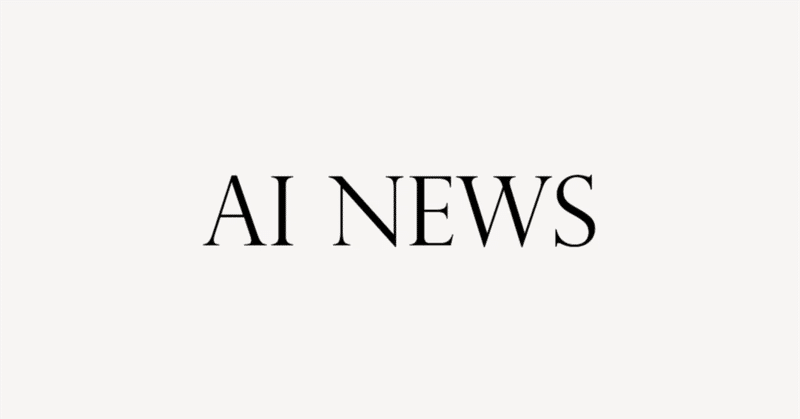
【Magic社のQ-Starモデルに匹敵する技術革新】英語解説を日本語で読む【2024年2月22日|@TheAIGRID】
民間企業がQ-Starモデルに非常に類似した重大な技術革新を達成したと複数の情報源が主張しています。このブレイクスルーは、OpenAIによって昨年開発されたQ-Starモデルに匹敵する能動的推論能力を実現する可能性があると言われています。この進歩は、AI技術の進化の速さに私たちを驚かせています。さらに、GitHubの元CEOであるナット・フリードマンとその投資パートナーのダニエル・グロスが、AIコーディングアシスタントを開発するMagicに1億ドルを投資したことが話題になりました。Magicの技術革新は、GitHub Copilotなどの既存のコーディングアシスタントを超え、完全自動化されたコーディング共同作業者を提供することを目指しています。Magicは、大規模言語モデル(LLM)の新しいタイプを開発し、Googleの最新のGemini LLMよりも5倍多い3.5百万語のテキスト入力を処理する能力を持つと主張しています。
公開日:2024年2月22日
※動画を再生してから読むのがオススメです。
It has been a crazy February, and it's about to get crazier because a privately owned company has just achieved a crazy technical breakthrough that is very, very similar to the Q-Star model, and that's what multiple sources are claiming.
2月は狂ったような月でしたが、民間企業がQ-Starモデルに非常に似た狂った技術的なブレークスルーを達成したと複数の情報源が主張しているため、さらに狂った状況になる予定です。
I'm going to be giving you guys the scoop on this because this is absolutely insane, and I was actually shocked at how quickly AI technology is evolving.
これについて皆さんに情報を提供しますが、これはまったく狂っていて、AI技術がどれだけ速く進化しているかに驚いています。
So here, you can see that it says that just as important, Magic also privately claimed to have made a technical breakthrough.
ここでは、重要なこととして、Magicは技術的なブレークスルーを達成したとも非公式に主張していると書かれています。
This breakthrough could enable active reasoning capabilities similar to the Q-Star model developed by OpenAI last year, according to a person familiar with its technology.
このブレークスルーによって、昨年OpenAIが開発したQ-Starモデルに類似した能動的な推論能力が可能になると、その技術に詳しい人物が述べています。
And that, ladies and gentlemen, is an absolutely astounding statement.
そして、皆さん、それはまさに驚くべき声明です。
Because we do know that Q-Star was a model/system that we didn't really know much about.
Q-Starは私たちがあまり詳しく知らなかったモデル/システムであることを知っているからです。
But there were so many leaks, so many theories, so many capabilities.
しかし、多くのリークや理論、機能が存在しました。
And at that time in which Q-Star was leaked, there were so many things going on at OpenAI.
Q-Starがリークされた当時、OpenAIでは多くのことが進行中でした。
That led us to believe that Q-Star was really true.
それが私たちにQ-Starが本当であると信じさせました。
Now, later on in the video, I'm going to dive into a bit more about Q-Star.
今後、ビデオの中でQ-Starについてもう少し詳しく掘り下げていきます。
Because there is actually a document that kind of holds a lot of knowledge on the Q-Star stuff and why it's such a big deal with whatever this company, this privately owned company Magic, has done.
実際には、Q-Starに関する多くの知識を保持している文書があり、なぜこの会社Magicという私有会社が行ったことがどれほど重要なのかがわかります。
And some of the details I'm going to show you guys are going to really shock you.
そして、皆さんにお見せする詳細のいくつかは本当に驚くことでしょう。
Because this goes to show how quickly we are moving in this space.
なぜなら、これは我々がこの分野でどれほど速く進んでいるかを示しているからです。
So essentially, what actually happened, okay, because I'm going to actually come back to this active reasoning because there's a lot to dissect here.
実際に何が起こったのか、わかりましたね。なぜなら、この能動的な推論に戻るつもりなのです。ここで解明すべきことがたくさんあるからです。
So essentially, it says that former GitHub CEO Nat Friedman and his investment partner Daniel Gross raised eyebrows last week by writing a 100 million dollar check to Magic, the developer of an artificial intelligence coding assistant.
元GitHubのCEOのナット・フリードマンと彼の投資パートナー、ダニエル・グロスが先週、人工知能コーディングアシスタントの開発者であるMagicに1億ドルのチェックを書いたことで注目を集めました。
There are loads of coding assistants already, and the top dog among them is Microsoft's GitHub Copilot.
既にたくさんのコーディングアシスタントが存在しており、その中でもトップのものはMicrosoftのGitHub Copilotです。
So what did Friedman and Gross see in Magic?
フリードマンとグロスはMagicに何を見たのでしょうか?
Remember, these guys wrote a 100 million dollar check to this company out of nowhere because they saw something that they were like, okay, if we invest 100 million, we are certainly going to make our money back, and they basically think that this is probably the best thing ever.
覚えておいてください、これらの人たちは突然その会社に1億ドルの小切手を書いたんです。なぜなら、100億を投資すれば、確実に元が取れると考えたからです。彼らはおそらくこれが最高のものだと思っています。
I'm going to get into more of that, okay, I'm just going to skim this bit quickly because I want to show you guys how all of this ties in.
これについてもう少し話しますが、すぐにこの部分をざっと見てみます。なぜなら、これがどのように関連しているかを皆さんに示したいからです。
So essentially, they state that the answer goes beyond the company's claim that it will soon be able to furnish its customers with a fully automated coding co-workers, not just a semi-automated assistant that finishes fragments of code writing as GitHub Copilot does.
会社が顧客に完全自動化されたコーディングの同僚を提供できるという主張を超えて、Magicが行うブレークスルーは、GitHub Copilotのようにコードの断片を完成させるだけでなく、完全に自動化された同僚を提供できるというものです。
So if you don't know, that's exactly what GitHub Copilot does, it kind of just finishes the fragments of code, and it's not like a fully automated co-worker.
GitHub Copilotが行うのはまさにそれで、コードの断片を完成させるだけであり、完全に自動化された同僚ではありません。
So essentially, I'm guessing that what they're moving towards is more of an agent framework, and that their breakthrough that they've done is clearly a really, really insane one, and essentially they say that the startup has created a new type of Large Language Model that can process huge amounts of data known as a context window.
彼らが進む方向は、おそらくエージェントフレームワークに向かっており、彼らが行ったブレークスルーは明らかに非常に狂ったものであり、スタートアップがコンテキストウィンドウとして知られる大量のデータを処理できる新しいタイプの大規模言語モデルを作成したと述べています。
Now, what I want to talk to you guys about is like this insane week, I think this month is probably going to be the biggest month of AI, probably this year.
さて、皆さんに話したいのは、この狂ったような週についてです。おそらく、この月はAIの最も大きな月になるでしょう。おそらく、今年の中でも。
I don't know that AI is on an exponential, but I think that with the election coming up later on in the year, I think that stuff might drown out the AI stuff, but this is crazy, okay, like this is probably the biggest thing, okay.
AIが指数関数的に進化しているかはわかりませんが、年末に選挙が控えているため、その後AIの話題が薄れるかもしれませんが、これは狂っています。これはおそらく最も大きな出来事です。
So essentially, and I know some of you guys didn't see this because this was overshadowed, so essentially it talks about Magic claims to be able to process 3.5 million words worth of text input, five times as much information as Google's latest Gemini LLM, which in turn was a big advance on OpenAI's GPT-4.
私たちの中にはこれを見逃した人もいると思いますが、基本的にはMagicはGoogleの最新のGemini LLMの5倍の情報を処理できると主張しています。これはOpenAIのGPT-4よりも大幅に進歩したものです。
In other words, Magic model essentially has an unlimited context window, perhaps bringing it closest to the way humans process information.
つまり、Magicモデルは本質的に無制限のコンテキストウィンドウを持っており、おそらくこれは人間が情報を処理する方法に最も近いものです。
Now, why is this crazy?
では、なぜこれが狂っていると言えるのでしょうか?
Well, of course, there are just several higher-reaching implications that are just absolutely insane.
もちろん、ただ単に信じられないほどの高い影響がいくつかあります。
But the first thing that I think is absolutely insane, and as you watch this video, you're going to understand how crazy this breakthrough is.
