAIエージェントを開発。これは従来のAIとは異なる革新的なロボットトレーニングツールで、ロボットの手でペン回しのトリックを実行できるだけでなく、多様なタスクもこなせる。EurekaはGPT4言語モデルで駆動し、継続的に自己改善するAIシステムである。この研究は、AIの自己改善や複雑なタスク習得、自律的報酬アルゴリズム生成など、AGIに必要な要素を示唆しており、NVIDIAのEurekaはAI研究の新しいマイルストーンとなっている。
公開日:2023年10月28日
※動画を再生してから読むのがオススメです。
NVIDIA Research has recently unveiled a groundbreaking AI agent known as Eureka.
NVIDIA Researchはこのほど、「Eureka」として知られる画期的なAIエージェントを発表した。
This innovative system is not just another AI tool, but a revolutionary approach to training robots with its advanced capabilities.
この革新的なシステムは、単なるAIツールではなく、その高度な能力でロボットを訓練する画期的なアプローチである。
Eureka has accomplished a feat that many might have deemed impossible - it has trained a robotic hand to execute intricate pen spinning tricks, matching the skill level of a human expert.
Eurekaは、多くの人が不可能だと考えたかもしれない偉業を成し遂げた。それは、ロボットハンドを訓練して、人間の専門家の技術レベルに匹敵する複雑なペン回しのトリックを実行させたのだ。
This is not a mere gimmick; the ability to perform such a task indicates a significant leap in robotic precision and learning.
これは単なるギミックではない。このようなタスクをこなす能力は、ロボットの精度と学習が大きく飛躍したことを示している。
In a spectacular display of Eureka's prowess, a video was released showcasing the robot's dexterity and pen spinning.
Eurekaの腕前を華々しく披露するために、ロボットの器用さとペン回しを紹介するビデオが公開された。
But that's just the tip of the iceberg.
しかし、それは氷山の一角にすぎない。
Eureka has been the guiding force behind nearly 30 tasks that robots have now mastered, from opening drawers and cabinets with ease to flawlessly tossing and catching balls, and even adeptly handling scissors.
Eurekaは、引き出しやキャビネットを簡単に開けることから、ボールを完璧に投げたりキャッチしたり、さらにはハサミを巧みに扱うことに至るまで、ロボットが現在マスターしている30近いタスクの指針となっている。
The range of tasks Eureka can teach is impressive.
Eurekaが教えることのできるタスクの幅の広さには目を見張るものがある。
Eureka's research, which has been made public today, encompasses an in-depth paper detailing its workings and the project's AI algorithms.
今日公開されたEurekaの研究は、その仕組みとプロジェクトのAIアルゴリズムを詳細に説明した論文を含んでいる。
What makes this even more intriguing for developers and AI enthusiasts is that they can now tinker with these algorithms.
開発者やAIファンにとってさらに興味をそそるのは、これらのアルゴリズムをいじれるようになったことだ。
NVIDIA has integrated Eureka with the NVIDIA Isaac JY, a revered physics simulation reference tool specifically designed for reinforcement learning research.
NVIDIAはEurekaを、強化学習研究のために特別に設計された、尊敬されている物理シミュレーション・リファレンス・ツールであるNVIDIA Isaac JYと統合した。
A notable aspect of Isaac Jim is that it stands on the robust foundation of NVIDIA Omniverse.
アイザック・ジムの特筆すべき点は、エヌビディア・オムニバースの堅牢な基盤の上に立っていることです。
This development platform specializes in crafting 3D tools and applications and is rooted in the open USD framework.
この開発プラットフォームは、3Dツールとアプリケーションを作ることに特化しており、オープンなUSDフレームワークに根ざしている。
And to top it all off, Eureka's intelligence is driven by the gp4 large language model, a testament to its advanced capabilities.
さらに、Eurekaのインテリジェンスはgp4大規模言語モデルによって駆動され、その高度な能力の証となっています。
Ana Anandkumar, NVIDIA's senior director of AI research and one of the esteemed authors of the Eureka paper, shared her insights on the project.
エヌビディアのAI研究担当シニア・ディレクターであり、Eureka論文の尊敬すべき著者の一人であるアナ・アナンドクマー(Ana Anandkumar)氏は、このプロジェクトについての洞察を語った。
She commented, Reinforcement learning has been at the forefront of numerous groundbreaking achievements over the past decade.
