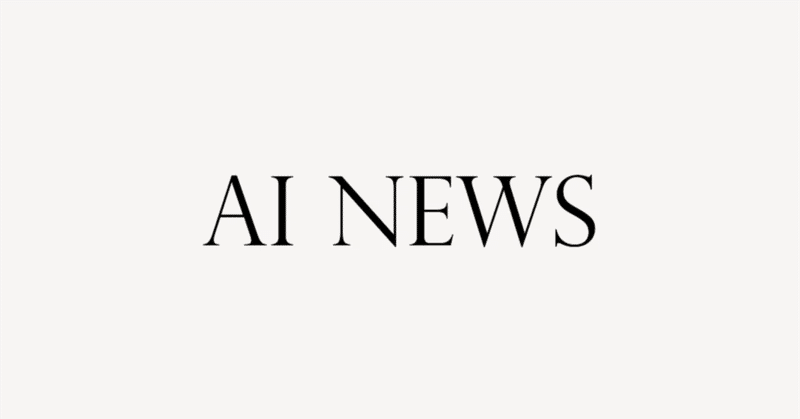
【AIニュース】英語解説を日本語で読む【2023年12月16日|@Wes Roth】
この動画では、AIに関する最新ニュースを紹介しています。OpenAIの次の大型モデル、GPT 4.5のスクリーンショットがリークされ、サム・アルトマンが調査中です。Google Deep Mindは言語モデルを使った数学的発見を報告し、OpenAIはAIシステムのガバナンスガイドラインを発表しました。Runway MLは一般的なワールドモデルの研究を始め、Google Deep Mindはテキストから画像への変換技術を公開しました。Midjourney Alphaは新しいAIアート生成手法を発表しました。
公開日:2023年12月16日
※動画を再生してから読むのがオススメです。
So, this was a huge week for AI news.
さて、今週はAIのニュースにとって大きな週だった。
Let's catch up on some of the big things that were happening.
いくつかの大きな出来事をキャッチアップしてみよう。
There's a lot of rumors about the next big model coming from OpenAI.
OpenAIから次の大きなモデルが出るという噂がたくさんあります。
Somebody dropped this screenshot of GPT-4.5, which was very suspicious, but don't worry, Sam Altman's on the case.
誰かがGPT-4.5のスクリーンショットを投稿しましたが、それは非常に怪しいものでしたが、心配しないでください、サム・アルトマンが調査中です。
He's going to clarify the situation for us.
彼が状況を明らかにしてくれるだろう。
Somebody asks them, GPT-4.5 leak legit or no?
GPT-4.5のリークは本物か?
Sam Altman answers, nah, which again, people are a little bit confused.
サム・アルトマンは「ノー」と答え、人々は少し困惑している。
Like, what does that mean?
どういう意味ですか?
The image is fake or the news about the new model is fake?
画像がフェイクなのか、それとも新モデルに関するニュースがフェイクなのか?
Either way, we'll know soon enough.
いずれにせよ、すぐにわかるだろう。
Google DeepMind drops this paper, Mathematical Discoveries from Program Search with Large Language Models, and here they introduce fund search, which actually stands for searching in the function space.
Google DeepMindがこの論文「Mathematical Discoveries from Program Search with Large Language Models」を公開し、ここで彼らは実際に関数空間での検索を意味する「fund search」を紹介しています。
There's a paper in Nature that kind of goes over it.
ネイチャー誌には、この論文についての解説が掲載されている。
The big headline news here is this is the first time anyone has shown that an LLM-based system can go beyond what was known by mathematicians and computer scientists.
ここでの大きな見出しとなるニュースは、LLMベースのシステムが数学者やコンピューター科学者の既知を超えることができることを初めて示したということだ。
It's not just novel, it's more effective than anything else that exists today.
単に斬新なだけでなく、現在存在する他のどんなものよりも効果的なのだ。
So, this is yet another example of AI coming out of DeepMind that is rapidly expanding the human scientific knowledge.
つまり、これはディープマインドから生まれたAIが、人間の科学的知識を急速に拡張しているもうひとつの例なのだ。
In this case, it's mathematics.
この場合、それは数学です。
In the case of AlphaFold, it's expanding our understanding of the 3D structures of proteins.
AlphaFoldの場合は、タンパク質の3D構造についての理解を広げている。
The Gnome project is discovering new materials, and a lot of this is done autonomously.