しかし、私がまず信じられないと思うことは、このブレークスルーがどれだけ狂気じみているかを理解するために、このビデオを見ているときです。
It's because if you haven't been paying attention, basically, Google's latest Gemini LLM was huge, okay?
それは、もし注目していなかったら、基本的に、Googleの最新のGemini LLMが巨大だったということです。
And many people did miss it.
そして、多くの人がそれを見逃しました。
And I'm about to dive into it.
そして、私はそれについて詳しく説明します。
Essentially, they're basically stating that it is five times, okay, five times as much as Gemini, okay?
基本的に、彼らはそれがGeminiの5倍、はい、Geminiの5倍であると述べています。
And I'm about to show you guys how crazy Gemini is.
そして、私は皆さんにGeminiがどれだけ狂気じみているかをお見せします。
And when you see that, you're going to be like, what on earth are you talking about?
そして、それを見たとき、あなたは、一体何を話しているのかと思うでしょう。
But I think the craziest thing here is, of course, the potentially unlimited context window.
しかし、ここで最も狂気じみていることは、もちろん、潜在的に無制限のコンテキストウィンドウです。
I think if that is true, and you know, potentially because when they've spoken about the kind of breakthrough, essentially that could be absolutely game-changing.
もしそれが本当だとすれば、そして、おそらく、彼らが突破口について話したとき、それはまさにゲームチェンジングなことかもしれません。
Now, one thing that I do want to also additionally talk about, okay, because this is something that you guys need to know, is that they're essentially saying that they were able to process as five times much information as Google's latest Gemini LLM, and if you haven't seen this because it got overshadowed by OpenAI's Sora product, which is of course something that was absolutely incredible, you guys are going to go ahead and see this.
今、私がさらにお話ししたいことがあります。これは皆さんが知っておくべきことですが、彼らはGoogleの最新のGemini LLMと比べて、5倍も多くの情報を処理できたと言っています。もしこれを見逃してしまったのなら、それはOpenAIのSora製品によって影が薄れてしまったからでしょう。もちろん、それは本当に素晴らしいものでした。これから皆さんに見ていただきます。
So essentially, Google's Gemini 1.5 Pro was released a couple days ago, and I know nobody paid attention to this unless you are super deep in the AI technology space because it was something that most people just didn't see.
GoogleのGemini 1.5プロが数日前にリリースされましたが、ほとんどの人が見逃してしまったのは、AI技術の分野に深く関わっていない限りです。
It probably would have been the main headline in the AI space if it wasn't for OpenAI's Sora text-to-video technology, and that is of course because it is great and amazing, but Sora stole the show.
もちろん、それは素晴らしくて驚くべきものですが、OpenAIのSoraのテキストからビデオへの技術がなかったら、AIの分野で主要な見出しになっていたかもしれません。
So what you're seeing on screen right now is essentially Google's Gemini 1.5 Pro, and the main thing about this model, if you didn't really know about it, is that it's able to process huge, huge context length.
今画面で見ているのは基本的にGoogleのGemini 1.5 Proです。このモデルの主な特徴は、非常に長いコンテキストを処理できることです。
I'm talking one hour of video, 11 hours of audio, 30,000 lines of code, and 700,000 words.
1時間のビデオ、11時間のオーディオ、30,000行のコード、そして70万語を話しているんです。
That is, I don't even know how many novels that is, but it's a lot.
それは、何冊の小説に相当するかさえわかりませんが、かなりの量です。
You can see it compared to Gemini, you can see it compared to GPT-4 Turbo, and you can also see compared to Claude 2.1.
Geminiと比較して、GPT-4 Turboと比較して、Claude 2.1とも比較できます。
This thing is absolutely insane, this is a killer, okay, of everything else that we knew, and we knew longer context windows were coming because we saw multiple different research papers that were just continually increasing the context window.
このものはまったく狂気じみています。これはすべての他のもののキラーです。私たちが知っていたすべてのことの、そして私たちが長いコンテキストウィンドウが来ることを知っていたのは、単にコンテキストウィンドウを継続的に増やしていくさまざまな研究論文を見ていたからです。
Now, the thing is, you might be wondering, okay, they've got longer context windows, they can analyze an hour of video, they can analyze 11 hours of audio, they can analyze 30,000 hours of code, 700,000 words, that's good and all, but is it even accurate because we know that some of the companies that have done that before, they've done it, but it wasn't that accurate in whatever they were doing.
さて、疑問に思うかもしれませんが、彼らはより長いコンテキストウィンドウを持ち、1時間のビデオを分析したり、11時間のオーディオを分析したり、30,000時間のコードを分析したり、70万語を分析したりできると言っていますが、それはすべて良いことですが、それが正確なのかどうかは疑問です。以前に同様のことを行った企業がありましたが、それは彼らがやっていたことに対してそれほど正確ではありませんでした。
So one thing that they did in order to test this and why I'm showing you guys about Google is because if Magic's claiming that they've done something better than Google have done, that is incredible, so you need to first understand how Google's Gemini Pro works.
これをテストするために彼らが行ったことの一つは、なぜ私がGoogleについて皆さんに示しているのかということです。なぜなら、MagicがGoogleよりも優れたことを主張しているのであれば、それは信じられないことです。ますますまずはGoogleのGemini Proがどのように機能するかを理解する必要があります。
And then when you understand if Magic's beaten it, why the implications of that are so crazy.
それから、もしMagicがそれを打ち負かしたと理解したら、その影響はとても狂っているということです。
So essentially, what Google did was, you know how I just basically said that Google had a super long context window.
基本的に、Googleがやったことは、私がちょうど言ったように、Googleが超長いコンテキストウィンドウを持っていたことをご存知ですか。
You can put like 11 hours of or 22 hours of audio, three hours of video, and seven million words or 10 million tokens.
11時間または22時間のオーディオ、3時間のビデオ、700万語または1000万トークンを入れることができます。
Essentially, what you know they did was, in order to test this because people were like, hmm, I wonder if this is accurate or not, basically they hid a secret phrase in the video.
基本的に、彼らがやったことは、これをテストするために、人々が「これが正確かどうか疑問に思っていたので、基本的に彼らはビデオに秘密のフレーズを隠しました。
And for the video, it was just one frame out of all of them for like, I think it was around two hours or so.
そして、そのビデオでは、すべてのフレームの中で、たった1つのフレームだけで、2時間ほどだったと思います。
That's what they did.
それが彼らがしたことです。
They hid one frame.
彼らは1つのフレームを隠しました。
They asked AI to find it and it did.
AIにそれを見つけるように頼んで、見つけました。
in audio, they hid like, one sentence or like three words and it found it.
オーディオでは、1つの文または3つの単語のようなものを隠し、それを見つけました。
It was like, what is the secret word?
それは、秘密の単語は何かということでした。
It managed to find it.
それを見つけることができました。
And then in text, it was able to do that as well.
そして、テキストでもそれができました。
And I think there were just some very, very small errors on text, but overall, you can see that on successful retrieval versus the unsuccessful retrieval, how crazy it is.
テキストでは、非常に小さなエラーがいくつかあったと思いますが、全体的に、成功した検索と失敗した検索との比較をすると、どれだけ狂っているかがわかります。
It's very, very accurate.
非常に、非常に正確です。
I think it was like 99.9 percent, something like that, pretty much perfect.
99.9パーセントくらいだったと思います、ほぼ完璧です。
So essentially, we're moving towards an era where these super long context windows are going to be absolutely insane.
基本的に、これらの超長いコンテキストウィンドウが完全に狂気じみている方向に進んでいるということです。
And that implication, okay, if Magic's done something where it's essentially beaten Google with like an unlimited context window, I don't even know if that's even possible.
そして、その含意、MagicがGoogleを無制限のコンテキストウィンドウで実質的に打ち負かしたということがあるなら、それが可能なのかさえわかりません。
I think the crazy implications of this is that of course we know that, remember, okay, OpenAI are going to be forced to pull something crazy out the back.
私は、これの狂った含意は、もちろん、私たちは知っていることです、覚えていてください、OpenAIは何か狂ったことを引き出すことを強いられるでしょう。
Now what I want to show you guys as well is, of course, two clips from Gemini's because if you think that this is AI, it's got a long context window, but who even cares because it's not smarter than GPT-4, it's not smarter than Claude 2.1.
今、私が皆さんに示したいのは、もちろん、Gemini'sからの2つのクリップです。なぜなら、これがAIだと思うなら、長いコンテキストウィンドウを持っているけれども、それはGPT-4よりも賢くないし、Claude 2.1よりも賢くないからです。
Just to let you guys know on the benchmarks, Gemini 1.5 Pro is actually surpassing GPT-4 Turbo on all the benchmarks, by the way, just to put that out there.
ベンチマークについて皆さんにお知らせすると、Gemini 1.5 Proは実際には、すべてのベンチマークでGPT-4 Turboを上回っています。ところで、それをお知らせしておきます。
And of course, if it can analyze an hour of video, 11 hours of audio, and all this other stuff, what you can actually do is different tasks, and that's where I'm going to show you guys these two demos.
そしてもちろん、1時間のビデオ、11時間のオーディオ、その他のすべてを分析できるなら、実際にできることは異なるタスクであり、それが私が皆さんにこれらの2つのデモを示すところです。
And then, I'm going to get back to exactly how that works.
そして、それがどのように機能するかを正確に説明します。
This is a demo of long context understanding, an experimental feature in our newest model, Gemini 1.5 Pro.
