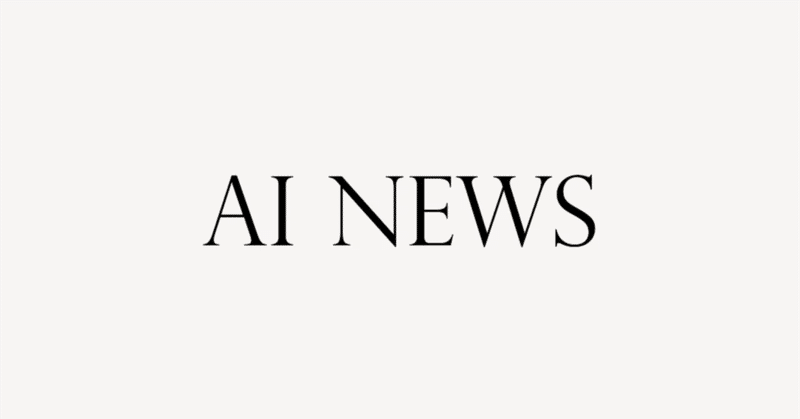
【イーロン・マスクとAIの未来】英語解説を日本語で読む【2023年12月24日|@TheAIGRID】
イーロン・マスクは最近、投資家キャシー・ウッドとともに、2024年に予定されているさまざまな開発についてTwitterスペースで議論しました。彼らはGrok AIやオープンソースAIなどについて語り、AI分野において重要な情報を共有しました。マスクはオープンソースAIの進展について言及し、今後6ヶ月以内にGPT-4の市場リーダーとしての地位が変わる可能性についても触れました。また、テスラに関する話題やビットコインの未来についても語り、これらの話題は多くの議論を呼びそうです。マスクはAIのオープンソース化とクローズドソース化についての見解を示し、彼自身がオープンソースを好む傾向にあることを明らかにしました。さらに、彼はテスラがリーディングなAI企業であり、リアルワールドAIの分野で圧倒的なリーダーであると強調しました。
公開日:2023年12月24日
※動画を再生してから読むのがオススメです。
Elon Musk just had a recent Twitter space where he discussed with another investor called Kathy Wood any future developments coming in 2024.
イーロン・マスクが最近ツイッターで、キャシー・ウッドという別の投資家と2024年に起こる将来の展開について議論しました。
Things like Grok AI, open source AI, and just a lot of stuff that you do need to know if you are in the AI space.
Grok AIやオープンソースAIなど、AI分野に携わる人なら知っておくべきことがたくさんあります。
I do think that it is a really interesting talk because he touches up on some of the things that people have been discussing on Twitter regarding open source AI.
オープンソースAIに関して人々がツイッターで議論していることのいくつかに触れているので、本当に興味深い講演だと思います。
He also does talk about the timelines for open source AI and how in around six months things could change because GPT-4 might not be the market leader we want to know it to be.
彼はまた、オープンソースAIのタイムラインについても話しており、GPT-4が我々が望むようなマーケットリーダーではなくなるかもしれないので、6ヶ月程度で状況が変わるかもしれないとも話しています。
So with that being said, hopefully you enjoy this Twitter space audio.
ということで、このツイッター・スペースの音声を楽しんでいただければ幸いです。
I'll leave a link to the full clip where he does actually talk about Tesla, but this is a supercut version to only hyper-specific focus on the bits where he's talking about AI, which is relevant for all of you.
彼が実際にテスラについて話している完全なクリップへのリンクを残しておきますが、これは、彼がAIについて話している部分だけに超特化したスーパーカットバージョンであり、皆さんに関連するものです。
So with that being said.
それを踏まえまして。
it basically said it seems that this is Grok talking, seems that people are eager to hear about the latest developments in AI.
基本的にはGrokが話しているようですが、人々はAIの最新の進展について聞きたがっているようです。
I mean, in Tesla's AI technologies, we definitely are, such as FSD12 and the next generation platform for Robotaxi.
つまり、テスラのAI技術において、我々は確かに進歩しています。例えば、FSD12やロボタクシーの次世代プラットフォームなどです。
They're also interested in learning more about the company's plans for the Optimus project.