彼女はコメントしました。「強化学習は過去10年間で数々の画期的な成果の最前線にあります」。
However, there still lie many challenges in its path.
しかし、その道のりにはまだ多くの課題が横たわっています。
One of the most daunting among them is reward design, which largely remains a process of trial and error.
その中でも最も困難なもののひとつが報酬の設計であり、試行錯誤のプロセスのままである。
She further added, With Eureka, we are taking a pivotal step.
Eurekaによって、私たちは極めて重要な一歩を踏み出そうとしています。
We aim to merge generative and reinforcement learning techniques, crafting algorithms that can tackle the most challenging tasks with finesse.
私たちは、生成学習と強化学習の技術を融合させ、最も困難なタスクに精巧に取り組むことができるアルゴリズムを作り上げることを目指しています」。
NVIDIA Research's Eureka is much more than just an AI agent; it's a transformative force in the realm of robotic training.
エヌビディア・リサーチのEurekaは、単なるAIエージェント以上のものであり、ロボット訓練の領域において変革をもたらすものです。
One of the most compelling aspects of Eureka is its ability to generate reward programs pivotal for robot learning through trial and error.
Eurekaの最も魅力的な側面の1つは、試行錯誤を通じたロボットの学習にとって極めて重要な報酬プログラムを生成する能力である。
According to the research paper, these Eureka-formulated reward programs surpass those written by human experts in over 80% of tasks.
研究論文によると、Eurekaが作成した報酬プログラムは、80%以上のタスクで人間の専門家が作成したものを上回るという。
This isn't a marginal enhancement; we're talking about a staggering performance boost averaging more than 50% for the robots involved.
これはわずかな向上ではなく、ロボットのパフォーマンスを平均50%以上向上させる驚異的なものだ。
But what powers Eureka to achieve such monumental success?
Eurekaがこのような大成功を収めた理由は何だろうか?
At its heart, Eureka leverages the gp4 large language model (LLM) and the principles of generative AI.
Eurekaの核心は、gp4大規模言語モデル(LLM)と生成AIの原理を活用していることだ。
This allows it to craft software code that provides robots with the rewards essential for reinforcement learning.
これにより、強化学習に不可欠な報酬をロボットに与えるソフトウェア・コードを作成することができる。
Unlike many traditional systems, Eureka stands out because it doesn't lean on task-specific prompts or preset reward templates.
従来の多くのシステムとは異なり、Eurekaが際立っているのは、タスク固有のプロンプトやプリセットの報酬テンプレートに依存しない点だ。
Instead, it brings in a more dynamic approach, seamlessly integrating human feedback.
その代わりに、人間のフィードバックをシームレスに統合し、よりダイナミックなアプローチを取り入れている。
This ensures that the rewards it determines are finely tuned and closely aligned with the developer objectives.
これによって、Eurekaが決定する報酬は、きめ細かく調整され、開発者の目標に密接に沿ったものとなる。
Eureka's efficiency is further amplified when coupled with GPU-accelerated simulations, particularly within the Isaac Jim environment.
Eurekaの効率性は、特にIsaac Jim環境のGPUアクセラレーション・シミュレーションと組み合わせることでさらに高まります。
Isaac Jim is a physics simulation environment specifically designed for reinforcement learning (RL) research in robotics.
アイザック・ジムは、ロボット工学における強化学習(RL)研究のために特別に設計された物理シミュレーション環境です。
It provides an end-to-end GPU-accelerated platform that enables thousands of environments to run in parallel on a single workstation, significantly speeding up training times for complex robotics tasks.
1台のワークステーションで何千もの環境を並列実行できるエンドツーエンドのGPUアクセラレーション・プラットフォームを提供し、複雑なロボット工学タスクのトレーニング時間を大幅に短縮します。
Isaac Jim leverages NVIDIA's FX GPU-accelerated simulation engine and focuses on reinforcement learning, allowing researchers to train AI-based robots more efficiently.
アイザックジムは、エヌビディアのFX GPU加速シミュレーションエンジンを活用し、強化学習に重点を置いているため、研究者はAIベースのロボットをより効率的に訓練することができます。
This combination empowers Eureka to swiftly assess the efficacy of a vast array of reward candidates, paving the way for streamlined and effective robot training.