これは、最新モデルであるGemini 1.5 Proの実験的機能である長いコンテキスト理解のデモです。
We'll walk through some example prompts using the Three.js example code, which comes out to over 800,000 tokens.
私たちは、800,000以上のトークンになるThree.jsの例コードを使用して、いくつかの例のプロンプトを歩きます。
We extracted the code for all of the Three.js examples and put it together into this text file, which we brought into Google AI studio.
私たちは、すべてのThree.jsの例のコードを抽出し、それをこのテキストファイルにまとめ、Google AIスタジオに持ち込みました。
Over here, we asked the model to find three examples for learning about character animation.
ここでは、モデルに、キャラクターアニメーションについて学ぶための3つの例を見つけるように求めました。
The model looked across hundreds of examples and picked out these three: one about blending skeletal animations, one about poses, and one about morph targets for facial animations.
モデルは何百もの例を見て、これらの3つを選び出しました:スケルタルアニメーションのブレンディングについてのもの、ポーズについてのもの、そして顔のアニメーションのモーフターゲットについてのもの。
All good choices based on our prompt.
私たちのプロンプトに基づいて、すべて良い選択です。
In this test, the model took around 60 seconds to respond to each of these prompts, but keep in mind that latency times might be higher or lower as this is an experimental feature we're optimizing.
このテストでは、モデルはこれらのプロンプトに対して約60秒かかりましたが、これは最適化中の実験的機能であるため、レイテンシータイムは高くなるか低くなるかもしれません。
Next, we asked what controls the animations on the littlest Tokyo demo.
次に、リトル東京デモのアニメーションを制御しているのは何かを尋ねました。
As you can see here, the model was able to find that demo and it explained that the animations are embedded within the gltf model.
こちらでご覧の通り、そのモデルはそのデモを見つけることができ、アニメーションがgltfモデル内に埋め込まれていることを説明しました。
Next, we wanted to see if it could customize this code for us.
次に、それがこのコードをカスタマイズできるかどうかを見たかった。
So we asked, Show me some code to add a slider to control the speed of the animation, use that kind of GUI the other demos have.
そこで、アニメーションの速度を制御するスライダーを追加するコードを示してください、他のデモにあるようなGUIを使用してください、と尋ねました。
This is what it looked like before on the original Three.js site, and here's the modified version.
これが元のThree.jsサイトでの見た目ですが、こちらが修正版です。
It's the same scene, but it added this little slider to speed up, slow down, or even stop the animation on the fly.
同じシーンですが、アニメーションをスピードアップ、スローダウン、または途中で停止させるためのこの小さなスライダーが追加されました。
It used this GUI library the other demos have.
他のデモにあるこのGUIライブラリを使用しました。
Set a parameter called animation speed and wired it up to the mixer in the scene.
シーン内のミキサーに接続するために、アニメーション速度というパラメーターを設定しました。
Like all generative models, responses aren't always perfect.
すべての生成モデルと同様に、応答は常に完璧とは限りません。
There's actually not an init function in this demo like there is in most of the others.
実際、このデモには他のほとんどのデモにあるようなinit関数がありません。
However, the code it gave us did exactly what we wanted.
ただし、それが提供したコードはまさに私たちが求めていたことをしました。
Next, we tried a multimodal input by giving it a screenshot of one of the demos.
次に、デモのスクリーンショットを与えることで、マルチモーダル入力を試してみました。
We didn't tell it anything about this screenshot and just asked where we could find the code for this demo seen over here.
このスクリーンショットについて何も伝えず、ただこちらで見たデモのコードをどこで見つけられるか尋ねました。
As you can see, the model was able to look through the hundreds of demos and find the one that matched the image.
ご覧の通り、そのモデルは何百ものデモを見て、画像と一致するものを見つけることができました。
Next, we asked the model to make a change to the scene.
次に、モデルにシーンを変更するように求めました。
Asking, How can I modify the code to make the terrain flatter?
「地形をより平らにするためにコードを修正する方法は何ですか?」と尋ねました。
The model was able to zero in on one particular function called generate height and showed us the exact line to tweak.
そのモデルは、generate heightと呼ばれる特定の機能にゼロインし、調整する正確な行を示してくれました。
Below the code, it clearly explained how the change works.
コードの下には、変更がどのように機能するかが明確に説明されています。
In the updated version, you can see that the terrain is indeed flatter, just like we asked.
更新されたバージョンでは、地形が実際に私たちが頼んだように平らになっていることがわかります。
We tried one more code modification task using this 3D text demo over here.
ここでこの3Dテキストデモを使用して、もう1つのコード修正タスクを試してみました。
We asked, I'm looking at the text geometry demo and I want to make a few tweaks.
私たちは尋ねました、「テキストジオメトリデモを見て、いくつかの微調整をしたいのですが」。
How can I change the text to saygoldfish' and make the mesh materials look really shiny and metallic?
テキストを「goldfish」と変更し、メッシュ素材を本当に光沢のある金属に見せるにはどうすればよいですか?
You can see the model identified the correct demo and showed the precise lines in it that need to be tweaked.
モデルが正しいデモを特定し、調整する必要のある正確な行を示しているのがわかります。
Further down, it explained these material properties, metalness and roughness, and how to change them to get a shiny effect.
さらに下に、これらの素材特性、金属度と粗さについて説明し、それらを変更して光沢のある効果を得る方法を説明しています。
You can see that it definitely pulled off the task and the text looks a lot shinier now.
確かにそのタスクを達成し、テキストは今はずっと光沢があります。
These are just a couple examples of what's possible with a context window of up to 1 million multimodal tokens in Gemini 1.5 Pro.
これらは、Gemini 1.5 Proの最大100万のマルチモーダルトークンのコンテキストウィンドウで可能な例のほんの一部です。
So now that you've seen that bit right there, okay, and you understand how crazy that is, okay, you can understand that this kind of breakthrough, whatever they did that enabled them to potentially beat Google with an essentially unlimited context window or 3.5 million words or five times as much as Google's latest Gemini.
ちょうど今見たあの部分を理解しているわけですが、それがどれだけ驚くべきことかを理解できるでしょう。彼らがGoogleを実質的に無制限のコンテキストウィンドウ、または350万語、Googleの最新のGeminiの5倍の量で打ち負かす可能性を可能にした何かをしたということがわかります。
I'm not sure if this was as latest Gemini 1.5 Pro, but even if they did surpass it, these guys invested like 100 million.
これが最新のGemini 1.5 Proだったかどうかはわかりませんが、たとえ彼らがそれを超えたとしても、これらの人々は1億ドルのような投資をしています。
That is no small amount, okay?
それは少額ではありませんね。
That is a really, really huge amount.
それは本当に、本当に莫大な金額です。
This is definitely something crazy, okay?
これは間違いなく狂ったことですね。
We have to take a look at, even if it's not the context window, we have to take a look at this, okay?
私たちは、コンテキストウィンドウでなくても、これを見なければなりませんね、わかりますか?
Because this is the crazy part, okay?
なぜなら、これが狂った部分なのです、わかりますか?
Like I said, okay?
言ったように、わかりますか?
They claimed, okay, that enable active reasoning capabilities similar to the Q-Star model developed by OpenAI, according to a person familiar with this technology, and that could help solve one of the major gripes with Large Language Models, which is that they mimic what they've seen in their training data rather than using logic to solve new problems.
彼らは、OpenAIが開発したQ-Starモデルに類似した能動的推論能力を可能にすると主張しました。この技術に詳しい人物によると、これは大規模言語モデルの主要な不満の1つを解決するのに役立つ可能性があります。それは、トレーニングデータで見たものを模倣するのではなく、論理を使用して新しい問題を解決することです。
As for how Magic develops its LLM, this person said it took some elements of transformers, a type of AI that powers consumer products like ChatGPT and coding assistants like Copilot, and fused them with other kinds of deep learning models.
Magicが大規模言語モデルをどのように開発しているかについては、この人物は、ChatGPTやCopilotなどの消費者向け製品を動かすAIの一種であるtransformersの要素をいくつか取り入れ、他の種類の深層学習モデルと融合させたと述べました。
And that is something that we'll be exploring later because different architectures are something that people haven't realized are a real, real thing, okay?
そして、これは後で探求することになるものです。異なるアーキテクチャは、人々が実際には本当のものだと気づいていないものですね、わかりますか?
The transformer architecture has just dominated the space since they were invented, and of course, it is something that is now being challenged by a few different ones.
transformerアーキテクチャは、発明されて以来、その領域を支配してきましたが、もちろん、今ではいくつかの異なるものに挑戦されています。
So essentially, what we also have here is the using logic to solve new problems.
ここにあるのは、新しい問題を解決するための論理の使用です。
Now, why does active reasoning change the game?
では、なぜ能動的推論がゲームを変えるのでしょうか?
Well, essentially, with logical problem solving, active reasoning involves the AI system engaging in a form of logical reasoning or deduction to solve problems.
基本的に、論理的問題解決において、能動的推論は、AIシステムが問題を解決するために論理的推論や演繹を行う形態に関与することを意味します。
This means that the system can, in theory, apply principles of logic to come up with solutions to problems it hasn't explicitly been trained on by understanding the underlying relationships and rules.
これは、理論上、システムが、根底にある関係や規則を理解して、トレーニングされていない問題に対する解決策を考えるために論理の原則を適用できることを意味します。
And this actually does go beyond pattern matching.
