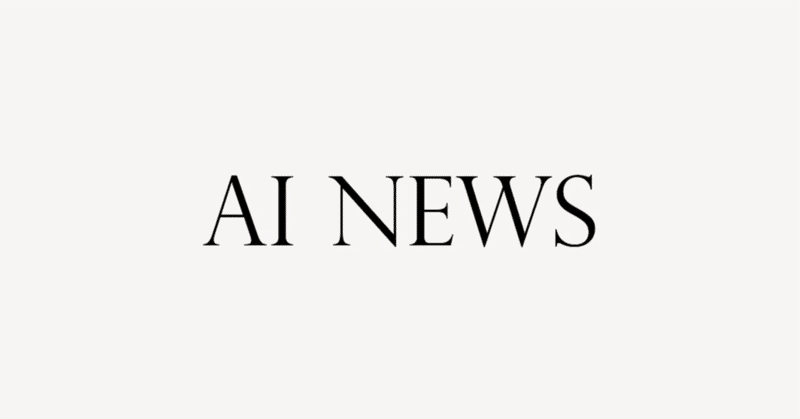
【AIによる革命的な画像・動画生成技術】英語解説を日本語で読む【2023年10月27日|@Matt Wolfe】
この動画では、AIを使った画像・動画生成技術を紹介しています。
公開日:2023年10月27日
※動画を再生してから読むのがオススメです。
So, I have a habit of collecting really cool AI research and tools that I come across and building it up for weeks and weeks and weeks, and then making a video like this to share all of the cool stuff that I've come across over the last several weeks.
私は、出会った本当にクールなAIの研究やツールを集めて、何週間も何週間も積み上げて、このようなビデオを作って、ここ数週間で出会ったすべてのクールなものを共有する習慣があります。
Some of the stuff is recently released research where we can use demos on places like Hugging Face or Google Collab, and some of it is research that the creators are kind of showing off what it can do, but we don't quite have access to it yet.
その中には、Hugging FaceやGoogle Collabのような場所でデモを使うことができる、最近リリースされた研究もあれば、クリエイターがどんなことができるかを披露している研究もある。
I love making videos like this because this is literally the future of AI and visual effects, and I love these little sneak peeks into where everything is going.
私はこのようなビデオを作るのが大好きです。なぜなら、これは文字通りAIと視覚効果の未来であり、私はこのような小さな覗き見が大好きなのです。
So, let's start with image generation.
では、画像生成から始めましょう。
Now, I'm personally in this AI bubble when I'm on Twitter or X or whatever, and lately I've been seeing a ton of this 0123 plus, which essentially is an AI where you can upload a single image.
私はTwitterやXなどでこのAIのバブルにいるのですが、最近はこの0123プラスというものをたくさん見かけます。これは、単一の画像をアップロードできるAIです。
For example, this fire extinguisher.
たとえば、この消火器です。
From this single image, it will actually show you what that image would look like from multiple other angles.
この1枚の画像から、その画像が他の複数の角度からどのように見えるかを実際に表示してくれます。
Here's another example of an image with a ghost eating hamburgers, and you can see that same ghost eating hamburgers from multiple angles and viewpoints.
これはハンバーガーを食べている幽霊の画像で、同じ幽霊が複数の角度や視点からハンバーガーを食べている様子を見ることができます。
I have this headshot image of myself on my desktop.
私のデスクトップにはこの自分の顔写真がある。
I'm kind of curious to see what happens when I pull this in.
これを取り込むとどうなるか、ちょっと興味がある。
We'll drag and drop it right into this Hugging Face demo.
このHugging Faceのデモにドラッグ・アンド・ドロップしよう。
Here, submit it, and 15 seconds later, I have my uh, face from all sorts of different angles.
送信して15秒後、いろいろな角度から見た私の顔ができあがります。
I uploaded this cool AI-generated wolf image, and I've got different angles on this, but it seems to struggle a little bit with the wider aspect ratio of the image because it kind of cut off the wolf in all the images.
AIが生成したクールなオオカミの画像をアップロードして、いろいろなアングルから撮ったんだけど、画像の縦横比が広いとちょっと苦労するみたいで、すべての画像でオオカミが切れてしまうんだ。
But pretty cool that you can upload a single image with one perspective and actually get a different image of all the various perspectives on that image.
しかし、1つのパースで1つの画像をアップロードし、その画像上のすべてのさまざまなパースの異なる画像を実際に得ることができるのはかなりクールだ。
Here's some more research out of Microsoft called idea to image.
マイクロソフトの "idea to image "と呼ばれる研究を紹介しよう。
The code isn't publicly available yet, but basically, it's going to let us do all sorts of cool stuff to generate images closer to what we're looking for.
コードはまだ公開されていませんが、基本的には、私たちが探しているものに近い画像を生成するために、あらゆる種類のクールなことができるようになっています。
So, some of the examples that they share here, they've got this object count five people sitting around a table drinking beer and eating buffalo wings.
ここで共有されている例の中には、ビールを飲みながらバッファローウィングを食べる5人がテーブルの周りに座っているオブジェクトがあります。
Not bad.
悪くない。
I actually think DALL·E 3 does that pretty well already.
実際、『DALL-E 3』はすでにかなりよくできていると思う。
A logo suitable for a stylish hotel, and it generated this logo here.
スタイリッシュなホテルにふさわしいロゴ、それがこのロゴを生み出した。
Here's another example, a photo of the object pointed by the blue arrow and a brown Corgi.
これは別の例で、青い矢印が指すオブジェクトと茶色のコーギーの写真です。
So, they uploaded an image of an arrow pointing at a ball, and it noticed that this was a ball and it put that same ball with a Corgi.
矢印がボールを指している画像をアップロードすると、これがボールであることに気づき、同じボールとコーギーを組み合わせました。
This one, they uploaded an image of somebody playing tennis here and gave the prompt a cartoon drawing of Mr. Bean playing tennis with the same clothes and pose as the given image.
こちらは、誰かがテニスをしている画像をアップロードし、与えられた画像と同じ服装とポーズでテニスをしているミスター・ビーンの漫画の絵をプロンプトに与えました。
You can see the result output over here on the far right, Mr. Bean wearing a yellow shirt with the same exact pose as the pose in this picture.
右端に出力された結果を見ることができる。ミスター・ビーンは黄色いシャツを着て、この写真のポーズとまったく同じポーズをとっている。
Here, image manipulation, a drawing with the background change to a beach.