この組み合わせにより、Eurekaは膨大な数の報酬候補の有効性を迅速に評価し、合理的で効果的なロボット訓練への道を開くことができる。
The AI agent doesn't stop there.
AIエージェントはそれだけにとどまらない。
Post-training, Eureka meticulously compiles a summary of pivotal statistics from the training outcomes.
訓練後、Eurekaは訓練結果から重要な統計データを丹念に集計します。
Using this data, it then guides the LLM to refine and enhance the generation of reward functions.
このデータを使って、LLMが報酬関数の生成を改良し、強化するように導きます。
This iterative process signifies that Eureka is not static; it's continuously evolving and self-improving AI system.
この反復プロセスは、Eurekaが静的なものではなく、継続的に進化し、自己改善するAIシステムであることを意味している。
Its versatility is evident in its wide-ranging applications, from quadruped and bipedal robots to quadrats, dextrous hands, collaborative robot arms (cobot arms), and more.
その多用途性は、四足歩行ロボットや二足歩行ロボット、クアドラット、器用な手、協働ロボットアーム(コボットアーム)など、その幅広い応用範囲からも明らかだ。
Eureka has proven its mettle across diverse robotic forms and functions.
Eurekaは、多様なロボットの形態と機能において、その実力を証明してきた。
For those seeking a deeper understanding, the research paper offers a comprehensive analysis of 20 tasks trained under Eureka's guidance.
より深い理解を求める人のために、研究論文ではEurekaの指導の下で訓練された20のタスクの包括的な分析が行われている。
These tasks are gauged against open-source dexterity benchmarks, which challenge robotic hands to showcase an extensive array of intricate manipulation skills.
これらのタスクは、オープンソースの器用さベンチマークに照らし合わせて評価されており、ロボットハンドが幅広い複雑な操作スキルを披露することに挑戦している。
To provide a visual testament to Eureka's achievements, results from nine distinct Isaac gy environments have been visually rendered using NVIDIA Omniverse, offering a captivating insight into the world of advanced robotic training.
Eurekaの成果を視覚的に証明するために、9つの異なるIsaac gyac環境からの結果がNVIDIA Omniverseを使用して視覚的にレンダリングされ、高度なロボットトレーニングの世界に対する魅惑的な洞察を提供しています。
Now, as good as NVIDIA Eureka's breakthrough is, what if this becomes the new normal?
さて、NVIDIA Eurekaの躍進が素晴らしいものであるのと同様に、これが新しい標準になるとしたらどうでしょう?
What if robots, combined with LLMs, get the ability to recursively self-improve?
LLMと組み合わされたロボットが、再帰的に自己改善する能力を手に入れたとしたら?
The fusion of robots with large language models (LLMs) in a recursive self-improving cycle presents a myriad of possibilities and implications.
ロボットと大規模言語モデル(LLM)の再帰的自己改善サイクルにおける融合は、無数の可能性と示唆を提示する。
One of the most profound advancements expected from recursively self-improving autonomous robots is the attainment of superhuman precision.
再帰的に自己改善する自律型ロボットに期待される最も大きな進歩のひとつは、超人的な精度の達成である。
As we look deeper into this facet, it's evident that this isn't just about accuracy; it's about reshaping industries, redefining standards, and revolutionizing how tasks are approached beyond human limitations.
この側面をより深く見ていくと、これは単に正確さだけの問題ではなく、産業を再形成し、基準を再定義し、人間の限界を超えたタスクの取り組み方に革命をもたらすものであることがわかる。
Human dexterity, while remarkable, has limitations defined by anatomy, fatigue, and even momentary lapses of concentration.
人間の手先の器用さには驚くべきものがあるが、解剖学的構造、疲労、そして一瞬の集中力の欠如によって定義される限界がある。
Robots, unhindered by these constraints and bolstered by continuous improvement, could achieve levels of precision currently deemed unattainable.
このような制約に邪魔されず、継続的な改善によって強化されたロボットは、現在では達成不可能と考えられているレベルの精度を達成できるだろう。
Micro and nano operations with superhuman precision, robots could operate at micro and nano scales with ease.