そして、これは実際にはパターンマッチングを超えています。
So instead of relying solely on statistical patterns in the data it was trained on, a system capable of active reasoning would be able to infer new information or make predictions based on logical deductions.
トレーニングされたデータ内の統計的パターンにのみ依存する代わりに、能動的推論が可能なシステムは、論理的推論に基づいて新しい情報を推論したり、予測したりすることができるでしょう。
This capability would allow it to essentially think more like a human in terms of applying general principles to specific and unseen scenarios.
この能力により、具体的で見たことのないシナリオに一般的な原則を適用する点で、本質的に人間のように考えることができるようになります。
Now, what's also cool about this is that active reasoning also implies the ability to dynamically update and adapt to new problems and situations by applying learned concepts in novel ways, not just recalling or recombining information from the training data.
さらに、能動的推論には、学習した概念を新しい方法で適用することによって、新しい問題や状況に動的に更新および適応する能力も含まれています。訓練データからの情報の単なる記憶や再結合だけでなく、新しい方法で学習した概念を適用することができるのです。
So that right there, guys, that dynamic adaptation, actively reasoning and adapting to new problems and situations is a kind of intelligence that only humans have possessed.
このダイナミックな適応、新しい問題や状況に主体的に理論的に考え、適応することは、人間だけが持っている種類の知能です。
And the difference is that the current LLM capabilities are that they're essentially pattern recognition and generators.
そして違いは、現在の大規模言語モデルの能力が基本的にパターン認識と生成であるということです。
LLMs primarily operate by recognizing patterns in vast amounts of data and text data and generating responses based on statistical likelihood.
大規模言語モデルは主に、膨大な量のデータやテキストデータの中のパターンを認識し、統計的な可能性に基づいて応答を生成することで動作します。
So they excel at producing text that is coherent and contextually appropriate based on the examples they have seen during training.
そのため、彼らは、トレーニング中に見た例に基づいて、連続性があり文脈に適したテキストを生成するのに優れています。
And essentially, they mimic human-like responses.
基本的に、彼らは人間らしい応答を模倣しています。
LLMs can generate responses that mimic human-like text across various domains and style.
大規模言語モデルは、さまざまなドメインやスタイルで人間らしいテキストを模倣する応答を生成することができます。
However, their understanding is a little bit limited to correlating input with similar context they've encountered in their training data without true comprehension.
ただし、彼らの理解は、トレーニングデータで遭遇した類似の文脈との入力を関連付けることに限定されており、真の理解はない。
And of course, they do have limited deductive reasoning.
もちろん、彼らは限られた演繹的推論を持っています。
So essentially, while LLMs can sometimes appear to be reasoning, their process is more about matching patterns than actual logical deduction.
基本的に、大規模言語モデルは時々推論しているように見えるかもしれませんが、そのプロセスは実際の論理的演繹よりもパターンの一致に関するものです。
And they can struggle with certain tasks that require genuine understanding, causality, and complex logical inference, especially if those tasks are not well represented in their training data.
特に、それらのタスクが彼らのトレーニングデータに適切に表現されていない場合、彼らは本物の理解、因果関係、複雑な論理推論を必要とする特定のタスクに苦労することがあります。
So the dynamic adaptation, the beyond pattern matching, being able to infer new information or make predictions based on logical deductions, and actively apply principles of logic to come up with new solutions or solutions to problems it hasn't been explicitly trained on by understanding underlying relationships and rules is definitely a true game changer.
それによって、動的な適応、パターンマッチングを超えた、新しい情報を推論したり、論理的な推論に基づいて予測をしたり、新しい解決策を考え出したり、明示的にトレーニングされていない問題の解決策を理解することで、基礎となる関係やルールを理解して論理の原則を積極的に適用することが、確かに真のゲームチェンジャーです。
And this is something that we've seen over the internet from the release of Gemini 1.5 Pro.
そして、これはGemini 1.5 Proのリリース以来、インターネット上で見られるものです。
Because essentially what people are now able to do is they're able to now solve a lot of long-form problems.
なぜなら、基本的に、人々が今できるようになったことは、長い形式の問題を解決することができるようになったということです。
If you have 30,000 lines of code versus just I guess you could say like 500 lines of code or even like 10 or like 100 lines of code, we're able to solve vastly different problems.
もしも、3万行のコードがある場合と、たった500行のコードや10行や100行のコードがある場合、我々は大きく異なる問題を解決することができます。
You're able to essentially get a lot more from when an AI system kind of understands all of that text and is able to digest all of that.
あなたは、基本的に、AIシステムがそのテキストを理解し、それを消化することができるとき、はるかに多くのことを得ることができます。
It just completely changes the game and it leads us more towards a human in terms of how a human thinks due to that active reasoning combined with that as well.
それは完全にゲームを変えるものであり、その積極的な推論と組み合わせて、人間がどのように考えるかにより近づけるものです。
So that is why this is going to be a complete game changer.
これが完全なゲームチェンジャーになる理由です。
Now, something that I did want to dive into that I did find kind of fascinating was the fact that Magic actually talked about proprietary architecture.
今、私が実際に興味を持ちたいと思ったのは、Magicが実際に独自のアーキテクチャについて話していたという事実です。
Okay, so here's this kind of like small presentation thing from Magic and this is about to get pretty crazy.
では、Magicからのこの小さなプレゼンテーションについて、かなりクレイジーになる予定です。
It says, Magic is working on frontier-scale code models to build a co-worker, not just a copilot.
Magicは、共同作業者を構築するためのフロンティアスケールのコードモデルに取り組んでおり、単なる共同作業者ではないと述べています。
And it says, Things we believe: code generation is both a product and a path to AGI.
そして、私たちが信じていることは、コード生成は製品であり、AGIへの道であると述べています。
And it says, AGI safety matters and is solvable.
そして、AGIの安全性は重要であり、解決可能であると述べています。
And it says, To build a great AI product, we need to train our own frontier-scale model, which is essentially what they're doing.
そして、優れたAI製品を構築するためには、自分たちのフロンティアスケールモデルを訓練する必要があると述べています。それが彼らが実際に行っていることです。
And the last point, and the top point, are going to be essentially points why I'm going to be talking about this.
そして最後のポイント、そして最も重要なポイントは、私がこれについて話す理由になるでしょう。
And the last point is something that I do want to touch on, is that they state that transformers aren't the final architecture.
そして最後のポイントは、トランスフォーマーが最終的なアーキテクチャではないと述べていることです。
We have something with a multi-million context token context window.
私たちは、複数のコンテキストトークンコンテキストウィンドウを持つものを持っています。
So that is something that is pretty crazy.
それはかなりクレイジーなことです。
Now, here's the thing.
さて、ここが重要な点です。
This tweet, I read it at first and I kind of saw it and was like, It doesn't really mean anything.
最初にこのツイートを読んだとき、なんとなく見て、「あまり意味がないな」と思いました。
and then i kind of read it again, and then i was like, oh okay, this is actually bigger than i think.
そしてもう一度読んで、ああ、これは実際に思っていたよりも大きいと気づきました。
So Nat Friedman, the guy who invested 100 million dollars, he stated that Magic Dev has trained a groundbreaking model with many trillions of tokens of context that performed far better in our evals than anything we've tried before.
14億ドルを投資した人、ナット・フリードマンは、Magic Devが過去に試したどんなものよりもはるかに優れた成績を収めた画期的なモデルを訓練したと述べました。
Okay, I want you guys to see what he said there.
彼がそこで言ったことを見てほしい。
Okay, this thing performed far better in our evaluations than anything we've tried before.
このものは、これまで試したどんなものよりもはるかに優れた成績を収めました。
Okay, and although this was, he did tweet this before the Google's Gemini Pro release, him saying that it's far better in our evals than anything we've tried before is something that is quite shocking because he's not saying it's slightly better, he's saying that it's far better.
GoogleのGemini Proのリリース前にツイートしたものでしたが、彼が「これまで試したどんなものよりもはるかに優れた」と言っていることはかなり驚くべきことです。彼はわずかに優れているとは言っていません。はるかに優れていると言っています。
Okay, he's saying they're using it okay to build an advanced AI programmer that can reason over your entire code base and the transitive closure of your dependency tree.
彼は、それを使って、あなたのコードベース全体と依存関係ツリーの推移閉包を理解できる高度なAIプログラマを構築していると言っています。
And if this sounds like Magic, well, you get it.
もしこれが魔法のように聞こえるなら、理解できるでしょう。
Okay, so essentially he's stating that he was so impressed that we are investing 100 million dollars in the company today.
彼は基本的に、彼は今日その会社に1億ドルを投資するほど感銘を受けたと述べています。
So I think that that is pretty insane that they saw something in that pitch deck.
私は、そのプレゼン資料で何かを見たということはかなり狂っていると思います。
Okay, these guys were working on their products and whatever, and they saw something that they tried and they were like, this is so crazy, we are putting 100 million dollars into it.
これらの人々は自分たちの製品に取り組んでいて、何かを試して、これはとても狂っていると思って、1億ドルを投入すると言ったのです。
This, you have to understand that these guys are going up against Microsoft's GitHub Copilot, okay, and essentially something that is backed by OpenAI, and this guy put 100 million dollars into it.
これは、MicrosoftのGitHub Copilotに対抗していることを理解する必要があります。基本的にOpenAIの支援を受けているものであり、この人は1億ドルを投資しています。
So he's basically betting that what they have is really, really good.