ここでは、画像の操作が行われ、背景がビーチに変わっています。
So, it's this image here, but then they put a beach behind it in pretty much the same pose with the same person in it.
つまり、この画像ですが、その後ろに同じポーズで同じ人物がいるビーチを配置しています。
They were even able to put two images in here, a photo of Bill Gates with the same clothes as the given image with a dog that looks like this one in this image.
ここには2つの画像を入れることができました。ビル・ゲイツの写真と同じ服装をした犬の画像です。
You can see on the far right here, Bill Gates wearing a pretty similar suit and a dog next to Bill Gates that looks like this dog.
右端には、よく似たスーツを着たビル・ゲイツと、ビル・ゲイツの隣にいるこの犬に似た犬が写っている。
Just imagine how much more dialed in we can get with our images and get to the exact idea that we have in our mind using some of this stuff.
このようなものを使うことで、どれだけイメージにダイヤルを合わせることができ、頭の中にあるアイデアを正確に実現できるか、想像してみてほしい。
Check this one out, blending images for new visual design, a logo with a design that naturally blends the two given images as a new logo.
新しいビジュアルデザインのための画像のブレンド、与えられた2つの画像を新しいロゴとして自然にブレンドしたデザインのロゴをご覧ください。
The first image is this stethoscope with a paw, and the second image is this little pug image here, and look, it generated this logo of a pug with a stethoscope around it.
最初の画像は、このステトスコープに足跡がついたもので、2番目の画像はこの小さなパグの画像で、これらを使ってステトスコープをかけたパグのロゴが生成されました。
This one, to me, is really, really impressive.
これは私にとって、本当に本当に印象的です。
Again, not something that we have public access to yet.
繰り返しますが、まだ一般公開されているものではありません。
And if you want even more ideas of what this is capable of, here, there's all sorts of examples that they share down here that you can click through and see exactly what it's capable of.
もしこれがどんなことができるのかもっと知りたいなら、この下にある様々な例をクリックすれば、どんなことができるのか見ることができる。
Really, really killer stuff.
本当に、本当に素晴らしいものだ。
I'll make sure I link to this in the description below.
以下の説明の中で、必ずリンクを張っておこう。
I link to all the research that I'm sharing in this video, and it looks like Matt vid Pro also made a video on this exact research, so check that out for an even deeper dive into what this idea to image is capable of.
このビデオで共有しているすべての研究へのリンクを貼っています。また、Matt vid Proさんもこの研究についてのビデオを作っているようなので、さらに詳しく知りたい方はそちらもチェックしてください。
Next up, let's check out pixart Alpha fast training of diffusion Transformers for photorealistic text to image synthesis.
次は、フォトリアリスティックなテキストから画像への合成のための拡散トランスフォーマーの高速トレーニングpixart Alphaをチェックしましょう。
Some of these names just roll off the tongue.
これらの名前の中には、舌を巻くようなものもあります。
So first off, just look at some of the images that it generates.
まず最初に、生成される画像のいくつかを見てほしい。
Now, these are some really good images, right?
これらは本当に素晴らしい画像ですね。
You've got a real beautiful woman, Luffy from One Piece, a poster of a mean iCal cat, technical schematics viewed from front, nature versus human nature.
本物の美女、『ONE PIECE』のルフィ、意地悪なiCalの猫のポスター、正面から見た技術回路図、自然対人間。
In my mind, these are like mid Journey DALL·E three-level results here.
私の中では、これらはまるでミッドジャーニー『DALL-E』3レベルの出来栄えだ。
These look really, really good.
これらは本当に、本当に良さそうだ。
But we've already got DALL·E, and we've already got mid Journey, so what's so special about this?
しかし、私たちは既にDALL·Eやmid Journeyを持っていますので、これは何が特別なのでしょうか?
Well, they've managed to really optimize the training of this model.
彼らはこのモデルのトレーニングを最適化したんだ。
So if we scroll down here, the training of this is so much more efficient than what else is available out there.
ここを下にスクロールしてみると、このモデルのトレーニングは他のものよりもはるかに効率的です。
You can see the CO2 emissions of the various models that are available.
利用可能なさまざまなモデルのCO2排出量が表示されます。
DALL·E 2 to train it had the CO2 emissions of five humans.
DALL-E 2のトレーニングでは、人間5人分のCO2排出量でした。
Imagin had the CO2 emissions of 8 of a human.
イマジンは人間8人分のCO2排出量だった。
Stable diffusion 1.5.7.
Stable diffusion 1.5.7。
And then pixart Alpha.
そしてpixart Alpha。
07, and then the cost to actually train these models.
07、そしてこれらのモデルを実際に訓練するためのコスト。
DALL·E 2, it cost $2.14 million to train DALL·E 2.
DALL-E 2のトレーニングには214万ドルかかった。
Imagine $366,000 stable diffusion 1.5.
366,000ドルの安定した拡散1.5。
320,000 pixart Alpha 26,000.
320,000ドルのピクサート・アルファ26,000ドル。
So it's just a much more efficient model of training, but the results are on par with something you get out of a mid Journey sdxl or DALL·E 3.
つまり、より効率的なトレーニングモデルでありながら、その結果はミッドジャーニーのsdxlやDALL-E 3から得られるものと同等なのだ。
I really, really love the contrast in these images.
この画像のコントラストは本当に大好きだ。
They remind me of like something I'd get out of mid Journey.
まるで『ジャーニー』の中盤から得られるようなものを思い起こさせる。
It also works with control net, so you can upload extra references like reference images and the outlines and things like that to dial in the image just like you would in a normal stable diffusion generation.
コントロールネットとも連動しているので、通常の安定した拡散生成と同じように、リファレンス画像やアウトラインなどの追加リファレンスをアップロードして、画像を調整することができます。
It also works with dream Booth, which if you've watched my past videos, dream Booth allows you to train your own sort of objects or likeness into the model.
また、ドリームブースとも連動します。私の過去のビデオをご覧になったことがある方はわかると思いますが、ドリームブースでは、自分のオブジェクトや似顔絵をモデルにトレーニングすることができます。
So you can actually train your own face into this model if you wanted to or your own dog's face into this model.
ですから、実際に自分の顔をこのモデルにトレーニングしたり、自分の犬の顔をこのモデルにトレーニングしたりすることができます。
And there are some more samples on this page and they're really, really good.