超人的な精度でマイクロやナノの操作を行うことで、ロボットはマイクロやナノのスケールで簡単に操作できるようになる。
In fields like medicine, this could translate to groundbreaking procedures such as cellular-level surgeries or targeted drug delivery, revolutionizing treatments and interventions.
医療などの分野では、細胞レベルの手術や標的を絞った薬物送達といった画期的な処置が可能になり、治療や介入に革命をもたらす可能性がある。
Enhanced quality control in industries like manufacturing and assembly, minute errors can lead to significant quality and safety issues.
製造や組み立てなどの産業では、品質管理が強化され、微細なミスが品質や安全性の重大な問題につながる可能性がある。
Robots with superhuman precision could ensure that every product, from electronics to automobiles, adheres to the highest standards, drastically reducing errors and recalls.
超人的な精度を持つロボットは、電子機器から自動車に至るまで、すべての製品が最高基準に準拠していることを保証し、エラーやリコールを劇的に減らすことができる。
Artistic endeavors, think of a robot that can replicate the brush strokes of master painters or sculpt with the intricacy of renowned artists.
芸術の分野では、巨匠画家の筆使いを再現したり、著名な芸術家のような緻密な彫刻を施したりできるロボットを思い浮かべてほしい。
Superhuman precision could lead to robots contributing to or even pioneering unique artistic expressions, challenging our perceptions of creativity.
超人的な精度は、ロボットがユニークな芸術表現に貢献する、あるいは先駆者となり、創造性に対する私たちの認識を覆すことにつながるかもしれない。
Complex task mastery through recursive self-improvement, robots might master tasks previously deemed too complex for automation.
再帰的自己改良による複雑な作業の習得 ロボットは、これまで自動化には複雑すぎると考えられていた作業を習得するかもしれない。
This could range from assembling intricate electronic devices without human intervention to cooking gourmet meals by analyzing and perfecting techniques from global cuisines.
これは、人間の介入なしに複雑な電子機器を組み立てることから、世界各地の料理のテクニックを分析し、完成させることによってグルメ料理を作ることまで、幅広い範囲に及ぶ可能性がある。
With all of this possible, that brings us to another interesting question: has Eureka brought us closer to AGI?
これらの可能性を考慮すると、もう一つ興味深い問いが浮かびます: ユーレカは私たちをAGIに近づけてくれたのでしょうか?
While Eureka itself isn't an example of artificial general intelligence (AGI), its features and capabilities hint at the progression towards AGI.
ユーレカ自体は人工汎用知能(AGI)の例ではありませんが、その特徴と能力はAGIへの進展を示唆しています。
Here's how: 1. Adaptive learning: Eureka's recursive self-improvement allows it to refine its algorithms continually.
その方法は以下の通りだ: 1. 適応学習: Eurekaの再帰的自己改良は、継続的にアルゴリズムを改良することを可能にする。
This adaptability, where the system can learn from its mistakes and improve without explicit human intervention, mirrors the general learning processes that AGI would require.
システムが失敗から学び、人間の明示的な介入なしに改善できるこの適応性は、AGIが必要とする一般的な学習プロセスを反映している。
2. Complex task mastery: The ability of Eureka to train robots in tasks previously deemed too intricate for automation, from dexterous hand movements to nuanced activities, showcases a move towards more generalized problem-solving capabilities, a hallmark of AGI.
2. 複雑なタスクの習得: 器用な手の動きから微妙な動作まで、以前は自動化には複雑すぎるとみなされていたタスクをロボットに訓練させるEurekaの能力は、AGIの特徴である、より一般化された問題解決能力への移行を示すものである。
3. Integration with advanced models: Eureka's utilization of the gp4 large language model (LLM) indicates a convergence of different AI specializations.
3. 高度なモデルとの統合: エウレカがgp4大規模言語モデル(LLM)を活用していることは、異なるAIの専門分野が融合していることを示している。
The fusion of robotics, reinforcement learning, and natural language processing is a step towards creating a more holistic and generalized AI system.
ロボット工学、強化学習、自然言語処理の融合は、より全体的で一般化されたAIシステムの構築に向けた一歩である。
4. Autonomous reward algorithm generation: One of the challenges in reinforcement learning is designing the right reward functions.