彼は基本的に、彼らが持っているものが本当に本当に良いと賭けていると言っています。
And like I said, he said that the best way to really understand if someone is really, really on the ball, I guess, or I guess you could say someone is backing their position is money, okay, and money does talk, and these guys are putting 100 million dollars.
そして私が言ったように、誰かが本当に物事を理解しているかどうかを理解する最良の方法は、おそらく、またはおそらく、その立場を支持しているかどうかを知る最良の方法はお金です。お金は話すものであり、これらの人々は1億ドルを投入しています。
They're putting the money where their mouth is, and they're saying, look, we think this thing's so good, we're gonna put 100 million dollars of our own money in there.
彼らは言葉にお金を出して、見てください、私たちはこのものがとても良いと思って、1億ドルの私たち自身のお金をそこに投入するつもりです。
Not just 10, not just 20, not just 30, 100 million dollars is quite a lot, and that's a pretty, pretty thing.
10億ドルでもなく、20億ドルでもなく、30億ドルでもなく、1億ドルはかなり多く、それはかなりのことです。
Okay, they're saying, better in our evals than anything we tried before, that is pretty crazy.
彼らは、「これまで試したどんなものよりもはるかに優れた」と言っていますが、それはかなり狂ったことです。
So, the next point is, of course, here, and I wondered, okay, I was wondering for some time, okay, and of course, this is just pure speculation, and of course, I don't know, but I'm guessing that potentially they maybe could have used this architecture.
次になるのは、もちろんここです。私はしばらくの間考えていました。もちろん、これは単なる推測ですし、実際、私はわかりませんが、おそらく彼らはこのアーキテクチャを使用している可能性があると思います。
Okay, so if you don't know what this is, okay, this is Mamba.
OK。もし分からないことがあれば、これはMambaです。
Now, essentially, around two months ago, there was this paper, okay, Mamba: Linear Time Sequence Modeling with Selective State Spaces, and essentially, this was touted to be a replacement for transformers with an exceptional performance on long context windows.
実際、約2か月前にこの論文がありました。Mamba: Linear Time Sequence Modeling with Selective State Spacesというもので、これは長いコンテキストウィンドウで優れたパフォーマンスを発揮するトランスフォーマーの代替として謳われていました。
So, it's not a direct replacement for transformers, but it is an alternative architecture that addresses some of the inefficiencies found in the transformer models that power ChatGPT and all of the other LLMs that we know.
これはトランスフォーマーの直接的な代替ではありませんが、ChatGPTや他の大規模言語モデルを駆動するトランスフォーマーモデルに見られるいくつかの非効率性に対処する代替アーキテクチャです。
Okay, so Mamba uses state space models, which are SSMs, to achieve linear time complexity for input computation, which can particularly be beneficial for processing long sequences efficiently.
Mambaは、入力計算のための線形時間複雑性を達成するために、状態空間モデル(SSM)を使用しています。これは特に長いシーケンスを効率的に処理するのに有益です。
Now, it's been shown to actually outperform transformers in inference speed and efficiency, especially on larger context sizes.
実際、推論速度と効率において、トランスフォーマーを上回ることが示されており、特に大きなコンテキストサイズで優れたパフォーマンスを発揮しています。
And it's important to know that while Mamba has demonstrated impressive performance on language modeling and tasks involving audio and DNA sequences, it's not superior in all aspects.
Mambaは言語モデリングやオーディオ、DNAシーケンスを含むタスクで印象的なパフォーマンスを示していますが、すべての側面で優れているわけではありません。
That's why I said I'm not really sure.
だからこそ、私は本当によくわからないと言ったのです。
For example, there was actually a study from the Kempner Institute at Harvard University that actually showed that transformers are better at Mamba at tasks that involve copying and retrieval from the input context, such as future learning and retrieval tasks that are common in foundation models.
たとえば、ハーバード大学のケンプナー研究所からの研究があり、実際に、トランスフォーマーよりもMambaの方が、入力コンテキストからのコピーと取り出しを含むタスクにおいて優れていることが示されています。
However, Mamba models are particularly better at transformers that involve tasks in processing long sequences efficiently and in scenarios where computational efficiency is crucial.
ただし、Mambaモデルは、長いシーケンスを効率的に処理するタスクや、計算効率が重要なシナリオにおいて、トランスフォーマーよりも特に優れています。
And the architecture of Mamba, which combines elements of state space models, SSMs, and recurrent neural networks, allows it to excel in several specific areas.
そして、Mambaのアーキテクチャは、状態空間モデル(SSM)と再帰ニューラルネットワークの要素を組み合わせることで、いくつかの特定の領域で優れたパフォーマンスを発揮できるようになっています。
So it can excel in language modeling.
言語モデリングに優れています。
It's shown impressive performance in language modeling tasks surpassing similarly sized transformers and even competing on par with transformers that are twice its size in both pre-training and downstream evaluation tasks.
同様のサイズのトランスフォーマーを上回り、事前トレーニングおよびダウンストリーム評価タスクの両方で、2倍のサイズのトランスフォーマーと同等のパフォーマンスを発揮しています。
And of course, long sequences.
そしてもちろん、長いシーケンス。
This thing can handle long sequences like a champ due to its efficient sequence modeling technique.
このものは、効率的なシーケンスモデリング技術によって、長いシーケンスを優れた処理能力で扱うことができます。
Mamba is actually better suited for tasks that require processing information over extended sequences.
Mambaは、情報を長いシーケンスで処理する必要があるタスクに実際に適しています。
And this is actually attributed to its ability to linearly scale with sequence contents length, making it particularly beneficial for applications where long context sizes are involved, like coding.
これは、シーケンスの内容の長さと線形的にスケーリングする能力に起因しており、コーディングなど、長いコンテキストサイズが関与するアプリケーションに特に有益です。
And it also demonstrates exceptional performance across varied domains.
さらに、さまざまなドメインで優れたパフォーマンスを示しています。
Of course, in audio and genomics, like we already talked about.
もちろん、音声やゲノミクスなど、すでに話したように。
And of course, it actually does address the computational limits in long context scenarios with transformers.
そして、もちろん、トランスフォーマーにおける長いコンテキストシナリオの計算上の限界に対処しています。
And another thing that it actually does have is, of course, in-context learning.
そして、実際に持っているもう一つのことは、もちろん、コンテキスト内の学習です。
So while Mamba matches the performance of transformer models for in-context learning, it's particularly noted for scaling well with the number of in-context examples.
Mambaは、コンテキスト内の例の数と特によくスケーリングすることで、トランスフォーマーモデルのパフォーマンスに匹敵します。
And this actually suggests that Mamba maintains a considerable performance edge in scenarios where leveraging context information is crucial for task performance.
Mambaは、コンテキスト情報を活用することがタスクのパフォーマンスにとって重要なシナリオで、かなりのパフォーマンスエッジを維持していることを示唆しています。
So it's clear that whatever kind of architecture that these guys do have, because they said that transformers aren't the final architecture, and we have something with the multi-million token context window, it could be Mamba.
これらの人々が持っているアーキテクチャの種類は明らかであり、トランスフォーマーが最終的なアーキテクチャではないと言ったので、マルチミリオントークンのコンテキストウィンドウを持つものがあるかもしれません。
I'm not entirely sure if it is this thing.
私はそれがこのものであるかどうか完全にはわかりません。
I mean, it wouldn't surprise me, but then again, of course, this thing does have some limitations.
つまり、私を驚かせることはありませんが、やはり、もちろん、このものにはいくつかの制限があります。
There's not that much of a good ecosystem.
あまり良いエコシステムはありません。
But then again, I do find it kind of crazy that this paper was released two months ago.
しかし、2か月前にこの論文が公開されたことはかなり驚きです。
and then all of a sudden google comes out with, I don't know, that google is kind of working with transformers, but all of a sudden google comes out with a 10 million kind of context window, and then these guys come out with something that's got multi-million context window, and it's pretty much unlimited.
そして突然、Googleが、トランスフォーマーと協力しているかどうかはわかりませんが、突然、Googleが1000万のコンテキストウィンドウを持つものを出して、これらの人々がマルチミリオンのコンテキストウィンドウを持つものを出して、ほぼ無制限です。
I'm wondering if they're using, mamba to kind of do any of this or they're just using a completely different architecture that they've developed combined with the essence of LLMs.
彼らがこれらのいずれかを行うためにMambaを使用しているか、それとも完全に異なるアーキテクチャを開発し、大規模言語モデルの本質と組み合わせているかどうか疑問に思っています。
I'm not entirely sure what architecture they're working on, but I think that once the new architecture does get I guess you could say, into the wider community because of course these guys are a private company, they're going to try and protect whatever it is that they have, whatever proprietary architecture that they're using.
彼らが取り組んでいるアーキテクチャが何かは完全にはわかりませんが、新しいアーキテクチャが広く普及すると、もちろんこれらの人々は私企業ですので、彼らが使用しているプロプライエタリなアーキテクチャを守ろうとするでしょう。
I think it will be kind of fascinating and of course, essentially the guy who's, so now here we have the CEO of the Magic company, okay, the Magic AI labs, and essentially he states that we are writing code on a mission to build a safe super intelligence.