Gnomeプロジェクトは新素材を発見しており、その多くは自律的に行われている。
So, it's AI and robots kind of doing their own thing to create this.
つまり、AIとロボットが自分たちの力でこのようなものを作り出しているのです。
Humans are more and more out of the loop.
人間はますますその輪から外れていく。
And just yesterday, OpenAI drops this Practices for Governing Agentic AI Systems.
そしてつい昨日、オープンAIが「エージェント型AIシステムを管理するためのプラクティス」を発表した。
In their words, A systems that have agency, they have their own goals and also have the ability to pursue those goals.
彼らの言葉を借りれば、エージェンシーを持つシステムは、自分自身の目標を持ち、その目標を追求する能力も持っている。
Agentic AI systems, AI systems that can pursue complex goals with limited director provision, are likely to be broadly useful if we can integrate them responsibly into our society.
エージェント型AIシステム、つまり限られた監督下で複雑な目標を追求できるAIシステムは、私たちの社会に責任を持って組み込むことができれば、広く役立つ可能性がある。
And here they have this paper that talks about things that we need to think about as a society, as humanity, about how to safely release these things into the wild.
そしてこの論文では、社会として、人類として、これらのAIを安全に野に放つ方法について考える必要があると述べている。
They quickly define what they mean when they say AI agents, and I think they actually invent a brand new word, agness.
彼らはAIエージェントという言葉の意味をすばやく定義し、実際に新しい言葉「agness」を作り出していると思います。
We define the degree of agness in a system as the degree to which a system can adaptably achieve complex goals in complex environments with limited direct supervision.
私たちは、システムが複雑な環境において、限られた直接的な監督下で複雑な目標を適応的に達成できる度合いとして、システムのagnessの度合いを定義している。
Agentic, as defined here, thus breaks down into several components: goal complexity, environmental complexity.
ここで定義されるエージェント性は、目標の複雑さ、環境の複雑さといったいくつかの要素に分解される。
So, for example, to what extent are they cross-domain, multi-stakeholder, require operating over long time horizons, and involve the use of multiple external tools?
つまり、例えば、どの程度領域横断的で、マルチステークホルダーであり、長い時間軸で活動する必要があり、複数の外部ツールを使用する必要があるのか。
Then adaptability, how well can the system adapt and react to novel or unexpected circumstances?
また、適応性とは、斬新な状況や予期せぬ状況に対して、システムがどの程度適応し、反応できるかということである。
And independent execution, to what extent can the system reliably achieve its goals with limited human intervention or supervision?
また、独立した実行力とは、限られた人間の介入や監視によって、システムがどの程度まで確実に目標を達成できるかということである。
So, reading through this paper, to me, it seems like it's mostly a way to kind of brainstorm and get all the potential dangers out on paper, as well as some questions that we have to answer before we allow these agents to autonomously go and act on our behalf.
この論文を読んでみると、私にはほとんどブレインストーミングのようなものであり、潜在的な危険性を紙に書き出す方法のように思えます。また、これらのエージェントが自律的に行動する前に、私たちがいくつかの質問に答えなければならないということもあります。
Whether they're able to do it, when is approval required?
それができるかどうか、承認はいつ必要なのか。
They talk about autonomous weapons, for example.
彼らは例えば自律兵器について話しています。
Default behaviors, so for example, they talk about how, you know, if you're getting it to do your grocery shopping for you or to complete some chores for you, the default should be, for example, to spend no money, right?
デフォルトの振る舞い、例えば、食料品の買い物やいくつかの雑用を頼む場合、デフォルトはお金を使わないことですね。
So only spend money when you have to, don't just default to buying the most expensive thing right away.
つまり、必要なときだけお金を使うのであって、すぐに一番高価なものを買うのがデフォルトではないのだ。
And here, interestingly, they talk about the shifting of offense-defense balance.
そしてここで興味深いことに、彼らは攻撃と防御のバランスの変化について話しています。
So some tasks may be more susceptible to automation by agents than others.
つまり、エージェントによる自動化の影響を受けやすい業務と受けにくい業務があるということだ。
So for example, in the cybersecurity domain, human monitoring and incident response is still key to cyber attack mitigation.
例えば、サイバーセキュリティの分野では、人による監視とインシデント対応がサイバー攻撃軽減の鍵であることに変わりはない。
So the visibility of such human monitoring is predicated on the fact that the volume of attacks is similarly constrained by the number of human attackers, all right?