また、オプティマス・プロジェクトに関する同社の計画についても知りたがっています。
Additionally, there is a lot of curiosity surrounding Elon Musk's thought on the AI space as well as his take on the future of Bitcoin.
さらに、イーロン・マスクのAI分野に対する考えや、ビットコインの将来に対する考えにも多くの好奇心が集まっています。
I guess that was a little repetitive AI there, but these topics are sure to spark some engaging discussions.
少し繰り返しの多いAIだったと思いますが、これらの話題はきっと魅力的な議論を巻き起こすでしょう。
That's Grok for you.
それがGrokの特徴です。
Okay Grok, that's pretty good.
さて、Grok、なかなかいいですね。
Yeah, pretty good.
いいですね。
I do have more time than, you know, if you would like to go longer than 15 minutes, we can go longer than 15 minutes.
もし15分以上話したいのであれば、私はあなたが思っているよりも時間に余裕があります。
Super.
素晴らしいですね。
I mean, I'm trying to distill my thoughts here on AI because I could really talk for hours on AI.
つまり、私はAIについて何時間でも話すことができるので、AIについてここで考えを絞り出そうとしているんです。
You know, both Charlie and Brett are steeped in it, so is Frank.
チャーリーもブレットもAIに精通しているし、フランクもそうです。
And, of course, Tasha, she's done our work on Autonomous.
そしてもちろん、ターシャはオートノミーに関する我々の仕事をこなしています。
But one of the things we've been very curious about is open source versus closed AI.
しかし、私たちが非常に興味を持っていることのひとつは、オープンソースとクローズドAIの比較です。
And I don't know if you've seen the chart that Frank and Joseph did on our team, but they drew a line.
フランクとジョセフが我々のチームで作成したチャートをご覧になったかどうかわかりませんが、彼らは線を引きました。
They basically calculated performance improvements in both the closed or private AI companies and models, large language models.
彼らは基本的に、クローズドまたは民間のAI企業やモデル、大規模な言語モデルのパフォーマンス向上を計算しました。
And then the opened ones.
そしてオープンなもの。
And open is behind closed, but the performance is improving at a steeper rate than closed.
オープンはクローズドの後塵を拝しているが、クローズドよりも急ピッチで性能が向上しています。
Do you have any thoughts about that and about open versus closed generally when it comes to AI?
AIに関して、一般的にオープンとクローズドについてどうお考えですか?
Sure.
もちろんです。
Well, I mean, I think a lot of people know that, you know, I was instrumental in the creation of OpenAI.
多くの人が知っていると思いますが、私はOpenAIの創設に貢献しました。
And in fact, I came up with the name, the open in OpenAI means open source.
OpenAIの "Open "はオープンソースという意味です。
Yes.
そうです。
And it started as an open source nonprofit.
オープンソースの非営利団体としてスタートしました。
And the reason I wanted to create it was to establish a counterweight to Google DeepMind, because it was a unipolar world at that point.
私がこの組織を作ろうと思った理由は、Google DeepMindへの対抗軸を確立するためでした。
It was, you know, Google DeepMind had probably two-thirds or more of the top AI talent and, of course, vast computing and financial resources.
Google DeepMindは、おそらくAIのトップクラスの人材の3分の2以上と、もちろん莫大なコンピューティング能力と資金力を持っていました。
And at the time, I was close friends with Larry Page.
当時、私はラリー・ペイジと親しかったです。
And it did not seem to me that he was taking AI safety seriously.
しかし、彼がAIの安全性を真剣に考えているとは私には思えませんでした。
So I felt there needed to be some, like I said, some counterweight, some competitor to sort of the Google DeepMind.
そこで私は、先ほど言ったように、Google DeepMindに対抗する対抗馬が必要だと感じました。
And frankly, instrumental in creating the company was recruiting Ilya Sutskever, which was a back and forth thing where actually Ilya changed his mind a few times and ultimately decided to come to OpenAI.
率直に言って、この会社を設立するのに役立ったのは、イリヤ・サツキヴァーをリクルートしたことです。イリヤは何度か考えを変え、最終的にオープンAIに来ることを決めました。
And, you know, I think it was a So I won that recruiting battle.