4. 自律的報酬アルゴリズム生成: 強化学習における課題のひとつは、適切な報酬関数を設計することである。
Eureka's ability to autonomously write reward algorithms reduces the dependency on human experts, indicating a move towards more autonomous and generalized decision-making processes.
報酬アルゴリズムを自律的に作成するEurekaの能力は、人間の専門家への依存を減らし、より自律的で一般化された意思決定プロセスへの動きを示している。
5. Human feedback incorporation: Eureka's capability to integrate human feedback without requiring task-specific prompting demonstrates a more general understanding and adaptability.
5. 人間のフィードバックの取り込み: タスク固有のプロンプトを必要とせずに人間のフィードバックを統合するエウレカの能力は、より一般的な理解と適応性を示している。
This human-AI collaboration is essential for AGI, as it needs to understand and operate within human-centric parameters.
AGIは人間中心のパラメータを理解し、その中で動作する必要があるため、このような人間とAIの連携は不可欠である。
6. Versatility across tasks: Eureka has demonstrated proficiency across a diverse range of tasks.
6. タスク横断的な汎用性: エウレカは多様なタスクに熟達していることを実証している。
This versatility, from understanding complex motor skills to adapting to varied feedback, showcases the kind of broad applicability that would be a feature of AGI.
複雑な運動スキルの理解から様々なフィードバックへの適応に至るまで、この汎用性は、AGIの特徴である広範な適用性を示している。
7. Real-world application and simulation: Eureka's use in real-world scenarios, combined with its integration with simulation environments like NVIDIA's Isaac Jim, means it's not just theory-based.
7. 実世界での応用とシミュレーション Eurekaの実世界シナリオでの使用は、NVIDIAのIsaac Jimのようなシミュレーション環境との統合と相まって、理論に基づくだけではないことを意味する。
AGI would need to operate and adapt in the real world, and Eureka's approach is a step in that direction.
AGIは現実世界で動作し、適応する必要があり、Eurekaのアプローチはその方向への一歩となる。
8. Continuous learning cycle: The continuous feedback loop in Eureka, where it assesses its performance and refines its algorithms, signifies a system that is continuously evolving and self-improving.
8. 継続的な学習サイクル: Eurekaの継続的なフィードバック・ループは、パフォーマンスを評価し、アルゴリズムを改良するもので、継続的に進化し、自己改善するシステムを意味する。
In conclusion, NVIDIA Eureka's capabilities and features are pushing the boundaries of robotic training and hinting at the progression towards AGI.
結論として、NVIDIA Eurekaの能力と機能は、ロボット訓練の限界を押し広げ、AGIへの進展を示唆している。
With its adaptive learning, complex task mastery, integration with advanced models, autonomous reward algorithm generation, human feedback incorporation, versatility across tasks, real-world application and simulation, and continuous learning cycle, Eureka is paving the way for a more holistic and generalized AI system.
適応学習、複雑なタスクの習得、高度なモデルとの統合、自律的な報酬アルゴリズム生成、人間からのフィードバックの取り込み、タスク間の汎用性、実世界への応用とシミュレーション、継続的な学習サイクルにより、Eurekaはより全体的で一般化されたAIシステムへの道を開いている。
And then reapplies them is reminiscent of the iterative learning process that AGI would employ.
そして、それらを再適用することは、AGIが採用するであろう反復学習プロセスを彷彿とさせる。
While NVIDIA's Eureka is undoubtedly a milestone in AI research, it's essential to note that the journey to AGI is multifaceted and complex.
NVIDIAのEurekaがAI研究のマイルストーンであることは間違いないが、AGIへの道のりは多面的で複雑であることに注意する必要がある。
Eureka represents one of many advancements required to achieve a truly generalized intelligence.
Eurekaは、真に一般化された知能を実現するために必要な多くの進歩のひとつを表している。
Nevertheless, its Innovations combined with those from other fields and research Endeavors contribute to the foundational knowledge and tools that could eventually lead to AGI.
とはいえ、Eurekaのイノベーションは、他の分野や研究努力のイノベーションと組み合わせることで、最終的にAGIにつながる基礎的な知識やツールに貢献する。
この記事が気に入ったらサポートをしてみませんか?