このページには他にもいくつかサンプルがあり、とても素晴らしいです。
Now on their project page, they do have a Hugging Face demo button, but when I click on it, it doesn't appear to be an actual working demo yet.
彼らのプロジェクトページには、Hugging Faceのデモボタンがありますが、クリックしても、まだ実際に動いているデモではないようです。
Next up, check out hyper human hyper realistic human generation with latent structural diffusion.
次は、潜在構造拡散を使ったハイパー・ヒューマンハイパー・リアリスティック・ヒューマン・ジェネレーションをチェックしよう。
This is a model with one goal in mind, to make the most realistic looking humans possible.
これは、可能な限りリアルな人間を作るという一つの目標を持ったモデルだ。
You can see here a young kid stands before a birthday cake decorated with Captain America.
キャプテン・アメリカがデコレーションされたバースデーケーキの前に立つ幼い子供を見てください。
Look how realistic this person is.
この人物のリアルさを見てください。
A man who is sitting in a bus looking away from the window.
バスの中で窓から目をそらして座っている男性です。
You see an image like this on Instagram and somebody tells you it's a real photo, probably going to believe him.
インスタグラムでこのような画像を見て、誰かが本物の写真だと言ったら、おそらく信じてしまうだろう。
An older man is wearing a funny hat in his dining room.
食堂で変な帽子をかぶっている年配の男性。
Man sitting on brick covered ground appearing dirty and tired.
レンガで覆われた地面に座り、汚れて疲れているように見える男性。
They've got some other examples down here that compare it to some other models.
ここには他のいくつかの例もありますが、他のモデルと比較しています。
If we take a peek here, you can see we've got a man riding skis down the side of a snow covered ski slope.
ここをのぞいてみると、雪で覆われたスキースロープの側をスキーで滑る男性が見えます。
And this is what it generated, super realistic compared to all these other ones that are here.
そして、これが生成されたもので、ここにある他のすべてのものと比べて超リアルです。
A pedestrian walks down the snowy street with an umbrella, ultra realistic.
歩行者が傘をさして雪の降る通りを歩いています。超リアルです。
Something going on funky with his left leg compared to his right leg, but compared to these other images here, pretty dang realistic.
彼の左足は右足と比べて何か変なことが起こっていますが、他の画像と比べると、かなりリアルです。
The sdxl one's pretty dang good though.
でもsdxlの方はかなりいい。
And then finally, a man riding on top of a brown horse, while wearing a hat.
そして最後に、帽子をかぶり、茶色の馬の上に乗っている男。
This is their generation compared to these other models.
これは他のモデルと比較した彼らの世代だ。
SDXL is pretty decent, but the realism on this model is so dang good.
SDXLはかなりまともだけど、このモデルのリアリズムはすごくいい。
So, if you look at this image, the skateboard is all jacked up, but the person on the skateboard looks pretty damn realistic.
だから、この画像を見ると、スケートボードはジャッキアップしているけど、スケートボードに乗っている人はかなりリアルに見える。
But compared to these other models, Stable Diffusion 2.1, like what's going on?
でも、他のモデルと比べると、Stable Diffusion 2.1は、どうなっているんだ?
This person's arm is as long as their entire body.
この人の腕は体全体と同じくらい長い。
Deep Floyd just lacks the detail.
ディープ・フロイドはディテールが足りない。
SDXL, this person's got three legs.
SDXL、この人の足は3本だ。
Human SD, like this person looks like they got ran over by a car.
Human SD、この人は車にひかれたみたいだ。
That's just the image generation Tech that I wanted to show you.
これが私がお見せしたかったイメージ・ジェネレーション・テックです。
I've got so much more cool stuff to show you here.
まだまだお見せしたいものがたくさんあります。
Around text to video, text to 3D World, text to 3D objects.
テキストからビデオへ、テキストから3Dワールドへ、テキストから3Dオブジェクトへ。
Before I do, I want to quickly tell you about today's sponsor, which is Wirestock.
その前に、本日のスポンサーであるWirestockについて簡単にご紹介したいと思います。
You can learn more about Wirestock over at wirestock.io.
Wirestockについては、wirestock.ioをご覧ください。
But if you're not familiar with them, they are a platform where you can upload your images or photographs, and they will distribute them to all of the stock photo websites for you.
Wirestock.ioでWirestockについて詳しく知ることができます。Wirestockは、あなたが画像や写真をアップロードすると、それをすべてのストックフォトサイトに配信してくれるプラットフォームです。
And many of the stock photo websites now allow you to sell AI generated images as well.
多くのストックフォトサイトでは、AIが生成した画像も販売できるようになっている。
Sites like Adobe Stock, 123 RF, Dreamstime, and Freepik all allow AI generated images.
Adobe Stock、123 RF、Dreamstime、Freepikなどのサイトでは、AIで生成した画像を販売することができます。
So, you can generate your AI art, upload it to Wirestock, and it will distribute it to all of these sites for you.
つまり、AIアートを生成してWirestockにアップロードすれば、Wirestockがこれらのサイトすべてに配布してくれるのです。
Write a description, write a title, add the tags for you, and let those sites know that it is AI generated so that you're in compliance with their rules.
説明文を書き、タイトルを書き、タグを追加し、AIで生成された画像であることをこれらのサイトに知らせることで、各サイトのルールに準拠することができます。
And you don't have to do anything but upload the image.
あなたは画像をアップロードする以外、何もする必要はない。
In fact, one other really cool feature of Wirestock is you don't even need a tool like Midjourney or Stable Diffusion or DALL·E. You can now generate AI art directly inside of Wirestock by clicking on this generate button at the top.
実際、Wirestockのもう一つの素晴らしい機能は、MidjourneyやStable Diffusion、DALL-Eのようなツールさえ必要ないということです。上部にある生成ボタンをクリックすると、Wirestock内で直接AIアートを生成できます。
And you can generate your own images.
また、独自の画像を生成することもできます。
You can upload an existing image and reimagine it.
既存の画像をアップロードして、それを再構築することもできます。
You can upload multiple images and mix the images together.
複数の画像をアップロードして、画像をミックスすることもできます。
And they just added a brand new feature where you can actually change the face on an image.
さらに、実際に画像の顔を変えることができる新しい機能が追加されました。
So, if I click into this image, for example, you can see there's now a button down at the bottom that says Change face.
例えば、この画像をクリックすると、下の方にChange faceというボタンがあるのがわかると思います。
I can click that, click on Upload a new face, pull an image of my own headshot in here, and now if I click Apply, it converted the man at the computer to me at the computer.