それはかなり魅力的だと思いますし、もちろん、ここには魔法の会社のCEOがいます。魔法のAIラボです。基本的には、彼は安全なスーパーインテリジェンスを構築するためのミッションでコードを書いていると述べています。
So it's clear that his goal is super intelligence and in the article it states that Magic's co-founder and CEO Eric Steinberger has grappled with the problem of getting AI models to reason before.
彼の目標はスーパーインテリジェンスであり、記事では、Magicの共同創設者兼CEOであるエリック・スタインバーガーが以前からAIモデルが推論する問題に取り組んできたことが述べられています。
He previously worked at Matter Platforms conducting research on how reinforcement learning, the machine learning techniques that help the pretty much the great performance of open eyes LLMs can help AI models find the optimal solutions to problems even with imperfect information.
以前、彼はMatter Platformsで働いており、強化学習という機械学習技術が、OpenAIの大規模言語モデルの優れたパフォーマンスを支援する方法について研究していました。これにより、AIモデルが不完全な情報でも問題の最適な解決策を見つけるのに役立ちます。
And his ambition is bigger than a coding co-worker.
彼の野望は、コーディングの同僚よりも大きいです。
Remember this company's goal, okay, is to develop AI super intelligence the same way that Google do, and that is the kicker, guys.
この会社の目標は、Googleと同じようにAIスーパーインテリジェンスを開発することです。それが重要な点です。
So the fact that they've made a breakthrough that's very similar to Q-Star and the fact that they are working on super intelligence is pretty, pretty incredible because I think the fact that they're both heading in the same direction means that they're eventually going to stumble across the same roadblocks and eventually they're going to get across some of the same roadblocks that they do now.
Q-Starに非常に似ているブレークスルーを達成した事実、そしてスーパーインテリジェンスに取り組んでいる事実は、非常に驚くべきことです。彼らが両方が同じ方向に向かっているという事実は、最終的に同じ障害にぶつかることになるということを意味していると思います。そして、最終的には、彼らが今抱えている同じ障害を乗り越えることになるでしょう。
This has some real, real ramifications and one of the things I do want to know is, of course, what is the product because essentially they say some of Freedman's former colleagues at GitHub have joined him at Magic and they include Max Shoning, Vice President of Design at GitHub, as well as some other GitHub designers.
これには実際の影響があり、私が知りたいことの1つは、製品が何かということです。基本的に、Freedmanの元同僚のいくつかがMagicに参加しており、その中にはGitHubのデザイン担当副社長であるMax Shoningや他のGitHubデザイナーも含まれています。
According to a person with knowledge of the hires, they'll likely be crucial to developing the company's first commercially available product, which I'm hearing is to be set to released in a next few months.
採用情報に詳しい人によると、彼らはおそらく会社の最初の商業製品の開発に重要な役割を果たすことになるでしょう。それは数ヶ月以内にリリースされる予定だと聞いています。
So I'm guessing that potentially what we're going to be having is something that blows I'm guessing GitHub Copilot out of the water.
私はおそらく、私たちが手に入れる可能性があるものは、GitHub Copilotを凌駕するものだと思います。
And think about it like this, guys.
考えてみてください、皆さん。
If these guys, okay, actually let's say, for example, these guys actually did this, okay.
もしもこれらの人たちが、例えば、実際にこれをやったとしたら、ということを考えてみてください。
So they have something that has active reasoning, which is similar to Q-Star.
彼らはQ-Starに似たアクティブな推論を持っているということです。
Remember that OpenAI, there was this whole debacle about Q-Star, some moment getting fired, which we're going to dive into in a moment.
OpenAIについて覚えているでしょうか、Q-Starに関する騒動がありましたね、ある瞬間に誰かが解雇されたことがありましたが、それについて詳しく説明していきます。
But if they have something that has active reasoning, something that has an active unlimited context window, something that dwarfs Google's Gemini 1.5 Pro, and let's say it's so good.
しかし、もし彼らがアクティブな推論を持つ何かを持っていて、アクティブな無制限のコンテキストウィンドウを持つ何かを持っていて、GoogleのGemini 1.5 Proを凌駕する何かを持っていて、それが非常に優れているとすると。
And these guys, they said it's better than our evals, anything that they've tried before.
そして、これらの人たちは、それが私たちの評価よりも優れていると言っています。
I think that if they release that product and that product is better than GitHub's Copilot, which I think potentially it's going to be, I think that we have a situation on our hands.
もし彼らがその製品をリリースし、その製品がGitHubのCopilotよりも優れているとしたら、それはおそらくそうなると思いますが、私たちには問題が発生すると思います。
Because if that product just blows out of the water, remember, GitHub is backed by Microsoft.
なぜなら、もしもその製品が大成功を収めた場合、GitHubはマイクロソフトの支援を受けていることを覚えておいてください。
I'm guessing that it's running using ChatGPT.
私はChatGPTを使用していると推測しています。
We're going to have a problem because what will happen is these guys will release their product, they're going to take the industry by storm.
私たちは問題を抱えることになるでしょう、なぜなら、これらの人たちは彼らの製品をリリースし、業界を席巻することになるからです。
and then, OpenAI are probably going to release GPT-5 or maybe an even advanced version because well they don't want to lose the race because everyone knows about ChatGPT.
そして、その後、OpenAIはおそらくGPT-5をリリースするか、さらに高度なバージョンをリリースするでしょう。なぜなら、彼らはレースに負けたくないからです。誰もがChatGPTについて知っていますから。
And if these guys are developing their own proprietary front-end model which they stated that they will, remember they stated they're going to release their own proprietary model. Remember, their goal is to build super intelligence.
もしもこれらの人々が独自のフロントエンドモデルを開発しているとしたら、彼らが独自のモデルをリリースすると述べていたことを覚えておいてください。彼らの目標は、スーパーインテリジェンスを構築することです。
It's not just a code, you know buddy.
それはただのコードではないのですよ、友よ。
Okay, it says you know, to build a great product we need to train our own front-end scale model and transformers aren't the final architecture.
すごい製品を作るためには、自分たちのフロントエンドのスケールモデルを訓練する必要があり、トランスフォーマーは最終的なアーキテクチャではないと言っています。
We have something with a multi-million context window now, apparently has active reasoning which means, okay, that the race could be on guys.
今、マルチミリオンのコンテキストウィンドウを持つものがあり、明らかにアクティブな推論を持っているということは、レースが始まる可能性があるということです。
The race could be on, this could be an insane race.
レースが始まるかもしれません、これは狂気じみたレースになるかもしれません。
Okay, and this is why I'm stating that this could be absolutely incredible.
よし、そしてこれが私が述べている理由で、これは本当に信じられないほど素晴らしいかもしれません。
Now, if you want to remember, Q-Star was pretty crazy because the day that you know Sam Altman was fired, okay, he alluded to a technical advance the company has made that allowed it to push the veil of ignorance back and the frontier of discovery forward.
覚えておきたいのは、Q-Starはかなりクレイジーだったということです。なぜなら、サム・アルトマンが解雇された日、会社が行った技術的な進歩をほのめかし、無知のベールを後退させ、発見のフロンティアを前進させることができたと述べていました。
Okay, and there was an interview that he said that.
そして彼がそのことを言ったインタビューがありました。
And of course, Q-Star essentially OpenAI made a breakthrough before Altman firing stoking the excitement and concern.
そしてもちろん、Q-Starは基本的にOpenAIがアルトマンの解雇前にブレークスルーを達成し、興奮と懸念を煽った。
I know that a lot of people were essentially wondering if this leak was true, but essentially it was because Sam Altman did comment on it himself.
多くの人々がこのリークが本当かどうか疑問に思っていたことを知っていますが、実際にはサム・アルトマン自身がそれにコメントしたためです。
And I think the fact that people forget that OpenAI has 702 employees, apparently it's actually 770 that signed the letter.
そして、OpenAIが702人の従業員を抱えていることを人々が忘れていると思いますが、実際には署名したのは770人だと言われています。
I think it was around 740 that did sign the letter.
おそらく署名したのはおよそ740人だったと思います。
And when you think about a company of that size, I don't think it's impossible to or implausible for two people to essentially go to the board and say, Look, this is crazy.
そのような規模の会社を考えると、2人が基本的に取締役会に行って、これは狂っていると言うことは不可能ではないと思います。
Because something that you might not know is that, I'm guessing that now OpenAI is compartmentalized because when they released Sora, I'm not even sure that the entire company knew about it.
なぜなら、あなたが知らないかもしれないことは、今OpenAIが区画化されていると推測しているからです。Soraをリリースしたとき、会社全体がそれを知っていたかどうかさえわかりません。
Okay, because I remember some of the employees tweeting about it, saying that, Wow, I saw some of the demos from Sora today.
なぜなら、私は従業員の一部がツイートしていたのを覚えています。今日、Soraのデモの一部を見ました、と言っていました。
This thing is absolutely incredible.
このものは本当に信じられないほどです。
So really and truly, it could be something.
本当に、これは何かになるかもしれません。
Some people are saying that how come we didn't get Q-Star leaked by the entire OpenAI team?
OpenAIチーム全体によるQ-Starリークが得られなかったのはなぜだろうと言っている人もいます。
Guys, OpenAI is compartmentalized, which essentially means that an organization where pieces of information are separated to prevent leaks is typically to refer to as employing a compartmentalization strategy.