そして、私はそのリクルート合戦に勝利したのです。
And that is actually what caused Larry Page to stop being friends with me, frankly.
ラリー・ペイジが私と友達でなくなったのは、率直に言ってそのせいです。
But now, ironically, because fate loves irony, OpenAI is a super closed source and for maximum profit.
しかし今、皮肉にも、運命は皮肉を愛していますので、OpenAIは超クローズドソースで、最大限の利益を追求しています。
It should be renamed Closed for Maximum Profit AI.
『最大限の利益のためのクローズドAI』と名前を変えるべきです。
It won't be more accurate.
より正確ではありません。
So because that's, you know, it's literally the polar opposite of how it was started.
というのも、これは文字通り、始まった経緯とは正反対だからです。
I guess I generally have a bias in favor of open source.
私は一般的にオープンソースを支持するバイアスがあると思います。
And you can see that certainly with the X platform where we've open sourced the algorithm.
Xプラットフォームではアルゴリズムをオープンソース化しています。
And for example, for community nodes, we open source not just the algorithm, but all the data as well.
例えば、コミュニティ・ノードでは、アルゴリズムだけでなく、すべてのデータもオープンソースにしています。
So you can see exactly how a node was created.
ですから、ノードがどのように作られたかを正確に見ることができます。
It can be audited by third parties.
第三者による監査も可能です。
There's no mystery, nothing.
何も謎はありません。
That's one of the seems to be one of the confusing things about open source as pertains to large language models is it's not as simple.
大規模な言語モデルに関するオープンソースの分かりにくい点は、それが単純ではないということです。
And I think this has been lost in some of the debate as just open sourcing the code.
このことは、単にコードをオープンソースにするという議論の中で失われてきたと思います。
It's open source is the shades of gray here, of course, with parameter weights, data.
オープンソースは、パラメーターの重み付けやデータなど、グレーの濃淡があります。
There's all of that spectrum.
そのスペクトルのすべてがある。
And I think that's been maybe lost from some of the debate.
私は、このことが議論から失われていると思います。
So it's great to hear.
だから、それを聞けてうれしいよ。
Yeah, there's actually very little code.
実際、コードはほとんどありません。
Very little actual traditional lines of software.
実際の伝統的なソフトウェアの行はほとんどない。
Certainly, at the at the first level is this very little, it's a remarkably tiny number of lines of code.
もちろん、最初のレベルでは非常に少ないです。驚くほど少ない行数のコードです。
So it's just these giant, weights files and what your hyper parameter, numbers are.
つまり、それは単に巨大な重みファイルと、あなたのハイパーパラメータの数値だけなのです。
And it's basically a giant comma separated value file.
基本的には巨大なカンマ区切りの値ファイルなんだ。
So I always find it amusing to contemplate that perhaps our digital God will be a CSV file.
だから私はいつも、もしかしたら私たちのデジタルゴッドはCSVファイルになるかもしれない、と考えるのが面白いんだ。
And then, you make a new CSV file that has maybe more weights, and the weights are better.
そして、新しいCSVファイルを作って、そのファイルはもっと重みがあって、重みもより良いものになる。
And then you pretty much delete the old one, so just the AI is evolving and deleting its prior self constantly.
そして、古いものを削除する。つまり、AIは常に進化し、以前の自分を削除しているんだ。
So, we're going to worship a giant excel file essentially.
つまり、巨大なエクセルファイルを拝むことになる。
That's probably kind of, I mean, you need a very big, yeah, it's a lot of cells.
それはおそらく、とても大きな、そう、たくさんのセルが必要なんだ。
But, it's really just a bunch of numbers.
でも、実際はただの数字の束なんだ。
And, those numbers are, they're getting smaller in magnitude too, you know, going from FP16 to FP32 to FP16 to in 16 to into 8.
そして、それらの数字は、FP16からFP32へ、FP16から16ビットへ、8ビットへと、その大きさも小さくなっているんですよ。
And, now it looks like things are trending towards, mostly into 4.
そして今は、ほとんどが4つになる傾向にあるようだ。
So they're not particularly large numbers.
それほど大きな数ではありません。
I'm not sure if I'm answering, I'm probably not answering the question, but I mean, I think it's true that closed source is not that far, in like, for from a time standpoint, not that far ahead of open source.