それをクリックして、新しい顔をアップロードをクリックし、自分の顔写真をここに取り込み、適用をクリックすると、コンピューターにいる男性がコンピューターにいる私に変換されます。
It's a pretty cool new feature that lets you even further dial in the images that you're looking to create before sending them to the stock photo sites.
これはかなりクールな新機能で、ストックフォトサイトに送る前に、作成したいイメージをさらに細かく設定することができる。
Now, this Reface feature is a premium feature, but if you use the coupon code Matt20 at checkout, you get 20% off a premium membership of Wirestock.
さて、このReface機能はプレミアム機能ですが、チェックアウト時にクーポンコードMatt20を使用すると、Wirestockのプレミアムメンバーシップが20%オフになります。
You can find it all over at wirestock.io.
詳しくはwirestock.ioをご覧ください。
Once again, if you do decide to upgrade to the premium account, use the coupon code Matt20.
プレミアムアカウントにアップグレードする場合は、クーポンコード「Matt20」をご利用ください。
Thank you so much to Wirestock for sponsoring this video.
このビデオをスポンサーしてくれたWirestockに感謝します。
I do really appreciate you guys.
本当に感謝しています。
Here's something I came across on Twitter that I thought was pretty cool from Jared Lou.
ツイッターで見つけたジャレッド・ルーのコメントで、とてもクールだと思ったものがある。
He says that the latest version of Adobe Express now has the ability to create character animations from your voice.
最新版のAdobe Expressでは、声からキャラクターアニメーションを作成できるようになったそうです。
Now, he says this is voice to AI character animation, but I couldn't actually confirm that this was using AI, so I'm not 100% sure.
彼が言うには、これは声からAIを使ったキャラクター・アニメーションだそうだが、実際にAIを使っているかどうかは確認できなかったので、100%確実ではない。
Regardless, I think it's a pretty dang cool feature.
ともあれ、かなりクールな機能だと思う。
If you check out Adobe Express at adobe.com/express, click on Get Adobe Express Free, here I can scroll down and under suggested quick actions, there's this one that says Animate from audio.
adobe.com/expressでAdobe Expressをチェックアウトし、Get Adobe Express Freeをクリックすると、下にスクロールして、提案されるクイックアクションの下にAnimate from audioというのがあります。
If I click on this, we have the option of multiple characters.
これをクリックすると、複数のキャラクターを選択できます。
And by the way, that character that I just saw a second ago, this guy, he looks very familiar.
ところで、先ほど見たキャラクター、この男、とても見覚えがありますね。
I think this is a trick that my buddy Olivio SAS here has known about for a little while,cause if you go to the end of one of his videos, he's got this little dude.
これは、私の友人であるOlivio SASさんが少し前から知っているトリックだと思います。彼のビデオの最後に行くと、この小さなやつがいます。
This is the end screen.
これが終了画面。
There's other stuff you can watch like this, but now I know how he did it.
このようなものは他にもありますが、彼がどのように作ったのかがわかりました。
It looks like he probably made it with Adobe Express using this tool character.
おそらくAdobe Expressでこのツールキャラクターを使って作ったようだ。
But let's go ahead and use a Talking Taco,cause everybody knows I love tacos.
でも、僕がタコスが大好きなのはみんな知ってるから、「おしゃべりタコス」を使ってみよう。
And I can change the background.
背景を変えることもできます。
I can make it a transparent background.
背景を透明にすることもできる。
And then, I can put the animation over any scene that I want, or I can use one of their existing backgrounds.
アニメーションを好きなシーンにかぶせることもできるし、既存の背景を使うこともできる。
Here, I'm a huge baseball fan, so let's put the Taco on a baseball diamond.
私は大の野球ファンなので、タコスを野球のダイヤモンドの上に置いてみましょう。
I could scale my character up or down.
キャラクターを拡大縮小することもできる。
Down, let's make it a giant taco and put it right in the center.
では、巨大なタコにして中央に置いてみましょう。
And then, I can change the aspect ratio, but I'm just going to go ahead and leave it at 1:1.
アスペクト比を変えることもできますが、ここでは1:1のままにしておきます。
And I can record.
そして録画する。
Hey, my name is Matt the taco, and I love baseball, and I love eating tacos.
僕の名前はタコスのマット。野球が大好きで、タコスを食べるのが大好きなんだ。
Does that make me a cannibal?
僕は人食い人種になるのかな?
Now it says, hang tight, generating a preview.
今、プレビューを生成しているところです。
And here's my video.
これが僕のビデオだ。
Hey, my name is Matt the taco, and I love baseball, and I love eating tacos.
僕の名前はマット・ザ・タコス、野球が大好きで、タコスを食べるのが大好きなんだ。
Does that make me a cannibal?
だからって人食い人種になるのか?
I think some of the animations are a little bit more pronounced if you use one of these actual characters here.
アニメーションのいくつかは、ここにある実際のキャラクターを使うと、もう少し顕著になると思う。
Uh, using the taco was probably not the best example to show it off, but if you want to see a really good example, go watch Olivio's videoscause he does it at the end of every single one of them.
タコを使ったのはおそらく最良の例ではありませんが、本当に良い例を見たい場合は、Olivioさんのビデオを見てください。彼はすべてのビデオの最後でそれをやっています。
Again, not 100% positive this is using AI, but it's really cool nonetheless, and I want to share it with you all.
繰り返しになるが、これがAIを使ったものだと100%断言できるわけではないが、それでも本当にクールなので、みなさんと共有したい。
Right now, let's shift into text to video.
さて、次はテキストからビデオに移行しよう。
Text to video has gotten so good lately.
最近、テキストからビデオへの変換がとても良くなっています。
You've got Runway Gen 2, you've got P laabs, you've got Moon Valley, you've got morph Studio, you've got animate diff.
Runway Gen 2」、「P laabs」、「Moon Valley」、「morph Studio」、「animate diff」などなど。
There's so many cool text to video options out there, and now we've got this new one called show one, marrying pixel and latent diffusion models for text to video generation.
テキストをビデオに変換するクールなオプションはたくさんありますが、今回は、テキストをビデオに変換するためにピクセルと潜伏拡散モデルを組み合わせた「show one」という新しいオプションをご紹介します。
And if you look at some of these examples here, they look much more realistic than what we've gotten out of some of the previous text to video.