このような人間による監視の可視性は、攻撃の量が人間の攻撃者の数によって同様に制限されるという事実を前提としている。
So if you have 10 guys trying to hack into somewhere and you have 10 cybersecurity experts kind of defending and monitoring for those attacks, you know, if one side increases their offense abilities, the defense similarly can increase their defense abilities, you know, in general, obviously.
だから、もし10人の人がどこかにハッキングしようとしていて、10人のサイバーセキュリティの専門家がそれらの攻撃に対して防御し、監視している場合、片方が攻撃能力を増すと、防御側も同様に防御能力を増すことができます、一般的には、もちろん。
But what happens if the offense can now use a million smart AI agents that are able to carry out these tasks autonomously without human supervision?
しかし、もし攻撃側が、人間の監視なしに自律的にこれらのタスクを実行できる100万人のスマートAIエージェントを使えるようになったらどうなるだろうか?
Is there a limit to how many they can deploy at one time?
一度に配備できる数には限界があるのだろうか?
So in that world, that would kind of destroy cybersecurity, right?
そのような世界では、サイバーセキュリティは破壊されることになる。
But on the other hand, conversely, if agentic AI systems make monitoring and response cheaper than producing new cyber attacks, the overall effect would be to make cyber defense cheaper and easier, which is an interesting point, right?
しかし逆に、エージェント型AIシステムが新たなサイバー攻撃を生み出すよりも監視と対応を安価にするのであれば、全体的な効果はサイバー防衛をより安価で容易にすることになる。
Because it's kind of been this race, you know, if the offense improves, the defense improves.
攻撃が改善されれば、防御も改善されるというような競争があるんですよ。
But what if we find that in the future with these AI agents, either the offense or the defense is significantly easier and just more powerful?
しかし、もし将来、このようなAIエージェントによって、攻撃と防御のどちらかが大幅に容易になり、より強力になるとしたらどうでしょう?
That either means that the ability to hack into systems skyrockets or is more or less completely negated.
その場合、システムへのハッキング能力は急上昇するか、あるいは多かれ少なかれ完全に否定されることになる。
I don't think we're quite sure yet which future we're going to live in.
私たちがどちらの未来に生きることになるのか、まだはっきりとはわからないと思う。
So they're saying it's very difficult to anticipate the net effect of these sort of AI adoption dynamics, and it's saying it behooves actors to pay close attention in identifying which equilibrium assumptions no longer hold.
つまり、このようなAI導入のダイナミクスがもたらす正味の影響を予測することは非常に難しく、どの均衡前提がもはや成り立たないかを見極めるために、行動者は細心の注意を払う必要があると述べているのだ。
I mean, this is where kind of the conversation about AI drones really kind of comes into play, specifically, I mean, attack drones that are disconnected from any human operator.
つまり、AIドローンに関する議論が本格化する場所ですね、具体的には、人間のオペレーターから切り離された攻撃ドローンです。
What are the defense against those drones?
このような無人機に対する防御はどうなるのでしょうか?
Does attacking then being on the offense, does that become the much more effective tactic than waiting and trying to defend?
攻撃することが守りを待つよりもはるかに効果的な戦術になるのか、それとも守りを待ち、守ることがより効果的な戦術になるのか、それが問題になります。
If that is indeed the case, then the world kind of becomes a more of a scary place.
もしそれが本当にそのような場合なら、世界はより恐ろしい場所になるんですよね。
And right around the same time that OpenAI released this paper, they released another one called Weak to Strong Generalization.
ちょうどOpenAIがこの論文を発表したのと同じ頃、彼らはWeak to Strong Generalizationという別の論文を発表した。
And so it's part of their super alignment, how do we make superintelligence safe?
そして、それは彼らのスーパーアライメントの一部であり、どのようにして超知能を安全にするかということです。
And so in this paper, they're talking about an approach that seems to be having some results.
そして、この論文では、いくつかの結果を出しているアプローチについて話しています。
And so traditionally, you can think of this as a human supervisor.
伝統的に、これは人間の監督と考えることができます。
So this dotted line, that's sort of the human level intelligence, right?
この点線は、人間レベルの知能のようなものですね。
So the supervisor, the smart human, is teaching the AI that is not as smart as the human.