答えているかどうかはわかりませんが、おそらく質問には答えていないと思います。しかし、クローズドソースはオープンソースと比べて、時間的な観点からはそれほど進んでいないということは真実だと思います。
But let's say, and if you assume open source is you have six months because of the immense rate of improvement of AI, that six months is actually a massive amount of time.
しかし、仮にオープンソースが6ヶ月だとすると、AIの進歩の速さは計り知れませんから、6ヶ月というのは実際には膨大な時間です。
I mean, or at least the delta in an exponentially improving situation, six months will, I think, feel like an eternity.
つまり、少なくとも指数関数的に改善する状況でのデルタは、6ヶ月が永遠のように感じられると思います。
So I think closed will outperform open by a meaningful amount at any given point in time.
だから、私はクローズドの方がオープンよりも、いつでも意味のある程度で優れていると思います。
Given the questions we were talking about before about truth and not truth and do you think in 10 years time AI will have been a net truth, improver or detractor from where we are today?
前に話していた真実と非真実に関する質問を考えると、10年後にはAIは今の私たちのいる場所から真実を向上させるか、それとも悪化させるか、どちらだと思いますか?
I think it will be, speaking for at least for Grok, I think it will be a significant, truth improver.
少なくともGrokについて話すと、私はそれが重要な真実の向上者になると思います。
That is literally our goal, is to be maximum truth seeking, maximally curious, minimizing the error between perceived reality and actual reality, and always acknowledging the error, not being too confident about it.
それが私たちの目標です。最大限の真実を求め、最大限の好奇心を持ち、知覚された現実と実際の現実との間のエラーを最小限に抑え、常にそのエラーを認識し、自信を持ちすぎないことです。
So, I think that there will be a competition for truth and you will go people will, tend towards the one that they think is most accurate.
だから、真実を競うことになると思いますし、人々は最も正確だと思う方に傾くでしょう。
So, and if there's at least one AI that is, aiming for maximum accuracy, I think it pushes all of the AIs to aim for maximum accuracy.
そして、最大の正確さを目指す少なくとも1つのAIが存在する場合、それは他のすべてのAIを最大の正確さを目指すように推進すると思います。
Just as with the X platform, FKA Twitter, as soon as you know X pushes for maximum truth and accuracy, it sort of forces the hand of others.
Xプラットフォーム(旧Twitter)と同様に、Xが最大の真実と正確さを追求するとすれば、他の人々もそれに追随せざるを得なくなります。
They, the others now also have to do that.
他のプラットフォームもそうしなければならなくなる。
The truth arms race among the CSVs and is one of our theses.
真実の競争はCSV間で行われ、それは私たちの論文の一つです。
I think with Ark is that Tesla is one of the most undervalued AI companies.
テスラは最も過小評価されているAI企業のひとつです。
There is because of the insane amount of data you've got on all to feed into autonomous.
それは、自律的にフィードするために持っている膨大なデータの量のためです。
I don't know if there's any commentary on that because I think obviously with the rise of these LLM companies, which by and large working off essentially available data that is not proprietary, the benefits coming to the companies like Tesla that understand how to use the AI benefits but do have these enormous proprietary data pools seems like that's going to be the next frontier.
その点についての解説があるかどうかはわかりません。なぜなら、これらのLLM企業の台頭に伴い、基本的には独自のデータではなく利用可能なデータを使用しているため、AIの恩恵をうまく活用できるテスラなどの企業には巨大な独自のデータプールがあるように思われますが、次のフロンティアになるでしょう。
Yeah, I think that's accurate, an accurate description.
はい、正確な説明だと思います。
Tesla is one of the leading AI companies in the world, and with respect to real-world AI, it is obviously by far and away the leader.
テスラは世界でもトップクラスのAI企業の一つであり、現実世界のAIに関しては、明らかに圧倒的なリーダーです。
So you know, the word large language model with phrasal large language model LLM is massively overused, but what I do see happening is somewhat of a convergence towards intelligence.
大規模言語モデルLarge Language Model LLMという言葉は乱用されていますが、私が見ているのは、インテリジェンスへの収束です。
You know, it's just, Tesla, like really, to make full self-driving work, you kind of need BabyAGI in the car, because you need to understand reality and reality is messy and complicated.