ここにいくつかの例を見ると、以前のテキストからのビデオよりもずっとリアルに見えます。
And it even looks like we can generate text inside of our videos now.
さらに、動画の中にテキストを生成することもできるようになりました。
So check out this comparison using the prompt of panda.
パンダのプロンプトを使った比較をご覧ください。
Besides the waterfall, there is a sign that says show Lab.
滝のほかに、ショーラボという看板があります。
Here's show one, where you can see the panda, waterfall, and the sign that says show Lab.
こちらは、パンダ、滝、そしてshow Labと書かれた看板が見えます。
This one, no sign at all, but you do have a panda and a waterfall.
こちらは、看板は全くありませんが、パンダと滝があります。
This is model scope zero scope.
これはモデルスコープ・ゼロスコープです。
You got some letters in there, you got the Pand on the waterfall.
中には文字があります。滝の上には「Pand」と書かれています。
And then some random letters floating in the sky.
そして空にはランダムな文字が浮かんでいる。
And then Gen 2 is, well, you can see what I see.
そして第2世代は、私が見たものを見てください。
Here's some more examples.
他にもいくつか例がある。
Look at the snail right here.
カタツムリをご覧ください。
Snail slowly creeping along, super macro closeup, high resolution, best quality.
カタツムリがゆっくりと這っている、超マクロ接写、高解像度、最高画質。
Model scope's actually pretty solid, but I mean, look at the colors in show one.
Model scopeは実際にかなり堅牢ですが、ショー1の色を見てください。
It just looks so good.
とてもいい感じだ。
Zero scope, we've got like a swarm of snails.
ゼロスコープ、カタツムリの大群のようだ。
And then gen two, not great compared to the rest.
そして第2世代、他と比べるとあまり良くない。
Speaking of text to video, we have new research called motion director, motion customization of text to video diffusion models.
テキストからビデオへの変換といえば、モーション・ディレクターという新しい研究があります。
Now, this is really cool because you can actually give it an input video and then have it generate new ideas based on the combination of the input video and your text prompt.
これはとてもクールなもので、実際に入力ビデオを与えて、入力ビデオとテキストプロンプトの組み合わせに基づいて新しいアイデアを生成させることができます。
So, they uploaded a few videos of people lifting weights and then gave it the text prompt, A bear is lifting weights.
そこで、人々がウェイトリフティングをしている動画をいくつかアップロードし、「クマがウェイトリフティングをしている」というテキストプロンプトを与えてみた。
And you can see, here's a video of a bear lifting weights, sort of following the way the video was doing it.
そして、こちらがクマがウェイトを持ち上げるビデオです。ビデオのやり方に従っています。
A dog is lifting weights.
犬がウェイトリフティングをしています。
You can see, here's the video of that.
こちらがそのビデオです。
They input a video of, like, a drone shot going around this house here, and then they gave a prompt of a pyramid in a forest.
彼らは、この家を回るドローンの映像を入力し、その後、森の中のピラミッドをプロンプトとして与えました。
And you can see, it sort of circles around this AI-generated pyramid in the same way it circles around the house.
AIが生成したピラミッドの周りを、家の周りを回るのと同じように回っているのがわかるだろう。
A temple on a mountain, same idea.
山の上の寺院も同じです。
Here's an input video of a car running on a road.
これは道路を走る車の入力映像です。
They changed it to a tank in the desert and a tiger in the forest, and it sort of followed the same path as that car.
それを砂漠の戦車と森のトラに変えましたが、その車と同じ経路をたどりました。
Here's some other examples.
他にもいくつか例があります。
Here, some input videos of people playing golf.
ゴルフをしている人たちの動画です。
You can see it compared to tuna video and several zero-scope models, and then the motion director model, which looks a lot more like a monkey playing golf than the rest of them.
マグロの動画や、いくつかのゼロスコープモデル、それからモーション・ディレクター・モデルと比べてみるとわかりますが、他のモデルよりもゴルフをしているサルに似ています。
And this page has several other examples that you can explore and see what this model is really capable of.
また、このページには他にもいくつかの例が掲載されていますので、このモデルが実際にどのようなことができるのか、ご覧になることができます。
Now, let's talk about audio with AI.
さて、AIを使った音声の話をしましょう。
This research recently came out called salmon speech audio language music open neural network.
サーモン・スピーチ・オーディオ・ランゲージ・ミュージック・オープン・ニューラル・ネットワークという研究が最近発表されました。
It allows speech, audio, and music inputs, and you can essentially chat with the audio to ask questions about the audio.
音声、オーディオ、音楽の入力が可能で、基本的にオーディオとチャットしてオーディオについて質問することができます。
And this one does have a working demo live over on gradio.
この研究はgradioでデモを公開しています。
Here, for example, you can upload an audio.
例えば、音声をアップロードすることができます。
They have some examples here.
ここにいくつか例があります。
Let's go ahead and use this first gunshots one and take a listen.
では、最初の銃声の例を使って聞いてみましょう。
Can you guess where I am right now?
私が今どこにいるかわかりますか?
So, if I click upload and start chat here, I can ask a question.
アップロードをクリックして、チャットを始めると、質問ができます。
What sounds are heard in the background?
背景にはどんな音が聞こえますか?
Gunshots and explosions are heard in the background.
背景で銃声と爆発音が聞こえます。
What is the person saying?
この人は何を言っていますか?
The person is saying, Can you guess where I am right now?
その人は言っています。「今、私がいる場所を当ててみてください」。
So, it listens and it can hear both the sound effects and the person speaking and understand it.
つまり、効果音と話している人物の両方が聞こえ、それを理解することができるのです。
Where is the person from the audio?
音声の人物はどこにいますか?
The person is from the United States.
その人はアメリカ出身です。
I was looking for, like, a war zone or something like that, but you get the idea.
私は紛争地帯か何かを探していたのですが、お分かりになりますか?
You can upload audio and then ask questions about the audio.
音声をアップロードして、その音声について質問することができます。
Here's something really interesting that's been circulating around the web lately.
最近、ウェブ上で広まっている非常に興味深いものがあります。
Is this video of a car on fire?
車が炎上している映像でしょうか?
Now, the environment is the real world, so everything you see, the car, cars, the streets, everything in the background, this is actually real world.
さて、環境は現実の世界です。ですから、あなたが見ているものすべて、車、自動車、道路、背景にあるものすべて、これは実際に現実の世界です。
But the car itself is actually computer-generated.