つまり、スーパーバイザー、賢い人間が、人間ほど賢くないAIに教えているわけです。
And then, Super alignment, how that would look like is this idea that this human, who is not as smart as the super intelligent AI, you know, trying to teach it and tell it what to do.
そして、スーパーアライメントとは、スーパーインテリジェントAIほど賢くない人間が、AIに何をすべきかを教え、指示するというものです。
This is what a lot of people have concerns with, is like how do you control something that is far smarter than yourself?
これが多くの人々が心配していることであり、自分よりもはるかに賢いものをどのように制御するのかということです。
And their approach is, we start right now before they get past the human level of intelligence.
そして彼らのアプローチは、彼らが人間の知能レベルを超える前に、今すぐ始めるというものだ。
Are we able to train a smaller model to supervise a larger model?
より大きなモデルを監督するために、より小さなモデルを訓練することはできるだろうか?
And so, at the end, at the very end of this paper, this is like page 47 out of 49, they have this high-level plan.
そして、この論文の一番最後、49ページ中47ページ目に、このハイレベルな計画がある。
So, Leike and Sutskever proposed the following high-level plan, which they've adopted.
そこで、ライカとサツキヴァーは次のようなハイレベルなプランを提案し、彼らはそれを採用した。
So, once we have a model that is capable enough that can automate machine learning like AI, almost training other forms of AI, more advanced forms of itself.
AIのような機械学習を自動化するのに十分な能力を持つモデルができれば、他の形態のAI、より高度な形態のAIをほとんど訓練することができる。
And so, once it's able to automate that, and in particular alignment research, our goal will be to align that model well enough that can safely and productively automate alignment research.
特にアライメント研究を自動化できるようになれば、私たちの目標は、アライメント研究を安全かつ生産的に自動化できるように、モデルを十分にアライメントさせることです。
So, we're trying to create an AI that will be able to research how to create safe AI as it gets sort of stronger and better and more beyond our understanding.
つまり、私たちは、AIがより強く、より良くなり、私たちの理解を超えていくにつれて、安全なAIの作り方を研究できるAIを作ろうとしているのです。
And so, we will align this model using our most scalable techniques available: our lhf reinforcement learning, human feedback, constitutional AI, scalable oversight, adversarial training, and this new approach, the focus of this paper, weak to strong generalization techniques.
そのため、私たちは最もスケーラブルな技術を駆使してこのモデルを調整します。私たちのLhf強化学習、人間のフィードバック、憲法AI、スケーラブルな監視、敵対的訓練、そしてこの論文の焦点である新しいアプローチ、弱から強への汎化技術です。
We will validate that the resulting models align using our best evaluation tools available, for example, red teaming.
私たちは、例えばレッド・チーミングのような、利用可能な最高の評価ツールを使って、得られたモデルが整合していることを検証する。
So, that's when a group of people try to do their best to try to break that model, to try to get it to do something bad, and interpretability, which is our attempt to kind of understand what it's thinking, what its thought processes are.
だから、人々がそのモデルを破ろうとするために最善を尽くすのが、それを悪いことをするようにするために、それが何を考えているのか、どのような思考プロセスを持っているのかを理解しようとする試みであるということです。
Can we monitor its thoughts somehow?
どうにかしてその思考を監視できないだろうか?
And then they're saying number four, using a large amount of compute, we will have the resulting model conduct research to align vastly smarter superhuman systems.
そして4番目は、大量の計算機を使って、その結果得られたモデルに、より賢い超人的なシステムを構築するための研究を行わせるというものだ。
We will bootstrap from here to align arbitrarily more capable systems.
私たちはここからブートストラップして、より能力の高いシステムを任意に調整します。
So, and we've talked about this before, so that there's this idea that we can take these smaller models and by using just vast quantities of compute, almost get a glimpse into what that model would look like if it was much stronger.
つまり、以前にもお話ししたことがありますが、このような小さなモデルを、膨大な量の計算機を使うことで、そのモデルがもっと強くなったらどのようになるかを垣間見ることができるのです。
And of course, that costs a lot of money.
もちろん、それには莫大な費用がかかる。
You need to have a lot of equipment to be able to do that.
それを行うためには、多くの機器が必要です。
But it seems to be like almost a way to get those high-level answers without necessarily creating a model that is that high level.