みなさん、OpenAIは区分されています。つまり、情報が漏れるのを防ぐために情報が分離される組織は通常、区分化戦略を採用していると言われています。
And essentially what this approach is, is it involves dividing up the organization into discreet sections or compartments where information is tightly controlled and only shared on a need-to-know basis.
そして、このアプローチの本質は、組織を分割して、情報が厳密に管理され、必要に応じてのみ共有される離散的なセクションや区画に分割することです。
And it's kind of used, unlike the military and of course intelligence agencies and some corporate environments, to enhance security and of course to protect sensitive information.
そして、それは、軍事や情報機関、そして一部の企業環境とは異なり、セキュリティを強化し、もちろん機密情報を保護するために使用されています。
Of course, with what we're doing now, all these kind of breakthroughs need to be protected.
もちろん、私たちが今行っていることでは、すべてのこのようなブレークスルーは保護される必要があります。
So I'm not surprised that OpenAI would have such a strategy because it does make sense.
OpenAIがそのような戦略を持っているのは驚きではありません。
And like I said, if they're doing that, okay, then I'm guessing that the leaks are definitely possible because it's a giant company.
そして私が言ったように、もしそれをやっているなら、リークは確実に可能だと思います。なぜならそれは巨大な企業だからです。
Okay we don't really know who it's going to be.
誰がそれになるかわかりません。
And even if they are compartmentalized, okay, and maybe it's like, it's just 100 people or 50 people or whatever, you're still not going to know who exactly did the leaks or whatever.
たとえそれらが区分けされているとしても、わかっているのは、たとえば100人や50人だろうと、漏洩をしたのが具体的に誰なのかはわからないということです。
So, I think that Q-Star is of course really crazy because I think it's kind of shocking that the company that's trying to work on super intelligence, of course, OpenAI are trying to additionally work on super intelligence.
だから、Q-Starはもちろん本当に驚くべきことだと思います。なぜなら、スーパーインテリジェンスに取り組んでいる企業であるOpenAIがさらにスーパーインテリジェンスに取り組もうとしているからです。
They may have made some real, real strides in there.
彼らはそこで本当に大きな進歩を遂げたかもしれません。
And essentially, of course, it's here an innovation by the company's researchers earlier this year that would allow them to develop far more powerful AI models.
そして、もちろん、今年初めに会社の研究者によって行われた革新が、彼らにより強力なAIモデルを開発することを可能にするものでした。
And of course, concerns among some staff that the company didn't have proper safeguards in place to commercialize such advanced AI models.
そして、もちろん、一部のスタッフの間で、会社がこのような高度なAIモデルを商品化するための適切な保護策を持っていないという懸念がありました。
This person said, so of course, this Q-Star innovation essentially that was able to solve math problems it hadn't seen before, which is an important technical milestone, is something that will change the game whenever it does come into it.
この人は言いました、もちろん、このQ-Starイノベーションは、これまで見たことのない数学問題を解決できるようになり、重要な技術的なマイルストーンであり、それが導入されるとゲームを変えるものになるでしょう。
Now, of course, there was some more bits on Q-Star.
さて、Q-Starに関するさらなる情報がありました。
And then I'm going to get onto a really, really, really big issue that I don't think enough people are talking about.
それから、私は本当に、本当に、本当に重要な問題に取り組む予定ですが、それについて話している人がまだ十分ではないと思います。
And whilst these developments are good, of course, there is a lot of stuff that is unfortunately quite bad.
これらの進展は良いですが、もちろん、残念ながら悪いこともたくさんあります。
Okay, so essentially, remember, okay, that openly I said that while super intelligence seems far off now, we believe that it could arrive this decade.
では、基本的に、覚えておいてください、私が公言したように、現在はスーパーインテリジェンスは遠いように見えますが、私たちはこの10年に到来する可能性があると信じています。
And that means that we could get ASI by 2030.
つまり、2030年までにASIを手に入れることができるかもしれません。
So that could be like, I mean the fact that OpenAI is saying that super intelligence seems far, but it could arrive this decade, it's not surprised because apparently some people have stated that once you get AGI, it's not far before you get ASI.
OpenAIがスーパーインテリジェンスは遠いように見えるが、この10年に到来する可能性があると述べていることは驚くべきことではない、ということです。なぜなら、明らかに一部の人々が、AGIを手に入れるとすぐにASIを手に入れることは遠くないと述べているからです。
So in addition, there was also this as well that earlier this year, Sutskever and his team discovered a variation of that method that prompted greater results in their efforts to train more sophisticated models.
さらに、今年初めに、サツキヴァーと彼のチームが、より洗練されたモデルを訓練するための努力において、より大きな結果をもたらすその方法の変種を発見したということもありました。
And of course, essentially, OpenAI is dedicating a fifth of its compute to solving super intelligence.
そしてもちろん、基本的に、OpenAIはスーパーインテリジェンスの解決にその計算の5分の1を捧げています。
And essentially, one last thing that I'm gonna cover is something that I want to talk to you guys about because this is really, really important and not enough people are talking about it.
そして基本的に、私がカバーする最後のことは、私が皆さんに話したいと思っているものです。なぜなら、これは本当に、本当に重要で、十分に話されていないからです。
And essentially, there is this Moloch concept, okay, and Moloch has come to signify a condition in which we humans are coerced to make futile efforts and compete with each other in such ways that we are eventually driven to our demise.
基本的に、このMolochという概念があります。Molochは、私たち人間が無駄な努力を強いられ、お互いと競い合うような状況を意味するようになってきました。
And this is really true.
そして、これは本当です。
And if you think I'm just adding this in the video, I'm just for the sake of it, trust me, it's not.
もしこれをただ動画に追加しているだけだと思っているなら、それは違いますよ、信じてください。
You guys are gonna want to see this because this could spell disaster.
みなさんはこれを見たいと思うでしょう、なぜならこれが災害を招く可能性があるからです。
So essentially, Liv Boor, I'm not exactly sure how you say your name, but she actually did a recent TED talk about the Moloch problem.
基本的に、Liv Boor、あなたの名前の発音が正確にはわかりませんが、彼女は実際に最近、Moloch問題についてのTEDトークを行いました。
And it's a really big problem that's only going to get worse as things go on because as systems become more powerful, we need more security.
これは本当に大きな問題で、システムがより強力になるにつれて、より多くのセキュリティが必要になります。
But of course, as they become more powerful, people will be deploying them even more.
しかしもちろん、システムがより強力になると、人々はそれらをさらに展開するでしょう。
So I'm going to show you guys a clip from this TED talk because it's actually really important to understand this issue because if you don't, and I know some people are good, happy about AI innovation, the existential risk is there.
だから、このTEDトークからクリップを見せるつもりです。なぜなら、この問題を理解することが実際に非常に重要だからです。AI革新について幸せな人もいるとは思いますが、存在リスクは存在しています。
Like literally, like 40% of AI researchers say that we should slow down with AI research.
文字通り、AI研究者の40%がAI研究を遅らせるべきだと言っています。
And of course, that's because of the, clear thing that super intelligence poses a really, really bad risk.
そしてもちろん、それは、超知能が実際に非常に悪いリスクをもたらすという明確な理由です。
So I'm going to show you guys the clip.
だから、このクリップを見せますね。
Those influencers are sacrificing their happiness for likes.
それらのインフルエンサーは、いいねのために自分の幸福を犠牲にしています。
Those news editors are sacrificing their integrity for clicks.
それらのニュース編集者は、クリックのために自分の信頼性を犠牲にしています。
And polluters are sacrificing the biosphere for profit.
そして、汚染者は利益のために生物圏を犠牲にしています。
In all these examples, the short-term incentives of the games themselves are pushing, they're tempting their players to sacrifice more and more of their future, trapping them in a death spiral where they all lose in the end.
これらすべての例で、ゲーム自体の短期的なインセンティブがプレイヤーを誘惑し、彼らを将来のさらなる犠牲を強い、彼らを最終的に全員が負ける状況に閉じ込めてしまう。
That's Moloch's trap, the mechanism of unhealthy competition.
それがMolochの罠であり、健全でない競争のメカニズムです。
And the same is now happening in the AI industry.
そして、同じことが今、AI産業でも起こっています。
We're all aware of the race that's heating up between companies right now over who can score the most compute who can get the biggest funding round or get the top talent.
今、企業間で最も計算を得点できるか、最大の資金調達ラウンドを得るか、トップの人材を獲得できるかという競争が激化していることに私たちは皆気づいています。
Well, as more and more companies enter this race, the greater the pressure for everyone to go as fast as possible and sacrifice other important stuff like safety testing.
さて、ますます多くの企業がこの競争に参入するにつれて、全員ができるだけ速く進むことと安全テストなどの他の重要なことを犠牲にする圧力が増しています。
This has all the haLLMarks of a Moloch trap because like imagine you're a CEO who in your heart of hearts believe that your team is the best to be able to safely build extremely powerful AI.
これはすべてMolochの罠の特徴を持っています。CEOであると想像してください。あなたは心の底から、あなたのチームが非常に強力なAIを安全に構築できると信じています。
Well, if you go too slowly, then you run the risk of other much less cautious teams getting there first and deploying their systems before you can.
もし速度を上げすぎると、より慎重でない他のチームが先に到達し、あなたがそれを展開する前に彼らのシステムを展開するリスクがあります。
So that in turn pushes you to be more reckless yourself.