ただし、車自体はコンピュータ生成です。
So, the car, the fire, the smoke, this is all computer-generated imagery.
したがって、車、火、煙などはすべてコンピュータ生成のイメージです。
You can see as they move this little pill-shaped thing through it, the fire and the smoke is all impacted by it.
この小さな錠剤のようなものを動かすと、火も煙もすべてそれに影響されているのがわかるだろう。
It's just so crazy because this is the type of thing that makes it harder and harder for people to actually believe their eyes when they see videos on the internet.
これは本当にクレイジーです。これにより、インターネット上のビデオを見るときに人々が目を信じるのがますます難しくなっています。
Now, do keep in mind, this video has been circulating on Twitter, and somebody actually wrote, This video was generated in Unreal Engine.
ただし、このビデオはTwitterで広まっており、実際に「このビデオはUnreal Engineで生成されました」と書かれています。
It's crucial to understand what fifth-generation warfare looks like.
第5世代の戦争がどのようなものかを理解することは極めて重要だ。
Social engineering and misinformation is the name of the game.
ソーシャル・エンジニアリングと誤報がゲームの名前だ。
But the creator of this actually said, My work is being reposted on Twitter as misinformation by claiming that it is misinformation.
しかし、実際にこれを作った人はこう言っている。私の作品は、ツイッターで誤報だと主張することによって、誤報として再投稿されている。
So, do your own research.
だから、自分で調べてください。
They then wanted to say, to give more context, it is, in fact, not generated in Unreal Engine and not rendered in real time.
彼らはその後、もっと文脈を説明するために、これは実際にはアンリアル・エンジンで生成されたものではなく、リアルタイムでレンダリングされたものでもないと言いたかったようだ。
But the point still does stand that seeing stuff like this does make it pretty hard to believe our eyes.
しかし、このようなものを見ると、自分の目を信じることが難しくなるという点は変わりません。
Now, once again, I don't necessarily know if this has anything to do with AI.
繰り返しますが、これがAIと関係があるかどうかはわかりません。
I don't know if any of this was actually AI-generated.
これが実際にAIによって作られたものなのかどうかはわからない。
I don't believe it was.
そうだとは思っていない。
I just thought it was really cool and wanted to share it with you.
ただ、本当にクールだと思ったので、皆さんと共有したいと思っただけです。
Now, check this out.
では、これをご覧ください。
This is called 3D GPT procedural 3D modeling with large language models.
これは3D GPT手続き型3Dモデリングと呼ばれるもので、大規模な言語モデルを使っています。
And this is a text-to-3D scene generator.
これはテキストから3Dシーンジェネレーターです。
So, here's an example.
これがその例です。
The desert, an endless sea of shifting sand, stretched to the horizon.
果てしなく続く砂の海、砂漠が地平線まで続いている。
Its rippling dunes catching the golden rays of the setting sun, creating an ever-changing landscape of shadows and light.
その波打つ砂丘は、夕日の金色の光を浴び、影と光の絶え間ない風景を作り出しています。
Or the lake, serene and glassy, mirrored the cloudless sky above, reflecting the surrounding mountains and the graceful flight of a heron as lily pads floated like emerald jewels upon its tranquil surface.
あるいは、穏やかでガラスのように澄んだ湖は、雲ひとつない空を鏡のように映し出し、周囲の山々や、その静かな水面にエメラルドの宝石のように浮かぶユリの花とサギの優雅な飛翔を映し出した。
And from that prompt, it generated this 3D scene.
そして、そのプロンプトからこの3Dシーンが生まれた。
Blinding sunlight reigns over the vast desert expanse, casting sharp shadows behind the few resilient trees.
広大な砂漠にはまばゆいばかりの陽光が降り注ぎ、逞しい木々の背後には鋭い影を落としている。
Small sand piles sculpted by the relentless wind pepper the golden terrain.
容赦ない風によって削られた小さな砂山が、黄金色の地形を彩る。
If you look at it, you basically enter a prompt, it creates the scene, converts it into Python code.
これを見ると、基本的にプロンプトを入力すると、シーンが作成され、Pythonコードに変換される。
And then, the python code then becomes a 3D model in blender.
そして、そのPythonコードがblenderの3Dモデルになる。
So, these scenes that you're creating, you should be able to pull into blender, Unreal Engine, Unity, any of those tools, and use these 3D scenes in your games or your video creations, or whatever you need 3D scenes for.
作成したシーンは、blenderやUnreal Engine、Unityなどのツールに取り込んで、ゲームやビデオ作品など、3Dシーンが必要なものに使用することができます。
Speaking of 3D scenes, we also got this research called dreamspace.
3Dシーンといえば、dreamspaceという研究もあります。
Dreaming your room space with text-driven panoramic texture propagation.
テキスト駆動のパノラマ・テクスチャ伝搬で部屋の空間を夢見る。
So, with this, you can actually film a real-world scene, you know, walk around with a camera and film, and it looks like it's creating a Nerf or a gajin Splat or that sort of thing.
これを使えば、現実世界のシーンを実際に撮影することができます。カメラを持って歩き回り、撮影すると、まるでネルフやガジン・スプラット、そういったものを作り出しているように見えます。
It then reconstructs the scene into 3D objects, and then you can apply text prompts to change what that room looks like.
そして、そのシーンを3Dオブジェクトに再構築し、テキストプロンプトを適用してその部屋の見た目を変えることができる。
So, you've got a sci-fi theme here or a Zelda theme here, where it looks like you've got Hyrule out the window there.
SFをテーマにしたり、ゼルダをテーマにしたり、窓の外にハイラルがあるように見せたり。
But what's even cooler is then they show off, you can actually then look around in this room in Virtual Reality.
でも、さらにクールなのは、バーチャル・リアリティーで実際にこの部屋を見て回れることです。
It looks like they're using a meta Quest or something here to look around this new room that they created with the prompt seeing through the Galaxy.
メタ・クエストか何かを使って、ギャラクシー越しにプロンプトを見ながら、この新しい部屋を見て回っているようです。
Here's another example of somebody's like apartment or house or something.
ここには他の例もあります。誰かのアパートや家などです。
You can see the 3D object scene that it created.
作成された3Dオブジェクトのシーンが見えます。
They applied the prompt cyberpunk, the prompt nebula, the prompt anime landscape, and the prompt Harry Potter, and got completely different scenes out of each one here.