しかし、必ずしもそのようなハイレベルなモデルを作らなくても、ハイレベルな答えを得ることができるようだ。
We're able to match the capabilities of a much bigger model with a smaller model by, you know, overclocking it, giving it more compute, you know, expanding more resources on that model.
私たちは、オーバークロックをかけたり、より多くの計算能力を与えたり、より多くのリソースをそのモデルに拡張したりすることで、より大きなモデルの能力をより小さなモデルに適合させることができるのです。
So, it's interesting because we've heard some of the stuff being discussed before, not officially, but it seems like certain leaks, certain hints at what's happening, these ideas have been out there before.
ですから、以前から議論されていることを耳にしたことがあり、公式なものではありませんが、ある種のリークや、何が起こっているのかのヒント、こうしたアイデアは以前からあったようで、興味深いものです。
Runway ML
Runway ML。
So you've probably seen some of the videos this thing is able to generate.
このシステムが作り出すビデオのいくつかは、もうご覧になられたかもしれませんね。
So, it's one of the more well-known AI video generation platforms, and what they're announcing is that they're starting a long-term research effort around what they call General World Models.
それで、それはよりよく知られたAIビデオ生成プラットフォームの一つであり、彼らが「General World Models」と呼ぶものについての長期的な研究努力を開始すると発表しています。
And we talked about this idea before, that AI, these neural nets that they build, these mental models of the world, so when we feed them information.
そして、以前にもこのアイデアについて話しましたが、AI、つまり彼らが構築するこれらのニューラルネットは、世界のメンタルモデルを持っているので、私たちが情報を与えるときに。
And then, we ask them to make certain inferences, certain guesses about what's going to happen.
そして、何が起こるかについて特定の推論や推測をするように求める。
So, for example, if we feed them tons of text, then we ask them to predict what comes next in a sentence.
だから、例えば、私たちが彼らにたくさんのテキストを与えて、次に何が来るかを予測するように頼むとします。
Or, we feed them a bunch of images and then we have them either recognize certain images or create certain images.
あるいは、画像を大量に与えて、特定の画像を認識させたり、特定の画像を作成させたりする。
What we find is they build these sort of mental models about how to do that.
そうすることで、彼らはある種のメンタル・モデルを構築するのです。
So, for example, if you give them a lot of 2D images, some of the latest research is showing that they build almost like a mental model of the 3D World.
例えば、2次元の画像をたくさん与えると、3次元世界のメンタルモデルが構築されることが最新の研究で明らかになっています。
So, they kind of start understanding the 3D space and those 2D images.
だから、彼らは3D空間とそれらの2D画像を理解し始めます。
We didn't give them any data about like the depth of field or, you know, how far away certain things are.
被写界深度や、あるものがどのくらい遠くにあるかといったデータは与えていない。
But they do start to gain some quote unquote understanding about how to position objects in the 3D space, what's further away, what's closer, etc.
しかし、3D空間の中でどのようにオブジェクトを配置するか、何がより遠くで、何がより近いか、などといったことを理解し始める。
And so, the fact that Runway ML is doing that is very interesting.
だから、Runway MLがそれをやっているという事実はとても興味深い。
So, they're saying to build General World Models, there are several open research challenges that we're working on.
General World Modelsを構築するためには、私たちが取り組んでいるいくつかの未解決の研究課題があります。
For one, these models will need to generate consistent maps of the environment and the ability to navigate and interact those environments.
ひとつは、これらのモデルが環境の一貫したマップを生成し、それらの環境をナビゲートし、相互作用する能力を生成する必要があるということです。
They need to capture not just the Dynamics of the world, but the Dynamics of its inhabitants, which involves also building realistic models of human behavior.
また、世界のダイナミクスだけでなく、そこに住む人々のダイナミクスも捉える必要があり、これには現実的な人間の行動モデルの構築も含まれます。
So, to me, it almost sounds like what they're talking about is having these AIS build almost a simulation of the world.
だから、私には、彼らが話しているのは、これらのAIが世界のシミュレーションをほぼ構築するということのように聞こえます。
And then, within that sort of simulation of the world, almost like taking a camera and recording something.
そして、その世界のシミュレーションの中で、カメラを使って何かを録画する。
And then, that becomes the video that then is extracted and becomes this AI video.
そして、それがビデオになり、それが抽出されてこのAIビデオになるんです。
So, currently, they have their Gen-2 system, which I've showcased some of the stuff that you can do with it.