それによって、あなた自身がより無謀になることを促します。
And given how many different experts and researchers both within these companies but also completely independent ones have been warning us about the extreme risks of rushed AI, this approach is absolutely mad.
そして、これらの企業内だけでなく、完全に独立した専門家や研究者が、急いでAIを進めることの極端なリスクについて警告していることを考えると、このアプローチはまったく狂っています。
Plus, almost all AI companies are beholden to satisfying their investors, a short-term incentive which over time will inevitably start to conflict with any benevolent mission.
さらに、ほとんどすべてのAI企業は、投資家を満足させることに縛られており、時間の経過とともに善意の使命と衝突するようになる短期的なインセンティブがあります。
And this wouldn't be a big deal if this was really just toasters we're talking about here, but AI and especially AGI is said to be a bigger paradigm shift than the agricultural or industrial revolutions, a moment in time so pivotal it's deserving of reverence and reflection, not something to be reduced to a corporate rat race of who can score the most daily active users.
これは、本当にトースターについて話しているのであれば、大したことではありませんが、AI、特にAGIは、農業革命や産業革命よりも大きなパラダイムシフトだと言われており、崇敬と反省に値するほどの時期であり、誰が最も多くのデイリーアクティブユーザーを獲得できるかの企業の競争に縮小されるべきではないものです。
I'm not saying I know what the right trade-off between acceleration and safety is, but I do know that we'll never find out what that right trade-off is if we let Moloch dictate it for us.
私は加速と安全の間の正しいトレードオフを知っているわけではありませんが、Molochにそれを我々のために決定させてしまうと、その正しいトレードオフがわからなくなることは絶対にありません。
So that clip there essentially, they're talking about the problem of how things are just developing too quickly.
そのクリップは、物事があまりにも速く進展している問題について話しています。
And I think this goes to show just like how I talked about why this is such a serious issue because this smaller company is essentially if they've pretty much got the same kind of Q-Star technology that OpenAI had, I think about it like OpenAI, okay, maybe unlike GPT-4, they safety tested GPT-4 for six months, okay, maybe with this AGI level system, they're gonna have to safety test it for a year and a half, okay, but what if this other AI system like they said, they said that they're deploying their system within a couple of months, is that gonna lead OpenAI to essentially you know, just forget about the guardrails essentially.
そして、これはなぜこれが非常に深刻な問題であるかについて話したように、この小さな企業は基本的にOpenAIが持っていたのと同じ種類のQ-Starテクノロジーを持っている場合、OpenAIがGPT-4を安全にテストしたのと同じくらい、このAGIレベルのシステムを1年半安全にテストする必要があるかもしれません。しかし、彼らが言ったように、この他のAIシステムが数ヶ月以内にシステムを展開すると、OpenAIは本質的にガードレールを忘れることになるのではないかと考えます。
and then deploy their system.
そして彼らはそのシステムを展開します。
Are we going to have some major ramifications of that in the future?
その将来的な影響は大きいでしょうか?
So essentially, it's a problem of, I guess you could kind of say a race to the bottom.
基本的に、これは、言ってしまえば、底辺への競争の問題です。
And this chart here is from arc investment management, and they've talked about how every year, like these breakthroughs just it's like a stock just keeps dropping until we get to AGI.
このチャートはアーク・インベストメント・マネジメントからのもので、彼らは毎年、これらのブレークスルーが起こると、まるで株価がAGIに達するまで下がり続けるような話をしています。
And you can see right here that gbt3, it went from 50 years all the way down to like 40.
そしてここで見ているように、GBT3は50年から40年まで一気に下がりました。
Then you can see google's advanced conversational agent lambda 2, boom, we got down to 18 years.
それから、Googleの高度な対話エージェントであるLambda 2を見ることができます。突然、18年になりました。
ChatGPT, boom, went down again.
ChatGPTは、突然、再び下がりました。
GPT-4 launches, boom, now it's eight years.
GPT-4が始動し、突然、8年になりました。
Like with this Q-Star breakthrough, are we going to be just dropped down again, boom, like are we going to be like four years away?
このQ-Starのブレークスルーによって、再び突き落とされることになるのでしょうか、突然、まるで4年前に戻されるような感じになるのでしょうか?
You can see that if the forecast error continues that, like like the forecast that they had, it was like, okay, we're going to be getting there by 2030.
予測誤差が続くと、2030年までにそこに到達するという予測のようになることがわかります。
But like the forecast is basically saying that with all these kind of breakthroughs.
しかし、予測は基本的に、これらの種類のブレークスルーによって言っていることです。
And you remember that ChatGPT was like, I wish I could like show you guys how much more AI stuff went on here because there has just been so much more engagement in terms of the AI uptick.
そして、ChatGPTが言ったように、ここでAIの進展がどれだけあったかを見せたいと思っていました。AIの活況が非常に高まっているため、エンゲージメントが大幅に増加しています。
So right here is like an acceleration point.
ここでのポイントは加速点のようです。
So we could argue that this is going to come straight down, which means that's this year.
これはまっすぐに降りてくると主張できます。つまり、それが今年です。
If you actually think about it, guys, like if this point right here is an exponentially growth, like that's going to come down into this year, like, you know what I mean?
実際に考えてみると、皆さん、このポイントが指数関数的な成長であるとしたら、それが今年に降りてくるということは、わかりますよね?
So that's something that's not surprising.
それは驚くべきことではないですね。
And if their forecast error continues, which to be honest, humans are very bad at extrapolating exponential growth, I wouldn't be surprised if, of course in 2025, something crazy happens.
もし彼らの予測誤差が続くと、正直言って、人間は指数関数的な成長を予測するのが非常に下手なので、2025年に何か狂ったことが起こっても驚かないでしょう。
So I mean, with all of these companies going on, and I think something that you guys do want to know as well, I mean, that's kind of weird, but OpenAI did actually talk about this.
私は言いたいのは、これらの企業が進んでいる中で、皆さんが知りたいと思うこともあると思います、それは少し変わっていますが、OpenAIは実際にこれについて話していました。
They actually said that, we, we essentially won't get super intelligence in, in a sense.
彼らは実際に言ったのですが、我々は実質的には超知能を手に入れることはないだろうと。
and of course we kind of will, but what they said about their safety testing thing, they basically said that we specify four safety risk levels and only models with a post mitigation score of medium or below can be deployed.
もちろん、我々はある程度手に入れることになるだろうが、彼らが安全テストについて言ったことは、基本的に、我々は4つの安全リスクレベルを特定し、中程度以下のポスト緩和スコアを持つモデルのみが展開されると述べていた。
Essentially what they mean is that only a model that's able to perform at a certain level is going to be deployed.
基本的に彼らが言っていることは、ある特定のレベルで機能するモデルのみが展開されるということです。
And if they have a system that they believe is too smart, they're just not going to put that into the wild.
もし彼らがあまりにも賢いと考えるシステムを持っている場合、それを実際に使うことはしないでしょう。
But this is the thing, this is OpenAI's safety square, this is their safety mitigation.
しかし、これがポイントなんです。これがOpenAIの安全性の四角形であり、これが彼らの安全性の緩和策です。
What are other companies going to do?
他の企業はどうするつもりですか?
Are the other companies going to abide by this?
他の企業はこれに従うつもりですか?
and if they do, I mean, you know what's going to happen to the world.
もし彼らが従うなら、世界で何が起こるかわかりますよね。
I mean, it's going to be a crazy place to be living in because so far they're basically saying that AGI by 2026 and now they're in 2024.
つまり、住んでいる場所が狂った場所になることでしょう。これまでに、2026年までにAGIと言っていたのに、今は2024年です。
That's a year and a half away.
あと1年半しかありません。
And I remember when people are stating that AGI in 18 months is crazy.
私は、18ヶ月でAGIが実現すると言っていた人々が狂っていると思っていたことを覚えています。
Now it's seeming like it's realistic.
今では、それが現実的に思えるようになってきました。
So I mean, with the amount of investment going into these companies, with the amount of things that are happening with Q-Star breakthroughs only a couple months ago, with active reasoning capabilities apparently recently discovered, with this huge context length just being there, with new architectures popping up, and with these massive investments, and these guys saying that this is better than anything we've seen before, I have no idea what they've developed.
これらの企業に投資されている金額、数ヶ月前にしか起こらなかったQ-Starのブレークスルー、最近発見されたアクティブな推論能力、巨大なコンテキスト長が存在すること、新しいアーキテクチャが現れていること、そしてこれらの大規模な投資、そしてこれらの人々がこれまでに見たものよりも優れていると言っていることを考えると、彼らが何を開発したのか全くわかりません。
But either way, I'm excited, scared, frightened.
とにかく、私は興奮していますし、怖いし、恐ろしいです。
I mean, so many words to describe such groundbreaking technology.
つまり、この画期的な技術を表現するためにはたくさんの言葉があります。
And I'm excited to see it involved.
そして、それが関わっているのを見るのが楽しみです。
So what do you guys think about this?
では、皆さんはこれについてどう思いますか?
Do you guys think this is crazy?
皆さんはこれを狂ったことだと思いますか?
Do you think it's boring?
それはつまらないと思いますか?
Do you think this is a good update?
これは良いアップデートだと思いますか?
Let me know what you guys think.
皆さんの意見を教えてください。
This has been an insane February, and I'll see you guys for another update tomorrow.
これは狂ったような2月でしたが、明日もまたアップデートします。
この記事が気に入ったらサポートをしてみませんか?