プロンプトのサイバーパンク、プロンプトの星雲、プロンプトのアニメの風景、プロンプトのハリー・ポッターを適用して、それぞれまったく異なるシーンを作りました。
So next, let's look at any portrait Gan animatable 3D portrait generation from 2D image collections.
それでは次に、2D画像コレクションからアニメーション可能な3D肖像画を生成する肖像画ガンについて見てみましょう。
So, this is research where you can actually upload 2D images and then actually turn them into sort of 3D movable avatars, where even their lips move and they talk, and you can see them smiling and opening their mouths and things like that.
ですから、これは2Dの画像をアップロードして、実際に3Dの動かせるアバターに変えることができる研究です。彼らの唇も動き、話すことができ、笑顔や口を開ける様子も見ることができます。
And I actually just find this animation here borderline hypnotic.
私はこのアニメーションに催眠術のようなものを感じている。
I hate to admit it, but I stared at this for way longer than I um care to share with you.
恥ずかしながら、私はこれを見つめている時間が長すぎたと思います。
But these are examples of these characters that are animated.
しかし、これらはアニメーション化されたキャラクターの例である。
You can see them moving their head, they're smiling, they're looking around, and the characters can actually be driven by real video.
彼らが頭を動かし、微笑み、周りを見回しているのを見ることができ、キャラクターは実際に本物のビデオで動かすことができる。
So, you can see a video of a real person talking here.
実際の人が話している映像がここにあります。
And then, these animations are actually following the real person talking.
そして、これらのアニメーションは、実際に話している人の後を追っています。
Same with this video below, somebody talking, the animations follow along.
この下のビデオも同じで、誰かが話していて、アニメーションがそれに従っています。
Now, let's talk about text to 3D because this is something that has made massive leaps and bounds recently.
では、テキストから3Dについて話しましょう。最近、これは大きな進歩を遂げています。
This one's called GSG text to 3D using Gajan splatting.
これは、Gajan splattingを使ったGSG text to 3D と呼ばれるものです。
You can see all sorts of examples here where they were able to create these 3D objects using text prompts and Gajan splatting.
テキストプロンプトとGajanスプラッティングを使って3Dオブジェクトを作成したさまざまな例をここで見ることができます。
Ever since Gajan splatting came out, I don't know, four or six weeks ago, it's created these massive leaps of what we can create with 3D scenes and 3D objects.
ガジャン・スプラッティングが登場した4、6週間前から、3Dシーンや3Dオブジェクトの作成が飛躍的に進歩しました。
We've got a plate of delicious tacos, a car made of sushi, a furry Corgi, a pineapple, and all of these are pretty dang good looking.
おいしいタコスの盛り合わせ、寿司で作られた車、毛むくじゃらのコーギー、パイナップルなど、これらはすべて非常に見栄えが良いです。
Here we can see it compared to previous models.
以前のモデルと比較してみましょう。
Look at this Corgi and Dream Fusion compared to their version.
このコーギーとドリーム・フュージョンを比べて見てください。
This Panda and Dream Fusion compared to their version.
このパンダとドリーム・フュージョンを比べてみてください。
Obviously, it's quite a bit better.
明らかに、かなり良くなっている。
If you want to know how it actually works and you know how to interpret this, here's a screenshot that shows how this works.
実際にどのように機能するのか、これをどのように解釈すればいいのか知りたい方は、スクリーンショットをご覧ください。
It's a little bit over my head, so it's not something I can totally explain how it works.
ちょっと私の頭では理解できないので、どう機能するのか完全に説明できるものではありません。
But from my understanding, you give it a text prompt, it generates a 2D image, then tries to generate a 3D point cloud from that 2D image.
しかし、私の理解では、テキストプロンプトを与えると、2D画像を生成し、その2D画像から3D点群を生成しようとする。
And then, with that point cloud, uses Gajan splatting to turn it into a 3D image.
そして、その点群を使ってガジャンスプラッティングで3D画像にする。
But I don't totally know what I'm talking about, honestly.
でも、正直なところ、何を言っているのかまったくわからない。
And then, we have Gajan Dreamer fast generation from text to 3D.
そして、Gajan Dreamerでテキストから3Dへの高速生成。
Gajan splatting with point cloud prior.
Gajan Splatting with point cloud prior.
This sounds like it's using a very similar method to what we just looked at, but they look even more detailed.
これは、先ほど見たものとよく似た手法を使っているようだが、より詳細に見える。
And this one, I feel, is a little bit easier to understand.
こちらは、もう少しわかりやすいと思います。
If we take a look at this image here, the prompt was given a fox.
こちらの画像を見てください。プロンプトはキツネです。
It uses a 3D diffusion model.
3D拡散モデルを使用しています。
It generates a point cloud.
ポイントクラウドが生成されます。
If you remember, Point E, that was one of the earlier models that was doing text to 3D, and they didn't look very good.
ポイントEは、テキストを3Dに変換する初期のモデルのひとつで、見た目はあまりよくありませんでした。
They created these sort of like point clouds of the 3D object, but they weren't very detailed.
3Dオブジェクトの点群のようなものを作成するのですが、あまり詳細ではありませんでした。
It then takes this point cloud and, using Gajan splatting, fills in the details of the points and gets a much more clear, slightly more realistic image.
この点群から、ガジャン・スプラッティングを使って点の細部を埋め、より鮮明で少しリアルな画像を得ることができます。
Here's an example of an axe where it starts with this point cloud.
ここでは、ポイントクラウドから始まる斧の例があります。
And then I believe using gajan splatting, it sort of filled in the details.
そして、ガジャンスプラッティングを使って、ディテールを埋めています。
There, here's some other examples: an airplane, a dragon, a flamethrower, a magic dagger, a mushroom boss, a fox, a banana, a jellyfish.
ここには他の例もあります。飛行機、ドラゴン、火炎放射器、魔法の短剣、キノコのボス、キツネ、バナナ、クラゲなどです。
Lots of cool examples.
クールな例がたくさんある。
And the detail of these 3D Generations has just gotten so good compared to what we just had weeks ago.
この3Dジェネレーションズのディテールは、数週間前と比べるととても良くなっている。
It's mind-blowing, honestly.
それは驚くべきことです。本当に。
Sticking with the theme of 3D generation, this one's not using gajin splatting, I don't think, but it's called MV dream multi-view diffusion for 3D generation.