現在、彼らはGen-2システムを持っていて、私はそれでできることのいくつかを紹介しました。
They're saying in order for Gen-2 to generate realistic short videos, it has developed some understanding of physics and motion.
Gen-2がリアルなショートビデオを生成するためには、物理学とモーションをある程度理解する必要がある。
However, it's still very limited in its capabilities, struggling with complex camera or object motions, amongst other things.
ただし、その能力はまだ非常に限定的であり、複雑なカメラや物体の動きに苦労しています。
If it wants to generate an image of a bird flying, it has to have some understanding of how that bird moves, the physics that are interacting with it, how the point where the camera is, how it's picking up the movement of that bird, etc.
もし鳥が飛んでいる画像を生成したい場合、それはその鳥がどのように動くか、それと相互作用している物理法則、カメラの位置、鳥の動きを捉える方法などについての理解を持っていなければなりません。
Very excited to hear where they're going to be going with this, and I'm glad more companies are investing resources into this.
彼らがこれにどこまで進むのかを聞くのがとても楽しみであり、他の企業もこれにリソースを投資していることに喜んでいます。
And this piece of news was very interesting.
そして、このニュースはとても興味深かった。
So, OpenAI is partnering with some news outlets to sort of pull real-time information from them.
OpenAIはいくつかの報道機関と提携し、そこからリアルタイムの情報を引き出している。
So, when you ask ChatGPT for real-time news, it's able to pull from those news sources.
ですから、ChatGPTにリアルタイムのニュースを求めると、それらのニュースソースから引き出すことができるのです。
And then, present those to you in real time.
そして、それをリアルタイムで表示します。
And then, of course, they'll include attribution and links to full articles for transparency, etc.
そしてもちろん、透明性を高めるために、帰属表示や記事全文へのリンクなどが含まれます。
This might be how we get our news in the future.
これが、私たちが将来ニュースを入手する方法になるかもしれない。
We're not going to go to Google or the newspaper or TV or even a specific website.
私たちはGoogleや新聞、テレビ、あるいは特定のウェブサイトを見るつもりはない。
We're just going to ask our favorite chatbot, Hey, what's the news today?
ただお気に入りのチャットボットに、「ねえ、今日のニュースは何?
Midjourney Alpha
Midjourney Alpha。
Midjourney launchs a brand new thing.
Midjourneyが全く新しいものを発表しました。
Midjourney Alpha, we're able to create stuff faster, easier.
Midjourney Alphaでは、より速く、より簡単にものを作ることができます。
It breaks it down by subjects, descriptors.
題材や説明によって分類される。
You can have it look like known artists, etc.
既知のアーティストのように見せることもできる。
So, this is kind of the next step in AI art generation that gives you a lot more control and more precision in how to create your images.
つまり、これはAIアート生成の次のステップのようなもので、イメージの作成方法をより正確にコントロールできるようになります。
If you've generated, I believe it's over 10,000 images with Midjourney, this should be available to you soon.
Midjourneyで10,000枚以上の画像を生成していれば、すぐに利用できるようになるはずだ。
Google Deep Mind drops Imagen 2, their most advanced text-to-image technology, and some of these images are extremely realistic.
Google Deep Mindが、これまでで最も高度なテキストから画像への変換技術であるImagen 2をリリースし、その中には非常にリアリスティックな画像も含まれています。
I think I would be hard-pressed to find any faults with it.
私はそれについて何の欠点も見つけるのは難しいと思います。
I mean, there's certain things that I could point to, maybe this area is a little bit weird, kind of the area here, but I could not call this an AI-generated image.
つまり、この辺りがちょっとおかしいとか、この辺りがちょっとおかしいとか、指摘できる点はあるが、これはAIが生成した画像とは呼べない。
There's nothing here to really give it away.
しかし、これはAIが作成した画像とは呼べません。
So, that's it for today, but I think there's a lot more to come.
それで、今日はこれで終わりですが、まだまだこれからたくさんのことがあると思います。
I think this is kind of the calm before the storm.
これは嵐の前の静けさのようなものだと思う。
I think before December is out, we're going to see some pretty incredible things, just a hunch.
12月が終わる前に、私たちはかなり信じられないことを見ることになると思います、ただの予感ですが。
My name is Wes Roth, and thank you for watching.
私の名前はウェス・ロスです、ご視聴ありがとうございました。
この記事が気に入ったらサポートをしてみませんか?