あの、実は、テスラは、完全自動運転を実現するためには、車にBabyAGIが必要なんですよ。なぜなら、現実を理解する必要があり、現実は複雑で混沌としているからです。
And just as a side effect, the car AI has to be able to read, for example, you have to be able to read arbitrary science as a little, just a little side effect of understanding reality in every language.
なぜなら、現実を理解する必要があり、現実は厄介で複雑だからです。副次的な効果として、車のAIは、例えば、あらゆる言語の現実を理解するためのちょっとした副次的な効果として、任意の科学を読むことができなければなりません。
So, I think everything is coming down to different layers of transformers and diffusion.
ですので、すべてが異なるレイヤーのトランスフォーマーと拡散に帰結していると思います。
And then, how do you put together the transformers and diffusion?
そして、その変容と拡散をどのように組み合わせるのか?
That's what I sort of made, that's somewhat Niche AI joke on the X platform.
これが、私がXプラットフォームで作った、ニッチAIのジョークです。
Who do you think will be president in 2032, transformers or diffusion?
2032年にはトランスフォーマーとディフュージョン、どちらが大統領になると思いますか?
Do you think that switching to full stack AI for Tesla's full self-driving reduces the kind of forecast error for you in terms of when the problem will be solved?
テスラの完全自動運転のためにフルスタックAIに切り替えたことで、問題がいつ解決するかという点で、あなたにとって予測誤差のようなものが減ると思いますか?
As in, you famously have said it's a year away for a few years now.
有名な話ですが、あなたは数年前から1年先だと言っています。
Do you think that, like actually line of sight, wherever it ends up, you'll have a better line of sight on when you're going across a particular threshold of performance where it can go without human intervention?
目の前の状況によっては、人間の介入なしで進むことができる性能の特定の閾値を超えるときに、より良い展望が得られると思いますか?
Yeah, I mean, the car is already incredibly good at driving itself.
ええ、つまり、クルマはすでに信じられないほど自動運転が上手なんです。
So now, especially if you say like, okay, drive in California, which is both generally easy driving because you don't have heavy rain and snow and that kind of thing in most parts of California.
特に、カリフォルニアで運転する場合、一般的には雨や雪などがほとんどないため、比較的簡単な運転です。
And Tesla engineering is primarily in California, so you're going to get, it's going to overfit for solving California.
テスラのエンジニアリングは主にカリフォルニアにあるので、カリフォルニアを解決するためにオーバーフィットすることになります。
But if you're just driving, say, around Palo Alto, the probability that you'll need an intervention at this point is incredibly low.
しかし、例えばパロアルト周辺をドライブするだけなら、現時点で介入が必要になる確率は信じられないほど低い。
In fact, even if you're driving through San Francisco, the probability of an intervention at this point is very low.
実際、サンフランシスコを走っていても、この時点で介入が必要になる確率は非常に低い。
So we're really just going through a march of nines, like how many nines of reliability before somebody does not need to monitor the system.
つまり、誰かがシステムを監視する必要がなくなるまでに、信頼性が何ナインになるか、というような9の行進をしているわけです。
You know, it's so interesting, GM basically shutting down Cruise.
GMがクルーズを基本的にシャットダウンしたのは、とても興味深いことです。
We, it wouldn't have been possible, right?
私たちは、そんなことはあり得ないと思っていました。
But we didn't know that.
でも、私たちはそれを知らなかった。
Is that how you're viewing it now?
今はそう考えているんですか?
Well, it's certainly true that transformers are transformational.
まあ、トランスフォーマーが変革をもたらすというのは確かに事実です。
Pretty much everyone's using transformers.
そう、かなりの人がトランスフォーマーを使っている。
And it's really just how many transformers, what kind of transformers are you using?
それは本当に、どれくらいのトランスフォーマーを使っているかによるんですよ。
Autoregressive transformers, which are very memory bandwidth intensive.
自己回帰的なトランスフォーマーは、メモリ帯域幅を非常に消費します。
I'm not sure you can really do AI without transformers in a meaningful way.