3Dジェネレーションのテーマにこだわると、これはガジン・スプラッティングは使っていないと思うが、3DジェネレーションのためのMVドリーム・マルチビュー・ディフュージョンと呼ばれるものだ。
And here we can see an example of what the process looks like as these images are generated in 3D.
そして、これらの画像が3D生成される過程でどのように見えるかの例をここで見ることができます。
And it generates both the object, but it also, on this version, puts a texture over the object.
これはオブジェクトを生成するだけでなく、このバージョンではオブジェクトの上にテクスチャを配置しています。
Here's some other examples: here, there's just the object untextured, then obviously with the texture applied to it.
ここでは、テクスチャのないオブジェクトと、テクスチャが適用されたオブジェクトがあります。
Here's just an object of Gandalf, and then if I apply the texture, you get that.
これはガンダルフのオブジェクトだけで、テクスチャを適用するとこのようになります。
And here's some comparisons against previous models: dream Fusion, magic 3D text to mesh, prolific dreamer, and then our model using the prompt, an astronaut writing a horse.
ドリーム・フュージョン」、「マジック3Dテキストをメッシュに」、「多作な夢想家」、そしてプロンプトを使ったモデル、「馬を書いている宇宙飛行士」です。
And you can just see how far these text to 3D models have really come just in a short amount of time, honestly.
このテキストから3Dモデルへの変換が、ほんのわずかな時間でどれだけ進歩したかを実感していただけると思います。
And what I find really cool about this is you can actually train your own images into it using dream Booth.
私が本当に素晴らしいと思うのは、ドリーム・ブースを使えば、自分の画像を訓練して3Dモデルを作成できることです。
So here, in this example, they trained images of a very specific dog into it and were able to generate 3D objects in different positions of the dog they trained into it.
この例では、非常に特定の犬の画像を訓練して、訓練した犬のさまざまな位置に3Dオブジェクトを生成することができました。
So here's one of the dog sitting, one of the dog jumping, one of the dog on a rainbow carpet, one of the dog sleeping.
犬が座っているもの、犬がジャンプしているもの、犬が虹色のカーペットの上にいるもの、犬が寝ているものです。
And these were all generated from a trained-in image of their dog.
これらはすべて、訓練された犬の画像から生成されたものだ。
So text to 3D, blowing my mind right now, how far it's come.
テキストから3Dへ、その進歩には驚かされるばかりだ。
Once again, one of my sort of longer-term goals is to try to create a game using something like Unreal Engine.
もう一度言いますが、私の長期的な目標のひとつは、アンリアル・エンジンのようなものを使ってゲームを作ってみることです。
And now we're getting text to scene generation with that 3D GPT, and we're getting really, really good 3D objects with text to 3D.
そして今、3D GPTでテキストをシーンに生成できるようになり、テキストを3Dに変換することで、本当に素晴らしい3Dオブジェクトができつつあります。
And I'm really excited because creating game assets is going to be a lot easier with these tools.
これらのツールを使えば、ゲームアセットの作成がより簡単になるので、とても楽しみです。
Creating game environments is going to get a lot easier with these AI tools.
これらのAIツールを使えば、ゲーム環境の作成はもっと簡単になるでしょう。
I'm really excited with the pace at which all of this is moving.
私は、このすべてが進むペースにとても興奮しています。
Obviously, if you've been following along to my YouTube channel, you're seeing the pace that all of this is accelerating at, and it's just getting so exciting and so fun.
もちろん、私のYouTubeチャンネルをフォローしている方なら、このすべてがどれだけ加速しているかを見ているはずです。本当にエキサイティングで楽しいです。
Anyway, that's all I got for you today.
とにかく、今日はこれだけです。
I love making videos like this where I break down all of the advancements as they're happening and try my best to explain them, even though I often don't even know what I'm talking about myself.
私はこのようなビデオを作るのが大好きで、起こっているすべての進歩を分解し、自分でも何を言っているのかわからないことが多いのですが、一生懸命説明しています。
But it's fun to explore and see where things are heading and look at where the future of AI is going.
しかし、物事がどこに向かっているのか、AIの未来がどこに向かっているのかを探求し、見るのは楽しいものだ。
If you're just watching the news videos and just seeing what's available now, you're only getting half the picture.
ニュースのビデオを見たり、現在利用可能なものを見たりするだけでは、イメージの半分しか得られない。
With videos like this, you get to see where things are going next and get ahead of the curve.
このようなビデオでは、物事が次にどこへ向かうのかを見ることができ、時代を先取りすることができる。
And I love being ahead of the curve on this AI stuff, and hopefully you do too.
そして、私はこのAIのことで、曲線の先端にいることが大好きだ。
So thank you so much for nerding out with me.
一緒にオタクになってくれて本当にありがとう。
This was a total nerdfest for me, and I'm excited to make more videos like this for you.
これは私にとって完全なオタク祭りで、皆さんのためにこのようなビデオをもっと作りたいと思っています。
If you haven't already, check out Future Tools.
まだの方は、Future Toolsをチェックしてください。
This is where I curate all the cool AI tools that I come across, all the latest AI news on a daily basis.
ここでは、私が出会ったクールなAIツールや最新のAIニュースを毎日紹介しています。
Here, and I've got a free newsletter.
無料のニュースレターもあります。
You can click this button, join the free newsletter, and I will send you all of the coolest AI tools and all the latest AI news directly to your inbox.
このボタンをクリックして、無料のニュースレターに参加すれば、最もクールなAIツールや最新のAIニュースをすべて、あなたの受信トレイに直接お送りします。
You can find it all over at Future Tools.
すべてFuture Tools.
IO.
IOでご覧いただけます。
So thank you so much for tuning in again, and thank you to Wirestock for sponsoring this video.
このビデオのスポンサーになってくださったWirestockに感謝します。
I really appreciate you guys.
本当にありがとうございました。
And if you enjoyed this video, maybe consider liking it.
このビデオを楽しんでいただけたなら、「いいね!」をお願いします。
And if you want to see more videos like this, I'd love it if you'd subscribe to this channel.
また、このようなビデオをもっと見たい方は、このチャンネルを購読していただけると嬉しいです。
It would really make me happy.
本当に私を幸せにしてくれるでしょう。
So thank you so much.
本当にありがとう。
Really appreciate you.
本当にありがとう。
Again, I can't say it enough.
本当に言い尽くせません。
I'll see you guys in the next video.
また次のビデオで会いましょう。
Bye-bye.
バイバイ。
この記事が気に入ったらサポートをしてみませんか?