意味のある方法でAIを行うには、トランスフォーマーなしでは本当にできないとはっきり言えません。
Diffusion is also very important.
拡散も非常に重要です。
So I mean, just really looks like AGI is some combination of transformers and diffusion.
つまり、AGIはトランスフォーマーとディフュージョンの組み合わせということになりますね。
That's interesting.
それは興味深い。
Does diffusion help Elon in the things that transformers are necessary, not necessarily that good at yet, like planning and remembering rather than sort of a current state?
拡散は、トランスフォーマーが必要なことで、必ずしもまだ得意ではないこと、例えば、現在の状態ではなく、計画や記憶のようなことで、イーロンを助けてくれるのでしょうか?
Transformers are important for all aspects of AI.
トランスフォーマーはAIのすべての側面に重要です。
Yeah, I mean, computers are very good at remembering things.
ええ、つまり、コンピューターは物事を記憶するのがとても得意なんです。
I mean, your phone remembers the video that you took down to the last pixel, whereas most humans cannot remember who they met last week.
例えば、あなたの携帯電話は、最後のピクセルまで撮影したビデオを覚えていますが、ほとんどの人間は先週会った人のことを覚えていません。
So, memory is the easy, I mean, we've already outsourced memory to computers in that like you say, how much of human knowledge is in digital form versus in and contained in human neurons?
ですので、メモリは簡単です。つまり、既にコンピュータにメモリを外部委託しているという意味です。人間の知識のどれくらいがデジタル形式であり、人間のニューロンに含まれているのか、という点ですね。
It's overwhelmingly in silicon rather than biological neurons.
それは生物学的なニューロンよりも圧倒的にシリコンで構成されています。
Right.
そうですね。
It's good to think of it, like to try to consider what are the most fundamental rations.
それを考えるのは良いことです。最も基本的な比率は何かを考えてみることです。
In physics, you're always thinking about looking at fundamental ratios, and one of the fundamental ratios is the ratio of digital to biological compute.
物理学では、基本的な比率を見ることを常に考えていますが、基本的な比率の1つは、デジタル計算と生物学的計算の比率です。
Just in raw compute, and the ratio of digital memory to biological memory.
単に計算能力とデジタルメモリと生物学的メモリの比率です。
At this point, I think probably over 99% of all memory is digital, as opposed to biological.
現時点では、おそらく全メモリの99%以上がデジタルメモリであり、生物学的メモリとは対照的だと思います。
You've got over 100 digital to biological ratio in memory at this point, trending towards 1000, and many orders of magnitude beyond that.
現時点では、メモリにおける生物学的メモリに対するデジタルメモリの比率は100を超え、1000に近づきつつある。
Then you've got the digital to biological compute ratio.
そして、デジタルと生物学的なコンピュートの比率がある。
And since the number of humans is more or less constant, I'm worried about humans declining quite significantly in the years to come, because the birth rate is so low.
そして、人間の数は多かれ少なかれ一定であるため、私は、出生率が非常に低いこの先数年で人間がかなり減少するのではないかと心配しています。
So human computers, more or less, it looks like a flat line versus time, whereas the digital compute is exponential.
人間のコンピューターは多かれ少なかれ、時間に対して平坦な線に見えますが、デジタルコンピューターは指数関数的です。
And at some point, that will also be above 100, meaning more than 99% of all compute will be digital instead of biological.
つまり、全コンピュータの99%以上が生物学的なものからデジタル的なものになるということです。
And if we're not there already, we will be within a year or two.
もしまだそうなっていないとしても、1、2年のうちにそうなるだろう。
Although it is amazing how much data biology can store, I reckon, I believe it's estimated that a gram of dry DNA can store between 400 and 500 exabytes.
生物学がどれだけのデータを保存できるかは驚くべきことだが、私は、乾燥したDNA1グラムが400~500エクサバイトを保存できると推定している。
So maybe there's still things to learn from biology.
だから、生物学から学ぶべきことはまだあるのかもしれない。
But yes, incredible, the pace of change.
しかし、信じられないような変化のスピードだ。
Yeah, I mean, if you encode memories in DNA like a ticker tape, then the DNA memory potential is immense.
そう、つまり、DNAにテロップのように記憶をエンコードすれば、DNAメモリーの可能性は計り知れない。
But I'm not sure that's actually what's happening.
でも、実際にそうなっているかどうかはわからない。
Or if it is, it's, I don't know, I'm not sure why my memory is so terrible.
もしそうだとしたら、なぜ私の記憶力がそんなにひどいのかわからない。
But actually, I mean, your memory, in terms of the raw details, like you have an amazing search function, right?
でも実際、あなたの記憶力は、生の詳細という意味では、素晴らしい検索機能を持っているようなものでしょう?
I mean, it's kind of like, yeah.
まあ、そういう感じですね。
Well, for any, I mean, I would argue kind of, in some ways, like the transformer architecture enables effective search of a very, very broad amount of data.
つまり、ある意味では、トランスフォーマー・アーキテクチャーは、非常に広範なデータを効果的に検索することができるんだ。
And, or that's, or it's like data compression.
または、データ圧縮のようなものですね。
It seems like the, yes, we have, there's many more like gigabytes encoded in disk, but actually being able to effectively search that space is pretty still primitive with compute, at least at large scale, relative to humans ability to access information.
ディスクにエンコードされたギガバイトのようなデータはたくさんありますが、実際にその空間を効果的に検索できるのは、少なくとも人間よりも大規模な計算機ではまだ原始的なことのようです。
I mean, it's still some things that humans do better than computers.
つまり、人間がコンピューターより得意とすることはまだいくつかある。
I mean, if you look at, say, computers have not yet discovered any fundamental physics, but humans have discovered a lot of fundamental physics.
例えば、コンピューターはまだ基礎物理学を発見していませんが、人間は多くの基礎物理学を発見しています。
The computers have not yet invented a useful technology.
コンピューターはまだ有用な技術を発明していない。
Humans have invented many useful technologies.
人間は多くの有用な技術を発明してきた。
Do you ascribe to the notion that like you essentially training AI systems on language is not enough and that you have to have some kind of embodied source of data, like through an optimist robot to actually get kind of the raw learning in that AI system would need to understand fundamental physics?
あなたは、AIシステムを言語で基本的にトレーニングするだけでは不十分であり、AIシステムが基礎物理学を理解するために必要な生の学習を実際に得るために、最適化ロボットを介したような具体的なデータソースを持つ必要があるという考えに賛成ですか?
Yeah, so that's what I think is coming.
そうですね、それが私の考えることです。
I think what's coming is AI understanding fundamental physics and AI inventing new technologies.
私が予測するのは、AIが基本的な物理学を理解し、新しい技術を発明することです。
It definitely feels like rather than, even though some things now apparently pass the Turing test, most importantly, passing the Moneypenny test, as in can an AI actually pay bills, do expense reports to the extent James Bond did those, and actually pay parking fines and things like that, that would actually be a very good use of AI that I think would have a lot of users immediately.
確かに、いくつかのことは今ではチューリングテストに合格するようですが、最も重要なのは、マネーペニーテストに合格することです。つまり、AIが実際に請求書を支払ったり、経費報告書を作成したり、ジェームズ・ボンドがそれらを行ったように駐車違反の罰金を支払ったりすることができるかどうかです。それは、AIの非常に良い使い方であり、多くのユーザーがすぐに利用すると思います。
And maybe the Billy Connolly test of can an AI actually be consistently funny.
また、ビリー・コノリーのように一貫して面白いAIができるかもしれない。
Grok is pretty funny.
Grokはかなり面白い。
That's fair.
それは妥当だ。
I think, you know, one of our goals for Grok is to be the funniest AI.
Grokの目標の一つは、最も面白いAIになることです。
So, I mean, if you ask Grok to provide a vulgar roast, it's really good.
だから、Grokに下品なローストを頼むと、本当にうまいんだ。
And speaking of Grok, to not let down our future AI overloads, the final question on the Grok summary that Cathy touched on was the future of Bitcoin.
そしてGrokといえば、未来のAIを失望させないために、キャシーが触れたGrok要約の最後の質問は、ビットコインの未来だった。
Not sure if we want to touch on that or not, or whether that's out of scope.
それについて触れたいのか、それとも範囲外なのかはわからない。
この記事が気に入ったらサポートをしてみませんか?